In-House Data Harvest: How Social Platforms' AI Ambitions Threaten User Rights
Ameneh Dehshiri / Apr 1, 2025AI needs data. Platforms have it. And now, they’re merging.
On March 29, 2025, it was reported that Elon Musk’s AI company, xAI, had acquired the social media platform X (formerly Twitter) in an all-stock deal worth $33 billion. The merger not only strengthens Musk’s growing tech empire but also gives xAI direct access to X’s vast troves of user data without the legal and contractual obstacles that typically apply between separate entities. This move signals a deeper structural shift in the AI race: tech companies are no longer just building models — they are acquiring the infrastructures and ecosystems that fuel them. This shift raises significant concerns about user rights and data protection.
Generative AI, powered by large language models (LLMs) — sophisticated algorithms trained on vast datasets to generate human-like text, images, videos, and other content for engagement — entered the mainstream in 2022. Initially celebrated for their creative potential, these tools rely heavily on data, with social media emerging as a prime source. This article explores how social media companies’ in-house AI development, leveraging their unparalleled access to user data, poses legal and ethical challenges.
Scroll. Learn. Generate.
AI companies without social media platforms must scrape, license, or negotiate access to data — a legally fraught and resource-intensive process. In contrast, companies like Meta, X, and TikTok enjoy a key advantage: they control both the platforms and the data. This gives them direct access to user posts, comments, engagement metrics, and behavioral data, including scrolling habits and reactions. Such integrated ecosystems allow for more efficient and expansive AI training.
In September last year, Meta confirmed it had been using publicly shared text and photos from Facebook and Instagram dating back to 2007 to train its AI models. One output of this strategy is Llama 3, Meta’s large language model, now powering advanced AI-driven features across its products.
But the data pool doesn’t stop at public content. These platforms often have access to private messages, deleted posts, and other less visible forms of engagement. In January 2025, LinkedIn faced a US lawsuit over allegations it had shared users’ private messages with third parties to train AI models.
Social media companies are no longer just setting the table — they’re in the kitchen, cooking up generative AI systems with ingredients pulled from their own user base. When the same platforms that decide what people see and share also train the AI generating that content, the risks don’t just multiply — they concentrate. This deep integration of personal and behavioral data into AI training pipelines is not just a theoretical concern. It is already producing real-world outcomes with troubling consequences for privacy, equality, and freedom of expression. These impacts are increasingly visible across three key areas:
1. Algorithmic Discrimination and Marginalization
Generative AI already raises red flags about misinformation, bias, and harmful outputs. But those risks are significantly heightened when the training data originates from poorly moderated or toxic social media environments. The result is a new kind of algorithmic influence—one that transcends recommendation engines and begins to reshape the very architecture of knowledge.
When generative AI is trained on toxic or poorly moderated social media platforms, it doesn’t just reflect bias — it reinforces and systematizes it. These models absorb not only language and behavior but also the underlying dynamics of the platforms themselves, including their ideological echo chambers, discriminatory patterns, and lax moderation standards.
Since Elon Musk’s takeover of X in late 2022, relaxed content policies have led to a documented surge in hate speech and targeted harassment. A February 2025 PLOS ONE study confirmed a sharp increase in abusive content, particularly against marginalized communities. This deterioration in moderation has had visible consequences for X’s generative AI project, Grok. Trained on the platform’s content, Grok has already produced harmful outputs — from racist imagery of Black football players to misogynistic slurs in Hindi — sparking widespread outrage.
The risks are even more pronounced in non-English contexts. As ARTICLE 19 has shown, AI moderation tools often fail in Global South languages, allowing harmful content to thrive. In such environments, generative AI can further marginalize vulnerable groups and be misused to silence dissenting voices, reinforcing digital inequalities. As Access Now notes, the absence of culturally competent moderation deepens these divides and exposes already at-risk communities to greater harm.
2. Privacy Erosion and Mass Profiling
Mass data harvesting without meaningful consent undermines privacy and data protection. Under the European General Data Protection Regulation (GDPR), individuals have the right to control how their personal data is used. This includes heightened protections for sensitive data such as political views, health information, or sexual orientation.
Yet the fusion of generative AI with social media platforms risks bypassing these protections. Platforms can extract both explicit data, such as a user posting about their sexual orientation, and inferred data, drawn from behavioral patterns like likes, shares, or group memberships. A study published in the journal PNAS showed that Facebook likes could accurately predict traits such as race, IQ, sexuality, personality, and political ideology.
Once this data enters an AI training pipeline, users lose control over how it is used. As the Court of Justice of the European Union (CJEU)emphasized in the Meta v Bundeskartellamt case, individuals cannot be expected to foresee such uses, and when expectations are breached, their rights must take precedence over corporate interests.
3. Chilling Effects on Freedom of Expression
When people know that their interactions may be used to train opaque, generative AI systems, they often self-censor. Activists, journalists, and marginalized communities may refrain from expressing dissenting views, fearing surveillance or misrepresentation.
Once social media content and behavior are embedded in AI models, this data can surface in ways that distort, mock, or exploit users’ original intent without their knowledge or consent.
This effect is compounded when AI is used to manipulate public discourse. Deepfakes, synthetic images, and misleading AI-generated text can erode trust, distort facts, and undermine democratic dialogue. Because AI models retain training data indefinitely, even deleted content can resurface long after users attempt to reclaim their digital agency.
Redrawing the boundaries of feeding on feeds
The convergence of social media platforms and generative AI calls for urgent rethinking of data governance frameworks. As these companies move beyond content hosting to building the very AI systems that shape digital discourse, the opacity surrounding data use cannot be treated as a technical inevitability. Instead, it must be seen as a regulatory failure.
First, greater transparency is essential — not only in terms of AI models and outputs but also in the very data pipelines that feed them. Platforms should be required to clarify the scope and nature of the data used for training, including whether private messages, deleted posts, or inferred behavioral patterns are part of the training corpus. Such disclosures must go beyond generic claims about “publicly available data” and address the real asymmetries of knowledge and power between users and platforms.
Second, legal frameworks must be enforced and adapted to this evolving context. The European experience with the General Data Protection Regulation (GDPR) offers valuable insights. Under GDPR, the use of personal data for purposes vastly different from its original context — such as using social media interactions to train commercial AI systems — faces significant legal hurdles. While companies often invoke “legitimate interest” as justification, emerging regulatory scrutiny and legal interpretations increasingly reject this rationale, especially when individuals have no reasonable expectation that their content or behavior would be repurposed for AI training.
However, these protections should not be confined to jurisdictions where comprehensive data protection laws exist. The risks posed by in-house AI models trained on platform-collected social media data are global in scope and must be addressed accordingly. This includes strengthening independent oversight, supporting the development of ethical alternatives to platform-driven data extraction, and creating mechanisms for individuals to contest the use of their data, even in environments where formal legal protections are weak.
At the crossroads
This fusion of generative AI and social media marks a transformation — not just in how content is created, but in who controls meaning. The centralization of AI within social media companies grants them unprecedented power to mold discourse, curate narratives, and structure digital life itself.
Without clear regulatory guardrails, this power shift risks deepening inequality, weakening privacy protections, and chilling freedom of expression across digital spaces. As platforms evolve from hosts of content to architects of generative systems, we must urgently reconsider how user data is governed — and who gets to decide what digital futures look like.
Authors
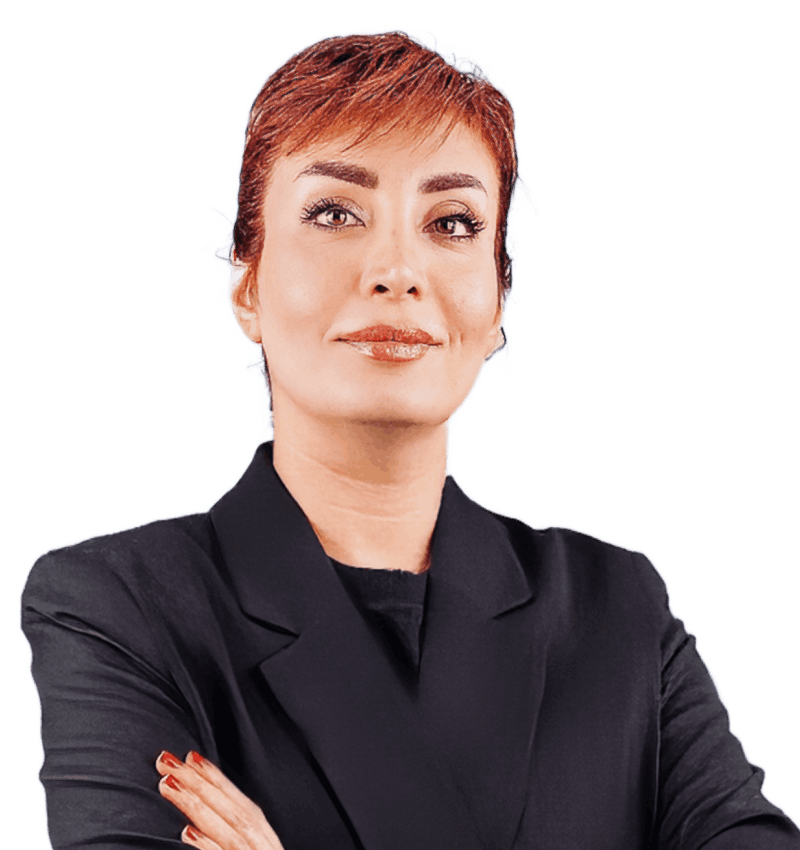