Dismantling AI Data Monopolies Before it’s Too Late
Courtney Radsch / Oct 9, 2024Courtney C. Radsch is director of the Center for Journalism and Liberty at the Open Markets Institute and a nonresident senior fellow at Brookings, the Center for International Governance Innovation, and the Center for Democracy and Technology, and serves on the board of Tech Policy Press.
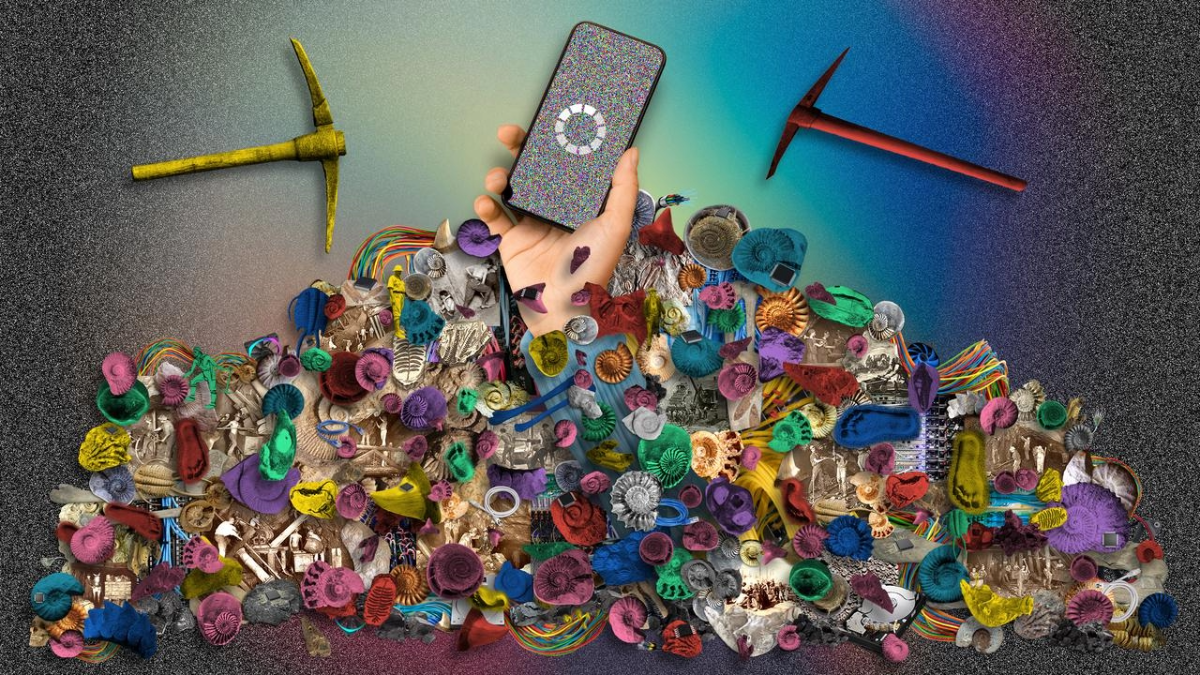
Luke Conroy and Anne Fehres & AI4Media / Better Images of AI / Models Built From Fossils / CC-BY 4.0
Less than two years after ChatGPT turned AI into a topic of dinner table conversation and propelled it to the top of the policy agenda, it is clear that AI is further concentrating power in a handful of Big Tech firms and their “partners” who have access to the building blocks needed to develop and deploy the most advanced generative AI systems: data, compute, and talent. The unprecedented amounts of data needed to train foundation models and make them capable of everything from writing poetry to diagnosing cancer is entrenching the power of existing tech gatekeepers while creating a potentially insurmountable advantage to incumbents.
Data is a defining characteristic of generative AI and critical to consideration of market power and dominance. As sophisticated prediction engines, the defining characteristic of generative AI is data, whereas compute and talent are factors that “apply more generally in industrial organization and antitrust” and are thus relevant to many fields. General purpose AI models, often called foundation models, were created and trained on vast amounts of data to learn and predict patterns, grammar, and context to generate coherent and contextually relevant content, while smaller models and the processes that make consumer-facing chatbots and information retrieval work also rely on access to real-time and high quality data.
Big Tech firms have access to vast troves of data to develop, train, fine-tune, and ground their AI models and the deep pockets to fend off lawsuits while indemnifying their customers for potential intellectual property claims in an effort to encourage wider adoption and integration of their GAI products.
The structural advantages these players enjoy, in addition to anti-competitive practices such as self-preferencing and tying, have put up huge barriers to entry for newcomers to the AI market or those who want to play fair. Late entrants are also running into obstacles acquiring usable data–giving Big Tech an enormous advantage, since the first movers have already scraped vast amounts of data and have access to proprietary datasets.
Most of the data used by AI systems thus far was culled from content on the internet, including Wikipedia, social media sites like Reddit, Twitter, and YouTube, and publishers of all types, as well as digital databases of books, code, patents, and other content repositories. Much of this “public” online content, however, was protected by copyright or provided under specific conditions of use, such as payment or attribution, including publishers with licensing mechanisms and many Creative Commons licenses that limited commercial use.
More than 30 lawsuits against AI companies have been filed, primarily in the US, for scraping content from websites across the internet without permission from, or compensation to, rights holders. But while the lawsuits meander through court, data is running out and the extractive practices of AI companies continue, entrenching the power of Big Tech firms and raising serious concerns about data monopolies.
AI researchers are learning that high-quality data is becoming more important even as it becomes less prevalent. Experts estimate that publicly available human-generated text online could run out as early as 2028 even as the preponderance of synthetically generated content could constitute upwards of 90 percent of internet content within just a couple of years. As more and more publishers, social media sites, and other content repositories block AI web crawlers or put up paywalls, the availability of quality content on the open web is declining while the proportion of low-quality, AI generated, junky content increases.
“Data quality seems to matter a lot,” said Jaime Sevilla, director of the research institute EpochAI, which has conducted studies into the availability of data for training LLMs. “I do suspect that a lot of the compute efficiency gains that we have seen in the last seven years or so are to one extent or another linked to the quality of data.”
The decline in human-generated content is problematic because without access to high-quality data, models degrade and even collapse. And even if smaller models built on far less much higher data (and with a much smaller carbon footprint) could achieve similar capabilities to their gargantuan rivals, as some research suggests might be possible, the need to obtain high quality data could intensify. “More data beats better algorithms, but better data beats more data,” renowned computer scientist Peter Norvig is credited as saying.
Furthermore, feeding AI models with more relevant, timely or accurate data – as opposed to vast quantities of data – is what makes a slew of consumer-facing applications like chatbots and generative search work.
This means that securing access to high-quality data is existential to the continued viability and safety of generative AI, from foundation models to a host of downstream applications, and could make access to proprietary data even more important, giving Big Tech companies and their AI partners a potentially insurmountable advantage.
Companies that have developed foundation models trained on unpolluted data also enjoy a first-mover advantage that is reinforced by the deep pockets needed to battle with lawsuits from rights holders while mining their own products and services for data. And since many of the dominant AI companies are already powerful incumbents in a range of digital services and products, they sit on top of massive archives and enjoy a regular stream of fresh data created by the millions of users across their platforms.
The online data shortage thus has significant implications for the power of Big Tech and reinforces the advantages of dominant incumbents in three main ways: proprietary data, including user-generated content and interactions across lines of business; data partnerships and permissionless scraping; and synthetic data.
As the research institute AI Now observed, corporations with the “widest and deepest” data advantages will be able to “embed themselves as core infrastructure” and become indispensable and irreplaceable in the AI ecosystem. That is why frontier AI companies are focused on securing access to data to maintain a competitive advantage that can further cement their dominance throughout the AI value-chain and ensure that the business models fueling the AI revolution continue. Whether they do this by obtaining human-generated data or making their own, the dynamics of data in the current environment further privileges Big Tech firms and entrenches their positions in the AI market.
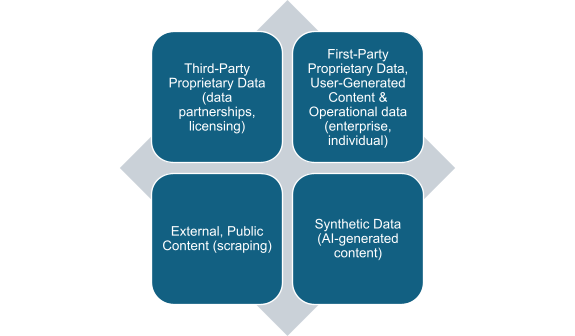
Data sources for generative AI systems.
First-party proprietary data
Tech giants have the advantage of being able to train their models on both the open web and their own vast proprietary datasets, giving them a colossal advantage over those who must rely only on the former. Data derived from a corporation’s other product lines, such as user-generated content, confidential and private data, metadata, archives, and other digital exhaust that can be analyzed with machine learning provide a competitive advantage that the biggest and most dominant AI firms are best placed to benefit from. Even where access to data is comparable, these dominant corporations control far greater computing capabilities and access to scarce advanced chips, enjoy better access to specialized expertise, and have developed vast experience in scraping, labeling, and analyzing data.
Generative AI models will increasingly train on proprietary data as publicly available data becomes scarcer, copyright uncertainties continue, and new techniques for machine learning make first-party data insights more valuable, further reinforcing the advantages that companies with reservoirs of proprietary data already enjoy. Tech monopolies also hold an advantage in more specialized AI development through their ownership of personalized, specialized datasets in areas such as health, finance, and transport, as with Google’s ownership of mapping and Fitbit data, or Amazon’s access to sensitive health data via its recent acquisition of One Medical.
The growing interest in proprietary data has been evident over the past several months as Big Tech companies have quietly granted themselves permission to scrape the content and mine the data created by users of their products.
Google uses data from Search and “public” content like reviews and videos, amassed through its various business lines, along with publicly available user information and content to help train Google’s AI models. The company also owns YouTube, the most extensive reservoir of imagery, audio and text transcripts in the world, with more than 500 hours of video uploaded to YouTube every minute, often with accompanying descriptions and transcription. If each second of video corresponds to about 30 tokens of text, the data created on YouTube each hour could amount to 54 million tokens, meaning that it would take less than a year to generate the equivalent of the entire corpus of publicly available text online, which currently stands at 300 billion, according to EpochAI. The richness of YouTube’s multimedia content and accompanying textual descriptions make it a valuable source of training data for large language and multimodal AI models, like Gemini, and could give Google a leg up on its rivals. Meta uses public Instagram and Facebook text and video posts to train their models, and Microsoft, which owns LinkedIn and a reported 49 percent of OpenAI, is likewise hoovering up LinkedIn content to train its AI systems. X, formerly Twitter, is sharing user data with Elon Musk’s xAI company to train its AI model, Grok.
Meanwhile, these same companies have sought to restrict the use of their data by rivals. As X was granting itself the right to use user data for its own AI training, it also added in prohibitions on crawling or scraping “in any form, for any purposes” without prior written authorization and threatened Microsoft with a lawsuit for “illegally using Twitter data.” Reports that OpenAI, Anthropic, Apple, and Nvidia all used YouTube content to train their AI models came as YouTube’s CEO warned that the platform’s terms of service do not allow transcripts and videos to be downloaded for training data. And although the EU’s data privacy law has led several of these companies to limit scraping content of users in that region, these opt-outs are limited to that jurisdiction and do not necessarily apply retroactively.
The users of the AI products created by these companies also provide useful training data, from the prompts and feedback generated through their chatbots to data generated by users of their cloud services and enterprise products. These feedback loops could become increasingly important if foundation models are able to learn from them and incorporate this feedback. These companies are also able to integrate their AI models across their various platforms and services, from search and social media to productivity and enterprise products.
As a result, incumbents have both an advantage in both data depth and breadth and can restrict access by competitors or prevent from using those data sources to train their own AI systems. The biggest and most popular platforms thus enjoy a steady stream of new training data even as they sit on massive archives of user-generated and proprietary data produced over the years, an advantage that competitors would be hard pressed to match.
Third-party proprietary data: data “partnerships” & “permissionless” access
Tech corporations with deep pockets are also best positioned to coerce and cajole publishers, record labels, social media companies, and content creators to strike deals, but are free to pick the winners and losers and often include anticompetitive practices such as tying, bundling, and exclusive dealing. The terms of these deals and ambiguously termed “partnerships” often include not just access to data and quality content, they also include cloud exclusivity, API credits, integrations that provide training, alignment and learning opportunities that provide real-time feedback to their AI systems. The risk that smaller firms are unable to compete to offer similar terms, or due to exclusivity or inadequate bargaining power further risks consolidating the dominance of Big Tech and their AI progeny.
Data partnerships thus have the potential to reinforce monopolistic dynamics in other parts of the AI tech stack, specifically with respect to cloud, contributing to a mutually reinforcing monopoly broth. Big Tech partnerships with AI unicorns – startups worth more than a billion dollars -- often include tying requirements and exclusivity provisions. For example, Microsoft’s “partnership” with OpenAI makes it the company’s exclusive cloud provider, meaning that a slew of other deals that include newsroom integration and API credits also tie those publishers to Microsoft’s Azure cloud and thus also reinforce Microsoft’s dominance in AI and potentially enhance their power to secure direct data deals themselves.
OpenAI has signed more than a dozen licensing deals, including one with NewsCorp worth an estimated $250 million that gives OpenAI access to real-time news and archives in exchange for cash and API credits, while its deals with AP and Time include access to their archives as well as newsroom integrations likely to provide useful training and alignment for its AI systems. Reddit’s $60 million deals with both OpenAI and Google provide access to a regular supply of real-time, fresh data created by Reddit’s 50 million users while locking in Reddit’s use of Google’s VertexAI cloud and OpenAI as an “advertising partner.” And only Google’s search engine currently has access to Reddit, which has blocked other crawlers from indexing its site, giving it exclusive access to what is seen as one of the most valuable sites on the internet due to its robust community of human commentators.
Synthetic data
Synthetic data that is artificially created to mimic real-world data can supplement existing data resources, allowing models to scale and improve more rapidly by mitigating training data shortages, and can be used in various machine learning tasks. Synthetic data can avoid some of the privacy pitfalls of training on real-world data and could mitigate some of the IP and bias concerns involved in using real-world data.
AI researchers seem hopeful that they will solve the model collapse issue to find a way to ensure the integrity of AI models trained on synthetic data. Sevilla said that this is the main focus for many in the field as the data bottleneck becomes more acute. Some in the field suggest that synthetic data could end up completely overshadowing real data, especially if self-improvement solutions, known as self-play in reinforcement learning, advance, allowing AI models to learn on their own when they produce poor outputs and improve themselves through reinforcement learning without needing to rely on human feedback.
“I expect that all major companies right now are training on synthetically generated math and coding problems," explained Sevilla, since answers in those domains can be automatically verified. Extending this to more qualitative and subjective domains is more challenging, but many of the leading AI firms are already at the forefront of using and investing in synthetic data research and use.
While the science of creating feedback loops that automate reinforcement learning remain unproven, the leading AI companies are heavily invested in trying to solve for this bottleneck and are best placed to reap the benefits if they can turn theory into practice.
Policy solutions to mitigate anti-competitive dynamics
Policymakers and law enforcement agencies can mitigate many of the problematic impacts on competition posed by the current trajectory of AI development by using their existing authorities and taking a more proactive approach to ensuring that innovation occurs through fair competition. They have the authority to investigate many aspects of these emerging data monopolies, and they should act swiftly to ensure that anticompetitive conduct and privacy violating practices do not further empower dominant players or entrench problematic data collection practices.
Competition authorities should probe data supply chains, and examine whether the use of proprietary data and data collected via different product lines and services to determine if they violate antitrust laws, and whether the unlicensed and/or uncompensated use of copyright protected materials constitutes misappropriation or unfair market power. They should also use existing antitrust law to investigate how existing data partnerships operate in practice and be as robust in monitoring these types of deals as they have been in reviewing potential mergers and acquisitions and cloud partnerships.
Privacy laws can also help mitigate this unfair and unsafe competitive advantage by prohibiting such practices or making them too risky to pursue. X/Twitter, Meta, and LinkedIn are no longer training their AI systems on user data collected in Europe, for example, where the General Data Protection Regulation (GDPR) provides a minimum level of privacy protections for individuals and their data and requires opt-in consent. The Dutch Data Protection Authority has already indicated that scraping social media posts, even if they are publicly available, is not permitted under the GDPR. But in Australia and the US, for example, which lack similar (or any) data protections, these companies have given themselves the right to harvest data from accounts and posts not set to private and may not even offer an opt-out option.
Countries with data protection agencies should investigate whether data privacy laws have been violated and maintain vigilance on unilateral updates to terms of service or cross-platform data-sharing provisions within the same company. Jurisdictions without existing privacy laws should follow the lead of GDPR and ensure that privacy regulations are designed to be opt-out as the default setting for user-generated content and personal data use for AI training as opposed to opt-in. Bans on data processing should be imposed where violations take place, and restrictions on international data transfers to jurisdictions without robust privacy protections, such as the US, should be considered.
As the debate over whether the unfettered seizure of the creative and intellectual labor of humanity is legal will take years to play out, during which time AI companies are pushing full steam ahead with their business models built on permissionless scraping that will provide an increasingly unfair competitive advantage while creating facts on the ground that will make it difficult to pursue a different approach.
Competition and data protection agencies must act now to leverage their existing authorities to prevent Big Tech from further entrenching their dominance and coordinate to ensure that their investigations mitigate anticompetitive practices that not only further entrench their power but also undermine fundamental human rights. Data monopolies are an increasing concern in the age of generative AI, and dominance in the realm of data should be dealt with as robustly as any other monopoly. Unless we dismantle these data monopolies and encourage practices that protect privacy and competition, innovation will be little more than a hallucination that benefits dominant incumbents at the expense of citizen and consumer welfare, choice, and rights.
The author wishes to thank the participants of the SSRC Data Fluencies workshop for their thoughtful feedback on an earlier version of this article.
This piece was updated on October 10 to clarify a quote from Jaime Sevilla.
Authors
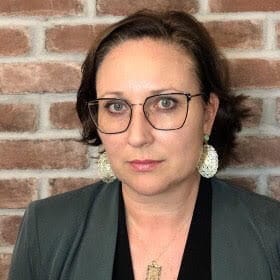