Lawmakers Push for AI Labels, But Ensuring Media Accuracy Is No Easy Task
Claire Leibowicz / Sep 9, 2024AI labels will not solve "trust in media" challenges. They need to be democratized to do so, writes Claire Leibowicz, head of AI and Media Integrity at the Partnership on AI.
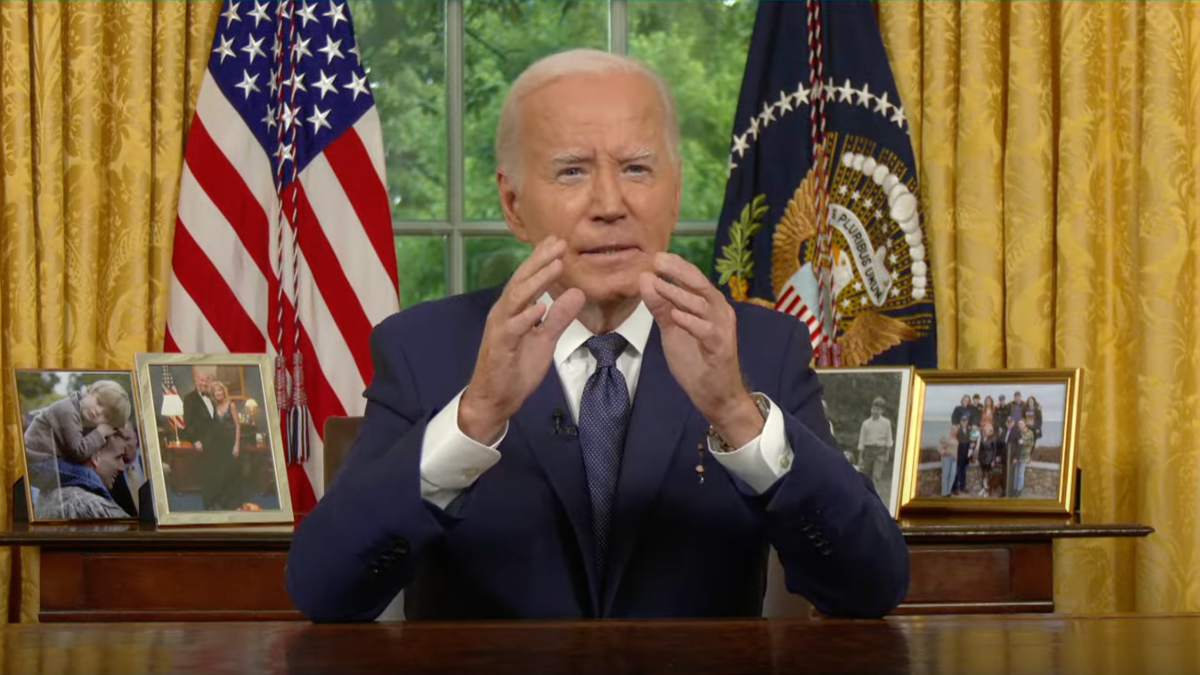
A July 14, 2024 White House address by President Joe Biden was manipulated and posted to X by the operator of a meme account.
When President Joe Biden announced that he was ending his campaign for re-election with a letter posted on X, conspiracy theories about the authenticity of his signature swirled.
The next day, a deepfake depicting Biden cursing about his opponents, alongside a fake PBS NewsHour label, circulated widely. Commenters on X questioned the artifact’s authenticity. PBS, posting from its official channels, helped alleviate the uncertainty, clarifying that the broadcaster does “not condone altering news video or audio in any way that could mislead the audience.”
Manipulating videos and casting doubt on real content are not new phenomena. Yet AI technologies have made it easier to mislead, amplifying existing challenges to trust and truth. AI not only provides cheap and accessible tools for creating realistic content (like the PBS deepfake), but also bolsters plausibility for those looking to cast doubt on real artifacts by claiming they are made with AI (like the letter).
In response, policymakers from Sacramento to Brussels have begun to take action. Many rightfully seek to bolster AI transparency, often by proposing labels that convey to users whether a piece of content is AI-generated or modified. They believe that by disclosing that, say, a Biden deepfake was made using AI, audiences will doubt misleading content. As the Federal Communications Commission summed up in its new proposal for disclosing AI-generated political content, “Voters deserve to know if the voices and images in political commercials are authentic or if they have been manipulated.”
But policymakers and practitioners must consider: What do audiences deserve to know? And how, and by whom, should that information be conveyed?
Today’s AI labels are not optimally responsive to the challenges that AI poses to truth and trust online. They must be coordinated to better reflect how audiences actually understand content – not simply based on descriptions of technical changes, but signals of whether or not such changes matter to them. This is especially important as AI-generated content becomes increasingly indistinguishable from human-produced content, and soon, is delivered by agents capable of behaving in human-like ways.
Doing so not only requires answering questions about what, how, and by whom content is labeled. It requires greater exploration of more democratic forms of labeling. Specifically, those distributing, building, and creating AI-generated media must share more data about the impact of content labels on audiences, collaborate with institutions and individuals that are trusted in civic life to improve media literacy, and consider developing infrastructure that supports community-driven labels.
What are we labeling? And how are we labeling it?
“Is it AI or not'' is often the default binary for determining what content should be labeled to promote audience understanding and trust, but it is inadequate. Just because something was made with AI does not mean it is misleading; and just because something is not made with AI does not mean it is necessarily accurate or harmless.
Meta recently experienced the hazards of relying on this simple “AI or not” threshold for labeling firsthand. In April, it announced it would be placing a “Made with AI” label on photos created with AI tools. A few months later, users pushed back against what they saw as punitive labels applied to insignificant edits. Those who used a tool called GenFill for minor edits like expanding backgrounds triggered Meta’s label. In response to the backlash, Meta revised the label to “AI Info” and suggested that it would shift what should be labeled based on assessments of the amount of AI editing.
But focusing on the amount of editing is not the perfect framework for labeling, either. It’s easy to imagine a version of the Biden PBS deepfake where only one word was generated with AI, and if that one word was a profanity, it could still belie Biden’s temperament. One does not need much, or any, AI, to mislead and misrepresent reality.
The Meta example was likely enabled by the organization’s commendable adoption of C2PA, a technical standard that allows content distributors to interpret impartial technical signals baked into the content that flows to their platforms. From such signals, social media platforms can intervene on content, including with labels.
Standardizing the ways technical authenticity characteristics travel across the web is vital, but so too is better standardizing how to convey those and other useful characteristics to audiences. And it must be done in a way that, as research has highlighted, helps them understand the content’s accuracy, evaluate the source, better grasp the context around a piece of content, and most importantly, trust the signals.
It’s wise, and politically pragmatic, for policymakers to develop interventions that avoid placing greater control of content evaluations in the hands of a few social media platforms. That’s why they often focus on the method of manipulation (i.e, technical descriptions of the use of AI) as a proxy for the normative meaning of the manipulation. There is even some evidence that this proxy works: research suggests that labels describing the process by which audio-visual content was edited or generated, like Content Credentials, which have been adopted by LinkedIn, Adobe, and the BBC for audio-visual content, may support audience understanding and trust in media.
However, researchers have also noted the limitations of relying on descriptions of technical changes to obliquely support how audiences understand content. AI labels may drive skepticism about authentic media, diminish trust in truthful content, and also fail to provide utility to communities across cultures and contexts. And ultimately, even when labels reflect technical changes separate from institutional judgments, consumers often evaluate them based upon the individuals and organizations deploying them.
Policymakers must recognize the limitations of labels that indicate the presence or absence of AI. But doing so does not not mean that policymakers should instead give technology companies more control over normative content decisions. To improve audience trust in truthful content, we must design systems and policies that embolden, and make more salient, trusted institutions and individuals who can help audiences make sense of online content in a way that meets them where they are. Improving transparency about label deployment on technology platforms, deepening media and AI literacy as key parts of civic life, and even exploring alternative paths for labeling content are great places to start. And they require collaboration between government, civil society, industry, and academia to succeed.
Share more data about the impact of labels
Those designing generative AI systems and those creating and distributing AI-generated content must share more details about the impact different AI content labeling tactics have on consumers. Doing so would not only enable richer coordination and design of more effective labels, but may also increase trust in those labels.
While some research has been published on how organizations disclose generative AI content to audiences – including a study from Google’s Jigsaw of responses to deepfake labels, a paper from Adobe Research, and the New York Times’ assessments of audience interpretation of content provenance in the journalistic context – detailed reporting on real-world users is not common from those with the most access to the people interfacing with content organically.
At a March 2024 workshop that Partnership on AI hosted with the National Institute of Standards and Technology (NIST), several participants from industry discussed key concerns about AI labels based on their own user research, echoing and amplifying those expressed by many in academia: some questioned what the goal of labeling should be, others described most labels they tested as ultimately doing more harm than good and prompting distrust in accurate content. The sharing, they said, was invaluable. So how can we figure out ways to facilitate even greater openness about such insights?
Policies that support disclosure of AI-generated content must also support transparency and sharing about the sociotechnical impact and effects of such disclosures – providing the field with more consistent metrics for studying the impact of labels, and examples for how they affect users, in practice. Partnership on AI is currently collating case examples for how a variety of synthetic media distributors, creators, and builders labeled synthetic media in specific instances, but voluntary commitments are not enough to ensure the practice becomes frequent and widespread. Policies supporting a coordination and sharing method for considering the impact of labels in practice – akin to centralized databases like the AI Incident Database – can enable development of clearer, more effective, and consistent labels in the future, likely moving faster than university research and more accurately reflecting real world dynamics of platform users.
Develop media literacy efforts drawn from trusted civic institutions, not just platforms
For interventions like AI labels to work, policies must deepen public education via AI and media literacy that is evocative, rigorous, and accessible across demographics. And importantly, while those building generative AI systems and those creating, and distributing content have a role to play in literacy efforts, the public would be better served by organizations already trusted in civic life–like libraries–playing a more central role in media and AI literacy.
This idea emerged prominently in a recently published series of 11 cases from organizations implementing Partnership on AI’s guidance on disclosing misleading AI content. Representatives from several organizations – ranging from the Canadian Broadcasting Corporation to OpenAI to TikTok – explained how labels that are applied to specific artifacts did not just have an impact in that particular instance, but were also related to broader societal attitudes and understanding of AI. Researchers at OpenAI wrote about how any decisions they made about image authenticity labels would exist in a context where policymakers and the public might be overconfident in the accuracy and utility of such signals. Building out deeper audience understanding about the limits of AI labels and how to engage with them will help ensure that AI labels are not interpreted as falsely equating the presence of AI to inaccuracy, or perceived as being applied perfectly to all manipulated content. Policies must also bolster multistakeholder work on the impact of different AI literacy techniques, how they can be mutually reinforcing and coordinated, and the role of different institutions–particularly those already trusted in civic life–for providing such education.
Explore community-driven methods for labeling content
While it is understandable why AI labels appeal to policymakers, since such disclosures avoid centralizing normative content judgments, there are alternative methods for labeling content in the AI age that might better support belief in the integrity of content. Policymakers should explore democratized methods for evaluating and labeling content that may be better trusted by audiences encountering it than technical signals conveyed top-down by often distrusted platforms.
X’s Community Notes is one of the clearest examples of community-driven, bottom up labels for content that provide details about context, source, and other elements that AI labels are also hoping to obliquely describe. Formerly rolled out on Twitter in 2021 as Birdwatch, a cohort of over 100,000 users may comment and annotate potentially misleading content, indicating whether it is likely AI generated or not. And the evaluations get shown to X users if enough raters from different sides of the political spectrum align on their annotations. Of course, Community Notes should not be the only way for X to scan for harmful material, like illegal terrorist content. However, some evidence on its accuracy in fields like health and science shows promise for how social media platforms might reimagine AI labeling. Indeed, there may be ways to label that deal directly with accuracy and the method of manipulation, without concentrating moderation power within companies (who are also often distrusted by audiences).
Academics have also advocated for greater focus on distributed labeling methods, an emphasis on trusted individuals, and for bolstering audience understanding of content in the AI age. TrustNet, a project driven by MIT computer scientists, takes a “trust-centric approach” to content labeling, letting anyone with their browser extension declare what is accurate and inaccurate on the web. Audiences can then see the information that has been verified by people and sources they trust, thereby maintaining user autonomy and increasing the likelihood that audiences may actually update their beliefs.
Embracing technical signals of AI’s presence is not the only way to support truth and trust online while maintaining audience autonomy. Instead, policymakers can consider systems that empower audiences to label themselves and to evaluate content manipulation, accuracy, and context.
Labeling, and enabling, humanity in the AI age
Ultimately, with trust in individuals and institutions so central to audience interpretation of what is fact and what is fiction, it will become increasingly important to ensure that identity is not weaponized in the AI age. This need is amplified by AI innovations which not only make it possible to represent individuals or institutions in content, like the PBS or President Biden himself, but to actually behave and interact like people and organizations – via AI agents.
Such developments make it vital for policymakers to not only consider ways to signal that AI has been used in content generation, but to also develop ways to assert that users on the web – who may soon be difficult to discern from AI agents – have actually been verified as real, without sacrificing privacy and anonymity. And beyond labeling of both AI agents and generated content, policymakers can advocate for regulations that make it harder to represent any person or organization in the first place – which is the premise of the bipartisan NO FAKES Act that is before the US Senate.
Transparency is of utmost importance in an era where it is increasingly easy to misrepresent reality and manipulate the truth. But embracing transparency requires recognizing the ways in which audiences actually make judgments about content and the social dynamics of how people choose to trust information on the web. Only then can AI labels, and related policies, enable human flourishing in the AI age.
Authors
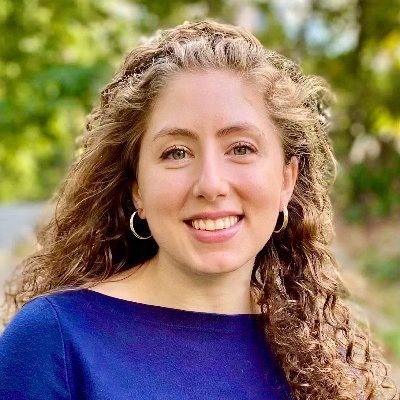