The Right to Be Forgotten Is Dead: Data Lives Forever in AI
Haley Higa, Suzan Bedikian, Lily Costa / May 20, 2025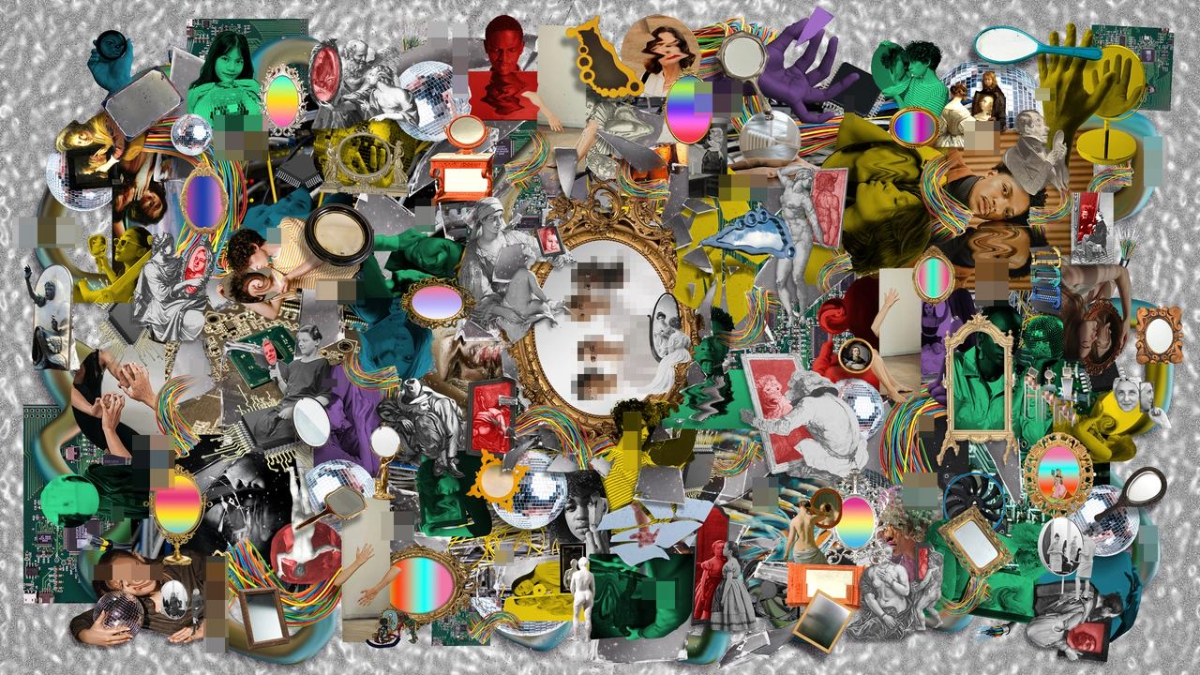
Anne Fehres & Luke Conroy / Better Images of AI / Data is a Mirror of Us / CC-BY 4.0
The internet remembers you, and it may never forget. The rise of generative AI and large language models (LLMs) has thrown a wrench into modern privacy rights, specifically, the right to be forgotten. Originally rooted in the idea that individuals should have control over their personal data, the right to be forgotten gained legal recognition through laws like the European Union’s General Data Protection Regulation (GDPR). If you no longer wanted your information to be publicly available, you could request its deletion. And it was relatively easy to remove information as it applied to discrete data sets, Google search histories, and more.
But in an era where AI continuously scrapes, processes, and repurposes vast amounts of data, deletion is not so straightforward. Once personal data has been absorbed into an LLM, can it ever truly be forgotten?
How Generative AI has made the right to be forgotten unworkable
Large language models (LLMs) and other generative AI models are built by being trained on massive amounts of publicly available data. The accuracy and efficiency of these models often rely on continuous updates. Even if a person’s specific data is deleted, AI models retain learned patterns, making true erasure infeasible. Engineers acknowledge that the only way to completely remove an individual’s data is to retrain the model from scratch—an impractical and potentially costly solution.
Efforts toward "machine unlearning" aim to delete specific data without dismantling entire models. However, the reconstruction of “forgotten information,” which has become proprietary inputs and continues to be recycled in model training, makes this seem like an impossible task today. LLMs and Generative AI often create outputs based on statistical patterns and relationships learned from training data – if an AI has been trained on an individual’s personal details before a deletion request, it may still infer, predict, or reconstruct similar details when prompted.
Further, the scale and proliferation of AI systems may be too big for take-down processes to work. For example, OpenAI’s GPT-4 uses 1.8 trillion parameters to generate its responses, and has a dataset that exceeds a petabyte. This dataset is constantly being recycled to learn patterns and inferences, making it nearly impossible to ensure that a person’s individual data is deleted. Moreover, the outputs of AI based on people’s data are often shared in unregulated and uncontrolled ways, making this “right to be forgotten” even more impossible. Even if it were possible to delete an individual’s data, it is unlikely that it would be possible to trace every output that was made based on that training data and have the users who received the output delete it as well.
Legal and ethical challenges
GDPR Article 17 grants individuals the right to request data erasure, but it does not define erasure in the context of AI. Traditionally, erasure was understood as the isolation and removal of specific records from structured datasets. AI models, however, do not store information in discrete entries. Once personal data is integrated into a model’s parameters, removal becomes nearly infeasible without costly retraining or experimental machine unlearning methods.
Although the European Data Protection Board (EDPB) has ruled that AI developers can be considered data controllers under GDPR, the regulation lacks clear guidelines for enforcing erasure within AI systems. Regulators may mandate deletion, but AI companies can argue that technical constraints make compliance impossible. Without an established mechanism to ensure data removal from AI models, there is no clear path for enforcement.
The GDPR also includes exceptions to its erasure requirement, further complicating compliance. Requests must be balanced against archiving purposes in the public interest, scientific or historical research, statistical purposes, and the right to freedom of expression and information, providing companies with legal grounds to deny deletion requests outright. Even if AI developers develop an effective way to erase personal data, they may still refuse requests, claiming that training models on personal data serves the public interest or that removal would infringe on freedom of expression. These broad justifications further undermine the practical application of the Right to Be Forgotten in AI.
In the United States, the Right to Be Forgotten is not recognized at the federal level. Some states have enacted privacy laws that grant individuals limited data deletion rights, but these generally do not extend to AI models. However, in September 2024, California became the first state to try to address this issue through Assembly Bill 1008 (AB 1008). This legislation would allow consumers to exercise their personal data deletion rights with respect to artificial intelligence systems that are capable of outputting personal information. While this marks a significant step forward, it remains to be seen how effectively this law will be enforced and whether other states or the federal government will follow suit. Additionally, questions remain concerning whether legal protections like California’s apply to personal data used to train these models in the first place.
Ethically, AI’s opacity creates accountability gaps. Even developers cannot fully explain how models process or recall data. This lack of transparency undermines individual control and increases risks of identity fraud, reputational harm, and privacy violations.
Potential approaches
As AI systems evolve into more complex data mechanisms that transform, generate, and replicate information, traditional notions of privacy, like the right to be forgotten, face significant limitations. Deleting a file or removing a post no longer guarantees true erasure when the data may have already influenced a machine learning model. Privacy must be reimagined to meet these changing needs. Here are some promising, if imperfect, strategies for aligning AI development with individual rights.
Federated Learning: Instead of centralizing all data, AI systems could be trained locally on user devices, preventing sensitive information from ever being stored in a way that makes it irrecoverable. But this faces bandwidth and computing challenges as training AI models collaboratively in multiple locations at once is computationally intensive.
Differential Privacy: This technique for protecting data privacy allows researchers to extract useful statistical information from a dataset without revealing any individual data by adding “a certain amount of random noise to the data.” Thus, it is nearly impossible to infer an individual’s specific information from the output. But there is an accuracy trade off as models that use differential privacy tend to perform worse due to the injected noise.
Algorithmic Destruction or Machine Unlearning: This process selectively removes certain data points from a trained machine learning model without retraining the entire thing. However, there is no universally accepted way of determining the effectiveness of machine unlearning, and for larger, more complex models, ensuring that everything is forgotten is almost impossible.
The right to be forgotten was designed for an internet that stored data. But today’s AI systems do not just store information; they also transform and regenerate it. While full implementation of the right to be forgotten in AI may not be entirely possible, a combination of federated learning, differential privacy, and algorithmic destruction could offer a path forward in ensuring greater individual control over personal data in the age of Generative AI.
Authors
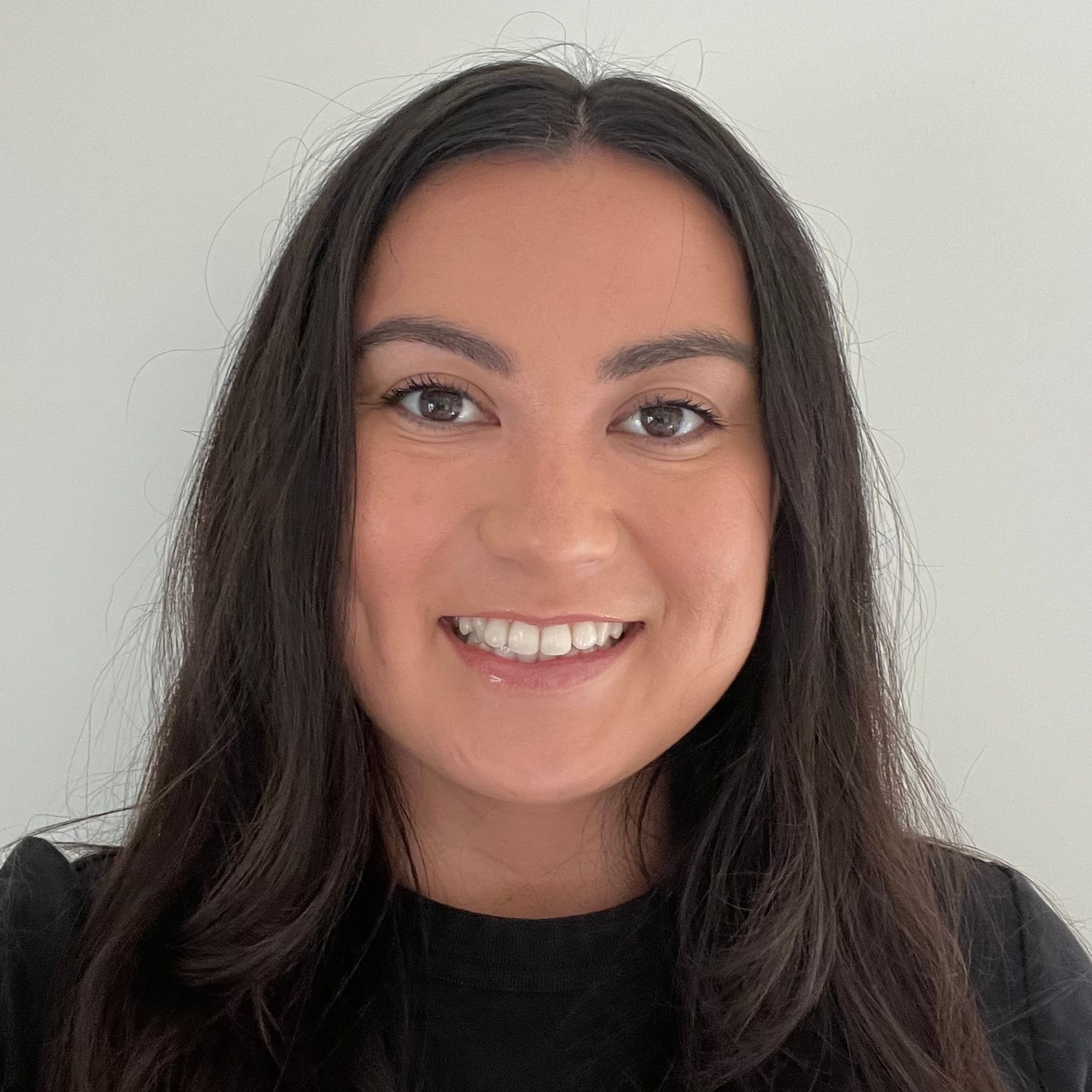
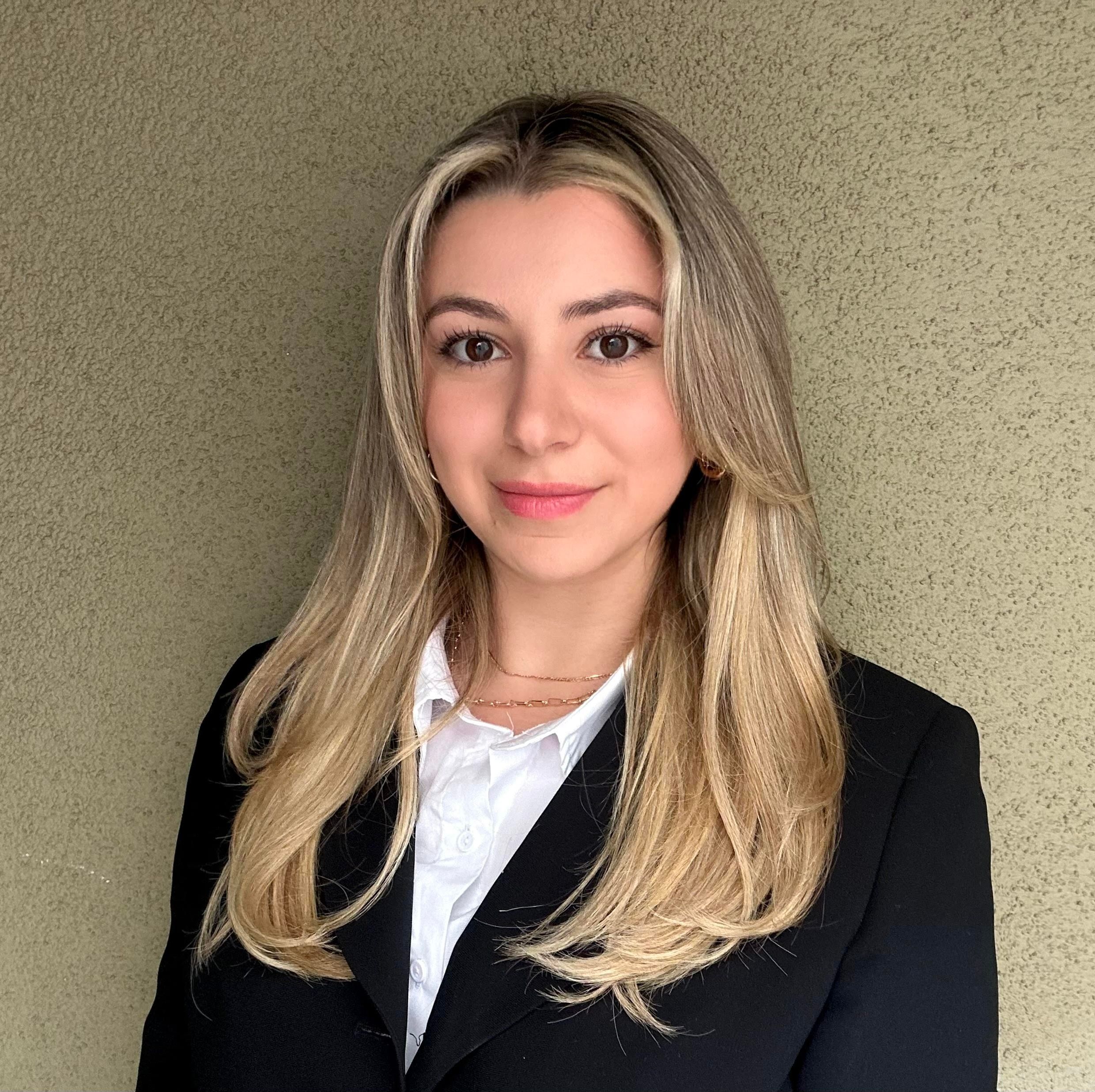
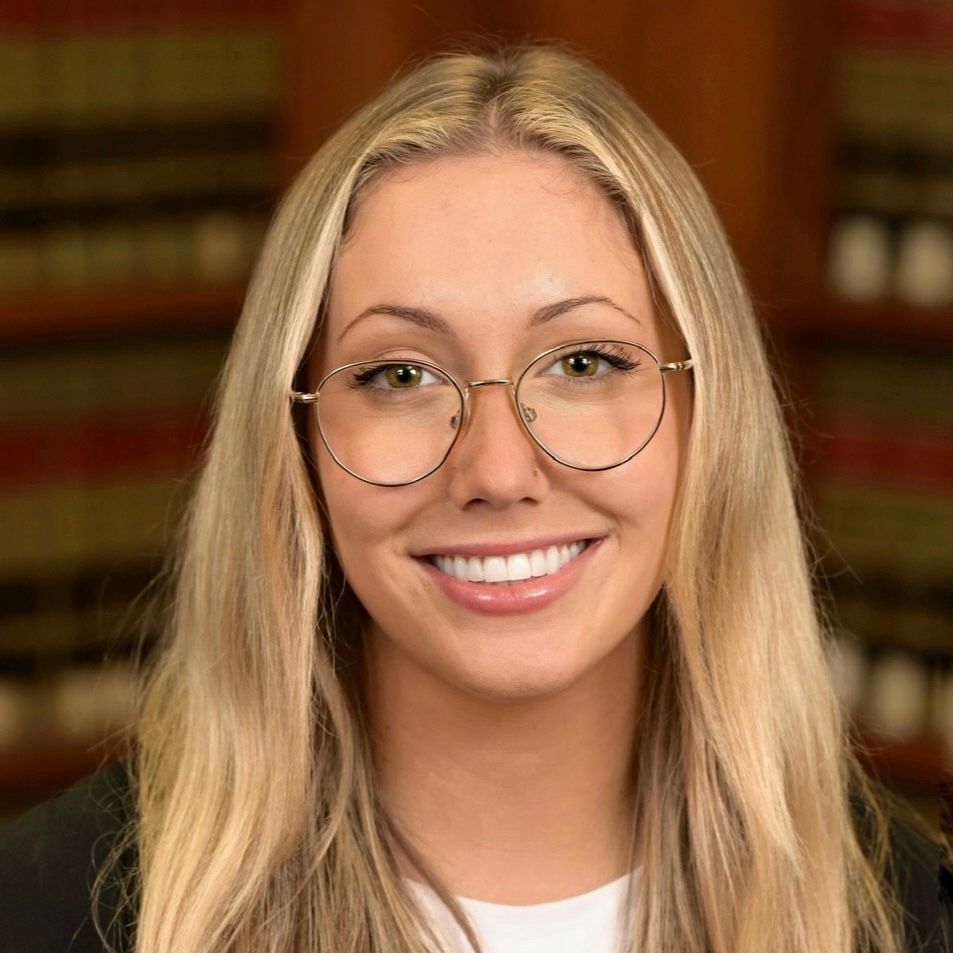