Imagining AI Countergovernance
Justin Hendrix / Feb 11, 2024Multiple past episodes of this podcast have focused on the topic of AI governance. But today’s guest, Blair Attard-Frost, has put forward a set of ideas they term "AI countergovernance." These are alternative mechanisms for community-led and worker-led governance that serve as means for resisting or contesting power, particularly as it manifests in AI systems and the companies and governments that advance them.
What follows is a lightly edited transcript of the discussion.
Blair Attard-Frost:
I am Blair Attard-Frost. I'm a PhD candidate at the University of Toronto's Faculty of Information.
Justin Hendrix:
Blair, can you describe your research generally? What is it that you are up to?
Blair Attard-Frost:
I look at the ethics and governance of AI. I'm really interested in Canadian approaches to AI policy and AI governance. So very interested in the perceptions of different stakeholders within the Canadian AI governance ecosystem, the development of Canadian AI policy initiatives. I'm also very interested in the ethics of AI value chains and supply chains, different ethical issues, political economy issues, as well as queer, trans and feminist approaches to AI just broadly.
Justin Hendrix:
So I got in touch having seen an essay called AI Counter Governance, which you published in December. We're going to spend some time talking about the ideas in that essay, but I thought I might just step back for a second and ask about your sort of general view of what's going on with regard to AI, the species, fascination with it and what it is that we're building here. Y
You have this essay you published in something called Heliotrope, and it starts off with the cults priests or conjuring an entity too complex for any of them to comprehend or control. It is an unruly assemblage of lithium mines and dump trucks, of shipping containers and undersea cables, microprocessors and server racks, data centers and data subjects, neural networks and knowledge workers, energy grids and power cords, machine learning decisions and predictions, and people and places and lives and stories and pasts and futures and sensors and models and data and knowledge and patterns far too slippery for anyone to grasp. What is it we're up to here with artificial intelligence?
Blair Attard-Frost:
That's a very good question, and in a sense, I think what I was trying to convey at the start of that piece in that giant run-on sentence is we are up to so many things with artificial intelligence, and it's really hard to put clear scope around what it means when we're talking anything related to AI because of that. So when we're talking about an AI system, we often neglect a lot of those material elements like the lithium mines or the cables or whatever other materials, human labor, are involved in it. And I think that kind of cascades, and we have conversations about AI ethics or AI governance into the way we approach those issues where we have this tendency to maybe forget about many of the social or material aspects that are involved in ethical reasoning around AI or making policy or governance decisions about AI, because we tend to slice off this relatively narrow set of technical features like data, algorithms, models, and we often neglect to consider that big picture view that's very slippery to handle.
Justin Hendrix:
Is that part of the problem of AI governance generally, that there's really... You're not the first to suggest there's really no coherence to the notion of artificial intelligence.
Blair Attard-Frost:
And I think it speaks to this overarching meta challenge of AI governance, which is that it's really hard to capture a shared understanding of what we mean when we talk about AI. And from that it's really hard to deal with a lot of these issues in a cohesive or relatively comprehensive way. And as you said, I'm definitely not the first person to point this out, but it's something that comes up again and again in a lot of the research literature on the subject and different intergovernmental organizations, governments themselves when they're trying to regulate AI now or making various governance initiatives around it, you often see some kind of acknowledgement that it's unclear what exactly AI is, but for the purposes of this initiative, this piece of policy, we're going to define it as such and such. And I think the effect that has and scopes off a really particular set of policy issues that are within scope and then many other things become treated as an externality.
Justin Hendrix:
In this essay, you refer to makers of artificial intelligence systems, including executives at companies like OpenAI, as the priests, the sort of keepers of this religion of artificial intelligence. That feels very right to me that there's a kind of religiosity, there's a faith that's being sold to people. How does that figure into your analysis of, I suppose, the much more nuts and bolts world of AI governance and policy?
Blair Attard-Frost:
I think a lot of conversations about the potential benefits of AI development revolve around this cultural power that AI holds, these narratives that we have of AI as this giant potential benefit and risk, things like artificial general intelligence, superintelligence, how those were ones purely science fiction but now we being told we need to take this seriously as a policy issue by some groups like OpenAI and it's all based around these longstanding cultural narratives of the giant promises, the giant perils that these futuristic systems pose. And I think when it comes to talking about what I write about in that essay specifically is superintelligence and more broadly speaking, the so-called frontier of AI, the advanced AI systems, be it in the short-term future or long-term future, I think that kind of affords a lot of proponents of these systems with a space for really speculating on the value that they want to drive in society in a way that's not necessarily attached to a lot of empirical evidence in some cases. And so it does feel like it's a faith-based assertion of what these technologies might be able to do.
Justin Hendrix:
You talk about the AI interregnum, what is this period we're in? How would you define it?
Blair Attard-Frost:
I think we're in this very transitional period in AI right now, especially in the last year or so. So the interregnum is conventionally used to denote a period between two different governments or two different states where things are a little bit chaotic over the course of that transition, over that course of changes in government. In the essay, I talk about it in relation to the trans studies scholar Hil Malatino's concept of the interregnum as this period of transition that trans people experience in the process of the gender transition.
But broadly speaking, I think what we are experiencing in AI right now is this ship that's occurred in the last 10 years with the advent of deep learning and all of the earlier stuff in the late 2010s around AI governance that came out of that. But it seemingly really picked up, accelerated with the advent of generative AI and really entered the public consciousness because of that in the last year or two where it feels like we're in this period where the technology is becoming more widely adopted now. There's broader, more detailed conversations about governance and regulation than there were a few years ago, stronger sense of urgency around that's being cultivated in the discourse about it too. And I think all of that has led to this sense that we're in this very uncertain time in AI right now where it's not quite clear where we could end up a few years from now.
Justin Hendrix:
How does the problem of explainability play into this, the idea that we can't really know how these systems work somehow fundamentally, that we won't be able to kind of open the black box, there's some limitation to the types of AI systems we're building now? I guess it almost does make them seem mythical in a way.
Blair Attard-Frost:
And I think it's really interesting to think about these kind of futuristic notions of frontier AI or superintelligence, and how they're often framed by the people who, the proponents of this kind of vision of AI in some ways as having the inexplicability of this system or the unpredictability, the uncontrollability of this system as being a key feature of it. But we already deal with a lot of explainability issues in present-day AI, and that really seems to represent when we're thinking about these speculative visions of it, an increase in scale and what we're already dealing with.
So explainability is one facet of this, could think about this in a number different ways too. Like human agency, we already talk about issues of human agency. Human control, these far future visions of superintelligence pose this problem of human control, human agency, automation bias just on a different scale. So I think it's this space where a lot of the problems we're already dealing with like explainability and the black box is just getting projected out into this future realm of, oh, no, what if we don't handle the black box problem properly? What if we don't handle fairness properly? What if we fail to make AI safe, secure, whatever else, it could lead to this horrible dystopian future. We lose all control. I think the way I think about it is we're already dealing with all of these issues right now, so why don't we try to get it right in the present and presumably, that would project out into the future and lead to better outcomes further down the line, focus on what's actually happening in the present and base policymaking based on empirical evidence that exists in the present.
Justin Hendrix:
Part of your essay on counter governance posits this idea that perhaps that's all well and good, that AI governance regimes will lead governments to implement something with regard to the regulation of artificial intelligence, but it might not be enough?
Blair Attard-Frost:
A really big challenge we're encountering in AI governance and the development of regulation, particularly here in Canada, but I know there's been some people who've been critical of the EU's approach for various reasons as well. So this is definitely an international challenge. It comes down to which stakeholders are involved in these conversations around the development of legislation and regulation, who has power to influence policymaking, who's being marginalized or left out of these conversations. And I think this kind of speaks to this notion that people often have in their heads when they think of AI governance. It sounds like this firm, that's governance is this thing that's the property of a particular class of industry and government elite who get to decide what decisions get to be made around policymaking and delegate decisions to people who work under them. It's this very top down approach that we often think of when we think about governance.
But I think there's a lot to be said for bottom up approaches to governance that emerge from the grassroots level, from communities, from workers. And this isn't just hypothetical. This is something that's already been done in many cases and that's what I try to point to in this essay is that we already have examples of bottom up approaches to community-led, worker-led resistance to AI governance and alternative proposals for AI governance frameworks that work better for the interest of those more localized workplaces or communities.
Justin Hendrix:
So you nest this idea of counter governance in prior theories of counter governance or resistance.
Blair Attard-Frost:
So I situate this in relation to few different bodies of literature. First, there's been some in the governance studies literature, broadly speaking, some discussion of this notion of counter governance. In the past, it was conventionally used to describe a response to governance decisions or governance framework made by one state, by another state, interstate or international counter governance initiatives. It got picked up more recently in the participatory governance literature to describe citizen opposition to state-led governance initiatives that fail to serve the interest of a particular community or interest. So I try to apply that to the world of AI governance, to think about AI counter governance as an approach to community-led or worker-led approach to opposing AI systems that don't work in the interest, in particular, community or group of workers.
And I also situate that notion of counter governance alongside other approaches that are typically very interested in resisting these top-down hegemonic approaches to AI design governance, participatory AI, for example. I love participatory AI approaches, but counter governance kind of differed within those and that participatory AI often tends to be focused on design interventions, so getting people who are impacted by the system, marginalized groups, giving them a bigger voice in the design of the system, often very focused on technical aspects and feminist AI approaches as well, which tend to be very focused... great approaches, but tend to be very focused on design elements, technological elements.
However, as counter governance approaches as they appear like the governance studies, participatory governance literature are much more concerned about organizational issues than they are with technological issues. So the focus of counter governance is an organization, it's social logics, it's political logics, economic logics, and the technology in a counter governance approach works in service to the organization, and the counter governance approach aims to ultimately change the organization's governance processes, its own governance frameworks by pushing back against in some way, trying to get the organization that you're opposing to internalize that all position in some way.
Justin Hendrix:
So in the essay, you give some examples of this in practice, let's talk about some of those. When you look out at the world, what have we seen already that looks like AI counter governance in practice?
Blair Attard-Frost:
So there's four examples that I go through in the paper, and one of these is the Google project Maven initiative that was, I think, launched back in 2018 where Google was proposing to have this military AI development contract for, I think, a drone based surveillance system with the Pentagon. Many Google workers protested this with a letter that they signed that was directed to, I believe it was the CEO of Google. I might be wrong about that, but it was an executive at Google, some people walked out of the workplace over it, some people quit over it. So there was this significant employee pushback over it and as a result of it, Google ultimately canceled that contract.
So we could think about that in hindsight, we weren't thinking about it as AI governance at the time, that wasn't as prominent as a framework as it is now. But Google had a corporate AI governance framework in which this military AI partnership and just partnerships with military as a potential avenue for business development played a role and by pushing back against it, the employees got them to change that part of their governance model to exclude the possibility of military partnerships. Google has since taken on military partnerships. That's a piece that I addressed in the essay as well, but at the time, that stopped Google from proceeding with that partnership.
I also, in the essay, write a bit about Sidewalk Toronto, which was a so-called smart study project that Sidewalk Labs, which is dead eerie of Alphabet, so sibling company, Google proposed to implement here in Toronto back in 2017, 2018 is when it started. The community pushed back against it. There were a number of concerns around different kinds of invasive technology, potential privacy violations, Sidewalk Labs financially exploiting public land for their financial gain. Long list of concerns that really reached ahead in 2019 and there was a number of town hall meetings, media engagements, alternative proposals for different kinds of data governance frameworks, alternative proposals for developing this parcel of old industrial abandoned land that was supposed to be the site of this development project. So lots of different alternative proposals for development that came up in the community and lots of other different, I guess you could call them, independent audits of the planning framers, proposals that were coming out of Sidewalk and the intergovernmental agency they were working with.
Then ultimately, Sidewalk Labs canceled the project. This was in the wake of COVID. It was May, 2020. They said, unprecedented economic uncertainty due to COVID. So we're pulling out, but a lot of observers have suggested that community backlash was a really significant factor in this decision as well.
Justin Hendrix:
And then I suppose a more recent example, the writers strike, the strike of union workers in the entertainment industry more generally in the US?
Blair Attard-Frost:
Yeah, yeah. So this is a really recent example from last summer where the Writers' Guild, WGA, and Screen Actors, SAG-AFTRA went on strike. They went on strike for a number of reasons, but a really core demand had to do with the use of generative AI in two different ways. One, the use of generative AI, the training of generative AI on union protected creative materials, and then also the use of generative AI within the creative workflow, I guess you could say, so the potential substitution of human creators with generative AI. So those were pretty significant parts of their collective bargaining.
I think WGA, I forget what specific wins they got from that. I think it was last November or last December where they reached an agreement, but there were some of their demands that were met, and if I remember correctly, the Screen Actors had some provision around that as well, though I'm not entirely sure about the specifics of that. I didn't have as much of a chance to follow up on the outcome of it and read through it in detail.
Justin Hendrix:
You also offer a set of suggestions for anybody interested in practicing AI counter governance. So whether you're an organizer or worker, I'm thinking about this maybe even through the context of someone who's in the business of doing tech accountability journalism or research. What should folks do if they want to be able to, as you say, take AI governance into their own hands?
Blair Attard-Frost:
There's really four main activities that I think about when I think about organizing AI governance from below, and I call them talk, investigate, build awareness and oppose. So I think talking is a really important first step to gain economy understanding amongst yourself and others in your community about what you want to see from AI in your community or in your workplace, what your shared values are, what your shared needs are, what kinds of threats, risks you perceive from AI, what kinds of potential benefits you perceive. Talking is really this brainstorming period dealing with a lot of, on the one hand, these definitional challenges where we start, that we talked about at the start, where it's like, what even is AI? What precisely is such a vague, ambiguous term? What precisely is it that we're worried about here? Is it just machine learning? Is it this broader set of different kinds of data considerations, infrastructure considerations, economic organizational factors? What is it that we're talking about here? How can we organize these conversations? Is it round table discussions, town hall meetings, workshops, whatever else?
Then with some basic understanding of that, I think it becomes easier to collectively investigate those issues in more detail that you are interested in building more awareness of, so you can learn more about what kinds of regulations are currently being developed in your jurisdiction, if there are any at all. What's currently being forced, if there's any existing regulations, say, privacy regulation, if that's being applied to AI systems and how effectively it's being applied, what other kinds of regulatory considerations are there that are already there? What kinds of regulatory gaps might there be?
Perhaps, you feel like local regulations or governance mechanisms don't serve your shared interest, and then we can talk more about what would or what other resources would, and then you can continue to build awareness around that with different resources within your community, different kinds of explainer documents, knowledge resources, reading list, viewing list, videos, podcasts like this, all kinds of different knowledge building tools. And then once you have a common awareness, it becomes a bit easier to collectively oppose any kind of AI system work or organization that you think doesn't meet the interest of your community or could potentially pose harms to your community.
Justin Hendrix:
One of the things I was wondering about reading this is the extent to which you have thought about how much folks engaged in AI counter governance need a technological understanding or perhaps even a similar technological imagination as the AI priests. What do folks who are opposing perhaps implementations of artificial intelligence that they regard as unjust or otherwise dangerous, what do they need to know about the technology? How deeply do they need to know the technology? And can they use the technology? For instance, if you're in the business of doing tech accountability activism or journalism or something along those lines, generative AI might be a very useful tool to helping you do the types of investigations you describe here.
Blair Attard-Frost:
So I think there's two pieces there. On the one hand, there's this piece around what the potential benefit could be to your community, and I think that's one where it's really context specific and something that you would really need to figure out with whoever's in your community, whoever you're working with. So the idea of generative AI being used as a tool to support investigative journalism, there could be potential ways using it for that, but I think there's also potential risks to privacy, to integrity of journalism, potentially to authenticity that need to be considered as well.
So I think whenever a benefit of AI to a community is being considered, it needs to be part of this broader conversation about what are the potential benefits, risks, threats, potential harms that could come about it, and it really needs to be rounded within that specific context of that group of people or of that group of workers who are looking at this tool together.
And then I think this question, how technically specific do we need to get is perhaps a matter of context sensitivity as well, because if you're planning to actually build a system and if you decide, hey, generative AI or some kind of automated decision making system might be useful for our workplace to help with some of our work, to improve the quality of our work, to improve the accuracy, to make it faster, more efficient, whatever it is, then I think that would necessitate a much more granular level of technical understanding of how the system works, what exactly it is you're going to build, what kinds of technical components you're going to need to get access to, what kinds of data you're going to need, what kinds of data preparation activities you're going to need to do, how you're going to evaluate the quality of the data of the model, what the lifecycle is going to look like, et cetera, versus something where, example would be, the police are using some kind of biometric surveillance tool or facial recognition tool to surveil a community, or an employer is using some kind of employee software to monitor the emotional states of workers, which is something I've seen a lot of startups focusing on lately.
Where in situations like that, some level of technical understanding of how the system works is needed, but it's perhaps at a higher level. And the bigger issue there, I think, is what the system is intended to do. And again, those kind of organizational, economic, political logics that the company or that the police force might have for implementing that system there. So in that case, it might be more of a question of social systems or economic systems or organizational systems than it is about the specific technical aspects of an AI system or it's life cycle.
Justin Hendrix:
So I guess one of the other things that occurred to me in thinking about this piece of it, like when you do enter the phase of opposition, if that's indeed what you're doing. So imagine activists opposing some implementation of biometric technology or surveillance system or something along those lines. If you find that artificial intelligence itself is a tool that will help you, it's not hard to imagine different groups imagining, for instance, building bots that might engage with people in order to advance certain ideologies or political perspectives. It's not hard to imagine them creating content that's generated by AI systems. Is there a sort of sense of proportionality here or something along those lines that we can apply to think through whether if in fact the use of artificial intelligence in opposition activities is appropriate and proportional?
It's something I'm wondering about because I suspect we're going to see, even now, we're going to begin to see a lot more groups that we would think of generally as pushing for social or economic justice, pushing back against state power, pushing back against corporate power thinking, well, actually, some of these tools that these corporations are building are actually ripe for use to potentially disrupt those same centers of power, so why wouldn't I use them?
Blair Attard-Frost:
I think it goes back to those first three phases and doing due diligence with talking about the system together, investigating it, so doing a bit more of a deep dive into what impacts the system might have, what impacts it's already had from other communities, and then building awareness within the community around those issues.
To my mind, the idea behind talking, investigating, building awareness is to be able to get as balanced of a view from as many different perspectives as possible and to gradually iterate on those teams and build them over time where you're considering it from the point of view of many people in the community in the workplace, you're considering many different sets of shared values, many needs, many potential benefits, many different threats. Taking stock of that through particular types of conversational venues, like round table discussions, workshops, et cetera, investigating it through particular resources that already exist, and provide papers, podcasts, videos, other kinds of documents that describe the potential benefits, risks, harms that have been documented in these systems. The important part there, if an activist, community of your advocacy group or whatever else wants to build an oppositional use of AI is you put it in their own activities.
I think the important part there before making that decision is to make sure you've done due diligence about the potential impacts, investigating those a bit more deeply and building awareness that you're planning to build this system or that it's one of many potential plans. Building awareness of that within your broader community or within your workplace more broadly to make sure there's not many other people who might disagree with it or be more concerned about that kind of decision.
Justin Hendrix:
You point to the necessity for folks who are developing AI governance tools to invest in more participatory policymaking, and we've seen some examples of this around the world. I feel like I've heard of examples in Canada where certain policy questions have been put to citizen councils or into participatory processes. Are there others that you'd point to that you think are good examples?
Blair Attard-Frost:
I think there's been citizen assemblies on various issues in Canada in the past, certainly around artificial intelligence more recently, and in digital and data policy issues more broadly. There's been a lot of civil society engagement in Canada, all kinds of different working groups, reports, briefs, other kinds of initiatives that have been published. In Canada, specifically, the online safety app, the public participation in that relative to some of the other digital policy initiatives, we've had a fairly more significant public participation push on it to get many people from across Canada to voice their own thoughts on it.
But again, in terms of just convention, the latter of public participation ranging from just consulting with people, getting their feedback and then doing what you want with it up to actively collaborating with them throughout the project or the policymaking process and iteratively help letting them shape it and then up to giving them complete ownership at the top of the ladder rate. And a lot of it is to mention errs towards the side of consult, and that oftentimes leads to consultations that are viewed by some of the communities like tokenizing, performative used to just legitimize the decisions that government had already made. So it's really a mixed bag methodologically, like the quality of public participation in digital policy in Canada.
Artificial intelligence is a really interesting space in this because the piece of artificial intelligence legislation currently tabled in parliament, the artificial intelligence and data app didn't really have any public consultation in the drafting of it, and this was despite the ministry that drafted the bill shortly after. So the bill was tabled in parliament in June, 2022, and in January or February of 2023, the same ministry has an AI public awareness working group that released this whole report for best practices for public participation in AI policymaking in Canada. And they interviewed, as part of this report, they commissioned a study involving 1200 or so Canadians. And the results pretty compellingly show that Canadians want to be consulted on policy co-design on AI regulation issues. So a disconnect from the working group and their findings in the one hand and the legislation on the other. But yeah, it's very much a mixed bag in Canada as far as the quality of public participation goes or the methodology, and I think there's a lot of different reasons for that.
Justin Hendrix:
I can't let you go without asking what the status of AI legislation is in Canada. What can we expect in the coming months? Is this about to become law? Is it going to get a vote?
Blair Attard-Frost:
Yeah, so right now the artificial intelligence and data app, the app that I was talking about there, it was debated in parliament last fall, so there were two votes on it, and it was ultimately voted ahead last summer in fall, and then it was voted ahead to committee study middle of last fall. So last October, I think, is when it's called The Standing Committee on Industry and Technology started studying this. In the process of that, many people from across the country have submitted briefs on the legislation. Many people have testified as witnesses. So that committee study is still underway right now.
They recently started up again for the new year. They're inviting more witnesses in, and then it's not entirely clear yet when the committee study's going to end. I would imagine sometime within the first half of this year, they'll produce a report on it. If they decide from the study that the bill is going to move ahead and move the legislative process, then there'll be a third vote on it. If it passes the third vote, then it goes ahead to the Senate. And once things reach the Senate, in Canada, it's largely procedural at that point, it's effectively a law. Sometimes there's a few small kind of tweaks to the legislation technical clause and ends, but it could very well be passed through the House of Commons and into Senate, which would essentially make it lock sometime later on this year.
Justin Hendrix:
What's next for you in your research?
Blair Attard-Frost:
Right now, I am taking a little pause for research. I'm doing a lot of teaching right now. So I'm teaching some courses on AI policy, ethics, governance this term that I'm really enjoying, and that's helping me reflect on my own research quite a bit. And then I'm nearing the end of my PhD after that. So once I finish up with that, I'm going to be finishing up my dissertation. I've been spending the last couple of years doing a really broad survey of what's going on in AI governance in Canada? What are different people's perceptions of how AI governance is going? What should be done differently? Going to tie that all together with a lot of my more theoretical work on AI ethics, AI policy, and then shut my dissertation.
And then after that, will see lots of opportunities open to me, but I'd be really interested in exploring a lot of these issues around community-led AI governance, worker-led AI governance, different bottom up approaches, as well as just different transgender issues in AI policy and the perspectives of trans people impacts of AI on trans people in more detail, potential role of trans people to play in AI policy or how trans lit[erature] has to be better represented in AI policy because trans people are a group where we're very often excluded from these conversations. I don't really hear anything about binary gender classifiers or any impacts of that coming up in any policy spaces, so I'd be really interested in looking at that a little bit further too. But for now, it's just wrapping up the very Canada-centric stuff.
Justin Hendrix:
One of the communities I assume that has probably been harmed most by issues around classification and different types of data labels and things of that nature that exclude the spectrum.
Blair Attard-Frost:
Yeah, absolutely. And I think there's some researchers who've done great work at this from an AI ethics point of view, looking at the potential harms of different issues around data labeling and representational harms that can come through bias in the dataset. But then there's also issues more around allocated harms, issues around the potentially disproportionate impacts that AI could have economically on trans people versus other groups of people. So there's a lot of ways to think through the question, both for the data algorithm technology side, as well as the broader social economic policy side. It's really a space that needs a lot more investigation.
Justin Hendrix:
Well, perhaps we'll be able to talk about that investigation as you continue it in the future. I hope your teaching goes well this semester, and maybe we'll talk later about what's happened in Canada and find out if there's been an outcome in the legislative process.
Blair Attard-Frost:
Yeah, that would be great. It's still all up in the air right now, so it'll be interesting to see how things play out in the next few months. There's been a lot of criticism of the government's approach, so I'm sure it will result in some interesting stories and conversations whenever the rest of the legislate process close.
Justin Hendrix:
Blair, thank you very much.
Blair Attard-Frost:
Thank you, Justin.
Authors
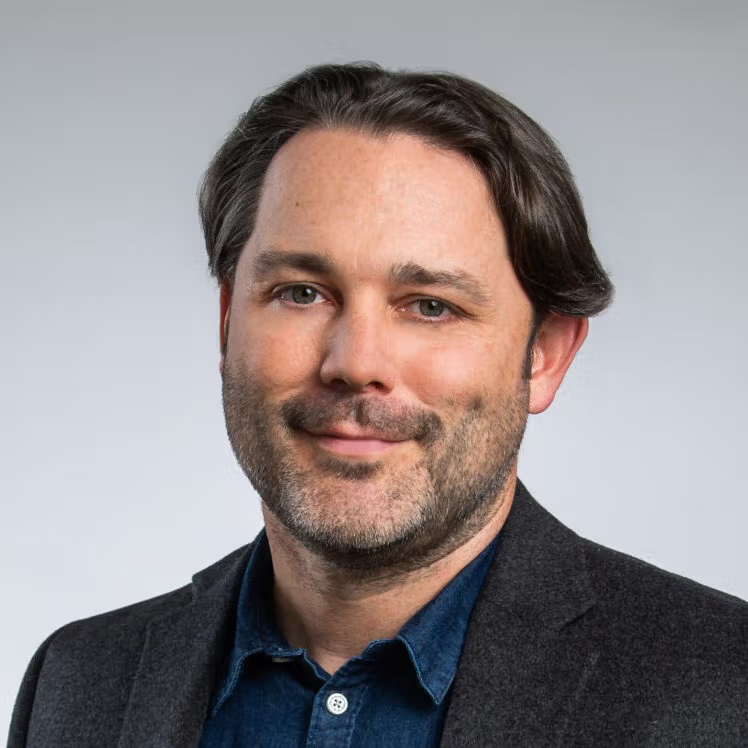