Forget Moonshots, AI Needs 'Chip Shots' to Win Public Trust
Kevin Frazier / May 5, 2025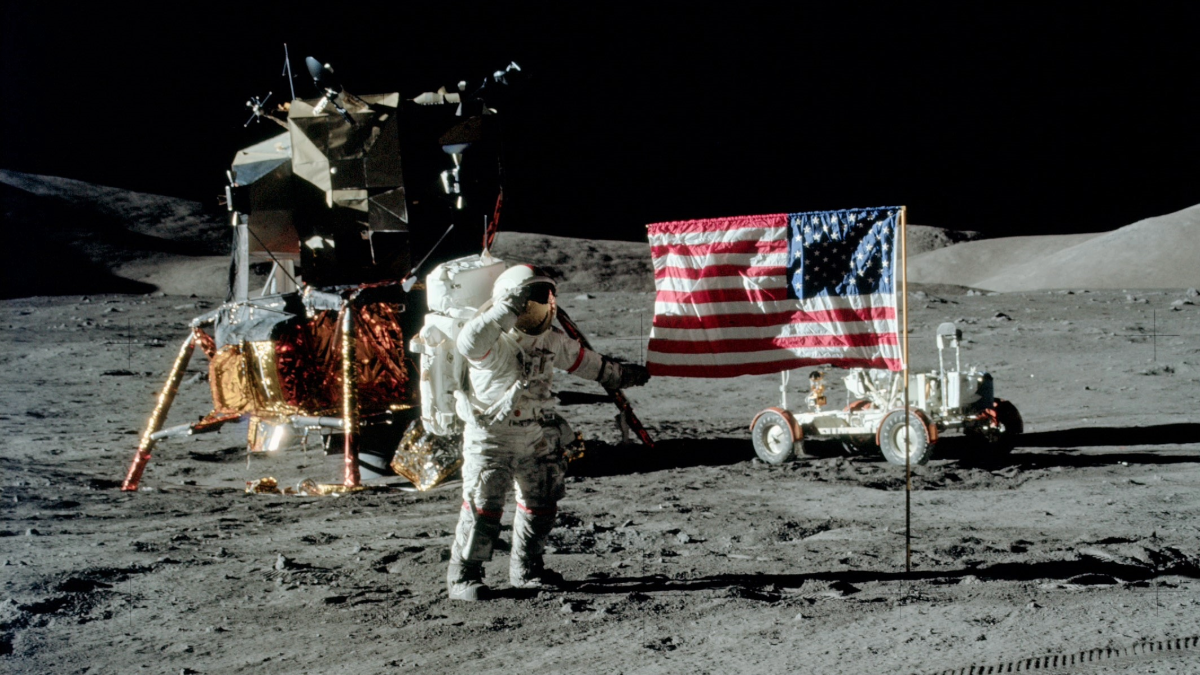
December 12, 1972—Eugene Cernan on the Moon during the Apollo 17 mission. Harrison Schmitt/NASA Wikimedia
A scan of the more than 10,000 comments submitted to the White House Office of Science and Technology Policy (OSTP) in response to its Request for Information on the Trump administration’s AI Action Plan suggests many of the respondents are in favor of high-risk, high-reward projects. A number of these comments refer to “moonshots.” For instance, Brightband, a startup company, called for an AI in weather prediction “moonshot,” while a submission from Brown University called for government funding of moonshots to deliver “transformative advances.” Similarly, the Institute for Progress proposed the creation of R&D moonshots. Many more mentioned the ongoing battle to outpace China’s AI development—a moonshot in its own right.
As tempting as it may be to frame AI policy around the most important geopolitical projects and loftiest visions for its technological potential, a different focus is warranted in the near term. Rather than solely shoot for the moon or race with our adversaries, the administration should incorporate “chip shots” into its AI Action Plan.
Big promises, little returns
Before describing the importance of incorporating chip shots in the forthcoming plan, it is important to provide a more thorough outline of the current state of AI development and public sentiment toward AI. With respect to the latter, it comes as no surprise that public opinion has fluctuated given drastically different assessments of AI capabilities. On one hand, the public hears breathless pronouncements of impending artificial general intelligence (AGI)—the ultimate "moonshot"—promising paradigm shifts that could reshape humanity. On the other hand, a growing chorus of skepticism focuses on the more immediate, often less glamorous, realities: AI generating uncanny selfies, enabling academic dishonesty, or powering recommendation engines that fuel polarization. This skepticism isn't entirely unfounded. While the potential of AI is immense, the most visible applications often fail to resonate with pressing public concerns, leading many to question if the societal and environmental costs associated with its development are truly justified.
From my vantage point as the University of Texas Law’s inaugural AI Innovation and Law Fellow and a Contributing Editor at Lawfare, where I write and podcast on AI, I see a dangerous disconnect emerging in AI development. The dominant players—the large labs, which command vast resources and capture headlines—seem locked in a race toward generalized capabilities. This pursuit, while technologically fascinating and culturally captivating (featuring boardroom drama, X battles, and geopolitical intrigue), often overshadows quieter, yet potentially more impactful, work happening elsewhere. Labs leveraging AI for specific, tangible benefits in areas like healthcare diagnostics, climate modeling, or materials science struggle for oxygen in a discourse dominated by chatbots and image generators. This leaves the public understandably wary, perceiving AI less as a tool for collective progress and more as a catalyst for chaos—amplifying misinformation, threatening jobs, and consuming vast energy resources for seemingly marginal gains.
Given this AI landscape, it becomes easier to understand why the public may not want to double down on investments in AI. On a regular basis, people pepper me with questions about the true value of AI, given how most people interact with ChatGPT, Gemini, and similar tools. They ask, “Do we really need to write our emails faster?” They wonder, “How many more Studio Ghibli selfies are necessary to satisfy our demand for silly images?” And, they joke, “This is the incredible future that the AI labs promised? Let me know when it can avoid making up 30 percent of its responses and finally get to solving climate change.”
These sorts of questions and doubts reflect the fact that we are inadvertently framing the AI challenge primarily as a moonshot endeavor—an incredible gamble requiring orders-of-magnitude progress across multiple fronts, often with uncertain timelines and societal benefits. Having been told that an unimaginable future is on the horizon (for better or worse, depending on your perspective), each passing day that looks more or less like the last one sows doubt in AI as a field. This is an understandable and worrying way of thinking about a transformative technology—one that is at once capable of producing incredibly useless content and also upending entire ways of thinking about complex areas of science, math, and physics.
In short, the problem is that AI as a moonshot has wholly captured the public’s imagination. While not necessarily a bad thing, this overly simplified view invites skepticism when progress seems slow or disconnected from everyday problems. What if, alongside the most ambitious long-term goals set for AI, we also prioritized a different kind of target— the "AI chip shot"?
Reasonable expectations
A chip shot, in contrast to a moonshot, is an objective well within expected technological trajectories but demanding precision, focus, and significant investment. It represents a targeted application of existing or near-term AI capabilities to solve a well-defined, significant public policy problem. It’s not about achieving AGI; it’s about demonstrating concrete value, now, on issues that matter to millions.
I propose we galvanize the AI community, particularly the innovative small and mid-sized labs often overshadowed by tech giants, by establishing ten “AI Chip Shots.” The criteria for selecting the challenges or goals included on this list would be demanding and specific, intended to prevent labs from spinning their wheels on speculative projects and instead direct them to make progress on problems that plague the here and now. Here’s a list of potential factors:
- Technological Feasibility: Each “shot” or challenge must be achievable within a strict timeframe – no more than two years – using current or realistically anticipated AI advancements. This grounds the effort in pragmatism, avoiding speculative leaps.
- Public Policy Focus: The project must directly address a recognized, significant public policy concern within areas like public health, environmental sustainability, education, infrastructure, or economic opportunity.
- Significant Impact: The solution must demonstrably benefit a substantial population, defined here as impacting the lives of at least ten million Americans. This ensures the efforts are directed towards problems of scale.
- Political Viability: The problem addressed should be one with broad, ideally bipartisan, recognition, increasing the likelihood of public acceptance and potential integration into existing systems.
To catalyze these efforts, we need more than just encouragement; we need structured incentives. Imagine a public-private partnership offering substantial rewards for the first labs to successfully deliver on the pre-defined chip shot challenges. Success wouldn't just mean a prototype; it would mean a deployable, validated solution. The reward? Full coverage of development costs plus a significant, unrestricted prize for the lab to reinvest as it sees fit.
Crucially, participation would be structured to foster a more diverse innovation ecosystem. Eligibility could be capped based on lab valuation or funding history, explicitly excluding the largest, most dominant players. This ensures the incentive targets the agile, potentially more focused organizations that might otherwise struggle for resources. Furthermore, a condition of participation would involve agreements around the licensing of relevant underlying technological advances developed during the project. The precise scope of this licensing – balancing the need to incentivize innovation with ensuring public benefit from publicly funded breakthroughs – requires careful consideration and would be a critical area for future policy design.
What might these chip shots look like? Consider these possibilities:
In material sciences, a chip shot prize could challenge teams to assist in the development of the next generation of batteries. Think of finding new battery materials like searching for a needle in a haystack. Testing each straw takes forever. This prize would go to the team that builds an AI that acts like a super-magnet: it could quickly analyze the basic recipe of potential materials and predict which ones are the "needles" – those that conduct energy well and are stable. This AI would massively speed up the search for better battery tech. In healthcare, AI could tackle diagnostic delays by developing tools specifically designed to rapidly flag subtle but critical indicators of conditions like sepsis or pulmonary embolisms in patient data or scans, freeing up clinician time and speeding intervention. Similarly, in drug development, a targeted AI challenge could focus on predicting the efficacy and toxicity of small-molecule drug candidates for notoriously difficult targets, like specific neurodegenerative pathways, aiming to significantly improve the success rate before costly clinical trials begin.
These focused AI applications deliver achievable gains now by cleverly using maturing tools like pattern recognition and predictive modeling to solve concrete problems—offering near-term value faster than waiting for basic breakthroughs. The technology isn't futuristic; the real hurdles lie in effective data integration, thorough validation, user-focused design, and mastering the complexities of actual deployment.
Focusing on chip shots offers several advantages over the status quo. First, it directly counters the narrative that AI primarily serves trivial or nefarious purposes. Successes in these areas would provide tangible, widely understood demonstrations of AI's potential for public good, building crucial public trust and support for the field. Second, it diversifies the AI landscape, providing pathways for smaller labs to achieve significant impact and recognition, fostering competition beyond the AGI race. Third, it creates near-term returns on investment—both financial and societal. While moonshots aim for distant, transformative payoffs, chip shots deliver measurable benefits within a political cycle, making continued investment more palatable. Finally, it forces a practical reckoning with the challenges of deployment, ethics, and governance in specific contexts, generating valuable lessons for broader AI implementation.
This isn't an argument against long-term, ambitious AI research. Moonshots remain essential for pushing the boundaries of knowledge. However, relying solely on them to justify the societal investment and disruption AI entails is strategically flawed. We need a parallel path, one focused on demonstrable, near-term public value.
By defining and incentivizing AI chip shots, we can steer innovation towards pressing societal needs, rebuild public trust through tangible results, and cultivate a more resilient and diverse AI ecosystem. It’s time to prove that AI can do more than generate novel images; it’s time to show it can solve real problems for real people, precisely and effectively.
Authors
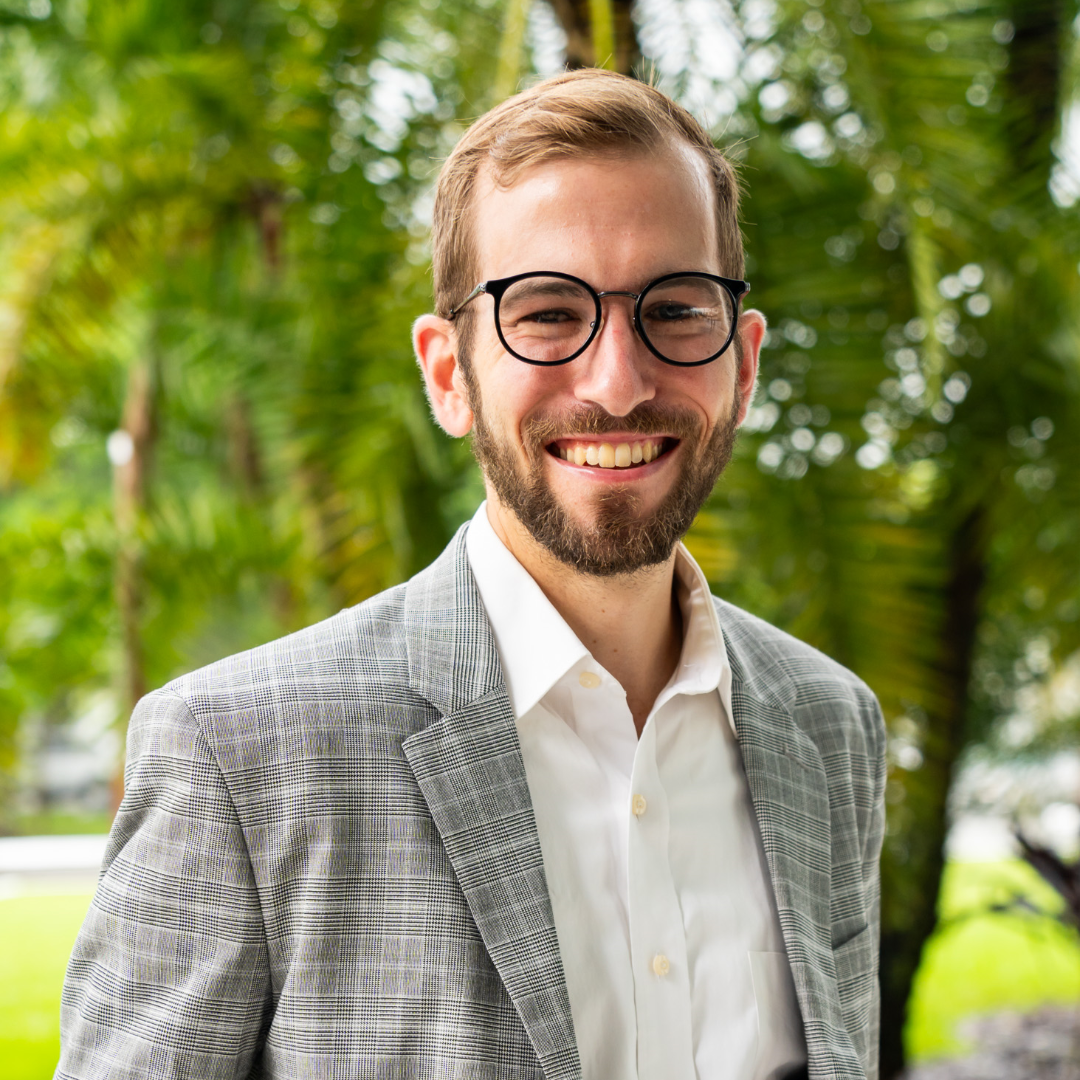