Bigger Might Not Be Better: The Limits of Regulating AI Through Compute Thresholds
Dinah van der Geest / May 21, 2025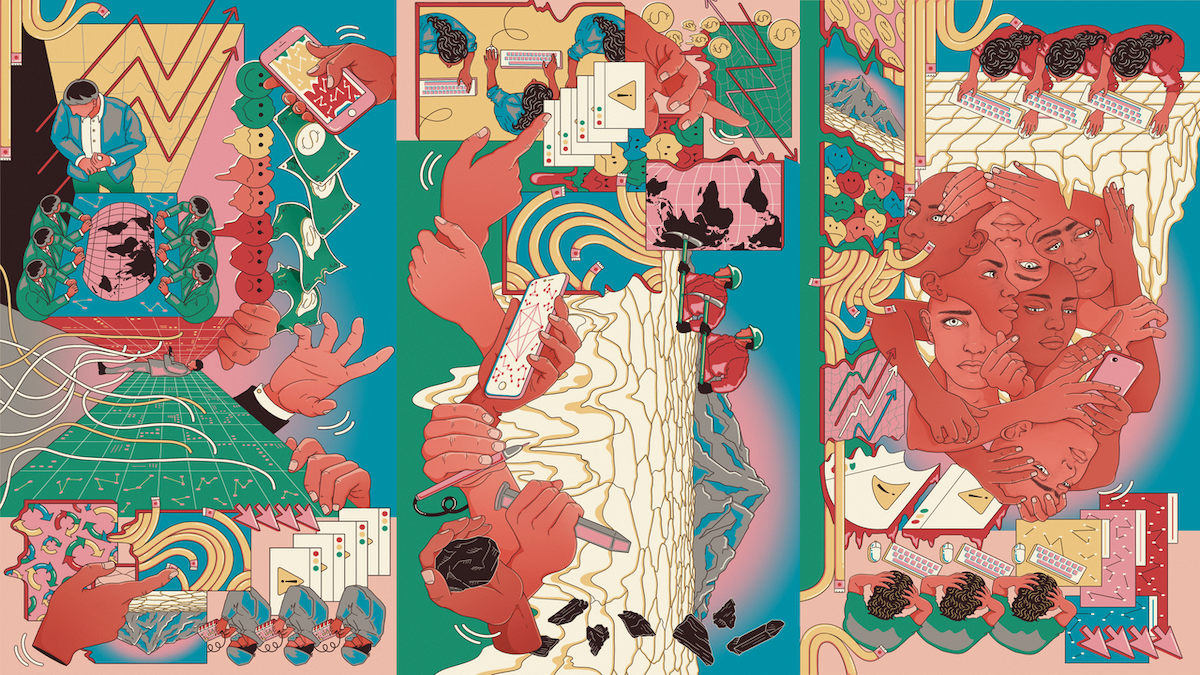
Clarote & AI4Media / Better Images of AI / AI Mural / CC-BY 4.0
The recent release of DeepSeek, a Chinese large language model that outperforms other models without relying on massive computational resources, sparked widespread attention because it was better without being bigger. As artificial intelligence (AI) becomes increasingly embedded in our everyday lives, relying primarily on compute thresholds for regulation risks is missing the bigger picture of how these systems actually affect people and society. Today’s frontier models may depend on vast computational resources. Yet, tomorrow’s breakthroughs could emerge elsewhere, from more efficient architectures, novel training model methods, or improved data quality.
Long before today’s high-compute models dominated the discussion, algorithmic systems with relatively low computational demands were already shaping decisions in policing, welfare, immigration, education, and employment. These systems often produced significant harm, especially for people of color, refugees, low-income communities, and other marginalized groups. By focusing regulatory attention primarily on compute-heavy models, there is a danger of repeating old mistakes: ignoring the deeply embedded AI systems already shaping lives today, simply because they fly under the regulatory radar.
Relying on technical thresholds, whether compute, dataset size, or another technical metric, in AI regulation creates a system that will quickly become outdated as AI development continues. Instead, we should focus on context-specific, harm-based human rights approaches that evaluate actual impacts on people and communities.As AI is integrated into society, policymakers and regulators are attempting to establish governance frameworks to assess and manage the risks and harms from these technologies.
Some of these attempts fall short. To keep pace with the evolving AI landscape, a more nuanced regulatory framework is needed. This framework needs to integrate adaptive, context-aware assessments of AI systems that account for how its underlying models are used, by whom, and for what purpose, to ensure AI systems align with human rights.
The state of the debate: compute-based AI regulation
One emerging approach that underpins AI regulation is the use of compute thresholds. These are measured in floating-point operations (FLOPs), as a proxy for model capability and a trigger for regulatory oversight. FLOPs are often used as a rough proxy for determining a model’s size, complexity, and potential capabilities. However, as leading researchers argue, compute metrics alone do not capture the full range of a model’s capabilities or its potential for harm.
We can think of hypothetical examples that bring the potential of low-compute harms to the fore. For example, government institutions may use a relatively small language model to screen asylum applications built to understand and generate human language with fewer computational resources than larger models. Yet, its decisions can have life-altering consequences, such as denying someone protection or family reunification based on opaque or biased reasoning. In this case, the risk emerges not from the model’s size but from the high-stakes nature of its deployment, as well as the lack of human oversight throughout the AI model design and development lifecycle.
Unfortunately, there are numerous real-life examples of small AI systems with harmful impacts.
For example, the Netherlands’ tax agency implemented a relatively simple risk-profiling algorithm that needed only modest computational power to analyze personal attributes like dual-nationality and income level for benefits fraud detection. This computationally lightweight system devastated lives across the country by systematically discriminating against minorities and low-income families. Its implementation resulted in unjust debt collection, family separations, and even suicides among those wrongfully flagged by the automated process.
This case shows that harm is not inherently tied to model size or compute intensity. Risk emerges from a model’s deployment context, data sources, and design choices. And crucially, how it interacts with existing societal inequalities. Relying on compute thresholds as a risk indicator in AI regulation promotes the misconception that risk is inherent to a single model, rather than arising from the broader context in which it operates. There are two particular assumptions behind the FLOPS paradigm that are important to understand, especially as DeepSeek has demonstrated how flawed these assumptions are.
The limitations of the prevailing FLOPs paradigm
There are two key critical limitations to using compute as the primary metric to assess AI risks and harms, both of which are crucial to understanding the actual impact of AI, especially when regulations focus only on how much computation a system uses. These limitations are particularly concerning when considering the potential human rights implications of AI systems. Below is a more detailed examination of each of these limitations:
Assumption 1: More FLOPs make for more powerful models
The main assumption of the compute threshold-based approach to AI regulation is that higher FLOPs correspond to more powerful models. Supposedly, because they can process more complex calculations, they lead to better performance and greater capabilities.
To explain this, consider an engineering metaphor: cars. Imagine you are trying to judge how powerful a car is just by looking at how much horsepower its engines have. More horsepower might mean more capacity, but that may not always be true. A car with better engineering, that runs on electricity rather than fuel, or has a lightweight body, might be significantly faster and more efficient, even if it uses less horsepower.
This analogy becomes especially relevant in light of models such as DeepSeek. It demonstrates that high performance doesn’t necessarily require massive computational resources. Despite having significantly fewer training FLOPs than models by well-known big tech companies (ChatGPT’s GPT-4V or Google’s Gemini Pro), it achieves comparable and in some tasks, superior results.
Assumption 2: More FLOPs = more problems
The second assumption is that as a model’s capability increases with more compute, so do the risks of harm. While compute offers a quantifiable indicator of model capability, it is inadequate for assessing potential harms.
Risk is not solely a function of model size or training intensity, but of the context of deployment, the design of the system, the data used, and the social structures it interacts with.
Just like an engine’s horsepower alone doesn’t determine a car’s risk, computational power alone doesn’t determine an AI model’s risk. It’s the combination of capability, context, and design that determines the risk it poses. We know this from the examples of the use of low-compute algorithms in the educational system and the government. This case illustrates that a model's potential for harm is not dictated by its computational power. Instead, the design, purpose, and deployment context of the model – factors that go beyond compute – are what ultimately determine the risks it poses.
The DeepSeek challenge to compute-based regulation
The emergence of models like DeepSeek’s R1 challenges the core assumption behind compute-based regulation that greater computational resources directly equate to higher model capabilities or risks. DeepSeek’s R1, through innovative architectural design, achieves performance comparable to much larger models from leading developers like OpenAI, while using significantly less compute. This undermines the notion that thresholds based on FLOPs can reliably indicate an AI system’s potential impact. As the link between compute and capability becomes increasingly tenuous, it becomes clear that smaller, more efficiently engineered models can still pose significant real-world risks, exposing the limits of compute-centric regulatory frameworks.
How the focus on FLOPS shapes current regulatory approaches
Concerns regarding the use of compute power to estimate risk are important given recent trends in using FLOPs to regulate AI. Policymakers in the United States (US) and the European Union (EU) are using FLOPs as a benchmark for AI regulation, reinforcing the prevailing assumption that higher computational power corresponds to higher risk. But why is this? Compute provides a quantifiable and externally verifiable metric that can be consistently applied across various systems. It is widely used in machine learning and computer science, allowing developers and researchers to assess hardware requirements or compare models, often at the early stages of AI development.
In other words, compute is used because it offers regulators a seemingly objective, easily measured threshold that sidesteps the complexity of evaluating actual AI capabilities and impacts. These characteristics, inter alia, make compute-based thresholds an attractive option for policymakers aiming to manage the risks associated with AI systems. Moreover, the use of compute as a regulatory proxy is closely tied to AI ‘doomer’ and long-termist narratives, which prioritize speculative existential threats over the more immediate and systemic harms AI poses today. At the same time, the compute thresholds function as a crude mechanism to differentiate between large tech firms and smaller developers, allowing regulators to concentrate oversight on a handful of dominant players without engaging with the full spectrum of risk.
In the US, for instance, the Executive Order on the Safe, Secure, and Trustworthy Development and Use of Artificial Intelligence sets compliance requirements for AI models trained with substantial computational power. Specifically, it defines high-risk models as those using over 10²⁶ FLOPs, while for biological sequence data, the threshold is 10²³ FLOPs. Meanwhile, the EU’s Artificial Intelligence Act is a landmark regulation that establishes a legal framework for the development and use of AI within the EU. It establishes a reporting requirement for AI models trained with more than 10²⁵ FLOPs, considering them to pose systemic risks. Although the specific FLOPs thresholds vary between the two jurisdictions, both require strict compliance measures for safety, security, and transparency once these limits are exceeded. But if they are not, current, real-world harms continue.
Regulating AI through technical specifications like compute thresholds seems to offer a simple and measurable framework. Yet, it fails to capture the real-world impact of these systems. Many of the most pressing risks of bias, surveillance, misinformation, and exclusion are not necessarily tied to how much computational power a model uses, but rather to how and where it’s applied, and who it affects.
A regulatory approach grounded in human rights, not compute
An effective regulatory approach must go beyond static technical metrics and instead evaluate AI systems based on their actual effects on people and society. This means asking how a model is used, what decisions it informs, who is impacted, and what safeguards are in place, irrespective of how much compute it requires. While it’s crucial to anticipate the potential risks posed by more advanced AI models, we must not lose sight of the existing AI systems that are already deeply embedded in society, influencing decisions that affect the day-to-day lives of millions of people in areas like employment, healthcare, education, and public services.
It is already clear that AI innovation is outpacing the logic of FLOP-based regulation. And even where the automated systems are simple and small, the harms can be significant. As a result, high-impact models that fall below a compute threshold could face less scrutiny, with potentially harmful AI escaping regulatory oversight. Yet, there still remains a risk that highly capable and also potentially harmful AI could operate with little oversight. Smaller models that match or exceed the performance of larger ones that use more compute could still pose significant risks without triggering regulatory oversight.
If regulation is tied too closely to compute thresholds, developers may deliberately optimize AI models to stay below these limits. This could result in AI systems that bypass regulatory scrutiny despite having a comparable or even greater adverse impact than heavily regulated models. If smaller, optimized models are used for autonomous decision-making or for mass surveillance, their risks could become more widespread and harder to mitigate. This is why we need to revisit our assumptions about what makes an AI model risky.
Recommendations for a stronger human rights-based framework
With that in mind, here are key recommendations for a stronger human rights-based regulatory framework:
- Use FLOPs as one input among many. Relying on them as the main indicator of risk is shortsighted. Multiple criteria should be used to assess model risk, reducing the limitations of any one measure. These could include evaluating the model’s specific deployment context, conducting qualitative risk assessments based on different types of harm, and considering factors such as the severity of the consequences of that harm, its long-term implications, and whether the harm is preventable or systemic.
- Combine compute-based thresholds with capability evaluations and impact assessments that evaluate real-world effects. While compute thresholds can provide useful insights into the technical scale of an AI system, they do not fully capture the broader societal risks posed by these technologies. Capability evaluations would assess an AI model’s ability to perform complex tasks, while impact assessments would examine the potential harms or benefits resulting from the deployment of the AI in various real-world contexts.
- Introduce dynamic regulatory thresholds that evolve with technological advancement. Instead of using a fixed, one-size-fits-all threshold (like a specific number of FLOPs), which might quickly become outdated, AI models need to be assessed relative to the best-performing ones at any given time.This approach compares new models to the current top performers, providing a more accurate assessment. It would also reduce the need for policymakers to constantly revisit, revise, and redefine a fixed threshold as technology evolves. Categorizing these dynamic thresholds by modality would further enhance their specificity and effectiveness, allowing for clearer distinctions between models with similar characteristics.
- Implement domain-specific requirements that acknowledge different risk profiles across sectors. AI systems used in sensitive areas like healthcare, criminal justice, or finance often pose higher risks due to their potential impact on people’s lives and well-being. By tailoring regulations to the specific needs and risks of each sector, regulators can ensure that AI technologies are deployed responsibly, with appropriate safeguards in place to mitigate sector-specific harms and protect human rights.
To develop meaningful, future-proof AI governance, we must look beyond FLOPs. It’s not just that factors like training data, deployment context, or safety interventions play a role in assessing risk; it’s that the combination of these factors that makes a model capable, safe, or risky is constantly evolving. Hinging regulation on any single variable, like compute thresholds, assumes a static relationship between that variable and risk. But in reality, the dynamics of model development are shifting fast. Improvements in data quality, training techniques, fine-tuning methods, and model architecture are all enabling powerful capabilities without needing more compute.
Tomorrow’s most capable model might not be the biggest; it might just be the smartest combination of factors. That’s why AI regulation must be adaptable and outcomes-based. It should account for how various elements interact and prioritize the actual impact a system has on people and society.
Authors
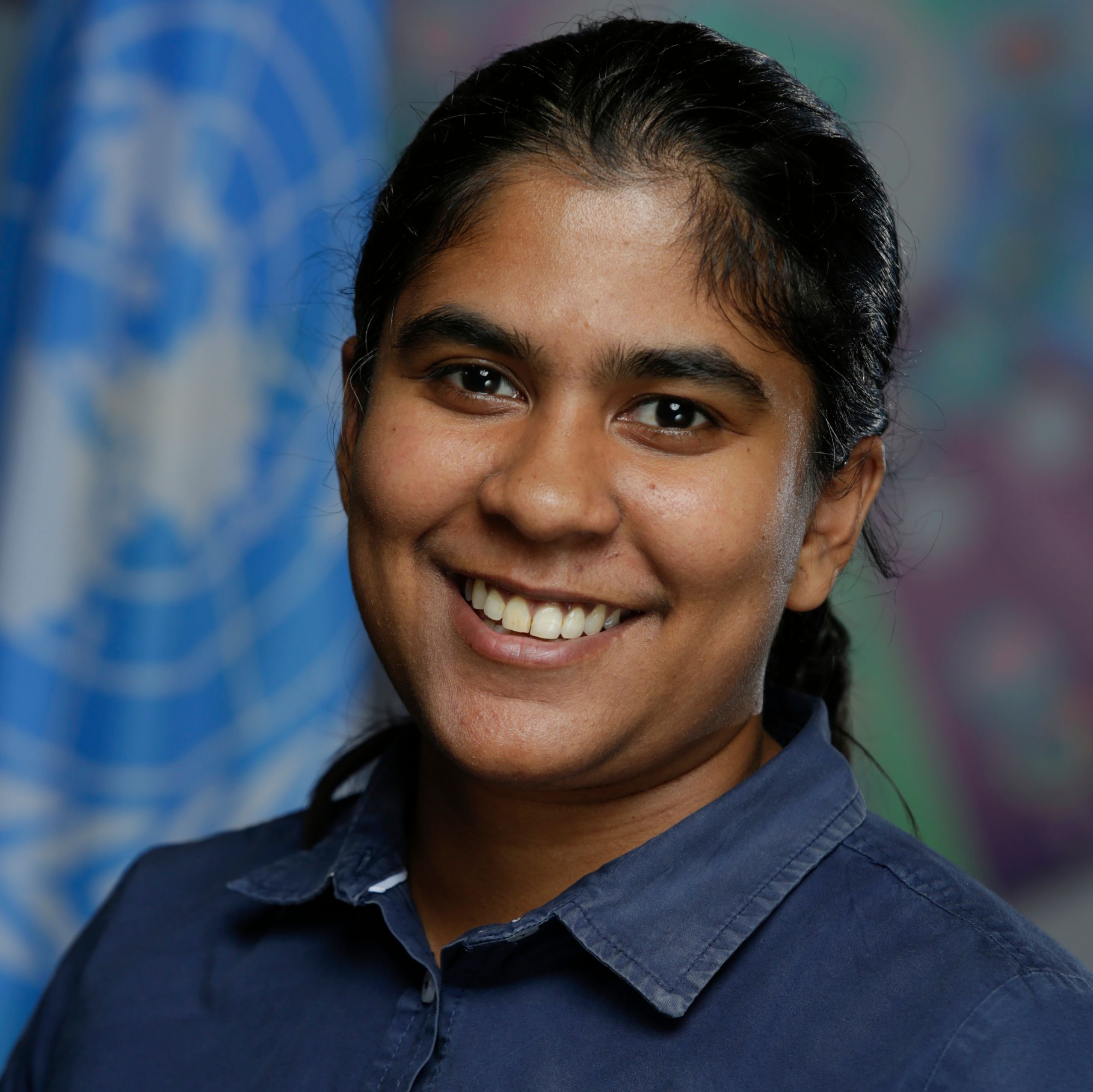