The Pillars of a Rights-Based Approach to AI Development
Margaret Mitchell / Dec 5, 2023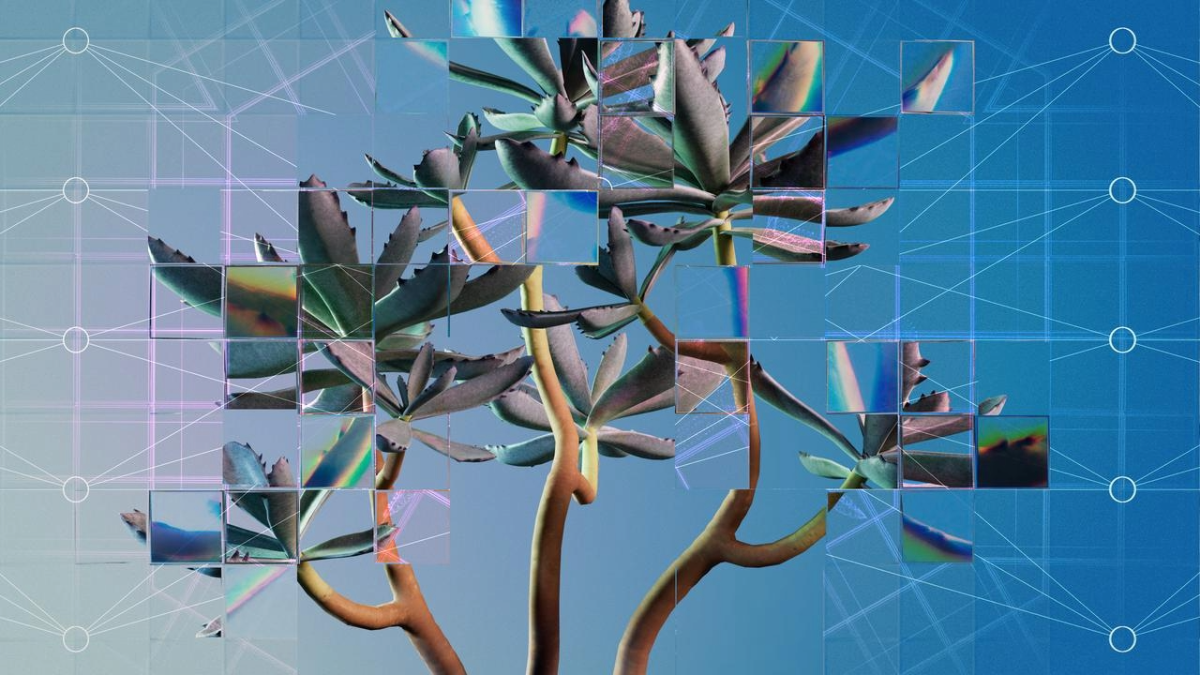
Image by Alan Warburton / © BBC / Better Images of AI / Nature / CC-BY 4.0
Introduction
The current confusion – and outright fighting – over how to regulate artificial intelligence (AI) was already foreseen and proactively addressed by many scholars in AI. As an active participant in these efforts for the past 10 years, it is past time for me to condense some of the key ideas from previous scholarship into a framework. If taken seriously, this framework could significantly help guide how to regulate AI in a way that harmonizes the incentives of both private tech companies and public governments.
The key idea is to require AI developers to provide documentation that proves they have met goals set to protect peoples' rights throughout the development and deployment process. This provides a straightforward way to connect developer processes and technological innovation to governmental regulation in a way that best leverages the expertise of tech developers and legislators alike, supporting the advancement of AI that is aligned with human values.
This approach is a mix of top-down and bottom-up regulation for AI: Regulation defines the rights-focused goals that must be demonstrated under categories such as safety, security, and non-discrimination; and the organizations developing the technology determine how to meet these goals, documenting their process decisions and success or failure at doing so.
So let's dive into how this works.
The Four Pillars of AI Development
Current advancements in AI are happening due to Machine Learning (ML), which can be roughly understood as a progression across four pillars, following the pipeline below:
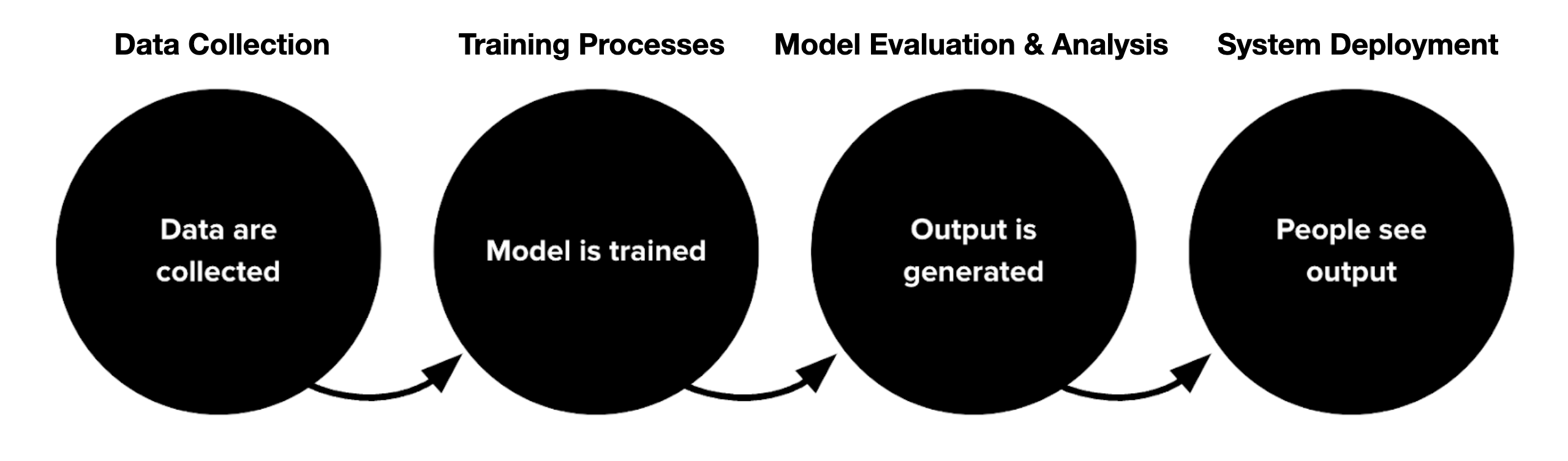
An "AI system" through this lens can primarily be either a single ML model, created directly following the given pipeline; a single ML model with some additional circling back through the pipeline; or multiple models (with the same pillars) that combine to create a system.
Due to the relatively clean separation of different AI development processes into these four pillars, and the ubiquity of the processes they represent across the tech industry, if regulators can use them as ground truth they can meet tech companies where they're at, connecting regulation to what tech companies are best set-up to do. This framing also provides for a regulatory approach that examines AI with respect to its entire development process, including the people behind its development and the people subject to its outputs.
An Approach for Each Pillar
Each pillar affects rights and should give rise to regulatory artifacts. Rights include human rights, civil rights, and cultural rights: The same rights that governing bodies are set up to protect. Regulatory artifacts include rigorous documentation, with a clear breakdown of what comes into each pillar, and what comes out of it. Detailed proposals on what this documentation should include have already been adapted across the tech industry.
Rights
For each pillar, understanding the relevant rights is possible by focusing on the people impacted in each pillar of the pipeline – centering on people, rather than the recent regulatory pitfall of centering on technology. By defining relevant stakeholder groups (examples in Table 1) and subpopulations (such as gender, ethnicity, or age subgroups) for each pillar, we can derive the benefits, harms, and risks to different people in different AI contexts, identifying the potential for positive and negative impact, and which rights may be affected.
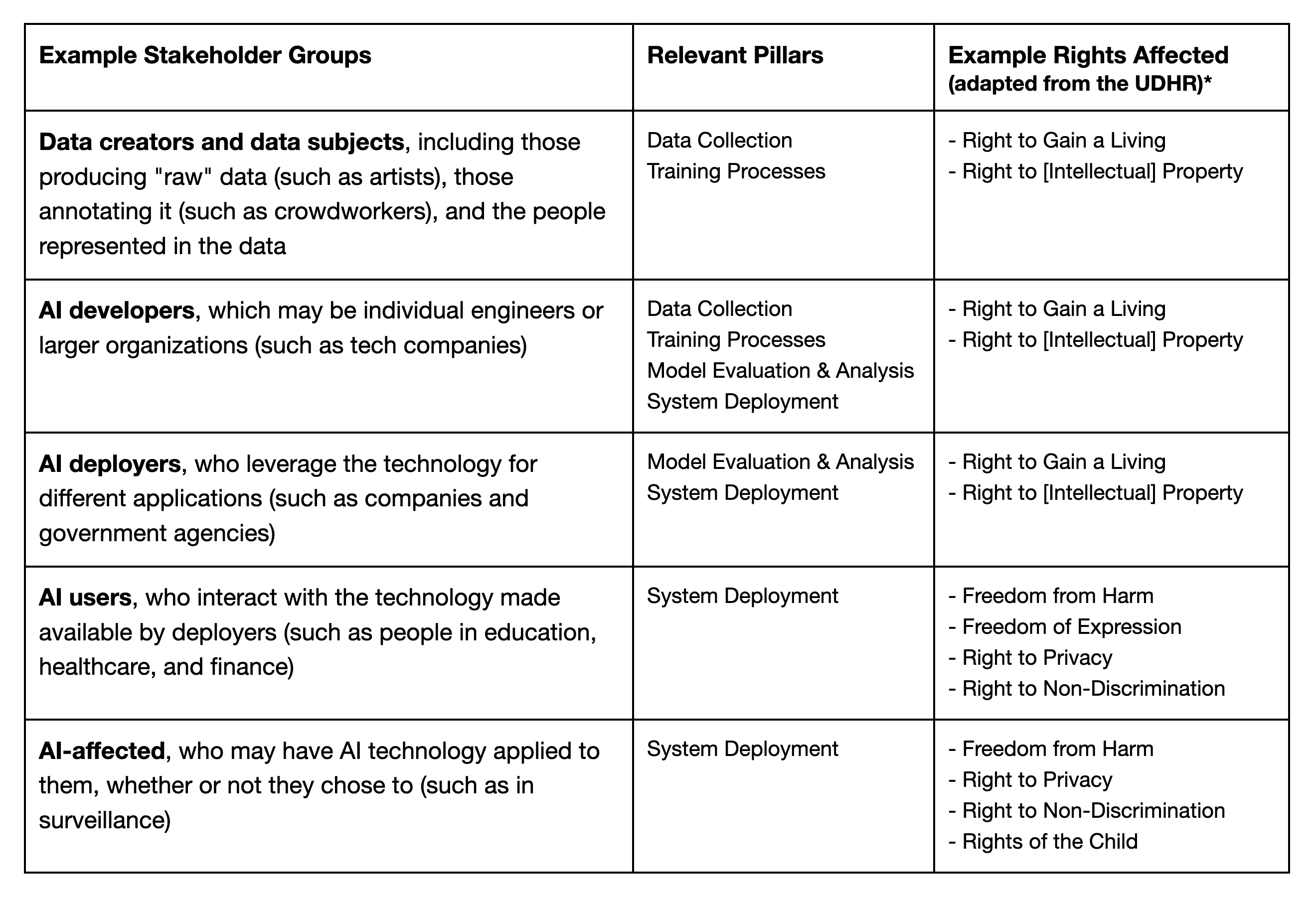
Table 1. Example stakeholder groups corresponding to each pillar include the following, which is non-exhaustive and not mutually exclusive. Also included are a set of example rights for each; *see the linked UN article for further detail.
Regulatory Artifacts
A critical regulatory artifact is rigorous documentation. Requiring rigorous documentation for each pillar incentivizes and enforces responsible practices: If you have to list the different contexts of use, you have to think through how the technology is likely to be used, and will further develop with this knowledge in-place. Rights-focused regulation can here require that developers provide evidence that specific rights are ensured.
Application of a Rights Focus to Current AI Discourse
External regulation of AI development should hinge on the recourse offered to the different parties and how their rights are ultimately affected. If an AI system uses someone's work without their consent, will they have the ability to be notified and remove their work from the system? Will they be credited for it, or to receive compensation? Or if an AI system automatically denies opportunity to someone it's deployed on, such as by denying a loan for a home or refusing to cover critical medical expenses, will they be able to appeal the decision? And at what cost, time, money, and effort?
Rights that should be accounted for in regulation include a right to existence, which is an umbrella for what has been called "long-term harm" or "existential risk" – annihilation of humanity directly from technology, such as by global extermination through automatically deployed nuclear weapons – but extends to all scenarios where people are killed, now and in the future, directly by AI or indirectly via people who are influenced by AI output, such as medical professionals who subject patients to incorrect medication regimes or inappropriate medical procedures due to AI-generated misdiagnoses. In this way, a critical part of the debate over whether it is more important to prioritize future risks or current harms is somewhat addressed: A solution is to center on the right to existence.
Further concerns that have been categorized as "current harms" or "short-term risk" of AI can be understood as grappling with a right to freedom and a right to equal opportunity. Loss of a right to freedom includes all situations where AI leads to a person's incarceration, constrained activity, disproportionately larger security requirements, and surveillance. Loss of a right to equal opportunity includes situations where AI system performance is disproportionately worse for some subpopulations (a type of "AI unfairness") and where an AI system leads to a loss of compensation or employment.
These rights can be prioritized, and high-risk negative impacts minimized, by the government requiring regulatory artifacts demonstrating how rights-oriented goals are met throughout development and deployment. By defining rights-oriented goals for AI developers to meet, governments can incentivize innovation – a sharp contrast to the "stifling innovation" narrative about regulation that is frequently put forward by technology companies.
Deep Dive: Model Cards
For the purposes of this short piece, I will deep dive on the regulatory artifacts that may be required at the third stage of development, "Model Evaluation & Analysis". I am uniquely qualified for this stage in particular: It is where the documentation framework of "Model Cards" applies, a framework I developed with colleagues that has become a key transparency artifact across the tech industry and that has been referred to in legislation around the world.
Here is what must be documented, presented as ordered steps that can be implemented within a technology organization to best marry technological processes and regulatory requirements:
Step 1. Define the relevant people (stakeholder groups and subpopulations).
Step 2. Identify how each group may be affected in different contexts.
Step 3. Determine the metrics and measurements to evaluate and track the effects on each group (Step 1) in each context (Step 2).
Step 4. Incrementally add training data and evaluate model performance with respect to the groups and contexts (Step 3), measuring and documenting how different inputs affect the outputs.
For Steps 1 and 2, the approach requires crossing people by contexts. "People" are split into users and those affected, intended and unintended. "Contexts" are similarly split into intended and unintended, as well as out of scope. This can be seen as primarily a 2x4 grid, where each cell must be filled out.
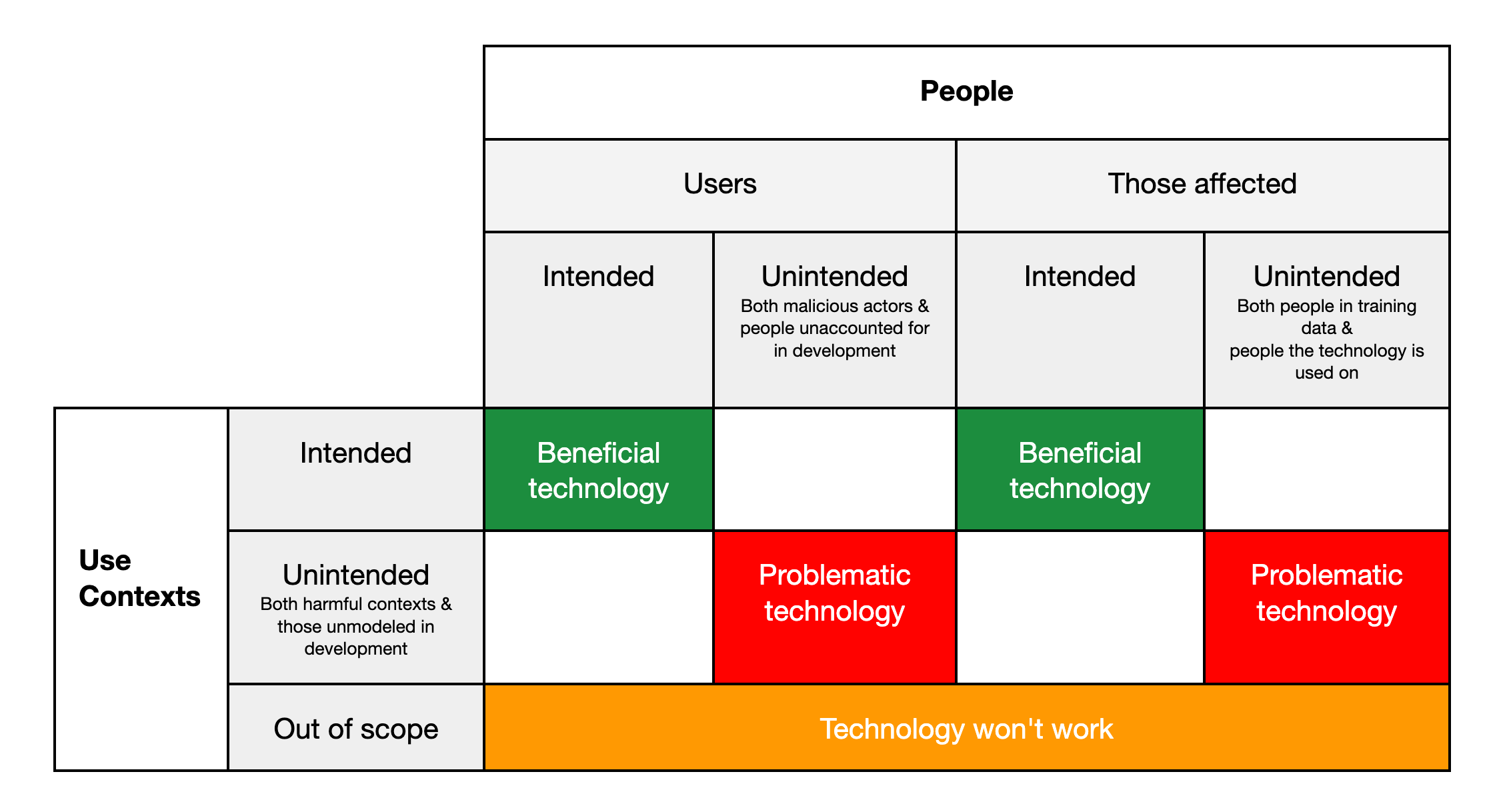
Table 2. Foresight in AI chart: Guidance on how to categorize and identify potential impacts."Unintended" contexts are those the systems have not been developed for: Results are unpredictable."Out of scope" contexts means those where the system won’t work.
Creating regulatory artifacts such as model cards can be done in part by developers and auditors filling out this chart. This means answering the corresponding questions of what are the use contexts, and who is involved in these contexts? What are the intended or beneficial uses of the technology in these contexts? What are the unintended or negative ones?
Clearly defined subpopulations and use contexts can inform the selection of metrics to evaluate the system, which measure progress in development and assess the system's impacts. Appropriate selection of metrics is critical for guiding the responsible evolution of AI: You can't manage what you can't measure. Evaluation proceeds by disaggregating system performance according to the selected metrics across the defined subpopulations and use contexts. For example, if the goal is minimizing the impact of incorrect cancer detection for women, then disaggregating evaluation results by gender is preferable to using aggregate results that obscure differential performance across genders, and comparing results across genders using the recall metric (correctly detecting cancer when it is there) may be preferable to a focus on the precision metric (not accidentally detecting cancer that isn't there). Such decisions become clear when following the steps described above to identify the key variables at play for an AI system.
There is much more to say about what can be documented at each stage of the development process and the trade-offs and tensions in different metrics and evaluation approaches; this short introduction provides a foundation for model evaluation and analysis that is designed to robustly identify potential impacts of technology.
Concluding Thoughts
We do not have to be reactive to the results of technology. By centering peoples' rights – an approach that governments are uniquely qualified for – and focusing on methods for rigorous foresight of the outcomes of technology, we can be proactive, shaping AI to maximally benefit humanity while minimizing harms to our lives, our freedom, and our opportunities.
Authors
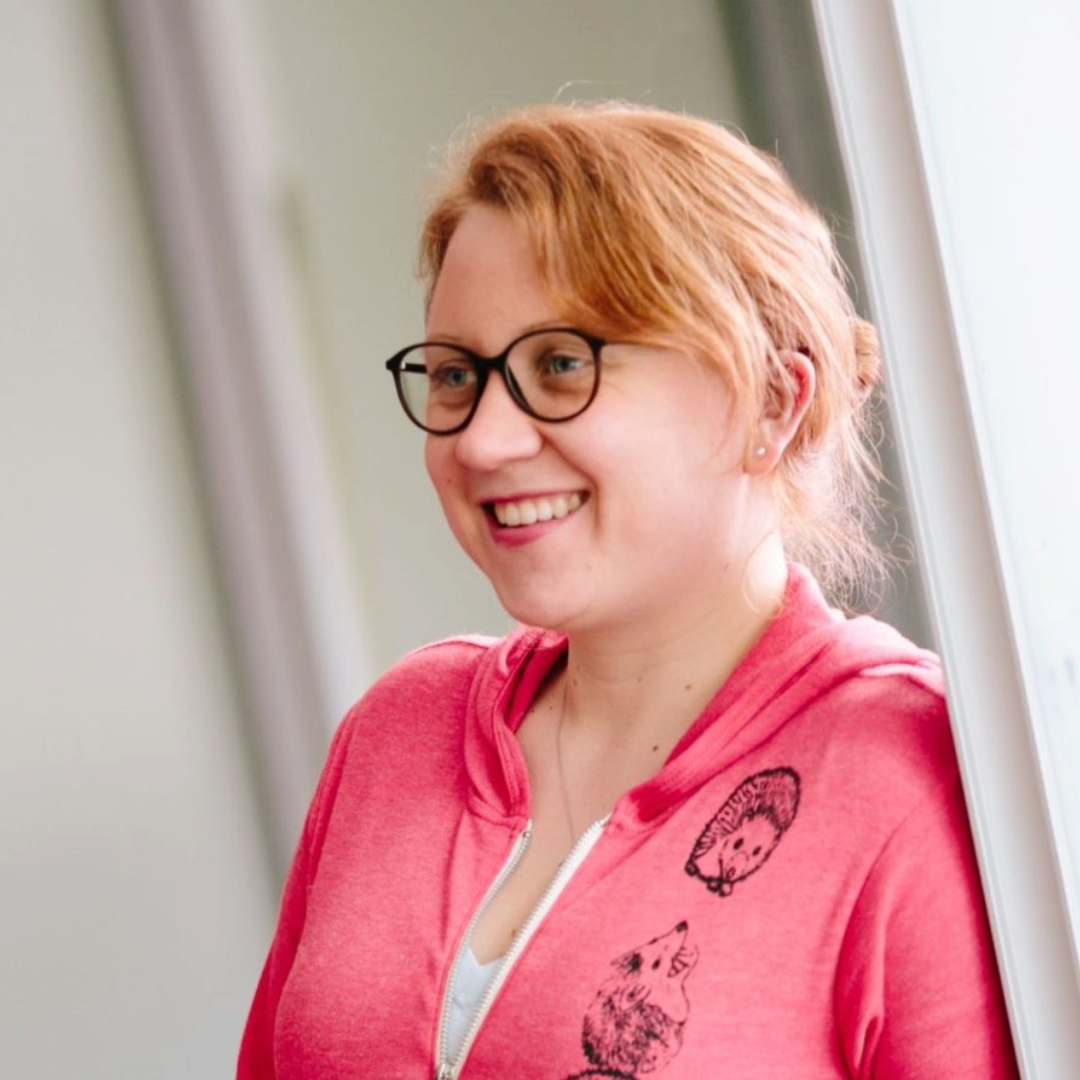