Navigating AI Safety: A Socio-Technical and Risk-Based Approach to Policy Design
Gautam Misra, Supratik Mitra / Dec 19, 2024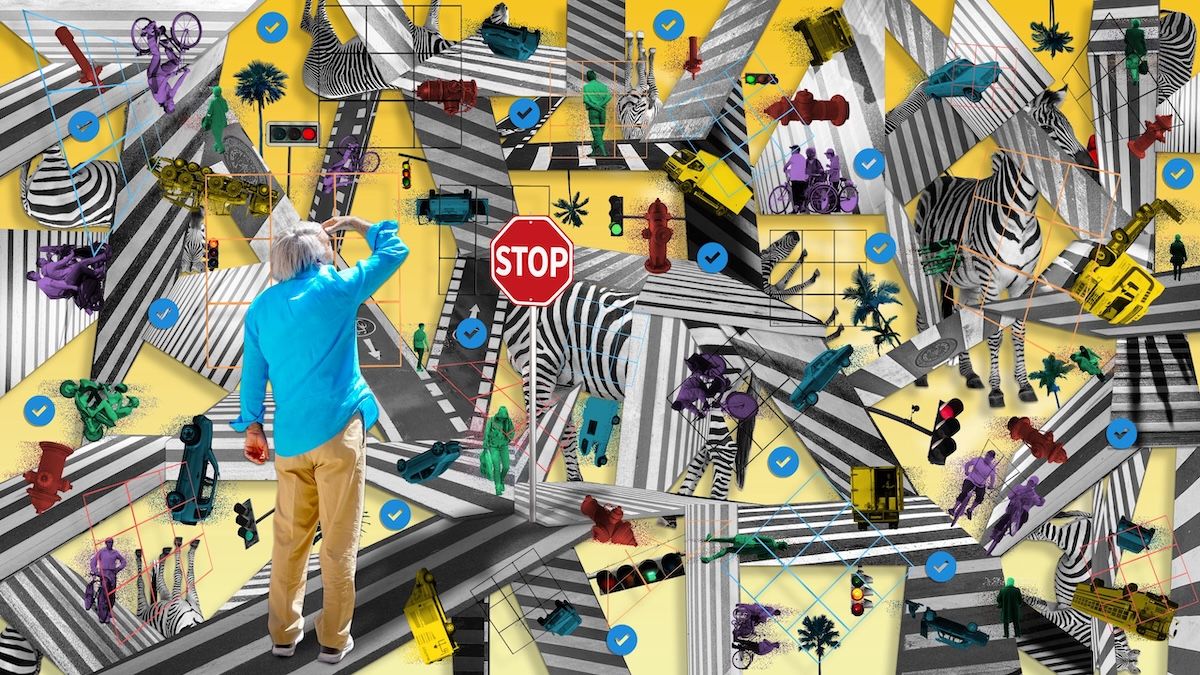
Anne Fehres and Luke Conroy & AI4Media / Better Images of AI / Hidden Labour of Internet Browsing / CC-BY 4.0
AI safety isn’t just about preventing harm; it requires a holistic understanding encompassing trustworthiness, responsibility, and the complex interplay between technology and society. Actors across the AI value chain—regulators, civil society, technologists, and other stakeholders—must grapple with the question: Is safety a standalone goal, or is it an integral part of broader frameworks of trust and responsibility? Trust in AI systems hinges not only on technical reliability but also on ethical considerations such as fairness, transparency, explainability, and bias mitigation. Without trust, safety measures lack impact, and without responsibility, those measures risk being ineffective or even misused.
To address these challenges, it is essential to combine a socio-technical approach with a risk-based approach. A socio-technical framework allows us to understand AI safety within its broader societal context. It emphasizes that AI systems don’t exist in isolation but interact with governance models, societal values, and human lives. This lens broadens the discussion of AI safety beyond technical fixes, highlighting the importance of understanding how human systems and technology co-evolve. At the same time, a risk-based approach categorizes AI systems by their potential impact—low, medium, or high risk—ensuring that governance mechanisms are calibrated to the level of risk posed by each system.
In the following sections, we further elucidate how integrating a socio-technical lens with a risk-based framework offers a more comprehensive understanding of AI safety, allowing stakeholders to design dynamic and inclusive governance structures that promote responsible AI development while addressing its inherent risks. We also shed light on the value chain ontology, with which these two approaches can be effectively mediated.
The socio-technical framework
The socio-technical framing, which originated with research looking at coal mining and labor studies in Britain, aimed to reshape how humans and technology are viewed in conjunction. The central tenet of the framing involved a recalibration of the dominant technocratic narrative, where technologies of the time were considered imperative and humans to be dispensable. In its attempt to confront this perspective, the sociotechnical system looks at the technical and the social as two subsystems that interact with each other to create a suprasystem (socio-technical system). The efficacy of the broader system involves the effective interaction and collaboration between the two subsystems. Thus, the socio-technical system is interested in looking at the ‘middle,’ between the social and technical, to understand the success and failure of larger systems.
Framing AI as a sociotechnical system is an empirical method to understand how technologies operate in the broader context. It recognizes that the outcomes produced by AI models and systems are a product of both the technical design and broader social factors (organization bureaucracy, social conventions, power structures, and human labor). Treating the technical and social as one coherent unit then expands our scope beyond the mechanical working of the technology to how these machines interact, change, and harm existing social systems. Additionally, as a method to gauge governance, it allows policymakers and researchers to think about AI safety from a broader lens. Granting more levers for governance and prompting an innovative policy design approach to the question of AI safety.
A value chain ontology goes hand-in-hand with this sociotechnical framework. It is a distinct ontology that encapsulates a broad network of actors, resources, stages, interrelationships, and situational social, cultural, and economic contexts inherent in the development process of any technology or product. The value chain can operationalize the socio-technical understanding of AI systems by plotting a broad scope of elements that work together to produce AI systems, encompassing both the technical design and social factors. This allows us to engage with nuances crucial to holistically promoting AI safety.
While such an ontological position for AI systems is still in its infancy, the value chain framework has helped researchers have a better look at the AI lifecycle. These academic discourses have emphasized the need to move from an abstract decontextualized discussion on AI ethics and governance towards a value chain perspective that places actors within their specific contexts and considers the diverse resources involved in co-creating AI systems. In line with this approach, policy practitioners and civil society organizations have also started using the value chain perspective to assess risks, implement mitigation strategies, and develop regulations tailored to the specific contexts of AI systems.
The Partnership on AI used a value chain approach in its research looking at risk mitigation strategies for open foundation models, allowing it to account for the non-linearity in the AI model development. This research identifies multiple actors, both active and latent, who contribute to AI development at various stages. Similarly, the Information Technology Industry Council (ITI) leverages the value chain perspective in its policy guide that advocates for informed policy decisions regarding foundation models.
A socio-technical framework for AI safety
The socio-technical framing, while being a powerful analytical tool to analyze outcomes of technology, its use in current responsible AI literature has been inconsistent. While often loosely defined to mean the interaction between technology and society, this simplification, though not entirely inaccurate, obscures the specific values of the sociotechnical perspective in AI policy. Thus, to begin thinking about AI governance and policy design from a socio-technical perspective, it is helpful to consider three key factors.
The social system around technology: The perception of AI as an independent, intelligent being has prompted a generalized view that divorces its impact on society. This has led to an overemphasis on regulations regarding technical specifications and machine learning techniques. This view misses that significant, if not all, positive and negative outcomes of AI arise from its interaction with other systems like the social. A sociotechnical perspective, however, allows us to broaden the scope from technical design thinking to address its interaction with people, institutions, and processes. Policy design, thus, must incorporate a broader sociotechnical system mindset, allowing for innovative policy that addresses both the technical and social.
- Example: While many AI models have made commendable improvements in their ability to detect symptoms of diseases and improve patient care. The inability of these systems to improve overall healthcare can be a problem of incompatibility with existing systems of patient care.
Problem selection: The ‘AI promise’ markets AI to be the solution for a plethora of global issues, from climate change to healthcare, but often overlooks the role of other systemic issues, such as politics, economics, and culture, in contributing to problems. Sociotechnical scholarship highlights how technologies like AI, when applied to complex social issues shaped by broader socio-political systems, have not only failed to address the problems but rather have exasperated them. Thus, ensuring AI systems are technically accurate and "fair" does not address the deeper systemic issues that contribute to algorithmic harms. Innovative policy design must also recognize the limitations of relying on technology to fix entrenched social problems.
- Example: AI systems designed to solve systemic issues without recognizing the root of the problems can end up aggravating and manufacturing harm by becoming punitive technologies that target marginalized communities.
Power inequalities: Power manifests in various ways, such as the concentration of capital and resources in the hands of a dominant few. Within the AI lifecycle, power flows unevenly from upstream to downstream actors, diminishing downstream actors' ability to influence AI safety discourse and policy, as well as the technology itself. The sociotechnical perspective examines the underlying power dynamics to better understand the problem and develop mitigation strategies by considering the social forces that influence technology's real-world performance. Therefore, policy design that addresses the broader power dynamics and social structures across actors and stakeholders is key to constructively administering accountability and responsibility.
- Example: The technical focus on developing fair and responsible AI systems has led to practices like debiasing and cleaning datasets from harmful content becoming standard. However, these practices have caused harm to the communities and workers tasked with such activities, who are often underpaid and lack voice and representation within the AI ecosystem.
An AI policy approach informed by these socio-technical factors is critical to moving away from technocratic regulatory models. Such an approach can provide a clearer understanding of AI technology's potential harms and opportunities. While navigating AI development is challenging, a sociotechnical approach that considers the value chain ontology offers a comprehensive and nuanced analytical framework. This approach creates richer opportunities for innovative policy design for AI safety.
Risk-based thinking for AI safety
Integrating a risk-based approach to AI safety, such as categorizing AI systems into low, medium, and high-risk use cases and framing this within socio-technical perspectives, is imperative to an environment more conducive to demystifying AI safety and its best practices.
A risk-based approach to AI safety is crucial in addressing the wide variety of AI applications, each of which carries different levels of risk based on its context, purpose, and design. Low-risk systems, such as those that assist with routine tasks (e.g., recommendation engines), pose minimal harm to users and require lighter regulatory oversight. Medium-risk systems, such as those used in education, warrant greater scrutiny due to their significant human impact, especially on children. Finally, high-risk AI systems—such as those used in healthcare, criminal justice, or autonomous vehicles—carry the most severe potential for harm due to the potential for bias and thus demand stringent safeguards, including enhanced transparency, accountability, and robustness.
However, managing these risks also demands ay a socio-technical approach that recognizes AI’s interaction with social, legal, and ethical norms. This approach underscores that safety outcomes are contingent on both the technical design and the broader human context, including power dynamics, labor considerations, and institutional practices.
Using a value chain ontology further helps to identify pain points within the AI lifecycle where risks can become entrenched. It also allows us to identify the roles and responsibilities of certain stakeholders, primarily influential tech companies and regulators, and then map the unique roles each has in promoting AI safety within different risk categories. Collaboration between tech builders and regulators is central to advancing AI safety, particularly when viewed through a sociotechnical framework. This framework emphasizes the interdependence between technological innovation and societal values, creating a space for these two core stakeholders to jointly shape AI’s trajectory in ways that are responsible, inclusive, and adaptive.
The role of tech companies and AI innovators
Tech companies and AI innovators drive the development and deployment of AI systems, making them central to ensuring these technologies are safe, reliable, and ethical. Their access to vast resources, technical expertise, and data enables them to innovate rapidly but also increases their responsibility to address AI risks proactively. AI innovators must take the lead in embedding safety by design, developing tools for transparency, and ensuring that AI systems meet ethical standards such as fairness and bias mitigation.
However, their drive for innovation and global scale often places them beyond the reach of traditional governance mechanisms, creating challenges for regulators. Collaboration is, therefore, critical. Tech companies can provide regulators with insights into technical complexities, emerging risks, and the limitations of current governance models, enabling regulators to develop evidence-based, adaptive policies.
The role of regulators
Conversely, regulators safeguard the public interest by setting enforceable boundaries for AI systems. They establish standards to ensure accountability and trustworthiness in AI applications, addressing issues such as data privacy, algorithmic transparency, and system robustness. Regulators must also balance the dual objectives of AI’s impact on society and streamline, avoiding overly prescriptive regulations that could stifle progress.
By engaging with AI innovators, regulators can develop risk-based governance frameworks informed by technical realities while addressing societal needs. For example, regulatory sandboxes allow for controlled testing of high-risk AI systems, ensuring their safety before broader deployment. However, the stringency and lack of explainability of these regulatory sandboxes may slow down the policymaking process.
Importance of cooperation
A socio-technical framework underscores that neither tech companies nor regulators alone can address the complex challenges of AI safety. The pace of AI development requires iterative and collaborative governance models where:
- Tech companies provide technical expertise: Companies share insights into system vulnerabilities, testing methodologies, and the potential for misuse to help regulators craft effective policies.
- Regulators establish guardrails: Policymakers enforce standards to prevent exploitation or harm while incentivizing ethical AI practices.
- Shared responsibility drives innovation: Collaboration enables the development of flexible frameworks that adjust to the risk profiles of different AI systems. For example, regulators and AI innovators can co-design the protocols for high-risk applications such as healthcare or autonomous systems.
- Global coordination reduces fragmentation: Given the transnational nature of technology and policy challenges, collaboration aligns international norms and standards.
By combining foresight and collaboration, the sociotechnical partnership between regulators and AI innovators can ensure that AI development and deployment prioritize both innovation and societal well-being. Ultimately, AI safety must be more than a set of regulatory or technical goals but rather a shared responsibility that prioritizes the ideals of democracy, such as liberty, pluralism, and equality, along with technical principles such as accountability, transparency, and explainability.
Conclusion
By combining a socio-technical framework and a risk-based approach, we can create innovative, adaptive policy frameworks that balance safety with technological progress. Risk-based policies ensure that high-risk AI applications, such as those in healthcare, financial services, or autonomous systems, receive stringent oversight. In contrast, lower-risk applications are not overburdened with unnecessary regulation. Meanwhile, the socio-technical approach ensures that safety measures consider the technical aspects of AI systems and their social, ethical, and organizational contexts.
The value chain framework also provides a powerful tool to analyze the intricate interplay between technical and social dimensions within AI systems. By breaking down these systems, we can more precisely identify and assign nuanced, stage-specific risks relevant not only to the technical aspects but also to the broader social systems and stakeholders involved. This approach allows us to map risks to specific sectors and social actors, creating a more comprehensive understanding of how these risks emerge and propagate.
Thus, these frameworks, when combined with a value chain model, enable a more holistic operationalization of AI safety. They help bridge the gap between abstract risk management frameworks and the practical realities of AI governance, allowing for policies that are better informed, socially attuned, and effective in safeguarding AI's development and deployment.
We thank our colleague, Rattanmeek Kaur, for her input on the value chain approach.
Authors
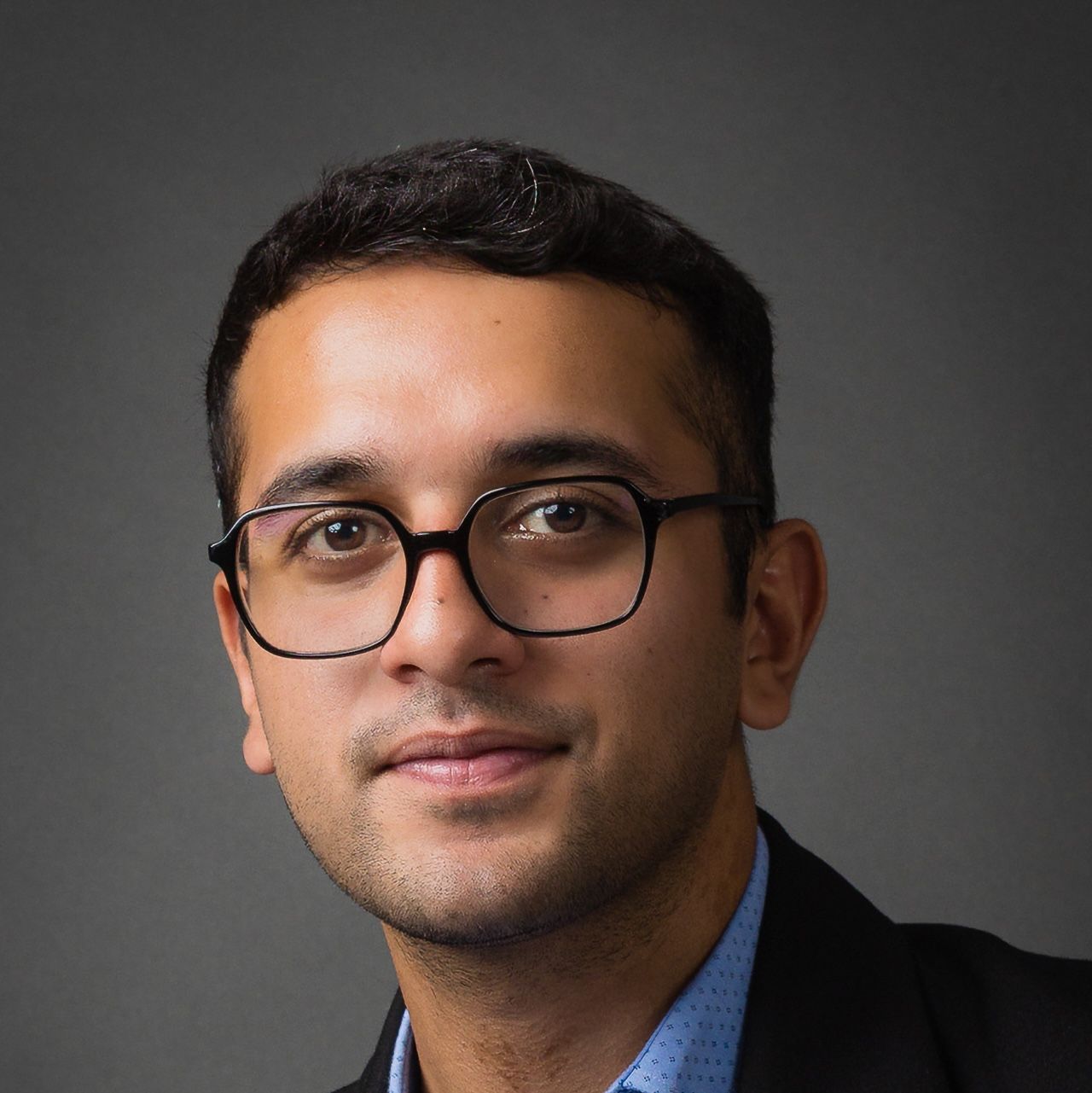
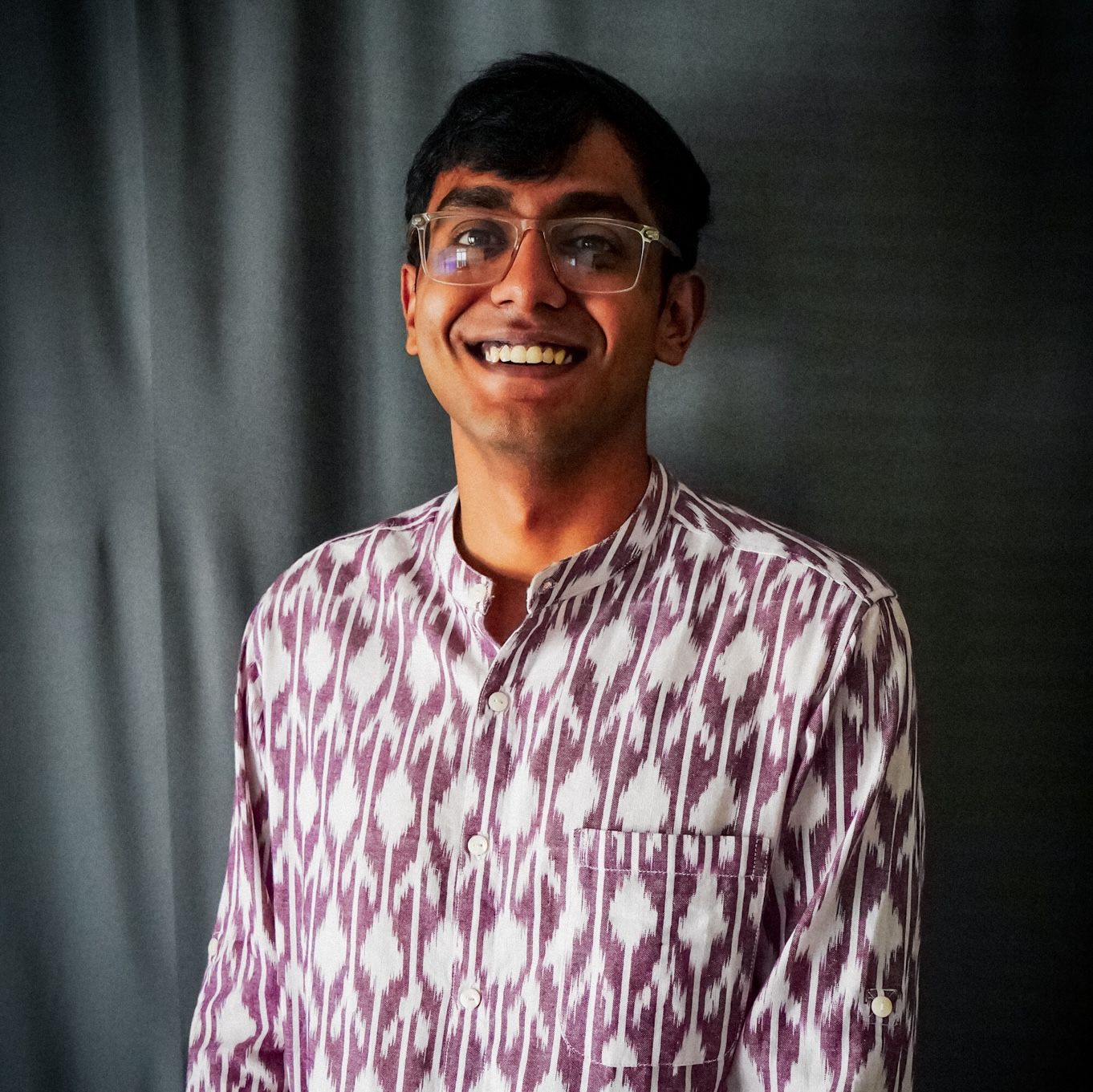