Amid Global AI Race, India’s Potential to Master the Art of Data Sharing
Arnav Nigam / May 8, 2025Arnav Nigam is an Associate Consultant at the Center for Responsible Technologies at Microsave Consulting (MSC). The firm works with government clients.
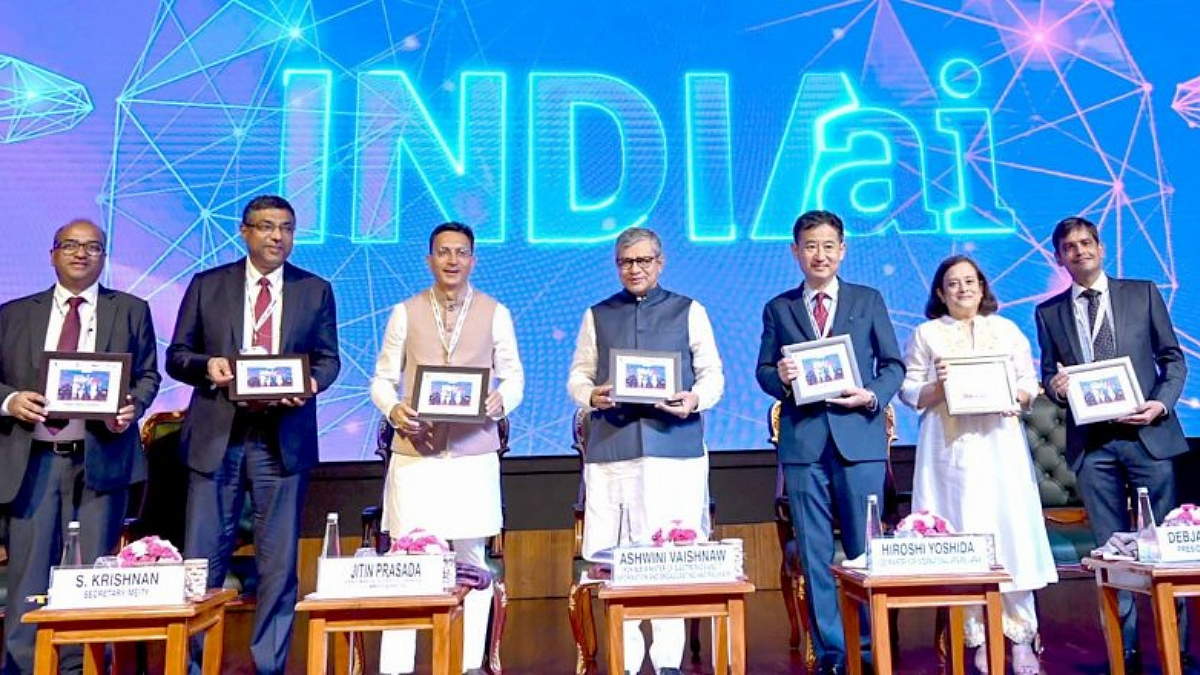
NEW DELHI—July 3, 2024: Officials gather for the Global IndiaAI Summit. Source
With the increasing adoption of generative AI, countries are advancing their strategies to regulate and leverage this transformative technology for their developmental goals. Over the past few years, many countries have integrated AI objectives into their national policies, aiming to both regulate and promote the technology. Notably, the African Union passed its Continental AI Strategy in July 2024, while ASEAN countries have introduced initiatives such as "AI-Ready ASEAN" and the "ASEAN Responsible AI Roadmap (2025-2030)." India, too, has been ambitious in AI adoption, introducing its National Strategy for Artificial Intelligence as early as June 2018.
However, the rapid evolution of AI has convinced many nations that it is necessary to take more proactive measures to advance indigenous AI development. India is among them. In January 2025, just days after China unveiled its DeepSeek R1 model, India’s Ministry of Electronics and Information Technology (MeitY) announced plans to develop a foundation model within a year. Through the India AI mission, MeitY has invited proposals from tech companies, startups, and researchers to build AI models trained on Indian language data.
While India’s national strategy for Artificial Intelligence (2018) focused on sectoral needs (including health, education, and agriculture) and reaping the benefits of AI in solving societal needs, it recognizes the importance of a robust data ecosystem and intellectual property framework, given the unique nature of AI application development.
AI’s potential hinges on data. Training models—whether large language models (LLMs) like ChatGPT and DeepSeek or specialized sectoral models—require vast, diverse, and contextualized datasets. However, the challenge lies in collecting, curating, and sharing this data responsibly for training AI models. Leading AI companies like OpenAI and DeepSeek have faced scrutiny over opaque data collection practices, raising ethical concerns. The question for India and other developing and emerging economies seeking to harness AI’s benefits is whether they can chart a different course on responsible AI development that differs from existing global models.
The third pillar of DPI: data exchange
India, with its billion-plus population and extensive digital footprint, has aspirations to be a data-rich nation, but the question is how to share this data effectively for AI model training. India has already demonstrated a unique approach to digital governance through its Digital Public Infrastructure (DPI) model. Currently, this vision of regulatory technologies comprises Digital Identity (Aadhaar) to facilitate identity verification, Digital Payments (UPI) to enable seamless financial transactions, and Data Exchange (which is yet to be fully realized) to establish structured mechanisms for data sharing among public and private entities.
Often compared to roads in a digital economy, these systems have been part of a broader strategy to transform public service delivery and financial inclusion. As of March 2025, the total number of Aadhaar authentications reached over 133 billion, and in October 2024, UPI processed 16.58 billion transactions, amounting to ₹23.49 lakh crore. However, the third pillar of DPI—data exchange— remains underdeveloped.
Traditionally, other DPI components have been use-case driven to get legitimized and illustrate their value to the broader public. For instance, Digital identity provides targeted social protection, and UPI provides seamless online transactions. Now, public AI can become the appropriate use case for developing a functional data exchange layer of DPI. Therefore, as AI reshapes economies, unlocking the potential of the data exchange layer is critical.
Consider healthcare: anonymized patient records could help AI predict disease outbreaks or optimize treatments. In agriculture, soil health and weather data could power AI-driven advisories for farmers. However, these opportunities remain untapped without structured mechanisms to pool such data while preserving privacy. India’s ability to ethically and efficiently harness its vast data resources will determine its place in the evolving AI landscape.
Look beyond LLMs when it comes to public AI
At this point, it is also important for developing and emerging economies (including India) to consider a more strategic approach, look beyond resource-intensive LLMs, and align their AI development and deployment with the national objectives and developmental goals. While global attention remains fixed on LLMs, developing economies’ real advantage may lie in small language models (SLMs) and sector-specific AI solutions. SLMs, requiring less computational power and data than LLMs, are ideal for localized applications, such as AI tools for regional language education or micro-credit underwriting. Sectoral models tailored to industries like healthcare, logistics, and climate resilience could address countries' unique challenges at the local and regional levels.
However, sectoral AI needs sectoral data. For instance, developing a crop-yield prediction model requires integrating data from farmers, agri-tech firms, and government agencies. Currently, this data exists in fragmented silos, constrained by bureaucracy, proprietary barriers, and privacy concerns.
The third pillar of DPI—data exchange—can provide the necessary technological and policy framework for developing high-quality context-specific datasets for public AI models. This may also be the first step towards public AI development and improving citizen services in the era of AI-led digital governance. However, unlike identity and payments, which involve direct, discrete transactions—such as verifying an individual’s credentials or facilitating financial transfers—data exchange operates on a more complex spectrum. It involves the continuous flow and reuse of information across different systems, raising concerns beyond mere functionality. Effective data exchange requires a careful balance between the necessary accessibility to foster innovation and efficiency and the privacy safeguards to protect individuals from misuse and breaches.
Looking ahead to ethical data sharing for responsible AI
Governments generate vast amounts of data, spanning sectors such as healthcare, agriculture, and climate monitoring. The data exchange layer essentially aims to make the responsible flow of data possible among public and private entities to understand and reap the data value.
With regards to government-held data, many countries have implemented data-sharing policies to harness public data for broader societal benefits, for instance, the open data policy in the US and the European Union’s Open Data Directive. India too introduced the National Data Sharing and Accessibility Policy (NDSAP) in 2012. Open data policy aimed to democratize publicly funded government-held data, however its impact has been limited due to persistent data silos, interoperability challenges, outdated datasets and often unavailability of critical datasets. Additionally, data published under NDSAP 2012 on the open government data portal in India is rarely useful for any AI model training due to inconsistent formats, standards, and data quality issues. Though the goal of open data policies across the world and NDSAP in India has been to enhance transparency and accountability in governance, revitalizing open data policies would be necessary for public AI development and making relevant high-quality government-owned datasets available. The government can also mandate the timely publication of non-personal, non-sensitive, high-value datasets (e.g., health, agriculture, climate).
To utilize privately owned non-personal non-sensitive datasets, private entities must be incentivized to contribute their data to national repositories. The recent AIKosh initiative by India AI, which invites private/non-governmental organizations to provide datasets for AI development, is a step in this direction.
In case of personal and sensitive data sharing for training and processing, a comprehensive mechanism should be developed, ensuring compliance with privacy safeguards and ethical considerations. To protect individual rights over their personal data, prevent data breaches, and ensure ethical and responsible sharing of data, India’s advantage may come from its DPI approach to digital governance, as the country has already laid the groundwork for a techno-legal solution in DPI. For example, in finance and payments, the Data Empowerment and Protection Architecture (DEPA) offers a consent-based framework for secure data sharing (though it is not for AI development, rather to provide financial services to users). DEPA’s “consent manager” (an account aggregator in the financial sector) enables individuals to securely share their financial data across banks and institutions and get financial services. Expanding DEPA’s principles to other sectors, individuals, and institutions can share data selectively, with audit trails ensuring accountability. Similarly, the Digital Personal Data Protection (DPDP) Act 2023 aims to protect the individual’s right over their digital personal data while recognizing the need to process such data for various purposes. However, its implementation rules remain under consultation, with critiques around privacy safeguards and legal mechanisms to protect individual rights. A fully operationalized DEPA framework, combined with a strengthened DPDP Act, could help India pioneer a balanced approach to data governance that avoids both China’s state-controlled model and the US’s market-driven model.
In the AI era, data's true value lies in its refinement and responsible flow. By leveraging its DPI legacy, India has the potential to create an AI-ready data ecosystem that is both open and ethical. As the world grapples with AI’s ethical dilemmas, India may have a unique opportunity to lead by example in data governance and AI.
Authors
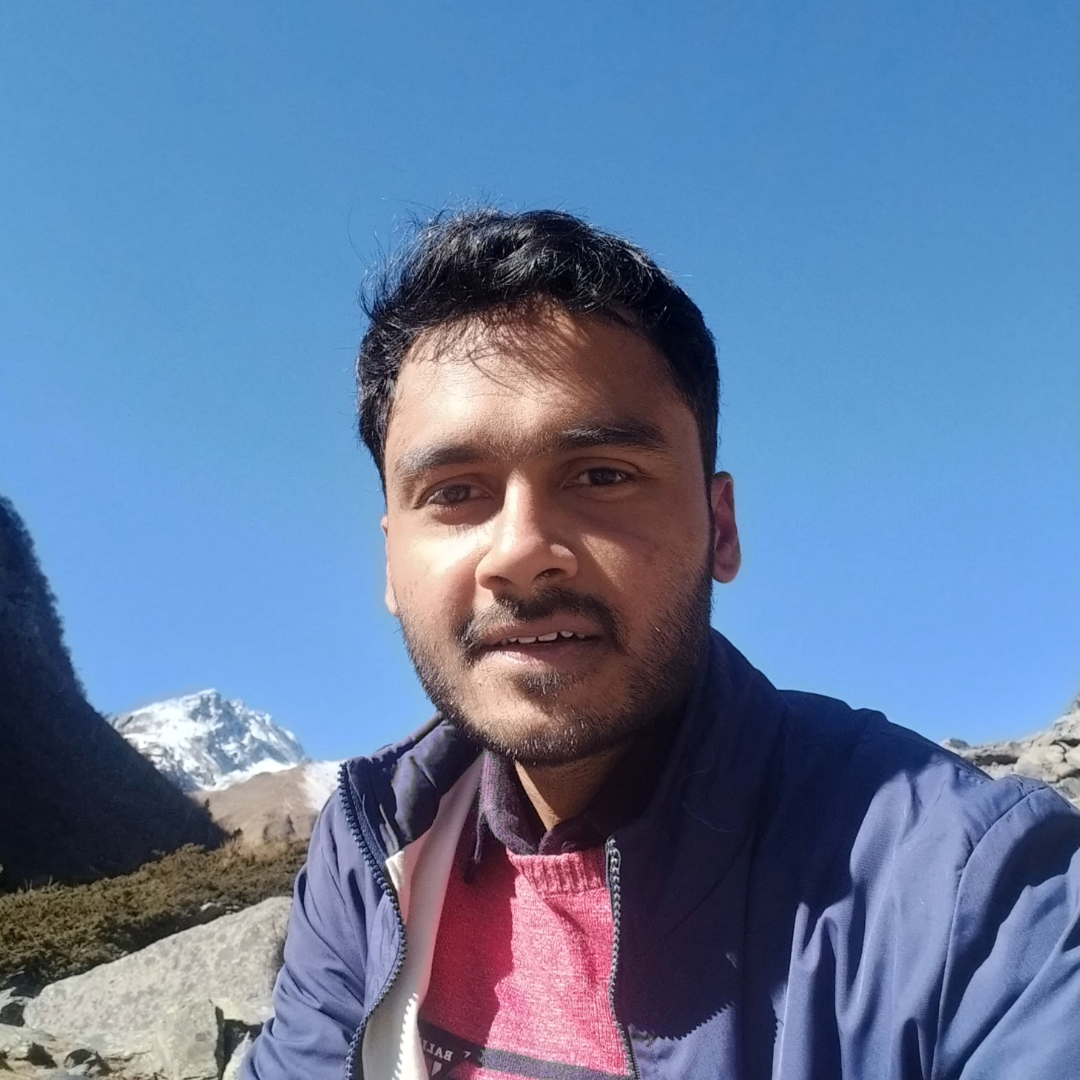