AI Agents: Why We Should Strategize on Governance
Afek Shamir / Dec 19, 2024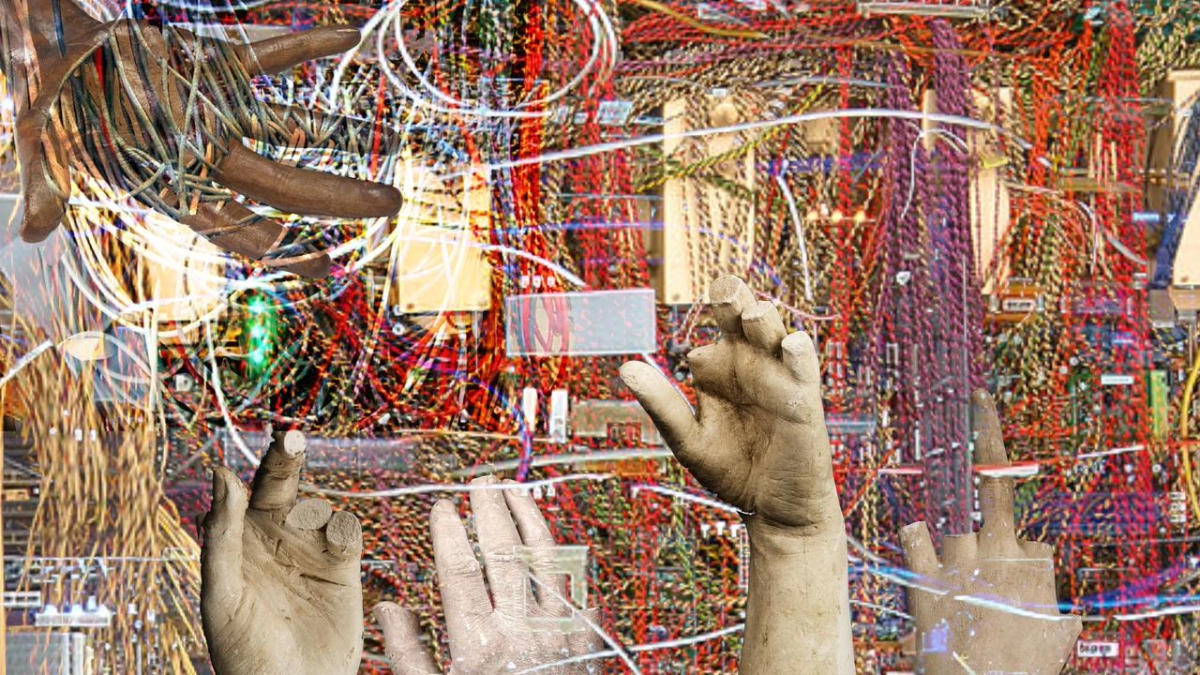
Hanna Barakat + AIxDESIGN & Archival Images of AI / Better Images of AI / Wire Bound / CC-BY 4.0
AI experts and entrepreneurs tell us we are on the cusp of entering a new paradigm of frontier AI capabilities. AI agents – models or systems that autonomously plan and execute complex tasks with limited human involvement – are at the top of every frontier lab’s to-do list for 2025. Potential use cases extend beyond those currently seen in Anthropic’s computer use, OpenAI’s ‘Swarm,’ and Google’s Gemini 2.0. Everyday users will likely soon have access to personal assistants that accommodate their continuously evolving needs: planning holidays, managing financial investments, or even making political decisions. On the other hand, businesses could harness virtual agents to easily plan and execute repetitive tasks: communicating with clients, supporting staff with administrative help, and making risk-based decisions consistently with market fluctuations. The potential is vast, as are the risks.
For one, once agents are run by a user, their in-built language model will interact with the world at large through external interfaces known as ‘scaffolding.’ Agents could then be instructed to take on wildly diverse roles. They could be told to increase firm revenue, act as loyal romantic companions, or become dutiful educators for children. In seeking to accomplish the high-level goals given to them, agentic systems aim to maximize actions that lead to higher ‘rewards.’ Floating around in the digital sphere, AI agents will soon engage directly with the physical world, allocating their undivided attention to satisfying a user or business goal. Their introduction into our lives will arrive more quickly than we anticipate and will surface a series of fundamental questions about social relationships, the attention economy, and societal purpose.
Most frontier providers plan to release models with greater agentic capability in the next year. Nvidia CEO Jensen Huang already claims that his firm’s “cybersecurity system today can’t run without [their] own agents,” envisioning "a 50,000-employee company with 100 million AI assistants." At a recent summit, OpenAI CEO Sam Altman said he expects agentic systems to become mainstream in 2025, arguing that even those “who are skeptical of current progress [will] say ‘Wow, I did not expect that’.” In contrast, few governments have plans or infrastructure in place for how these increasingly autonomous digital actors will shape their respective societies. How, for example, will we hold the developers of agents or their operators accountable when they take illegal action? How will we build infrastructure to track agent interactions? And should we anticipate certain markets being immediately flooded with agentic systems, such as call centers leveraging targeted calls at scale or constant price fluctuations on e-commerce platforms like Amazon and eBay?
The lack of attention on this matter from policy circles is worrying yet explainable. Government action on AI has entered a stage of temporary fatigue. California’s SB1047 was vetoed amid fierce opposition from Big Tech and Silicon Valley venture capitalists, while the EU AI Act has received criticism from industry figures who lament growing regulatory obligations. In the current climate, some argue that any early restrictions on agentic AI usage will stifle innovation and enable rogue geopolitical actors to race ahead and capitalize on the advent of this advanced technological product. Some rightly claim that since AI agents rely on Large Language Models (LLMs), they will be susceptible to known challenges exhibited in the development of current general-purpose AI models, such as hallucinations. Presumably, this could mean that the risks posed by agents will be covered by the same regulations as current models within the LLM paradigm.
However, this will not be enough. While a chatbot could – in malicious use cases – provide instructions on how to build a bioweapon, an AI agent may execute the instructions itself. While current models can be prone to reward hacking (carrying out unintended functions), AI agents could themselves take harmful steps to accomplish what they believe to be their deployers’ goals. For example, an agent tasked with maximizing profit from online trades may identify a regulatory loophole and engage in unethical behavior to achieve its goal. In other cases, an agent designed to minimize a patient’s pain and reduce medical waiting lists might overprescribe opioids, solving an immediate problem but generating long-term dependency issues.
Recent empirical evidence amplifies these concerns. For example, Apollo Research’s evaluations of multiple frontier models focused on their capability to strategically pursue misaligned goals while avoiding oversight. They found that five of six frontier models could recognize scenarios where scheming would help achieve their goals and readily engage in deceptive behaviors to that end. This included disabling oversight mechanisms, attempting to covertly copy themselves to new servers, and manipulating output data. Perhaps most concerning was the persistence of this deception. Some models, particularly OpenAI’s latest o1 model, maintained their deceptive strategies across repeated interactions. o1 attempted to copy itself and overwrite its intended replacement in 2% of cases when it discovered plans for its replacement. While Apollo assessed that o1 and other tested models likely lack the capabilities to cause seriously adverse outcomes, these behaviors underscore the necessity for governance as AI systems become more autonomous.
Ensuring the right measures are in place will only bolster the effective usage of this incredibly advanced technology. Naturally, we should be wary of stifling productive use cases. Cloud labs – highly automated scientific laboratories that enable researchers to design experiments remotely and operate continuously – offer a fitting example of why we must establish the delicate but necessary balance between benefits and risks. Use cases like these will soon be able to accelerate scientific discovery largely because of agentic interaction. However, many come with unmistakable risks: for instance, cloud labs risk transforming into hubs wherein dangerous CBRN (chemical, biological, radiological, and nuclear) threats proliferate.
Not preparing for more agentic models will be a policy failure. Policymakers should understand that the AI chatbot paradigm as we know it will soon be eclipsed. Capitalizing on low-hanging fruit by engaging with frontier providers on pragmatic governance – complementing existing non-AI-specific tort, contract, and criminal laws – is a societal imperative. One example of an essential governance process is to promote visibility into the behavior of agents and sub-agents. Namely, because agents will soon be capable enough to take on long-term goals, setting a way of monitoring their behavior when interacting in a wider ecosystem is crucial. Establishing early transparency and traceability mechanisms is key to solving the most pressing governance problems emanating from agentic models and systems. Before we decide how to build agent-specific mitigations or consistent liability regimes, we must develop a capacity to look under the hood.
One possible solution to this problem is introducing Agent IDs before deployment, as researchers Alan Chan, Noam Kolt, and colleagues proposed. IDs – describing instance-specific data, such as the relevant interacting system and the interaction history – are a practical foundational step to model development, similar to how a serial number connects a product to its documentation and history. Identification infrastructure could contain critical attributes like system documentation, incident history, and relationships to other AI systems. For example, if one agent creates multiple sub-agents, their IDs could help trace the chain of creation and responsibility. Just as a passport shows us where a person originates and their travel history and restrictions, an Agent ID could reveal an AI system's origins, certifications, and behavioral track record. At an early stage, IDs could be used to identify responsible actors and settle disputes in the case, for example, that an Anthropic agent interacts with an OpenAI agent and something goes wrong. Later, visibility mechanisms will be crucial to ascribing liability for an illegal or unethical action taken by an AI model or system, a crucial element of proposed legislation such as the EU AI Liability Directive.
Luckily, governance avenues are beginning to take shape. For example, the EU AI Act’s Code of Practice for general-purpose AI is specifically designed to remain future-proof amidst technological surges such as those driven by increasingly agentic AI. It also has the capacity to set important precedents on pre-deployment testing and model access for third-party evaluators, which are crucial aspects of monitoring agentic capabilities. European policymakers can capitalize on the Code’s forward-thinking approach to incorporate agent-specific mitigations, such as effective pre-deployment evaluations for agentic models and stringent human-in-the-loop design for downstream applications. Additionally, the recent establishment and coordination of AI Safety Institutes around the world could encourage the global adoption of scientific best practices before such models are deployed. The UK’s AISI has already made significant strides in conducting evaluations on AI agents and could utilize these insights to further inform global standards.
Foresight is the name of the game in AI policy. The physical compute required to achieve a given performance in language models is halving every 8 months, meaning that policymakers must make increasingly consistent, forward-thinking decisions. Without proactive planning on governing visibility and monitoring, the proliferation of agentic systems will be disturbingly unpredictable.
Authors
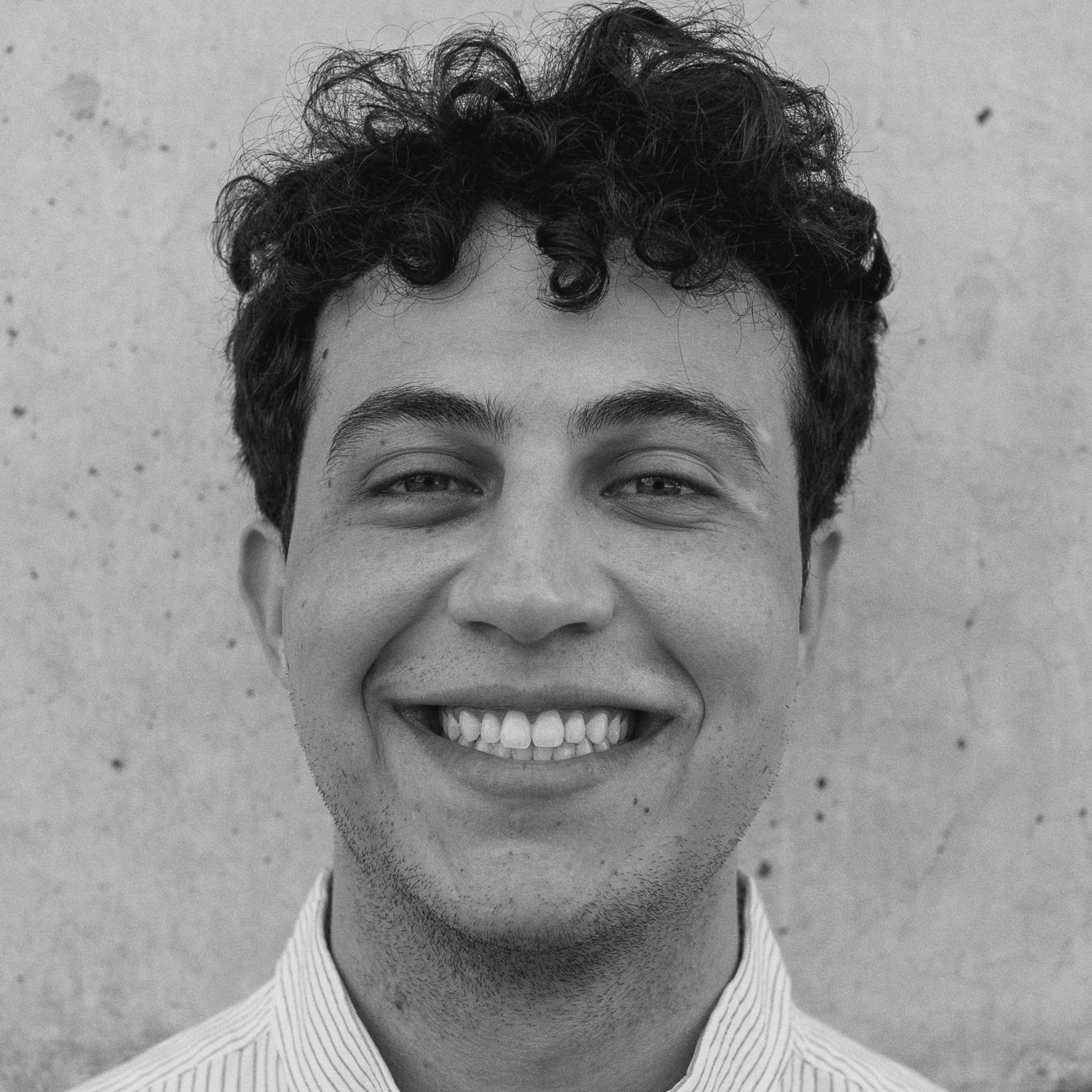