Interoperable Agentic AI: Unlocking The Full Potential of AI Specialization
Sam Adler / Dec 3, 2024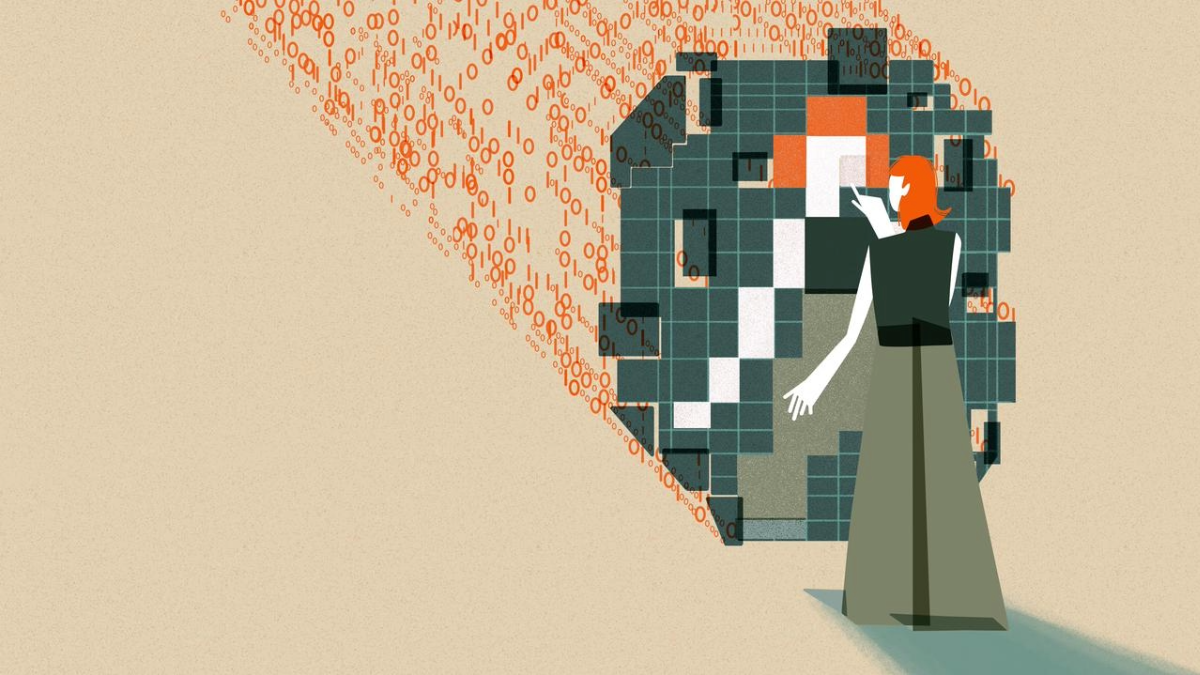
Yutong Liu & Kingston School of Art / Better Images of AI / Talking to AI 2.0 / CC-BY 4.0
Adam Smith’s division of labor theory helped lay the foundation for the Industrial Revolution. More than two hundred years later, the theory’s integration into AI systems may help galvanize the fourth industrial revolution. Applying Smith’s seminal concept to AI systems, developers have embraced compound AI systems to advance performance beyond that achieved through monolithic models. Now the promise of AI agents presents a new class of specialized, autonomous laborers that developers can integrate into collaborative multi-agent systems. Much like division of labor ushered in the benefits of specialization to the industrial revolution labor market—such as developing a skilled trade market and fostering trade-specific innovation—task-oriented AI agents can bring skilled AI labor and AI use-case-specific innovation to the fourth industrial revolution’s digital labor market.
To maximize the benefits of specialization, however, the market for agentic AI must remain competitive in spite of upstream consolidation. As the substrate on which developers build agents, foundation models can wield a form of platform power over downstream agent developers and users through unfair pricing or bundling, data-sharing demands, and self-preferencing. Walled garden ecosystems of AI agents built around existing foundation models will be inevitable without proactive regulation driving the adoption of increased ecosystem interoperability and data portability. Interoperability and data portability, however, come with inherent privacy and security trade-offs that take time to balance for optimal implementation. Therefore, interoperability and data portability should be considered from the start of this nascent market to avoid the headache of retroactive efforts or mandates that attempt to restructure an already entrenched ecosystem.
To realize an idealized future of agentic AI assistants that independently execute errands, policymakers must prioritize the difficult task of crafting nuanced AI interoperability and data portability regulations to undercut the trend towards centralization without inviting a new host of countervailing threats.
Rise of AI Agents
The agentic AI boom has arrived. Within the past six months, OpenAI released Swarm, Anthropic released computer use, Microsoft announced the launch of Copilot Studio and both Google and OpenAI unveiled AI-search. A growing list of industry players have extolled the future of agentic AI and previewed their investment in this agentic frontier. OpenAI even declared 2025 as the year agentic AI will go mainstream. Given the agentic AI hype and growing trend of deceptive AI claims, it is important to first address a fundamental yet complex question: what is agentic AI?
Much like the term AI itself, agentic AI does not have a clear-cut definition. Both exist on a spectrum of autonomy and capability. The Society of Automotive Engineers’ (SAE) autonomous driving taxonomy provides a helpful illustration of this continuum in an automotive context. SAE developed six levels of autonomy for motor vehicles, ranging from Level 0 (no automation) to Level 5 (full driving automation) based on a collection of autonomous driving capabilities. Level 0 through Level 2 emphasize that the human is still driving the vehicle despite driver-assist features. Beginning at Level 3, the human gradually cedes driving control to the smart vehicle until, at Level 5, the smart vehicle has complete driving autonomy under all conditions.
Similarly, AI systems range in “agenticness” based on their incremental ability to autonomously achieve more complex goals in increasingly complex environments. Whereas Level 0 through 2 represents AI systems that enhance human decision-making, Level 3 and onwards represents AI systems that grow in self-executing capacity in increasingly complex environments. AI may predict an outcome from a large data set, but increasingly agentic AI takes that prediction and autonomously implements an action plan. Today, AI assistants may automatically add events to your calendar or send email responses without supervision, but future generations of AI agents could act as loan underwriters, software developers, and even civil servants. Agentic AI systems are currently quite limited, but transformative advancements may come sooner rather than later.
Multi-Agent Systems (MASs)
Recently, AI developers have transitioned from monolithic models to compound AI systems. Monolithic models represent individual statistical models trained on increasingly enormous sets of training data. Compound systems take a more modular approach to intelligence, incorporating multiple calls to external tools, retrievers, or models to complete complex tasks. So why compound over monolithic?
Put yourself in the shoes of Danny Ocean, the lead character in the movie Ocean’s 11. You have a complex goal: pulling off a multi-million-dollar heist in Sin City. A monolithic approach to the heist would require Mr. Ocean to embody a super-thief that excels at explosives, surveillance, acrobatics, pickpocketing, auto-mechanics, and cons. The training and energy required to develop and maintain a super-thief is astronomical, and a jack of all trades is inevitably a master of none.
But, there is a reason the film is called Ocean’s 11. While the monolithic super-thief would need mastery across explosives, surveillance, mechanics and more, the team, or compound approach, leverages individual expertise for greater collective capability at lower individual cost. Mr. Ocean learns to delegate: He recruits accomplices and tasks them with particular, intermediary objectives that leverage their specialized skillsets in pursuit of the complex goal. Successfully done, this approach can accomplish a host of complex goals (be it heists or online shopping).
Danny Ocean wasn’t the first to see the value in teamwork. Adam Smith beat him to the punch by a few years. Just as Smith observed that dividing labor among specialized craftsmen led to greater efficiency than having generalist workers, so, too, are AI systems now evolving from generalist models to specialized agents working in concert. This same principle applies to AI systems, where specialized agents can collaborate more effectively than a single model trying to master everything. Compound systems offer improved performance without increasing compute demand, bucking monolithic model scaling laws. Rather than requiring exponential training data that fuels the “great scrape” to improve performance, compound systems offer heightened performance at a lower input cost by modularizing the system. And, when improvements need to be made, the specific component effects can be retrained instead of the entire model.
Advancements and increased investment in AI agents have naturally resulted in the integration of agents into compound AI systems, driving the growth of multi-agent systems (MASs). MASs consist of a network of AI agents capable of collaboratively achieving complex goals without a Danny Ocean figure managing the operation. Seamless interaction between specialized agents introduces greater autonomy into the compound system, allowing the system to exceed the competence of its manager. Although the market for AI MASs is still nascent, Microsoft and CrewAI’s platforms for facilitating AI MAS development demonstrate the growing investment in AI MAS workflows. As corporate demands for efficiency to drive profit grow, so too will the demand for enterprise AI MAS products.
Foundation Model Market Power & The Risk of a Walled AI Agent Garden
AI agents run on foundation models. This dependency on foundation models as the underlying scaffolding for AI agents creates potential bottlenecks in the development ecosystem.
A handful of firms dominate the AI foundation model market. Four firms operated over forty percent of the industry-released foundation models in 2023, and some reports estimate that five firms control roughly ninety percent of the foundation model market. While a highly concentrated foundation model market that may trend toward natural monopoly does not necessarily raise competition concerns, the ability to leverage this market power for anti-competitive ends warrants observation. Foundation model firms may exert this power downstream of the AI value chain, creating walled agent gardens. While apps from different companies currently work together relatively seamlessly, AI agents may only communicate with other agents built on top of the same foundation model. As the substrate on which developers create AI agents, foundation models function as platform technologies capable of cultivating their own agent ecosystems.
Platform markets are no stranger to antitrust scrutiny. Cloud services, app stores, online advertising, and search represent just a sampling of platform markets under competition regulators’ watchful eye. In many ways, foundation model firms have already begun to reinscribe platform gatekeeping frameworks and techniques into the nascent AI agent marketplace. OpenAI’s launch of the GPT Store recreated the app store but for AI agents. Google, Anthropic, and OpenAI are now competing over fully integrated enterprise platforms, including integrated MAS products, that facilitate vendor lock-in and product bundling. Moreover, Google and OpenAI recently unveiled AI search that paves the way for AI agents to access information from the open internet but also raises anticompetitive preferencing and AdTech concerns.
Foundation model firms present a real risk of leveraging gatekeeping power to proliferate closed ecosystems that carve up the AI agent marketplace and likely result in ecosystem lock-in at the expense of consumer choice and mix and match optionality. To be clear, the agentic AI market does not map neatly onto a platform model, but a comparable drive to entrench gatekeeping power should draw scrutiny similar to existing platform markets. In many instances, the very firms operating powerful incumbent platforms operate leading foundation models. While foundation models and agentic AI may usher in novel digital markets, they do not raise fundamentally novel competition concerns.
Before delving into the harms of segmenting the agentic AI market by underlying foundation models, it is important to first note the benefits to walled garden ecosystems. Walled garden AI agent ecosystems present three major benefits: technical integration and reliability, user experience, and security and privacy. Closed ecosystems improve technical integration and reliability by fostering seamless agent communication, standardizing security protocols, and facilitating more predictable agent interactions. They also enhance user experience through a consistent interface and design language, and streamlined support services. Finally, walled gardens strengthen security and privacy because they have reduced third-party attack surfaces, more easily audited data flows, and clearer chains of responsibility. Overall, walled garden AI agent ecosystems provide greater control over the environment in which agents interact.
While this control grants numerous benefits, it comes with significant downsides. From a consumer perspective, closed ecosystems force users to adopt entire ecosystems—even if certain parts are subpar—and limit the choice of specialized tools and customized workflows. From a market perspective, it reduces competition, stifles innovation, concentrates power, heightens switching costs, and facilitates anti-competitive pricing practices. Finally, from a societal perspective, walled garden AI agent ecosystems risk amplifying biases, reducing democratic control of AI development, and increasing the potential for social manipulation.
An open and competitive agentic AI ecosystem that champions consumer choice grants users greater agency in the AI marketplace. Where active use functions as a voting mechanism for software features, freer markets allow users to more effectively indicate their preferences and better direct agentic AI development.
Interoperable AI Agents
Adam Smith's theory of specialized labor presumed that specialized workers could freely participate in the broader market, selling their skills to whoever valued them most. Similarly, the benefits of compound agentic AI systems can only be fully realized in an open market where specialized agents can be freely combined across foundation model ecosystems.
Just as Smith recognized that labor specialization required key market infrastructure—from transportation networks to standardized currency—AI agent specialization depends on its own fundamental market conditions. A walled garden environment prevents AI MASs from realizing their full specialization potential and impedes consumer choice in the broader agentic AI market. To fully realize the benefits of AI MASs and the agentic AI market, the ecosystem must embrace competition. Technical interoperability—the ability of agents to communicate and work together across different foundation models—and data portability—the ability to transfer an agent's learned behaviors and user data between systems—serve as the digital equivalent of Smith's transportation networks and standard currency. These capabilities allow for a mix and match approach and helps avoid vendor lock-in by mitigating switching costs, creating the conditions necessary for a truly competitive market of specialized AI agents.
Interoperability and data portability implementation can range from purely voluntary to strictly mandatory measures. On the more voluntary side of the spectrum, market-led approaches may include self-regulation through standards consortiums, technical solutions such as middleware, or consumer advocacy-led pressure campaigns. Stepping up a rung, more tempered interventions may include transparency requirements, financial incentives, and public-private partnership initiatives. Finally, stricter interventions may include mandatory interoperability standards or even universal interoperability requirements, separation of platform and agent development or forced divestitures, and active regulatory enforcement. This broad spectrum of approaches can be done gradually or immediately, individually or in combination, nationally or internationally, sectorally or universally, for all companies or only some. The sheer number of permutations offer policymakers maximum flexibility in crafting a nuanced and responsive approach.
Implementing interoperability and data portability measures to address competition in digital markets is far from novel. Most recently, the EU’s Digital Markets Act mandated interoperability and data portability of messaging apps to address the gatekeeping power of Big Tech companies. While the DMA contains many positives, the act’s difficulties stemming from its rapid, reactive implementation stress the importance of proactivity. The open banking movement provides similar counsel: proactive rather than reactive interoperability and portability measures offer the greatest chance of disrupting concentrated market power.
As Jonathan Zittrain has noted, the time to address AI agents is now. While some have already begun spearheading this AI interoperable initiative, success relies upon more systemic buy-in in and support.
Interoperability & Data Portability Tradeoffs: Classic Concern with New Considerations?
Interoperability and data portability is not a risk-free proposition. The interoperability and data portability tradeoff between competition and security is well documented. The DMA faces this very issue. Existing interoperability and data portability tradeoff concerns will almost certainly translate to the agentic AI context. However, an open question remains: what unique considerations do interoperable AI agents, with their ability to learn, adapt, and make autonomous decisions, introduce to the tradeoff conversation?
Classic Interoperability & Data Portability Tradeoffs
Technological interoperability and data portability always raises tradeoffs. Classic interoperability and data portability tradeoffs generally center on security and privacy, innovation, user experience, technical implementation, and regulatory enforcement. As “tradeoff” implies, each include their own pros and cons:
- Security & Privacy: Grants users control over their data, reduces risk of single-point system failure or too big to fail companies, and empowers communal issue-spotting but also increases attack surfaces, complicates data security across multiple systems and in transit and creates a complex permissions management system.
- Innovation: Lowers barriers to market entry, decreases costs through greater competition, and increases competition but is also extremely expensive to implement, makes it more difficult to achieve scale, and can result in technological ossification.
- User Experience: Empowers users to select the best tools for discrete needs and eliminates vendor lock-in but also limits seamless integration and creates a more complex account ecosystem, with many credentials to maintain.
- Technical Implementation: Produces reusable standards that reduce development time and allows for shared, communal maintenance but also complicates customization and introduces version compatibility challenges.
- Regulatory Enforcement: Facilitates standardization that improves transparency, enables easier monitoring, and helps reduce the risk of regulatory capture arising from market concentration but also increases the number of companies to monitor, distributes blame, and increases the complexity of market interactions.
Agentic AI Interoperability & Data Portability Tradeoffs
Agentic AI interoperability and data portability tradeoffs incorporate all of those mentioned above but may introduce new considerations that policymakers must weigh.
- Communication Protocol: Establishes natural language as a flexible, universal interface for agentic AI interaction that may facilitate easier human review but also encumbers security validation and may lead to misunderstandings between agents.
- Adaptability: Allows agents to learn from diverse, inter-ecosystem interactions but also increases the risk of agents learning undesirable behavior and complicates the correction of problematic adaptations.
- Capability & Goal Alignment: Enables mixing-and-matching specialized agents with different goals to optimize performance but also risks less capable agents adversely influencing more capable ones and potential conflicts between agents’ objectives with no clear resolution mechanism.
- Information Sharing: Lets agents share information across ecosystems but also risks undesired transfers of information between systems.
- Version Control: Permits the upgrade of individual agents but also increases the complexity of backward compatibility.
To successfully untether agentic AI from concentrated foundation model firm power through interoperability, policymakers must navigate these tradeoffs. Committing the necessary, albeit significant, resources to create an interoperable ecosystem from the start will pay dividends later. Steep upfront costs are a small price to pay for freer, more competitive AI markets.
Authors
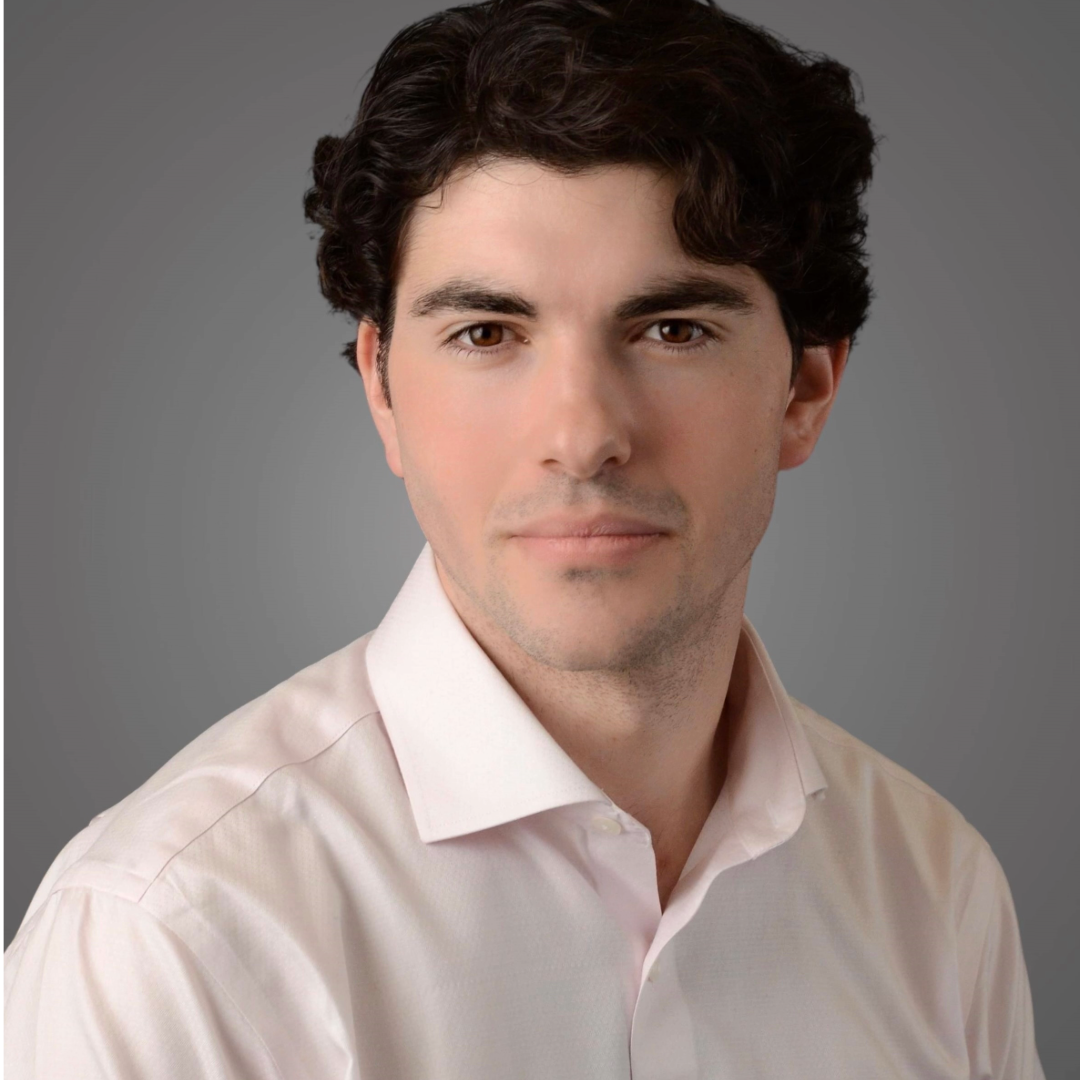