We Need an Interventionist Mindset
danah boyd / Mar 27, 2025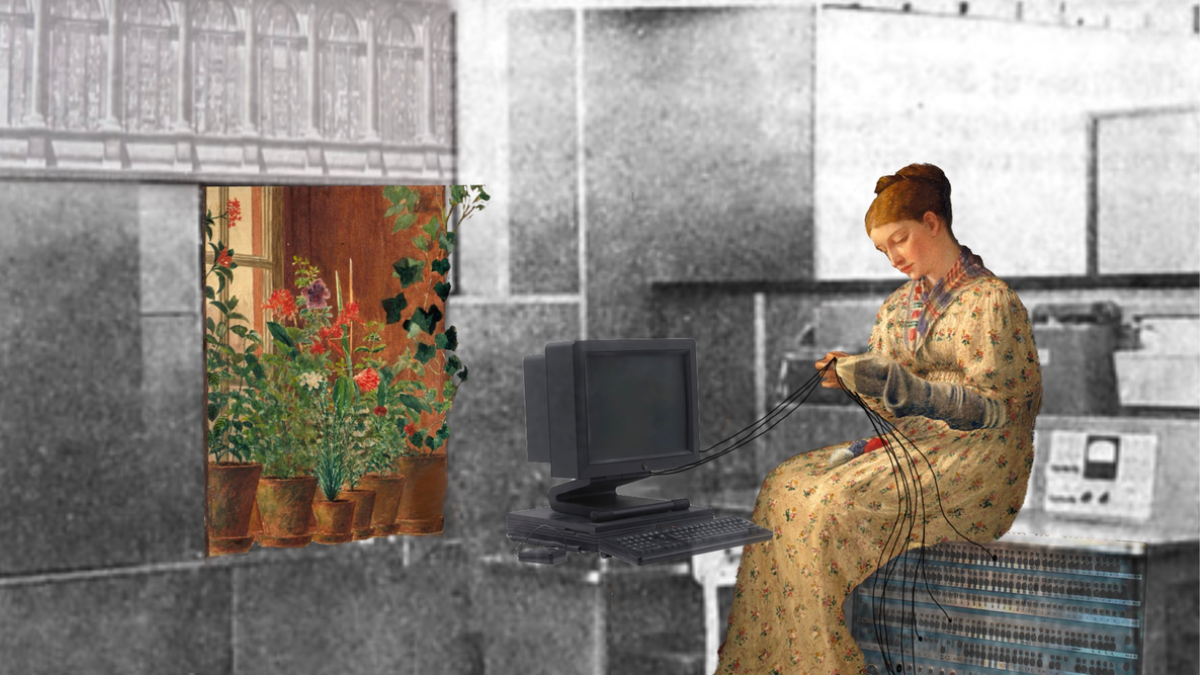
Hanna Barakat & Cambridge Diversity Fund / Better Image of AI / CC by 4.0
On March 5th, danah boyd, an incoming Professor of Communication at Cornell University, and B Cavello, Director of Emerging Technologies at Aspen Digital, addressed a gathering organized by Columbia World Projects and the Centre for Digital Governance at the Hertie School. This event was part of Digital Governance for Democratic Renewal, a joint initiative sponsored by the Knight Foundation. (Editor's note: the Knight Foundation has also provided grants to Tech Policy Press.)
During the session, danah discussed how to design and govern digital platforms to stabilize and enhance democratic practices, advocating for an interventionist approach to AI regulation. You can watch the presentation here. Below is her contribution in full.
We Need an Interventionist Mindset
Technologists and policymakers have a lot in common. Both seek to find solutions to problems. Both also seek to bend the future to their will. Among practitioners in both worlds, novel and innovative solutions are valorized. However, this also means that both technologists and policymakers tend to fall into traps of their own making. To make matters worse, policymakers tend to harden the solutionist logics of the technology industry in pursuit of regulating it, concretizing their power rather than serving as a check to it.
This talk invites everyone listening to shift their orientation away from solutionism in order to meaningfully challenge the existing arrangement of money and power that configures our contemporary sociotechnical environment. Rather than looking for “solutions,” explore “interventions.” An interventionist framework ensures a more iterative and non-deterministic approach to shaping AI futures.
Identifying Determinism
The concept of determinism can be summed up as the notion that “if X than Y.” Throughout history, as theologians struggled with the existence of God, many philosophers eschewed free will and took a deterministic stance. A deterministic orientation towards the human experience suggests that the future is pre-ordained. At a more micro level, every action produces a knowable outcome.
Engineers and technologists have also repeatedly pursued determinism within their own systems, with little recognition of the ecclesiastical roots of their orientation. Their mechanistic sensibility craves certainty. As such, they want to ensure that, if a person flicks a switch, the outcome is predictable.
Modern-day technologies, however, are often complex sociotechnical systems. This is especially true for systems that interact with unpredictable humans and messy social contexts. While many technologies may be designed to produce a knowable output to a user action, generative AI systems are intentionally designed to be non-deterministic. It may be possible to read the code or know what data a model is trained on. Yet, the power of generative AI stems from the ability to work with so much complexity that the output is probabilistic at every turn.
Even as AI specialists grapple with the conceptual and social consequences of building non-deterministic systems, their rhetoric about these systems’ role in society is trapped by the dominant paradigm of their industry. From the moment that ChatGPT was launched, technologists pronounced inevitable futures. Rather than being challenged, their deterministic prognostications were reinforced by journalists, companies, scholars, and policymakers, all of whom scrambled in response to the idea that “AI will change everything.” Instead of resisting this claim, supposed critics of tech reinforced the deterministic outcomes of the systems while fretting that they were already too far behind.
There is power in propagating determinism using “a discourse of inevitability” to constrain the range of possible futures. To get at this, we can look to the “Social Construction of Technology” (SCOT). SCOT scholars use historical case studies to describe the process by which new innovations emerge, get adopted, and become stabilized. The most canonical example concerns the bicycle. It was not initially inevitable that the standard bicycle would have two same-sized wheels and a seat in the middle where the rider faces forward; there was a lot of “interpretive flexibility” in the early development of this technology. The creation of a “standard” bike was not determined by some abstract idea of “best.” Rather, competing actors struggled to make their vision the dominant one. In the language of SCOT, the end result of this struggle is known as “closure.” Closure does not mean that no other type of bicycle may exist. Rather, it means that one approach dominates and sets the standard for others.
Resisting Solutionism
We are in a moment of interpretive flexibility, but technologists and their financial backers are highly incentivized to create closure around their system. This is how then that technological determinism plus inevitability rhetoric gets shifted into technological solutionism. Within this logic, we must have technology to solve a problem. And of course, we will define the problem so that technology can solve it. This has been the story of how the tech industry has sold new technologies for decades.
But, notably, María Angel and I found that policymakers are going one step further. Through “duty of care” provisions, they are now arguing that since technology has caused problems, it’s now necessary for technologists to design their tools better to fix the problems. This is a form of legally required solutionism, what María and I call “techno-legal solutionism.”
Solutionistic frames are rooted in the arrogance of determinism. Not only do they suggest that the future is known, but they suggest that a single policy or technology can create permanent closure around an issue. In the process, such an orientation fails to reckon with the ripple effects that such policies or technologies create. An interventionist frame is significantly different.
While there are plenty of hubristic doctors, the dominant contemporary paradigm in medicine is not oriented around solutions. Rather, since doctors operate probabilistically within a universe of uncertainty, they conduct interventions. Later, they follow up to evaluate the efficacy of said intervention. Although some might make predictions about the outcome of a particular intervention to calm the nerves of patients, doctors are excruciatingly aware of the possibility of side effects or other negative byproducts of their interventions. They take steps to minimize these negative outcomes, but they cannot ensure that their interventions will “solve” the problem. Medical interventions are evidence-based, but they are not deterministic. As a result, interventions must be evaluated. From there, a doctor iterates.
Shifting from a solutionistic approach to an interventionist one may seem like a game of semantics, but changing frames can support new actions. Resisting deterministic thinking is a muscle that we need to build. An interventionist approach means embracing probabilistic models since certainty is not guaranteed. An interventionist mindset also highlights the need for evaluation since, while there is a desired outcome, it is not clear that the intervention achieved that. This mindset also invites the interventionist to account for context. After all, one intervention might be more effective when the conditions are best suited for that intervention. One can also intervene at a different level by trying to shape the conditions for future interventions.
Human-in-the-Loop Solutionism
To make this concrete, let’s explore one commonly proposed “solution” to governance of AI: humans-in-the-loop. This sounds fantastic, but positioning humans in a way where they are to decide when and where to override the AI often results in them landing in a position that Madeleine Elish calls a “moral crumple zone” where they function just to absorb liability on impact.
In the 1970s, Congress approved auto-pilot for aviation, but required that pilots and co-pilots stay in the cockpit to take over in case of an emergency. Today’s pilots are glorified machine babysitters. When the machine breaks down, the probability of them successfully landing is low and pilot error is often blamed when systems break because the pilot was the last one touching the gear. But let’s look at an exception to this.
In 2009, Captain Sully successfully landed an Airbus A320 on the Hudson River in New York, saving all 155 people who were on board. After taking off from LaGuardia airport, the engines on Sully’s plane had ceased functioning because the plane flew into a flock of Canadian geese. Air traffic controllers instructed him to glide to Teterboro airport based on their models. Sully refused, arguing that he would not make it. He was instructed not to attempt a water landing because of how difficult such a landing is.
Because of his experience, Captain Sully resisted the recommendations of air traffic controllers and prioritized his own expertise over what he was being told, knowing full well that this was in violation of protocol. Unlike most pilots, Captain Sully had significant experience flying without autopilot; he had a second job retraining commercial pilots how to fly in emergencies. He also knew the New York region well. The air traffic controllers were also quite experienced and the strategies they used to support pilots were also well-honed, but neither had complete information. Captain Sully was more confident in his ability to land on the Hudson than to get to Teterboro.
After his nearly perfect landing on the Hudson, an investigation began and Sully was of course required to participate in it. Through this process, it became clear that the models used by air traffic control did not account for new construction in New Jersey; Flight 1549 would not have made it to Teterboro. Moreover, Sully argued that his inability to override computer-imposed limits that affected his glidepath created unnecessary injuries. This seemingly esoteric point flagged how contemporary pilots are presumed to be less intelligent than the machines that they fly.
As we are painfully watching in real-time, aviation is breaking down. Over decades, we’ve added AI into planes, air traffic control, and the construction of airplanes. And we’ve put disempowered humans-in-the-loop. And we have put more and more pressure on those humans. And then we’re surprised by the increase in accidents.
Humans-in-the-loop only works as a strategy if the incentives, skills, and structures are properly aligned. Currently, when humans are put into the loop on an AI system, they are primarily there to either absorb liability or uphold the mirage. Humans are the undervalued “ghost workers” as more and more supposed AI systems are rolled out with humans doing invisible labor behind the scenes (Gray and Suri, 2019). Humans are expected to override risk assessment scores, but are politically disempowered to do so (Brayne and Christin 2021).
Rather than thinking in terms of humans-in-the-loop, we need to be focused on how to properly construct an arrangement of peoples and technologies so that system degradation does not result in accidents. This requires accounting for maintenance and repair as well as looking for the vulnerabilities in the system. After all, left alone, infrastructures will break down.
Disruption is a Chess Move
A new technology is not inherently disruptive. It is disruptive if and when it is placed into an environment in a manner that benefits some people over others. The reason why the technology industry valorizes disruptive technologies is because venture capitalists and well-funded companies are well-poised to capitalize on these disruptions. In his treatise on communication power, sociologist Manuel Castells (2009) highlights how the most powerful actors are those who can arrange the networks of people, institutions, and flows of information and money to their advantage. Technologies can be introduced in a manner that disrupts existing networks, creating an opportunity for other actors to rearrange those networks in a manner that suits them. Those who are prepared for such disruptions are best equipped to respond strategically to them.
Leveraging disruption can prove quite lucrative. Not only is there money to be made on placing the right bets, but companies who are poised to leverage disruption can push for closure before competitors are able to take action. Because laws have the potential to hamper the gains from disruption, it behooves those invested in disruption to enroll policymakers into their project. This is a key lesson that the tech industry derived from Larry Lessig’s (1999) argument that code is power if and only if the market, social norms, and the law do not serve as a counterweight.
As we look to the emergent fights over AI, we need to keep our eyes wide open. This is not simply a technical debate. What we are watching is a strategic arrangement of actors and mechanisms to define a certain future as inevitable. When we play into this inevitability rhetoric and repeat industry’s deterministic orientation – even to argue that everything is bad – we do different futures a disservice. Instead, it behooves us to resist closure, eschew determinism, and eradicate “solution” from our discussions of technology. The future is not preordained. It is up to us to define it.
Authors
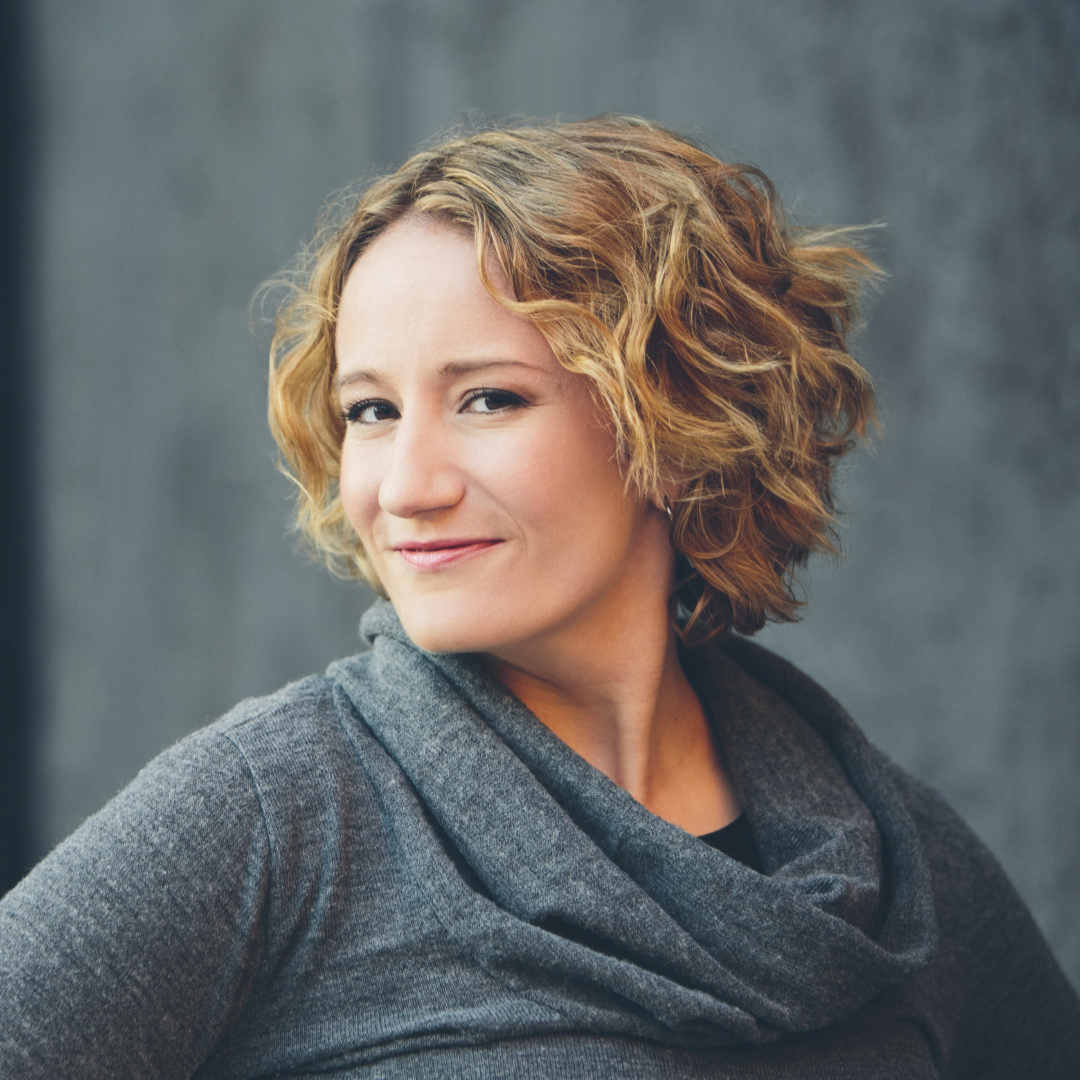