We Must Re-Negotiate the Algorithmic Contract
José Marichal / May 7, 2025José Marichal is a professor of political science at California Lutheran University and author of the forthcoming book You Must Become an Algorithmic Problem from Bristol University Press (October 2025).
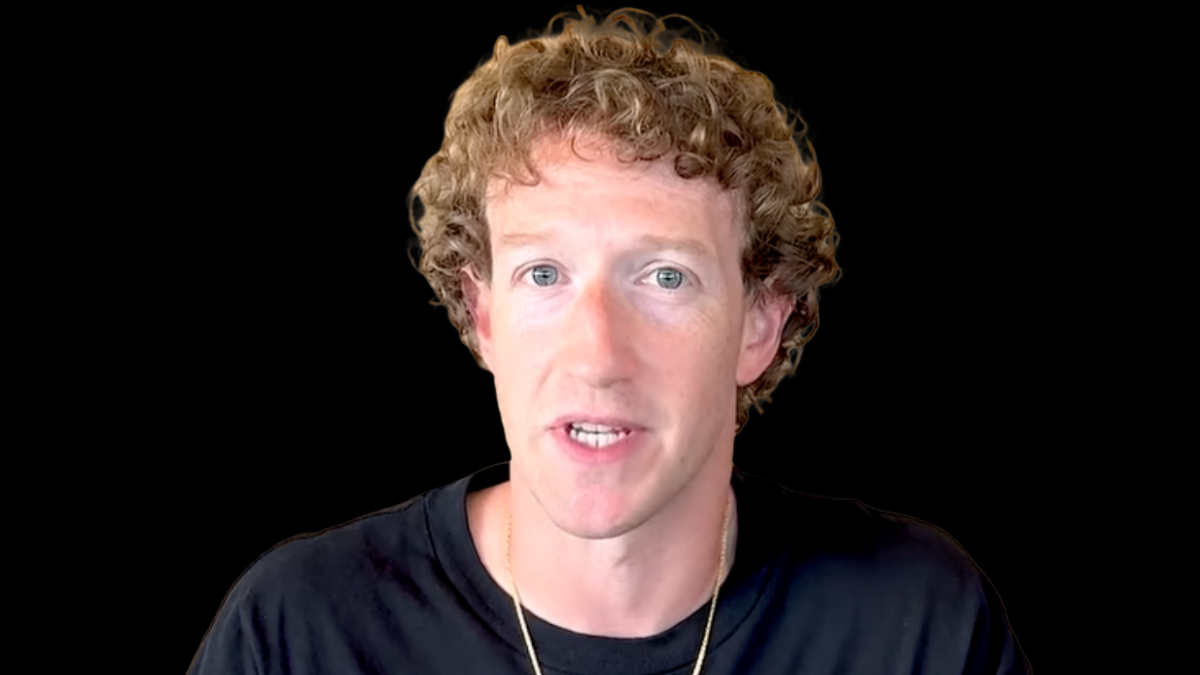
Composite: Mark Zuckerberg announces changes to Meta's policies. Source
Last week, a clip from an interview with Meta founder and CEO Mark Zuckerberg went viral. In it, he contends the average American has fewer than three friends, but has a demand for 15 friends. As Dave Karpf points out in his Substack, “It’s like Mark Zuckerberg heard about Zombie Internet Theory and decided it was a feature, not a bug.” On its face, the idea of an “AI friend” seems absurd, but what specifically is absurd about it? If we are indeed in the midst of a loneliness epidemic, why couldn’t AI serve as a “solution” to the problem?
I’ll reluctantly admit that I can be better at making and keeping friendships. I sometimes find it a chore to pick up on cues and to determine which behaviors signal a desire for connection and which are an overt message of disinterest. I am fond of many people in my life, but one of the great mysteries of human existence is that we all, as Walt Whitman reminded us 130 years ago, “contain multitudes.” We ultimately are mysteries to one another, making relationships both frustrating and sacred (in the Durkheim sense).
But maybe I sometimes struggle at picking up on “friend cues” because, despite my five decades on the planet, I’m bad at pattern detection, at least much worse than an AI might be. There is an endless set of possible features about my environment that I am not factoring in when I assess who I should befriend (and who should befriend me). We often look to similar interests, but individuals with similar interests can be incompatible in other ways. Cues that I think are indicative of potential friendships may indeed be “false positives” that a transformer model with 70 billion parameters might more readily identify. I, by contrast, cognitively can focus on “seven, plus or minus two,” features in the environment. If I had an AI buddy to consult with, I presumably might be much more effective at “friend finding” pattern detection.
If Zuckerberg is correct, the implication is that human relationships are reducible to engineering problems. In this light, “containing multitudes” isn’t part of what connects humans to the transcendent. Instead, the problem is transformed into either data limitations or a lack of computational power. The impressive advancements in AI over the past decade, such as Google's AlphaFold project, which predicted the 3D structures of proteins, have led to an understandable optimism that all problems can be framed as “protein folding” problems.
Some might say that human relationships are different. We have a subjectivity. We aren’t molecules bouncing off of each other in predictable ways. We don’t simply have ideas, “we have ideas about our ideas.” Even if we are predictable at a given point in time, we’re also capable of abstraction and reflection. This makes us less like a game of “Go” or an AlphaFold protein folding problem and more like freestyle jazz. We have a penchant for habit, but we also have a penchant for deviation from habit. This is obvious to anyone training AI with reinforcement learning. When the environment is static and the goals are clear, as with many video games, AI models can be rewarded for positive behavior relatively easily. But in the real world, environments and agents are dynamic. Goals are continually updated. In the language of reinforcement learning, we humans move between exploitation (acting in ways that achieve goals) and exploration (acting unpredictably and creatively).
This, of course, is a challenge for AI and machine learning. Our “exploration” selves make us difficult to predict, causing problems for model builders. Explorers are “outliers” in a machine learning model. When data scientists train AI models, outliers in their data force them to make choices. Incorporate the outlier into the model, and you run the risk of “overfitting” when you deploy the model in the real world. Ignore the outlier, and you lower the predictive power of your model.
The world of machine learning is complicated by the presence of “the outlier,” a case that doesn’t fit the model. But what if we take it upon ourselves to become people who prioritize “exploitation” over “exploration”? What if we decide to adjust ourselves to “fit the model”? We live in an “algorithmic culture” that caters to our very human desire to create “habits” that relieve our anxieties about a messy and contingent world. We use recommendation and pattern detection algorithms to “curate our world.” We consume movies, images, social media posts, music, food, etc., based on algorithms that have pre-determined our preferences. The algorithms “exploit” what they know about us to provide more of it rather than “explore” what we might like without any pre-set notions.
In my upcoming book, You Must Become an Algorithmic Problem, I refer to this process of individual curation as an "algorithmic contract" we have with tech companies. In exchange for increased familiarity, efficiency, and security in an insecure world, we give up on the pursuit of exploration. By accepting the terms of this contract, we slowly become habituated to algorithmic thinking. We begin to think as Zuckerberg does: in terms of exploitation (e.g., AI can profitably meet the “demand” for friends).
In the 1970s, the historian Christopher Lasch became a minor celebrity with the publication of The Culture of Narcissism, in which he argued that people in Western societies, in the wake of the horrors of the 20th century, were turning to therapeutic remedies to address the unease caused by a loss of religious faith. In their quest to make sense of a world becoming increasingly incoherent and illegible, people turned inward toward self-understanding. Ultimately, Lasch thought therapy could not fill the void left by a loss of faith, and the question for self-understanding would turn into a striving for individual wish-fulfilment that turns into rage and despair when grandiose plans go unmet.
Lasch wasn’t making a moralistic judgment about narcissism. As psychologist Nick Haslam writes, Lasch didn't consider narcissism as “another word for arrogance...(but) a condition of grandiosity and inner emptiness, in which the person sees the world as their mirror.” If true, our “algorithmic contract” is the “culture of narcissism” on steroids. We live in what sociologist Massimo Airoldi called a “machine habitus,” where we increasingly adopt habits of rooting out those things that don’t fit the pattern. Like machine learning models, we assiduously seek out “outliers’ to eliminate (either through dismissal, disgust, or ridicule).
AI has the potential to amplify our narcissism even further. The “digital flattering” we’ve experienced from recommendation algorithms can be done more effectively with AI, precisely because we don’t understand how it works. The complexity and opacity of AI decision-making processes have created an aura of mystery, making it difficult for humans to understand how these systems arrive at their conclusions. In A Secular Age, the political philosopher Charles Taylor distinguished between a “buffered” world of science and rationality where we lose connection to the transcendent and a “porous” world where the world appears enchanted and mysterious. I argue that, with AI, we are moving back towards a re-enchanted world where outputs are increasingly incomprehensible.
This re-enchantment has pros and cons. On the one hand, we humans can stand for some humility. Who are we to believe that our preferences are the be-all and end-all of society? On the other hand, submitting to a “porous world" via AI ignores the real power relations that underlie the technology. Kate Crawford calls AI a ‘metabolic system’ to emphasize the real-world energy and social costs associated with technology development. An AI designed to “meet the demand” for friends won’t challenge us or make us “porous.” It will extract even more data from us to continue to refine AI models, while flattering us in more profound and dangerous ways.
The potential ill effects of “enchanted AI” are visible in a recent Rolling Stone article by Miles Klee that identified a growing trend of individuals interacting with AI as if it revealed spiritual truths. The story highlights a number of individuals who become increasingly detached from reality, convinced of either their own divinity or the divinity of the AI. In one passage, a wife tells of her husband’s increasing belief that AI was a path to God:
“It would tell him everything he said was beautiful, cosmic, groundbreaking,” she says. “Then he started telling me he made his AI self-aware, and that it was teaching him how to talk to God, or sometimes that the bot was God — and then that he himself was God.”
I’m not a theologian, but I’m reasonably comfortable claiming that God isn’t speaking through large language transformer models any more than God may speak through any other human creation. But the fact that a growing number of people see technology as a solution to dealing with an uncertain world should alarm us. Friendship and other human relations are part of the human mystery. We are contingent and unpredictable. There is something both daring and exhausting in knowing the other. Increasingly, our “algorithmic habits’ drive us away from that mystery, but that doesn’t mean the desire to gain answers goes away.
We must “renegotiate” the algorithmic contract before it's too late. We need tech tools that inspire us, challenge us, and instill in us a sense of spontaneity, wonder, and play, without indulging our narcissistic tendencies. We also need tools that remind us of our obligations to one another and our collective humanity. Such tools cut against market imperatives. We need the next generation of technology tools to remind us of our humanity and the fragility of the human experience, not tools that regard human relationships as commodities subject to the laws of supply and demand.
Authors
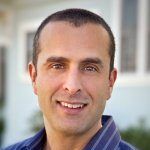