The Race to Detect AI-Generated Content and Tackle Harms
Akash Pugalia, Farah Lalani, Sameer Hinduja, Fabro Steibel, Anne Collier, David Ryan Polgar, Nighat Dad, Ranjana Kumari / Mar 11, 2024One of the well-known risks of generative AI is the proliferation of deepfakes. There are alarming implications of synthetic media for everything from the integrity of elections to celebrity nudes. Examples of harms documented to date include scammers using fake audio to steal money by posing as family members in crisis, videos created of politicians seeming to say things that are completely fabricated, and photos of people being sexualized into nude images and video that are then used for the purposes of sextortion or other forms of harassment. Beyond individual pieces of fabricated content, generative AI is being used to create deepfake websites, thereby making it easier to create and distribute additional false content at scale.
These harms are just some examples of a complex problem that will require a whole of society approach, including government regulation, industry self-regulation, public and private technological advances, and education. It is impossible to entirely mitigate the threats of synthetic media, but certain strategies may reduce the problem substantially.
Incorporating markers into AI-generated content
As a first step to addressing these issues, many experts highlight the importance of being able to distinguish between AI-generated, AI-altered, and human generated content. This is critical for identifying content ownership for the purposes of understanding IP rights, creating accountability and responsibility for content safety with the original content owner, and for the purposes of prioritizing investigations of real-world harm (e.g. rescue of real children in videos or images showing abuse vs. wasting resources investigating fabricated victims in AI-generated content). However, the current set of AI-content detection tools show both limited and inconsistent efficacy.
Recent research conducted and published in the International Journal for Educational Integrity evaluated the efficacy of AI content detection tools in differentiating between human and AI-generated text. The study abstract highlights how human-generated and AI-generated (from ChatGPT Models 3.5 and 4) paragraphs were evaluated by AI content detection tools developed by OpenAI, Writer, Copyleaks, GPTZero, and CrossPlag. The findings reveal that the AI tools exhibited inconsistencies, producing false positives and uncertain classifications when applied to human-generated text, underscoring the need for further investment in improving consistency and accuracy of these tools. Embedding such solutions, as they are refined, into devices at an operating system level to notify users of AI-generated, manipulated, or otherwise suspicious content could be helpful. This is especially important given that most content is consumed on smartphones, where identifying fabricated images may be more difficult (vs. a laptop or desktop) due to the smaller screen size.
There are a few different solutions to be able to distinguish between human vs. AI-generated content.
- The first is watermarking. One technique is “invisible watermarking, designed to be machine-detectable, rather than an embedded visual marker as commonly found on stock photos, which either obscures the image or can be removed very easily.” However, some experts argue that this is not foolproof as bad actors can find ways to remove or alter watermarks or other digital signatures through color adjustments, file format changes, or other manipulations.
- Another solution is adding metadata to content; “cameras have been doing this for decades. Blocks of text are often marked up as well. When you type something in bold, or set a font’s color on a website, the word processor or browser labels your content with metadata. But it’s application-specific: Paste some bold text into your address bar, and the formatting is gone.” Said another way, any images or videos created by a human would have metadata added to it by the device used to capture it, and that metadata would be proven authentic via cryptographic signature.
- A third solution intended for text outputs is Unicode, a universal numbering system for text. Using such a standard would essentially give AI its own character set. Again, this solution poses practical challenges such as the finite amount of room in Unicode and many languages it would be necessary to support.
Automated labeling may also help users determine what is machine-generated. For instance, X labels certain content as “misleading information,” a “disputed claim” or an “unverified claim,” and says it may label media that has been altered of synthetically generated. On February 6, Meta said it was going to label AI-generated images on Facebook, Instagram, and Threads. When someone uses Meta’s AI tools to create images, the company will add visible markers to the image, as well as invisible watermarks and metadata in the image file. The company says its standards are in line with best practices laid out by the Partnership on AI, an research nonprofit funded by industry.
Content provenance is an important part of the solution that many experts believe needs to be paired with the solutions described above. Provenance points to the origin and history of a piece of digital content, such as location and date of creation and any changes that have been made throughout its distribution. The Coalition for Content Provenance and Authentication (C2PA) has released an open-source specification to standardize this process through technological solutions, and is backed by some of the biggest tech and media institutions in the world. On February 8, Google announced that it would join existing tech giants, such as Microsoft, in the steering committee of C2PA and that it will include its watermark, SynthID, in all AI-generated images from its new Gemini tools. Meta is also participating in C2PA. OpenAI also announced new content provenance measures recently, saying watermarks would be placed in images generated with ChatGPT and DALL-E 3.
Initiatives like these are gaining traction given the velocity at which AI features are being added to popular online products and services. Provenance solutions go deeper than detection and are critical given that “much of the problematic content online now is actually a real video that has been misrepresented as being from a different time and place,” according to the Content Authenticity Initiative (CAI), another industry group that includes Adobe, Microsoft, and a variety of media companies and NGOs. ”Provenance can provide a scalable way to debunk “cheapfakes” [AV manipulation created with inexpensive, easily accessible software, or none at all] which are at present a far more widespread problem than deepfakes.”
Information about content provenance could be stored on a secure digital ledger system (e.g., blockchain) and – along with a number of other variables related to origin – could be embedded within images and videos created by AI. Content is typically created to be shared with others, and if major content hosts– cloud services, social media companies, web hosting services, and news organizations– prohibit the uploading and posting of noncompliant text, photos, and videos that are missing key provenance information, we may see less malicious of use these technologies to deceive, trick, and harm others.
Bad actors are likely to improve in their ability to obfuscate AI content detectors and manipulate provenance solutions; therefore, solutions need to be multi-pronged to increase the difficulty of removing, bypassing, or altering these techniques across both detection and provenance. For example, running AI generated content through a separate model may remove any watermarking efforts. Making detection tools publicly available also allows those intent on harm to check and re-check their creations until they pass muster, before deploying them widely.
Developing new laws and regulations
Regulatory requirements are quickly evolving to counter the threat of deepfake content:
- In the EU, the Code of Practice on Disinformation addresses deepfakes. The code was initially introduced as a voluntary self-regulatory instrument in 2018 but now has the backing of the Digital Services Act (DSA). The DSA, which came into force for all websites in February, increases the monitoring of digital platforms for various kinds of misuse, and threatens fines of up to 6 percent of global revenue for violators.
- Under the EU AI Act, deepfake providers would be subject to transparency and disclosure requirements. It is expected that this groundbreaking law will be used as a guiding framework to inform what other countries do over time.
- In markets such as the US and Canada, there aren’t specific passed laws targeting deepfakes (although the Deepfakes Accountability Act and the Preventing Deepfakes of Intimate Images Act have been proposed), however there are laws targeting the spread of non-consensual intimate images, including those that are AI-generated.
- Recently proposed in California is a bill that would require companies in this space to watermark any content created from their AI models. In time, we may see bills across different nations that allow individuals who have been harmed by generative AI technologies to obtain financial compensation from the companies that built them (one in progress is in the EU, titled the AI Liability Directive).
Implementing such regulation successfully in democracies will be a challenge, given concerns over free expression. To develop effective policy will require improvements in technology, regulatory enforcement mechanisms, and industry collaboration efforts such as those spearheaded by the CAI to develop open, technical standards to trace the origin of different types of media. In the meanwhile, educating users to think critically about the source, purpose, and authenticity of content is likely to be the best defense.
Industry’s critical role in educating end users
Towards this end, some platforms have built “Safety Hubs” or “Help Centers” designed to help users set up app-specific controls through clear descriptions, screenshots, and walkthroughs. For instance, Roblox designed a Civility curriculum, Google built a virtual world called “Interland,” and TikTok launched campaigns to share strategies and safety features via in-person workshops in the community. While proactive education regarding generative AI harms exists through dedicated websites, training programs, and even offline events, their efficacy faces empirical limitations. These resources require users to actively seek them out. In practical settings, particularly outside mandated environments like schools or workplaces, uptake of such material remains demonstrably low until an individual directly encounters a harmful situation – precisely the scenario such resources aim to prevent..
To support, equip, and empower users, platforms hosting generative AI tools must prioritize seamlessly integrating educational content within the user experience. This can be achieved through subtle interventions such as contextual notifications, targeted prompts, interstitial messages, or even engaging pre-roll content during loading screens. Gamification techniques, like badge rewards, also may help to further incentivize engagement. The effectiveness of this approach hinges on compelling content delivery. Leveraging relatable scenarios, incorporating influencer participation, and maintaining a degree of intrigue all contribute to increased user attention and knowledge retention. Cultivating skills like media literacy, digital citizenship, and online civility are crucial in the emerging landscape of generative AI. Platforms have a unique opportunity to strategically and captivatingly equip their userbase with these essential competencies. By seamlessly integrating impactful educational content, platforms can not only encourage responsible and prosocial use of AI tools, but also demonstrably reduce overall user vulnerability to generative AI harms.
Conclusion
The proliferation of deepfakes and synthetic media poses significant challenges to privacy and public trust. The efforts outlined above are only a start towards addressing what is a rapidly metastasizing problem. The harms are real– from young girls targeted with pornographic deepfakes in grade school to growing suspicion over the veracity of events in conflict zones. Everyone involved in the development, commercialization, regulation, and application of generative AI tools must join the effort to contain the negative impacts of these technologies.
Authors
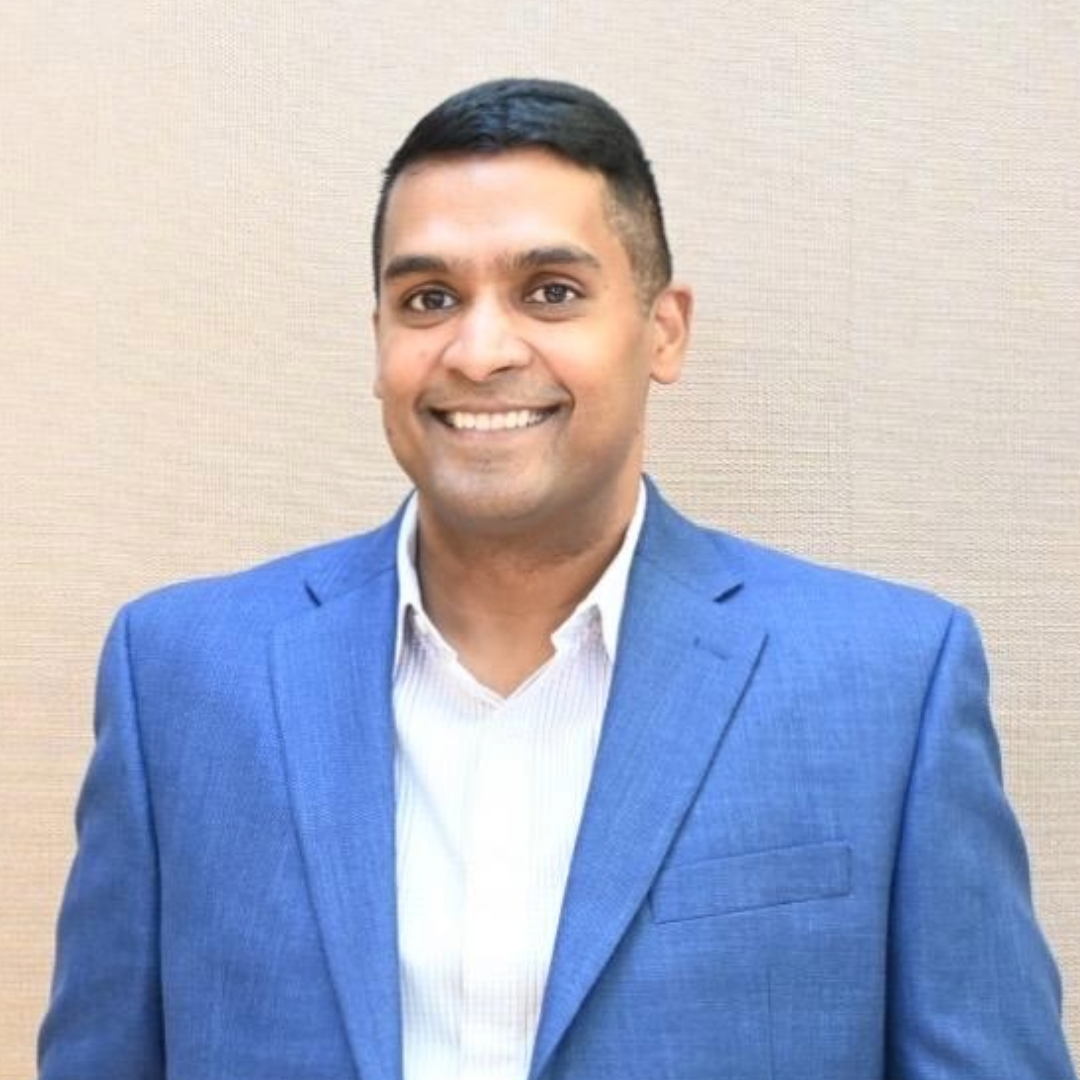
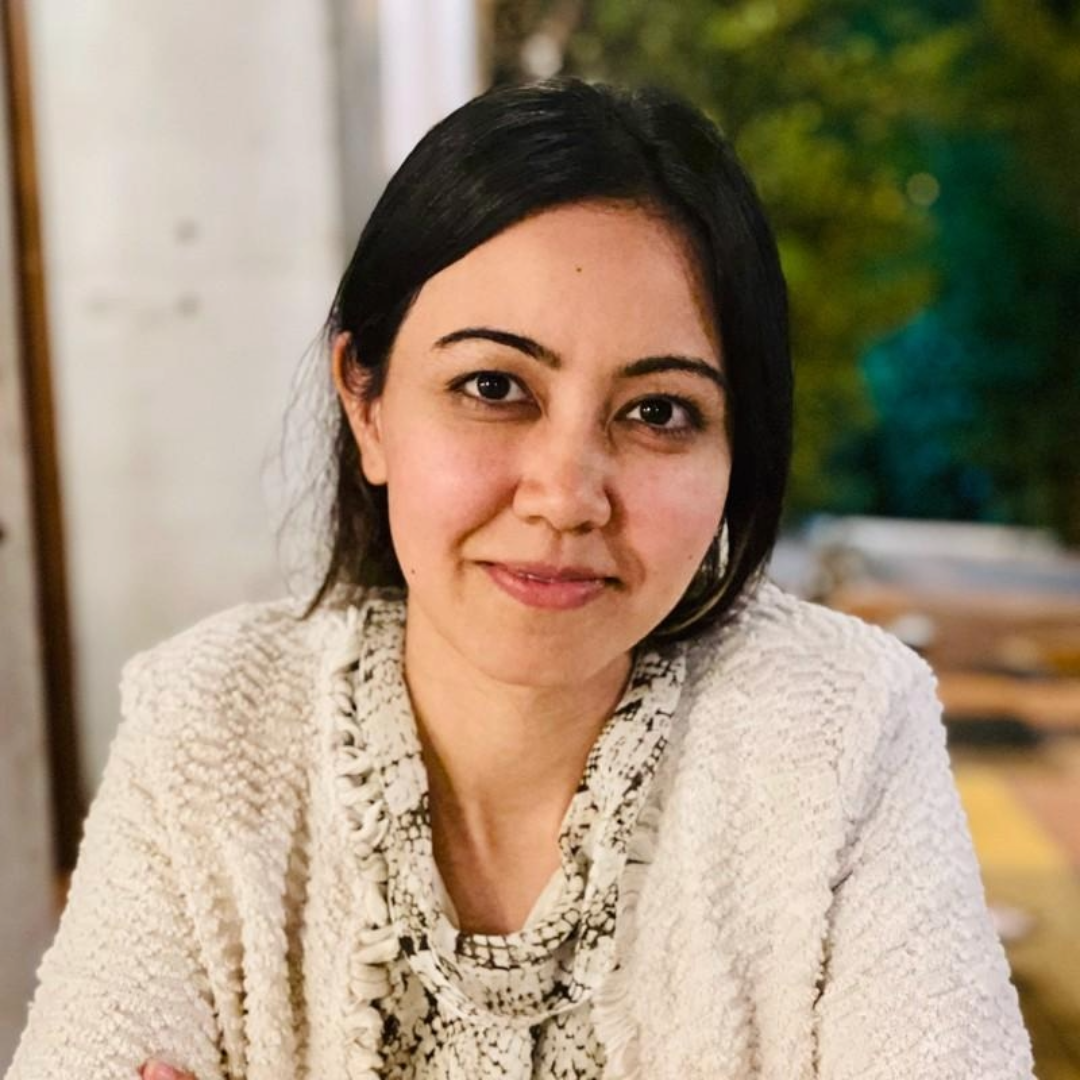
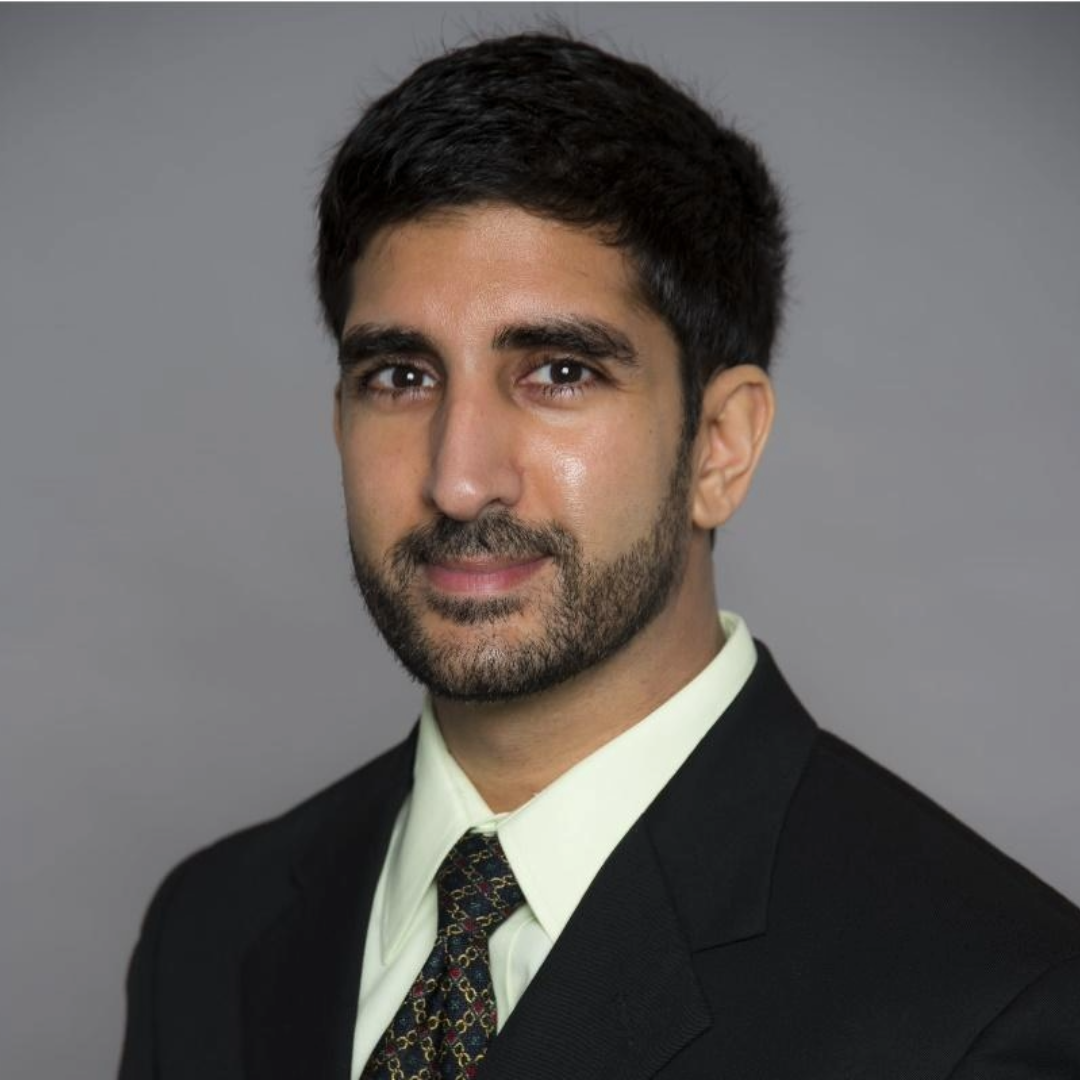
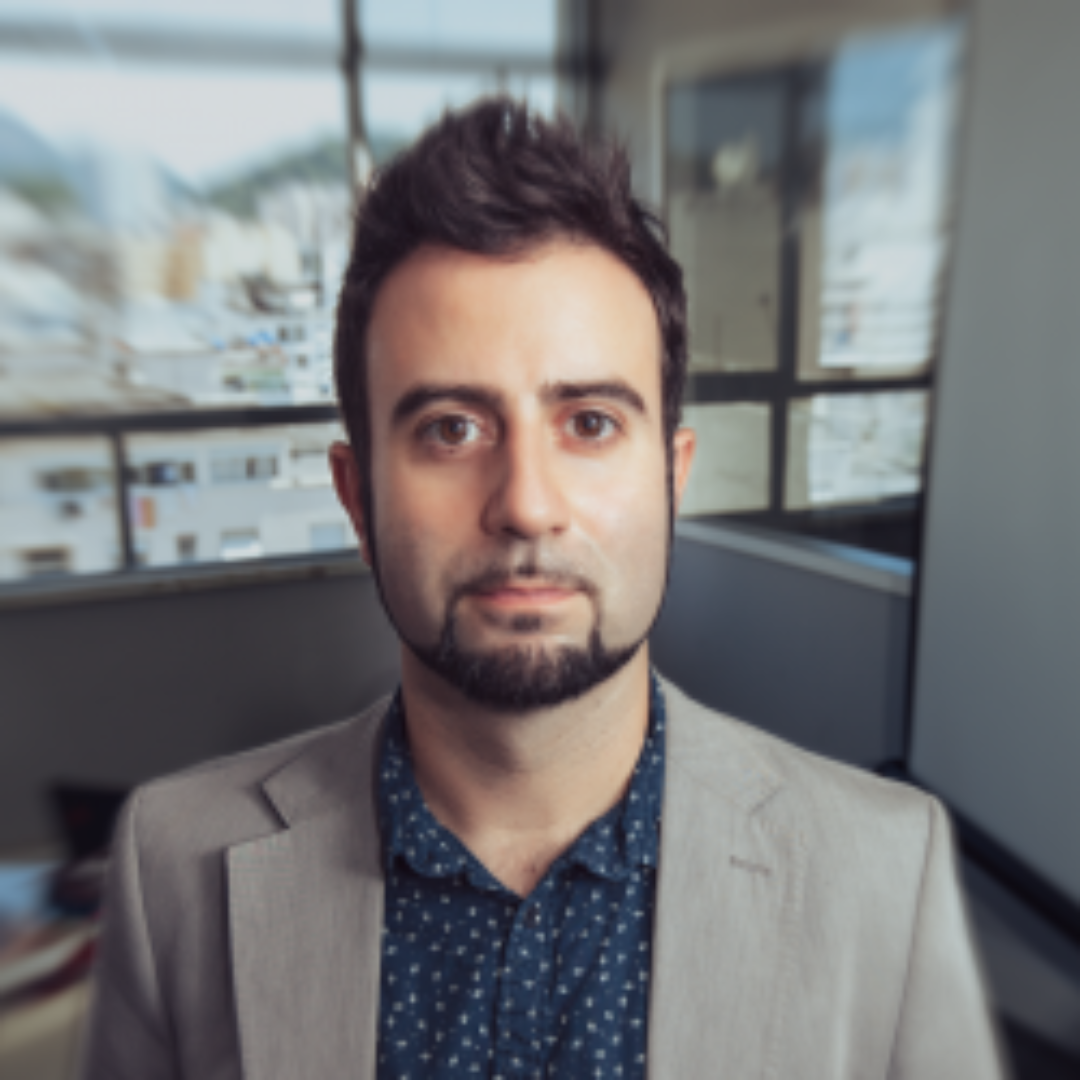
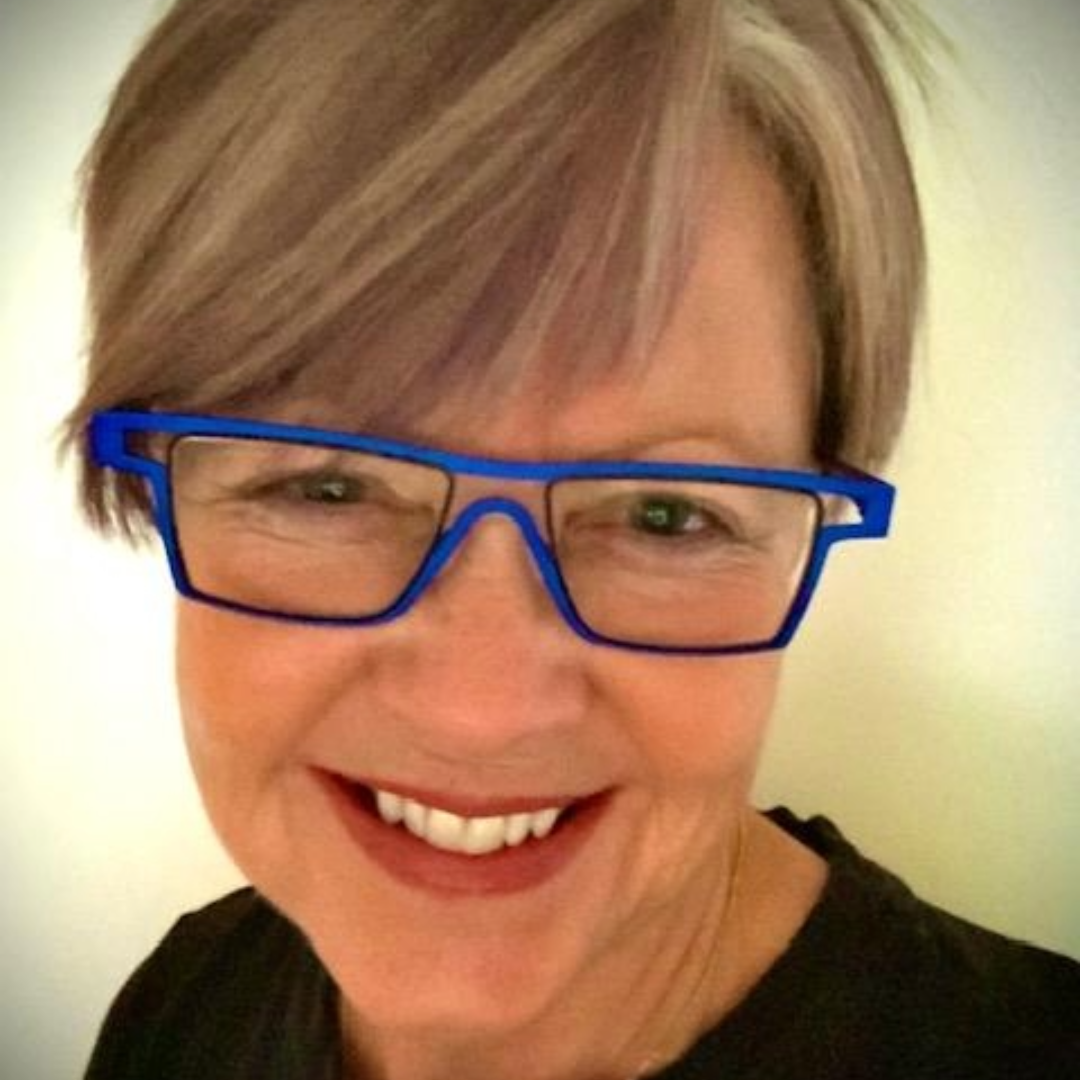
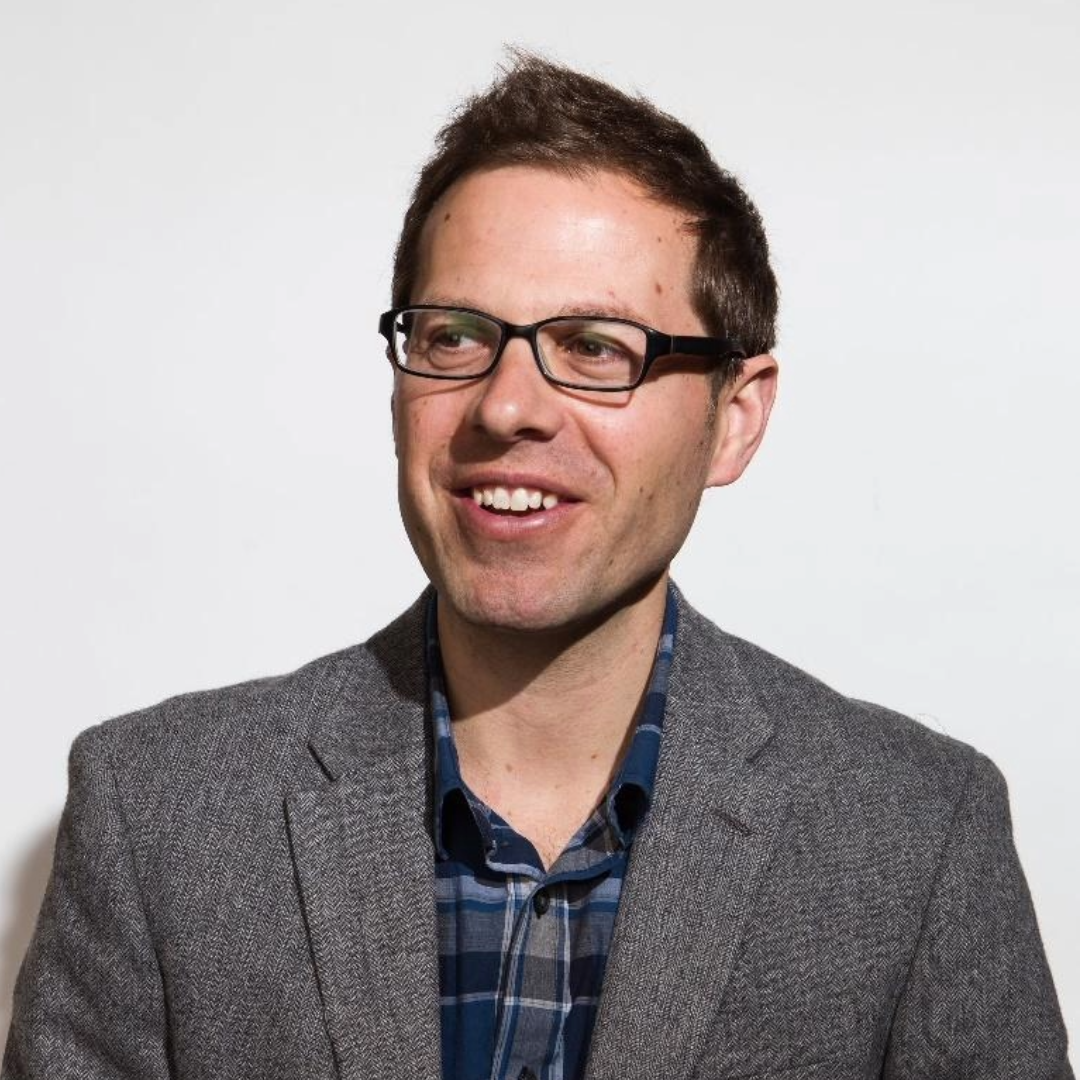
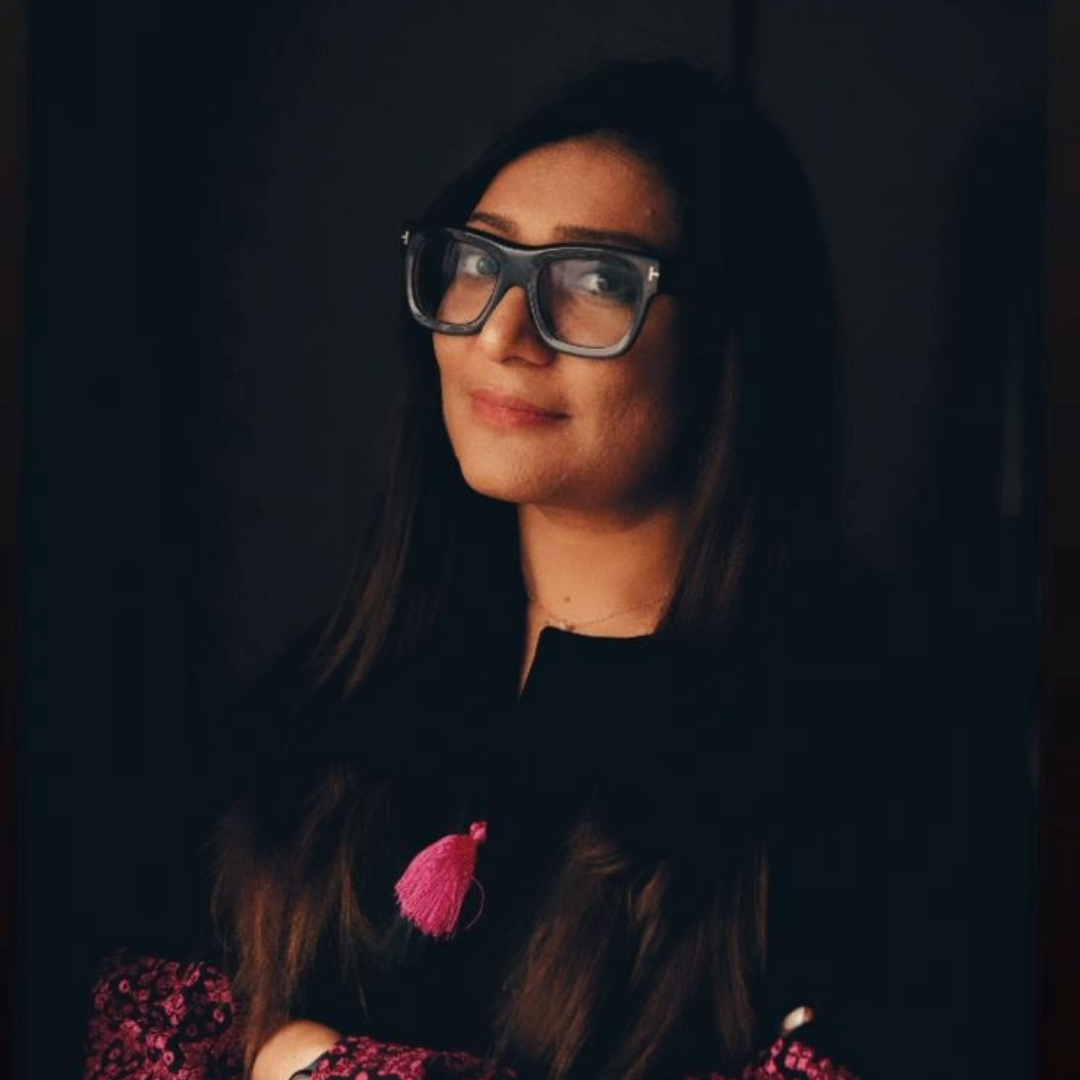
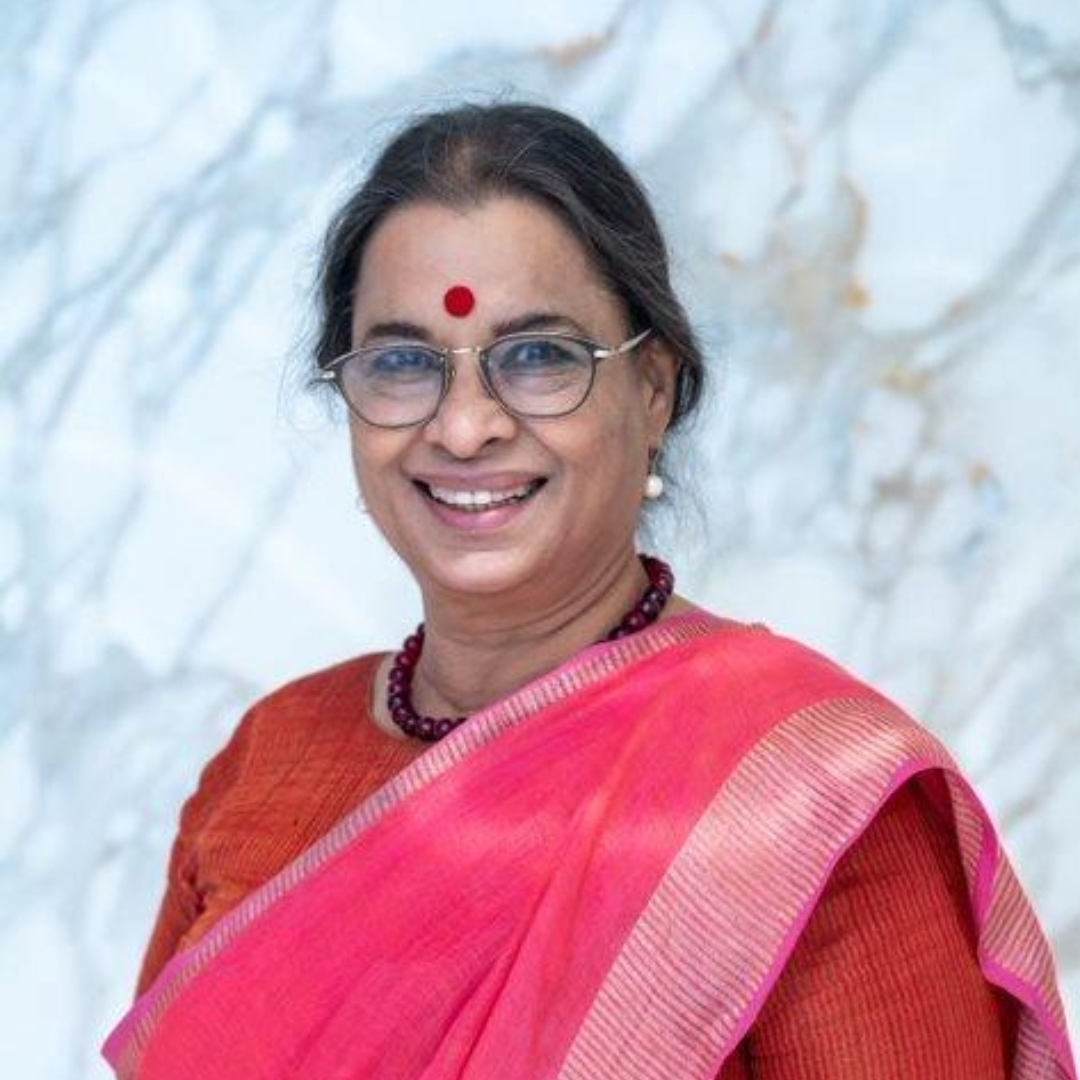