The Critical Role of Research in the Fight for Algorithmic Accountability
Marissa Gerchick, Olga Akselrod / Oct 23, 2024Marissa Gerchick is a Data Scientist and Algorithmic Justice Specialist with the ACLU’s Technology Team, and Olga Akselrod is a Senior Staff Attorney in its Racial Justice Program.
Employers across industries are now widely using automated tools in their hiring processes, including for screening applications, assessing candidates, and conducting interviews. These tools can exacerbate existing bias in hiring and employment in a number of ways, including through adding automation or algorithmic elements to tests that already fundamentally discriminate based on race, gender, disability, and other protected characteristics.
Take, for example, the use of AI-driven tests and assessments in hiring. Cognitive tests used in hiring can unfairly disadvantage applicants with cognitive disabilities and have long been known to encode racial disparities. Likewise, personality tests used in hiring often ask general questions that have little to do with a person’s ability to do the job, instead capturing traits closely related with diagnostic criteria for autism and mental health conditions such as depression and anxiety. In today’s world of automated hiring, where these tests are now often gamified or algorithmically driven, the problems with these assessments are only exacerbated through the incorporation of automation and algorithmic elements that can themselves discriminate on the basis of race, disability or other protected characteristics and create new pathways to discrimination.
To fight against these digital barriers to employment, in May, the ACLU filed a complaint with the Federal Trade Commission (FTC) against Aon, a major hiring technology vendor, for deceptively marketing its online hiring tests as “bias free,” even though the tests carry a high risk of discriminating against job seekers based on race and disability. The complaint details issues with several Aon products, including an algorithmically driven personality assessment, a gamified cognitive ability assessment, and an AI-driven video-interviewing tool. We also filed charges with the Equal Employment Opportunity Commission (EEOC) against both Aon and an employer on behalf of a biracial (Black/white) autistic job applicant who was required to take Aon assessments as part of the employer’s hiring process. Together, these actions are a first step in seeking accountability when discriminatory technologies are used in hiring. These actions should serve as a warning to all hiring tech vendors and employers that they must comply with anti-discrimination laws, but additional actions against other vendors and employers will be necessary to truly spur reform.
Academic researchers also play a critical role in the fight for accountability when algorithmic tools are used in high-stakes areas like hiring. As an example, independent research was critical to identifying problems with Aon’s video interviewing tool, vidAssess-AI. This tool allows employers to administer interviews asynchronously — candidates record video responses to selected interview questions — and the interviews can be analyzed and scored using AI. vidAssess-AI relies on Aon’s personality assessment (which itself poses a high risk of discrimination, as discussed in our FTC Complaint) and introduces additional discrimination concerns through its incorporation of AI and machine learning (ML) elements. These AI and ML features are used to generate transcripts of applicants’ videos and to analyze those transcripts and score applicants accordingly. But independent research has established that these types of systems perform worse for Black speakers compared to white speakers, for speakers whose first language is not English, and for speakers with speech disabilities and other disabilities.
This research examining the models underlying many automated hiring tools is critical, but we also need much more research focused on the specific automated hiring products on the market — i.e. the resume screener applications, the automated video interviewing platforms — and the ways that these products are deployed by employers. Some important independent research has been conducted on the large well known tech platforms used for employment sourcing such as LinkedIn or Facebook, but very little research has been conducted on the hundreds of products flooding the market dubbed as “Little Tech” that are used by employers to evaluate applicants. Applicants have virtually no transparency into an employer’s hiring process, and generally do not know when an automated tool is being used, let alone that it may be discriminating against them. That lack of transparency makes it harder to hold employers or tech vendors accountable.
Research that examines how specific automated hiring tools are being used by an employer, or that examines how specific tools are used across a host of employers, can provide critical information for regulatory agencies and the private bar about where discrimination is happening in practice — but is currently hidden — and more effectively empower accountability through legal avenues. Research that identifies discrimination through particular products can also influence employer purchasing decisions and the market as a whole. If employers stop purchasing a harmful tool because of information uncovered through academic research, the vendors of the tool will stop selling it or mitigate the harm.
To be sure, conducting such research is not without challenges. The lack of transparency that creates a barrier for regulators and litigators likewise creates barriers for researchers. Vendors and employers building and deploying these systems are usually not forthcoming about the data used and choices made in designing, developing, and deploying such systems. As a result, research on the impacts of automated hiring tools in practice is often shaped by the vendors and employers selling and using those tools. Nonetheless, this challenge must be confronted by researchers, while regulators and advocates also push for greater transparency from vendors and employers. In addition, emerging work leveraging novel methods to externally audit automated systems used in hiring offers promising directions that academic researchers could further develop and expand. For example, existing methods that have long been used to conduct civil rights testing in employment and housing, such as correspondence studies and matched pair testing, could be adapted to specifically audit the use of automated hiring tools.
To help explain key details about Aon’s products in a streamlined format for researchers who may be interested in studying these tools, we created “model cards” for three of Aon’s tools that we discuss at length in the FTC Complaint – gridChallenge, ADEPT-15, and vidAssess-AI. Proposed by a group of prominent AI researchers, model cards are short documents that highlight key details about an automated system and can serve as a valuable framework to encourage transparent reporting about how automated systems should and shouldn’t be used. Our model cards adapt the framework of Mitchell et al. to focus on how automated tools used in hiring are designed, deployed, and evaluated.
Authors
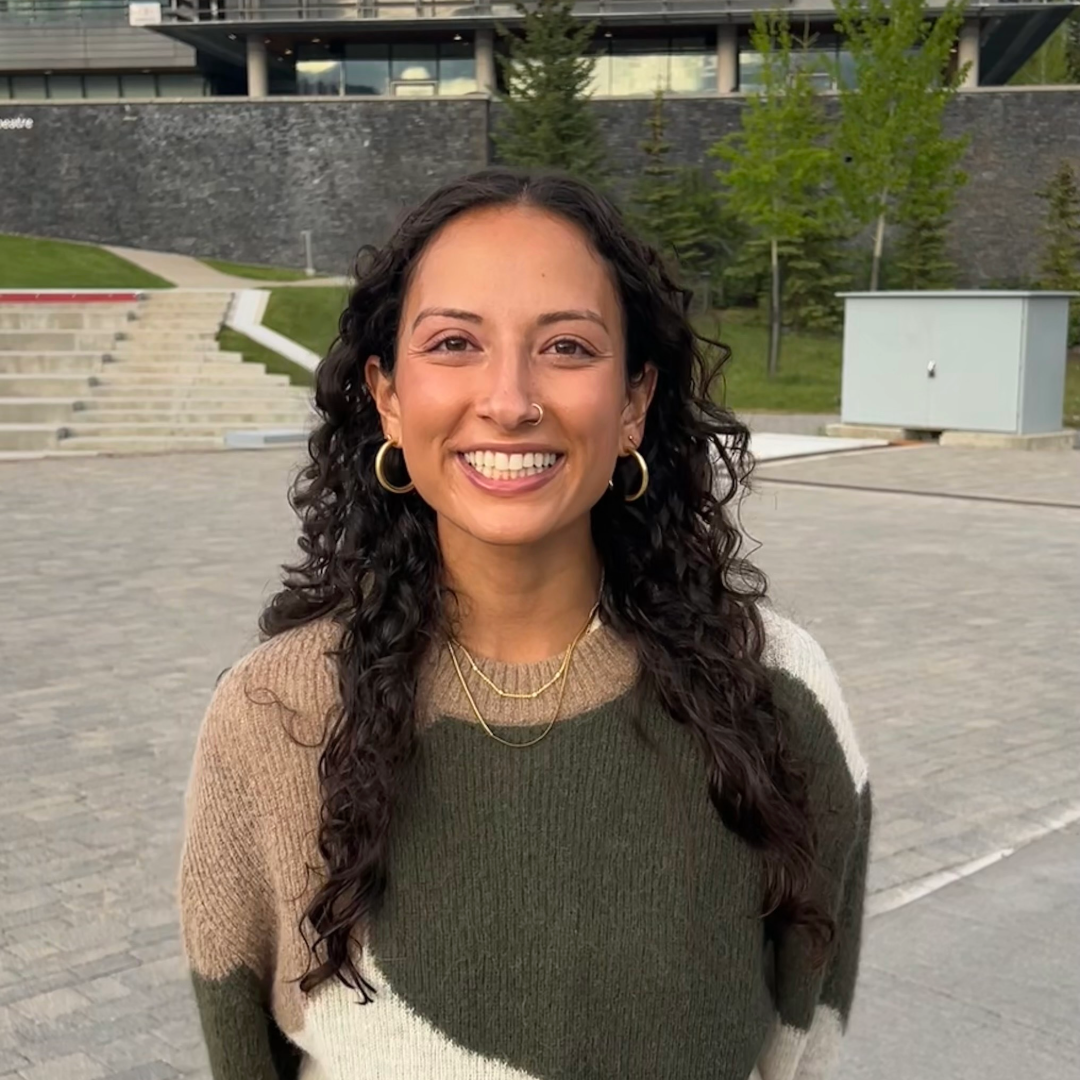
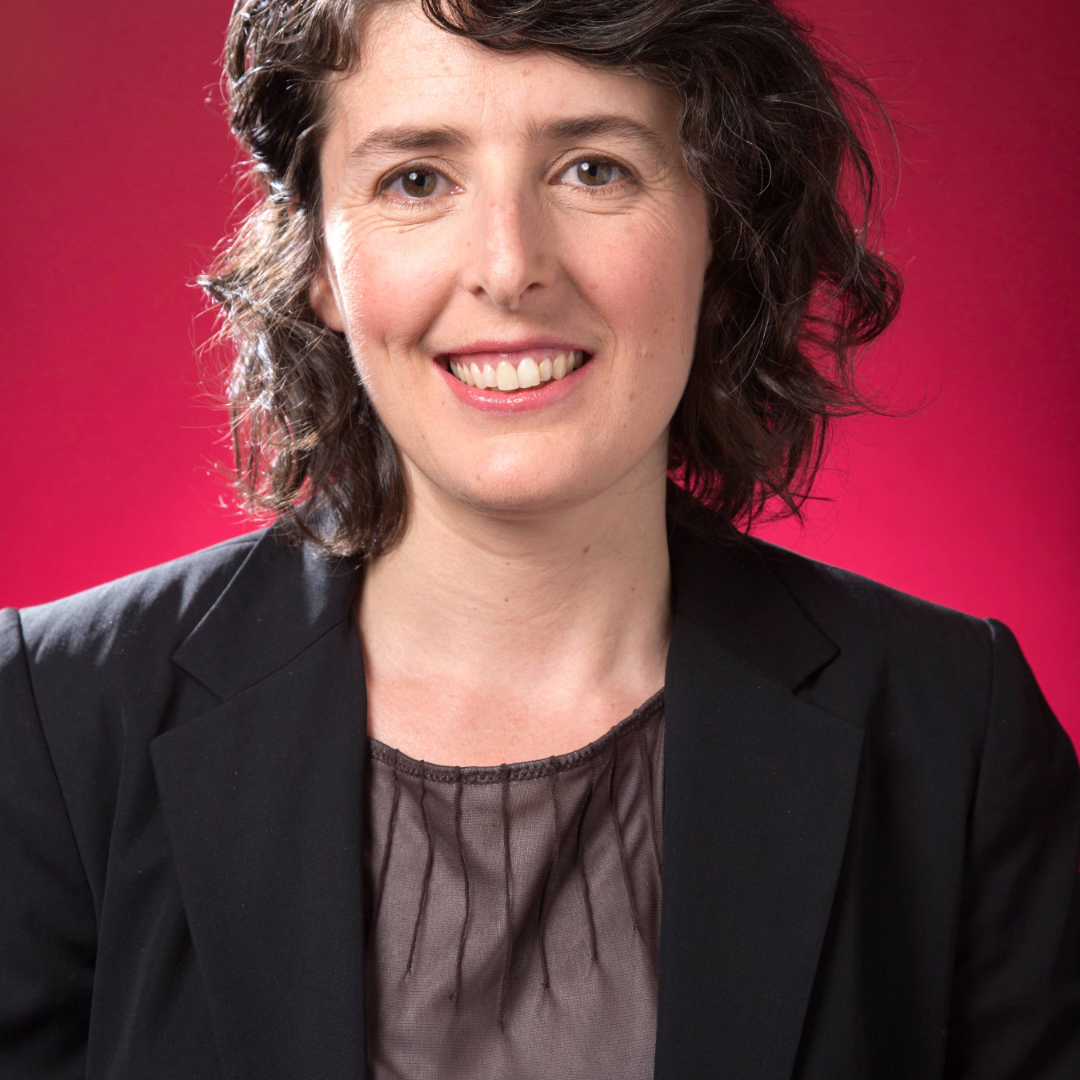