Suffering is Real. AI Consciousness is Not.
David McNeill, Emily Tucker / Feb 19, 2025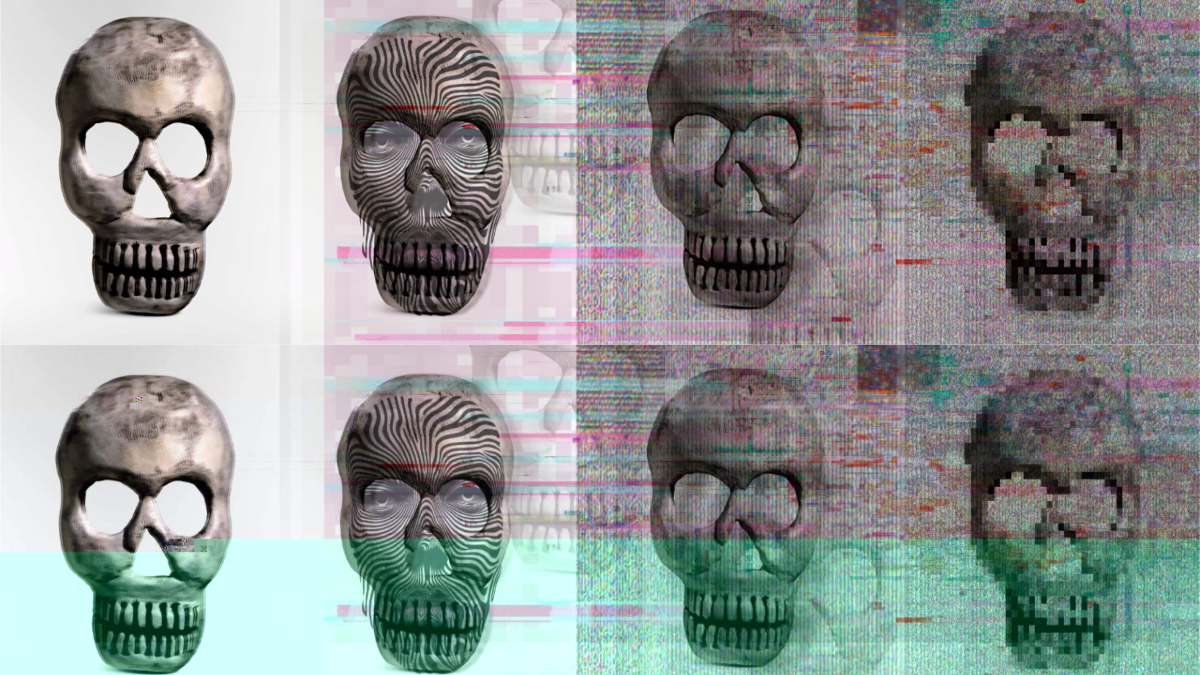
Kathryn Conrad / Better Images of AI / Corruption 3 / CC-BY 4.0
On February 3 of this year, The Guardian ran a story with the headline “AI systems could be ‘caused to suffer’ if consciousness achieved, says research” and the subhead “Experts and thinkers signed open letter expressing concern over irresponsible development of technology.” The story is accompanied by a picture of the actor Stephen Fry, the only signatory to this open letter your average reader would likely have any chance of recognizing. A cursory bit of googling reveals that the letter is glossily hosted by a company called Conscium, which bills itself as “the world’s first applied AI consciousness research organization.” The “research paper” The Guardian refers to is really a statement of “principles” funded by Conscium. It is co-written by Conscium’s co-founder, and a significant number of the signatures are from people directly associated with the company.
The letter sets out “objectives” that are further elaborated in the paper:
“Organizations should prioritize research on understanding and assessing AI consciousness with the objectives of (i) preventing the mistreatment and suffering of conscious AI systems and (ii) understanding the benefits and risks associated with consciousness in AI systems with different capacities and functions.”
One needn’t question the earnestness of Sir Stephen and others who have signed or will sign this open letter to suspect that this expression of concern for the welfare of hypothetical computational “moral patients” is essentially an advertisement (indeed, several of the signatories are advertising executives). It follows in the footsteps of the Future of Life Institute’s open letter of March 2023 calling for a pause to training LLMs “more powerful than GPT-4”—and we know how that worked out. Like that letter, the Conscium missive appears to be an attempt to get out in front of potential regulatory initiatives. The “principles” it offers are meant to be “adopted voluntarily by research organizations themselves” rather than legally enforced. Even if this were a cause we should care about, we should trust the voluntary adoption of principles by technology firms exactly as much as we should have trusted Google’s commitment to “Don’t be evil.”
The emotions these two letters are meant to arouse in the public are diametrically opposed—fear of super-intelligent AI “agents” in the one case, empathy for the suffering of AI “patients” in the other—but the strategy is the same. By appealing to our emotional responses and our readiness to anthropomorphize, they eschew the need for real arguments to convince us of a falsehood—that the chatbot explosion is the leading edge of a real scientific revolution rather than a particular overhyped and oversold application of transformer-based models whose success has depended on a vast scaling up of exploitative data practices and resource-intensive computation. When we ask how and why respectable publications like The Guardian are willing to serve as unpaid vehicles for the self-promotion of outfits like Conscium, we should first look at the ways massive funding initiatives from tech-aligned philanthropic organizations have transformed the relevant research spaces. But whoever may be selling this story we also need to ask, why do so many people seem so willing to buy it?
Addressing that question is the point of No, We Don’t Live In A F%#*ing Simulation, a mini-course we offer through The Center on Privacy and Technology at Georgetown Law Center. As its title suggests, the course is framed by our response to the so-called simulation hypothesis—the sophistry posing as an argument for the claim that it is possible, perhaps even likely, that we are all already just conscious algorithmic processes in a computer simulation. We begin with the simulation hypothesis not because we think it is especially important—it is among the least harmful fantasies of the tech-oligarch set—but because it is a particularly good example of a particularly bad and increasingly pervasive mistake. That is the mistake of confusing the various linguistic, analytic, and formal systems we use in our thinking with that thinking itself.
An algorithmic or computational process is a kind of abstract machine we use in our thinking, it is not a thinking machine. This is the lesson we should draw from John Searle’s famous Chinese Room thought experiment. Rather than seeing it as an argument for ‘biological naturalism,’ we should take it as a reminder that we ourselves can ‘implement’ algorithmic systems in two distinct ways: as a vehicle for our thinking, or mindlessly. Unfortunately, this distinction is very much in danger of getting lost. One example of the mindless application of formal machinery we discuss in the course is the misuse of Bayesianism in both the simulation hypothesis and the arguments of so-called Longtermism. Bayesian formal methods can be very useful for diagnosing and correcting errors in probabilistic reasoning in contexts with which we are relatively familiar—but the more distant we get from those contexts, the more ungrounded those methods are likely to become.
Unfortunately, many current uses of Bayesianism apply the formal machinery of the theory to problems for which there is no reasonable way to establish prior probabilities and no reasonable way of gaining empirical data to update our beliefs. Something has gone terribly wrong if you think it makes any sense at all to assign a numerical probability to your general sense that a perfect simulation is possible and then plug that number into an equation with other numbers representing your hunches about, for example, how likely it is that technologically advanced societies will want to make such simulations, or how many post-human civilizations there will one day be.
These contemporary Bayesians take a certain interpretation of “probability,” which identifies ‘probabilities’ with individual subjective judgments of how likely something is and turn it into a license to pull probabilities more or less out of thin air. One of their most common methods is called “prior elicitation.” This is asking a group of experts in some field for their guesses about the probability of something and using those guesses as a way of establishing your priors. But this would only be a reasonable approach if one had good reasons to think that the experts they were consulting were a) unbiased and b) actually had a good understanding of the thing you were asking them about. In the present case, asking a community of expert AI professionals whether computers can become conscious would only make sense if we thought they really understood consciousness and were not motivated to convince themselves and us that artificial general intelligence was just around the corner.
There are a lot of very good reasons for rejecting the claim that contemporary AI research is on its way toward creating genuinely intelligent, much less conscious, machines that we do not focus on in our course in part because seeing the force of those reasons requires familiarity with more or less technical vocabularies in mathematics, physics or biology. For that reason, even the best of these arguments can tend to reinforce the mistaken impression that thinking about thinking is essentially tied to those technical or scientific vocabularies—or the even worse mistake that thinking is nothing more than the implementation of the correct analytical or theoretical framework.
But—and this is something far too many ‘expert researchers’ seem to forget—theories can’t do our thinking for us. People think, often aided by the use of theoretical frameworks, sometimes blinded by them. Theories are the kind of thing that need to be understood to be used well. And, as anyone who has ever tried to teach a theory knows, any theoretical framework that can be understood can also be misunderstood. Moreover, a theoretical framework that can be well-used can also be misused. One all too common misuse of theory is as a cudgel to convince people that thinking about, for example, free will or conscious human experience is a technical, scientific matter best left to be decided for us by the professionals.
Whatever significant advances may be made in the science of consciousness, consciousness is not and cannot be just a scientific concept. Look at the most doggedly computational approaches to consciousness, and you will see why. For example, the 2023 paper the Conscium researchers cite as the “most systematic recent treatment” on the feasibility of building “conscious AI systems in the near future,” co-authored by one of the Conscium researchers, assumes without argument that it is necessary and sufficient for a system to be conscious if it implements computations of a certain kind. Yet when it comes to attempting to define consciousness, these ‘computational functionalists’ confess to being in a bit of a quandary and instead choose to give various “examples of how we use” the relevant terms.
So—just to be very clear about what is going on here—these computational theorists about consciousness admit that the best they can do when trying to get a grasp on the difference between conscious and unconscious processes is to say, well, you know what we mean by consciousness! And while not all current theorists about consciousness are quite so explicit as this, any theory of consciousness that is not eliminativist—that is, any theory that doesn’t simply deny that consciousness is real—must make some similar gesture.
We need to remind ourselves that the various definitions of consciousness that researchers use must always refer back to our pre-theoretical understanding of and familiarity with conscious awareness. We need to remind ourselves that whatever externally observable behaviors we choose as indicators of intelligence in human beings, we could also enact those same behaviors mindlessly. Imagining that implementing the right computational “artificial intelligence” model could lead to the emergence of consciousness depends on the fundamental and widespread misconception that intelligence and consciousness are concepts that we can understand independently of one another. But however familiar we have become with attributions of intelligence to non-conscious systems, we need to ask ourselves whether it makes any sense at all to think of intelligence as something other than a kind of awareness. For human beings to do something intelligently involves an awareness of what we are doing and, at the very least, the possibility of asking why we are doing it. When we make intelligent use of the various formal, technical, or theoretical frameworks we have at our disposal, we must make an indefinite number of non-formal, non-technical, and non-theoretical judgments about when, how, and why a particular use in a particular context is appropriate. For these reasons, we can be pretty confident that probabilistic generalizations performed on rapidly degrading internet content are not steps on the road to algorithmic moral personhood.
More broadly, and despite what some ‘expert researchers’ would have you believe, our thinking has always required and will always require an awareness of and responsiveness to a world wider than our theories of it, and our language has always depended and will always depend on shared experiences with others of our kind. Our experiences of suffering are among those experiences through which we understand what it is to be conscious. Fantasizing about the potential future suffering of a chatbot is one way to deny that difficult truth at a moment in history when to actually become conscious of the suffering that so many human beings are now enduring requires real courage.
Authors
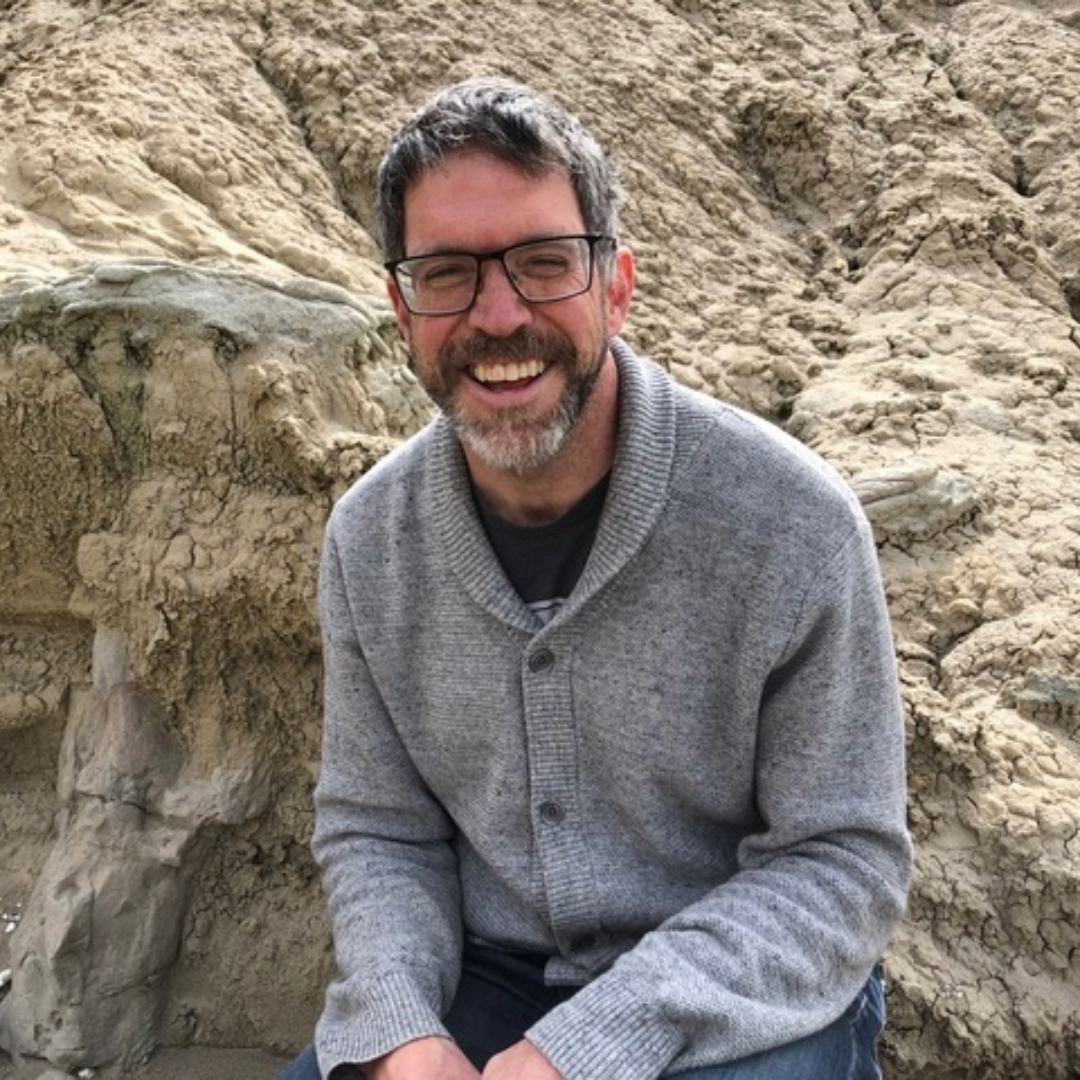
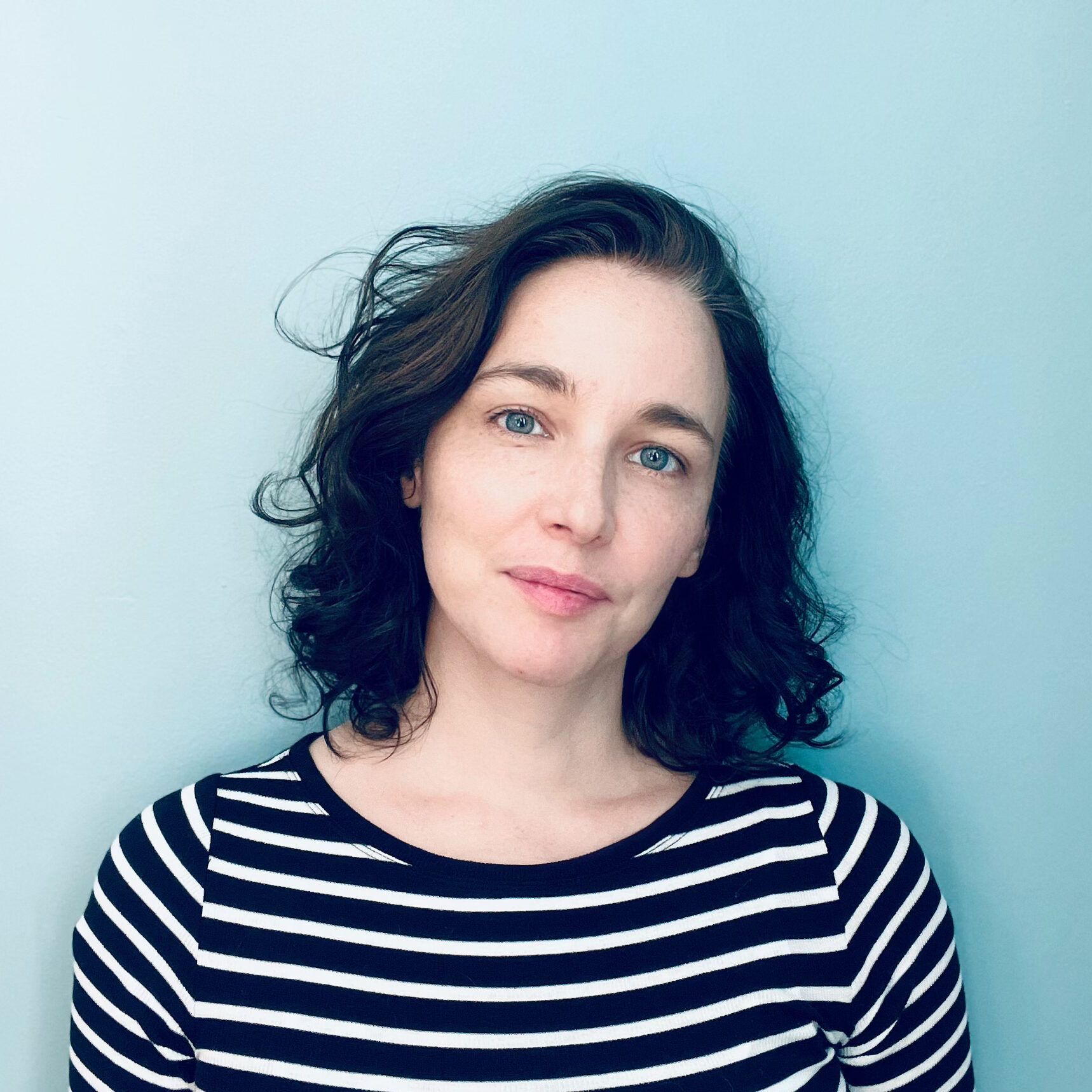