Regulating Transparency in Audiovisual Generative AI: How Legislators Can Center Human Rights
Sam Gregory, Raquel Vazquez Llorente / Oct 18, 2023Raquel Vazquez Llorente is the Head of Law and Policy — Technology Threats and Opportunities at WITNESS. Sam Gregory is the Executive Director of WITNESS.
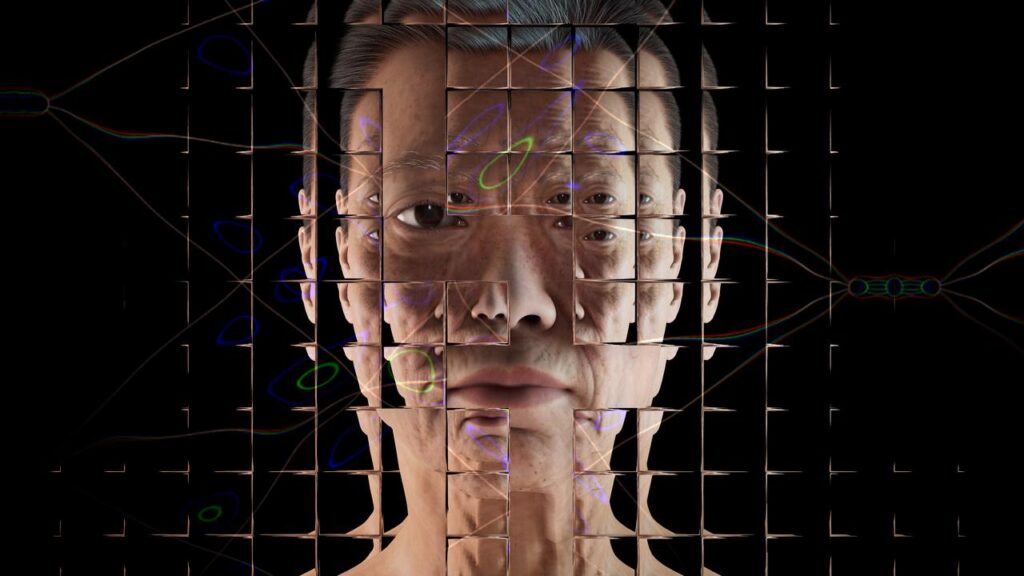
In an era marked by rapid technological advancements, the interplay between innovation and human rights has never been more crucial. While there are creative and commercial benefits to generative AI and synthetic media, these tools are connected to a range of harms that are impacting disproportionately those communities that were already vulnerable to mis- and disinformation, or targeted and discriminated against because of their gender, race, ethnicity, or religion. Given the lack of public understanding of AI, the rapidly increasing verisimilitude of audiovisual outputs, and the absence of robust transparency and accountability, generative AI is also deepening distrust of both specific items of content as well as broader ecosystems of media and information.
As policymakers and regulators grapple with the complexities of a media landscape that features both AI generated and non-synthetic content (and the mix of both), there is much to be learned from the human rights field. Democracy defenders, journalists and others documenting war crimes and abuses around the world have long faced claims by perpetrators and the powerful dismissing their content as fake or edited. They have also grappled with similar questions about the effectiveness, scalability and downsides to tracking and sharing how a piece of content is made. In this blog post, we explore how legislators can center human rights by drawing from the thinking that many human rights organizations and professionals have advanced with regard to transparency, privacy, and provenance in audiovisual content.
Safeguarding the integrity of audiovisual information: legislative proposals
Synthetic media tools are now able to produce images of real-life events and convincing audio of individuals with limited input data, and at scale. Generative AI tools are increasingly multimodal, with text, image, video, audio and code functioning interchangeably as input or output. These fast developments have prompted a growing debate about the need to address transparency in audiovisual content, and the year 2023 has seen several legislative proposals on the table that may affect people beyond jurisdictional borders. For instance, the REAL Political Ads Act–which is a narrower version of Representative Yvette Clarke’s (D-NY) Deepfakes Accountability Act from 2021, revived in September this year–has proposed visible watermarks or signals in AI-based imagery used in election advertising. The AI Disclosure Act by Representative Ritchie Torres (D-NY) requires generative AI output to include a label that notes ‘disclaimer: this output has been generated by artificial intelligence’. Beyond this sparse guidance, the Act does not give further details about how this disclosure should actually be implemented. Similarly, the Advisory for AI-Generated Content Act by Senator Pete Ricketts (R-NE) requires watermarking but leaves the establishment of these standards to government bodies. In Senator Amy Klobuchar’s (D-MN) Protect Elections from Deceptive AI Act, the intention is to prohibit the distribution of materially deceptive AI-generated audio or visual media relating to candidates for Federal office–but the Act includes exceptions if the broadcast or journalistic outlet disclose that the content is ‘materially deceptive’ AI-generated. In September, Senators Richard Blumenthal (D-CT) and Josh Hawley (R-MO), announced a bipartisan framework for AI legislation intended to be a ‘comprehensive legislative blueprint’. Among other proposals, the framework proposes that AI system providers ‘watermark or otherwise provide technical disclosures of AI-generated deepfakes’.
The AI Labeling Act introduced in the US Senate in July by Senators Brian Schatz (D-HI) and John Kennedy (R-LA) is the most detailed proposal regarding transparency in AI generated output to date. It provides guidance about how to incorporate a visible label for image, video, audio or multimedia–separating text in another provision–and includes responsibilities for developers and third party providers. The Act puts forward constructive steps towards the standardization of the ‘how’ of media production (instead of focusing on the identity of the creator), and a more responsible AI pipeline that includes some elements of upstream and downstream accountability. The proposal also goes beyond visible watermarks and includes metadata-based disclosure. Despite these positive notes, uncertainties remain about how a visible labeling requirement that is not specific to a subset of content, like political advertising, could be applied across the board in a meaningful way or without unintended consequences. For instance, the Act says that the disclaimer should be ‘clear and conspicuous’ when the content is AI generated or edited in a way that ‘materially alter[s] the meaning or significance that a reasonable person would take away’. Moreover, for any content that is solely visual or solely audio, the disclosure shall be made through the same means. At a practical level, this raises questions about how the format of the disclaimer would work in tandem with image descriptions or alt-text. The proposal also omits any direct references to the language of the disclaimer, missing the opportunity for a more inclusive technology policy that is grounded in the realities of how digital content spreads.
More importantly, the viability of generalized ‘clear and conspicuous’ disclosures over time is unproven and unclear. For example, with images, audio or video the modification may be just a second or two, part of a frame, communicated in audio but not video, or involve in-painting into a real photo. These could all ‘materially alter the meaning or significance that a reasonable person would take away from the content’ and therefore meet the labeling obligation. In these instances, simply indicating the presence of AI may not be that helpful without further explanation of how it is used and which part of the artifact is synthetic. Additionally, tracking the different usages at scale will bring incredible complexity. Lastly, most visible labels are easily removable. This could happen even without deceptive intent–essentially leaving it to the users to decide whether they think they are materially altering the meaning of a piece of media, but making developers and providers liable for these decisions. It would not be out of order to think that distributing the burden in this way could end up with companies taking an over cautious approach and restricting certain uses completely, such as for political satire.
In the European Union, the AI Act requires image, audio or video content that ‘appreciably resembles authentic content’ to be disclosed as generated through automated means. The EU Code of Practice on Disinformation includes similar voluntary commitments from platforms. In Canada, discussions are ongoing regarding the introduction of measures to label and track AI-generated content to enhance media literacy and combat disinformation. In Australia, policymakers are exploring potential frameworks for the identification and labeling of deepfake and synthetic media. Countries in Asia, including Japan and South Korea, are actively researching ways to promote transparency and responsibility in the AI and media landscape.
Watermarking, labeling and content provenance in a complex media landscape: promises and pitfalls
AI-generated images are already being blended with non-synthetic content–such as videos whose visual content has not been edited using AI, but have synthetic audio; or photographs taken on a phone or camera that have in-painting or out-painting elements. We will hence require systems that explain both the AI-based origins or production processes used in producing a media artifact, but also document non-synthetic audio or visual content generated by users and other digital processes. It will be hard to address AI content in isolation from this broader question of media provenance.
There is not a clear taxonomy around the concepts of watermarking, disclosure and provenance. Visible signals or labels can be useful in specific scenarios such as AI-based imagery or production within election advertising. However, visible watermarks are often easily cropped, scaled out, masked or removed, and specialized tools can remove them without leaving a trace. As a result, they are inadequate for reflecting the ‘recipe’ for the use of AI in an image or video, and in a more complex media environment fail to reflect how generative AI is used. Labels also bring up questions about their interpretability and accessibility by different audiences, from the format of the label, to their placement or language they employ.
Technical interventions at the dataset level can help indicate the origin of a piece of content and be used to embed ‘Do Not Train’ restrictions that could give people more say in who is allowed to build AI models using their data and content. However, many datasets are already in use and do not include these signals. Additionally, small companies and independent developers may not have the capacity and ability to develop this type of watermarking. Dataset-level watermarks also require their application across broad data collections, which brings questions around ownership. As we are seeing from copyright lawsuits, the original content creators have generally not been involved in the decision to add their content to a training dataset. Given the current data infrastructure, they are unlikely to be involved in the decision to watermark their content.
Cryptographic signature and provenance-based standards track the production process of content over time, and enable the reconnection of a piece of content to a set of metadata if that is removed. They also make it hard to tamper with them without leaving evidence of the attempt. The human rights field was a pioneer in developing solutions that are now becoming part of the standardization debate. Microsoft has been working on implementing provenance data on AI content using C2PA specifications, and Adobe has started to provide it via its Content Credentials approach. These methods can allow people to understand the lifecycle of a piece of content, from its creation or capture to its production and distribution. In some cases they are integrated with capture devices such as cameras, in a process known as ‘authenticated capture’. While these approaches can allow creators to choose whether their content may be used for training AI models or other data purposes, they conversely cast doubt on the true provenance of an item when the credentials are misused–for instance if a third party has maliciously claimed copyright over a piece of content that has not been yet cryptographically signed. Legislators should also consider situations in which a user inadvertently removes the metadata from a piece of content, and how this provenance approach fares vis-à-vis existing systems for content distribution (for instance many social media platforms strip the metadata prior to publication for privacy and security reasons).
Invisible watermarks, like Google’s SynthID, generally focus on embedding a digital watermark directly into the pixels of AI-generated images, making it imperceptible to the human eye. These types of approaches are increasingly resilient to modifications like adding filters, changing colors and lossy compression schemes. However, they struggle with scalability and are not yet interoperable across watermarking and detection techniques–without standardization, watermarks created by an image generation model may not be detected confidently enough by a content distribution platform, for instance. Similarly, the utility of invisible watermarking may be restricted beyond closed systems. According to Everypixel Journal, more than 11 billion images have been created using models from three open source repositories. In these situations, invisible watermarks can be removed by deleting the line that generates it. Promising research by Meta on Stable Signature roots the watermark in the model and allows tracing the image back to where it was created, even being able to deal with various versions of the same model.
Centering human rights in generative AI legislation: what we can learn from the frontlines of transparency, privacy and security
For nearly a decade, the human rights sector has been debating about how to balance the need for provenance information with other rights and the risks that this metadata collection may bring, and many organizations have created tools to collect verifiable content that have been widely adopted within niche use cases. Organizations like ours have extensively explored these software solutions and lessons learned as provenance infrastructure started to become mainstream. From these experiences on the avant-garde of content authenticity, we can safely state that there is clear agreement that people should not be required to forfeit their right to privacy to adopt emerging technologies. Personally-identifiable information should not be a prerequisite for identifying either AI-synthesized content or content created using other digital processes. The ‘how’ of AI-based production is key to public understanding about the media we consume, but it should not necessitate revealing the identity of ‘who’ made the content or instructed the tool.
The obligation to embed invisible watermarks or metadata should not extend to content created outside of AI generators. In these situations, it should always be a choice of the user (i.e. ‘opt-in’). We must always view these tools through the lens of who has access to the technology and can choose to use it, which may depend on their security situation and the purpose behind the use, or other sensitive factors. Building trust in content must also allow for anonymity and redaction of non-AI content, which becomes more complex when synthetic and non-synthetic artifacts co-exist in a piece of media. Lessons from platform policies around the use of ‘real names’ tell us that many people—for example, survivors of domestic violence—have anonymity and redaction needs. While specifications like the C2PA focus on protecting privacy and do not mandate the disclosure of individual identity, this privacy requirement needs to be protected and explicitly mentioned in legislative proposals. We should be wary of how these authenticity infrastructures could be used by governments to capture personally identifiable information to supercharge surveillance and stifle freedom of expression, or facilitate abuse and misuse by other individuals.
We should also ensure that technologies that track how media is made are interpretable across a range of technical expertise. Provenance data for both AI and user-generated content helps understand the integrity of the media and provides signals—i.e. additional information about a piece of content—but does not prove truth. An ‘implied truth’ effect simply derived from the use of a particular technology is not helpful, nor is an ‘implied falsehood’ effect from the choice or inability to embed a watermark or cryptographic metadata. Otherwise we risk, for instance, discrediting a citizen journalist not using provenance solutions to assert the authenticity of their real-life media, while we buttress the content of a foreign state-sponsored television channel that does use a cryptographic signature. Their journalism can be foundationally unreliable even if their media is well-documented from a provenance point of view.
Where to go from here: A multilayered technical, regulatory and societal approach to synthetic media
Transparency has become a central issue in the debate around AI content production. Regulators and policy-makers should ensure human rights are reflected in the language of their proposals, paying special attention to privacy protections, the optionality of use, and issues around accessibility and interpretability. Regulatory proposals should avoid introducing a blanket requirement for compulsory disclosure of audiovisual AI content unless carefully thought through (while recognizing that for some types of content, visual disclaimers can be useful). For audiovisual content that is not produced via AI, legislators should steer clear from legally requiring that provenance data is embedded or visibly disclosed. Overall, mandatory propositions around content provenance should be mindful of how they may stifle freedom of speech or deter technology whistleblowing, or worse, enable surveillance and open the door to government misuse across the globe.
Most of the regulatory attention is centered on visible disclaimers and provenance data. Communicating how content is produced can help us navigate a media complex landscape, but we must urge legislators to not focus exclusively on this approach. Responses to the risks of generative AI tools cannot be adequately addressed by regulatory agencies or laws alone without a pipeline of responsibility across foundation models, developers and deployers of AI models. Implementing a comprehensive approach to transparency will also require standards that focus on how to design resilient enough machine-readable solutions that can provide useful signals to information consumers, as well as other actors in the information pipeline, such as content distributors and platforms. Single technical solutions will not be sufficient, though. These initiatives should be accompanied by other measures such as mandatory processes of documentation and transparency for foundation models, pre-release testing, third-party auditing, and pre/post-release human rights impact assessments. Lastly, media literacy will still be key to help users be inquisitive about the content they are interacting with, but it is unreasonable to expect people to be able to ‘spot’ deceptive and realistic imagery and voices.
The basis for this piece is the written testimony given by Sam Gregory, Executive Director, WITNESS on September 12, 2023, before the U.S. Senate Committee on Commerce, Science and Transportation. You can read the transcript from the hearing here or watch it here.
Authors
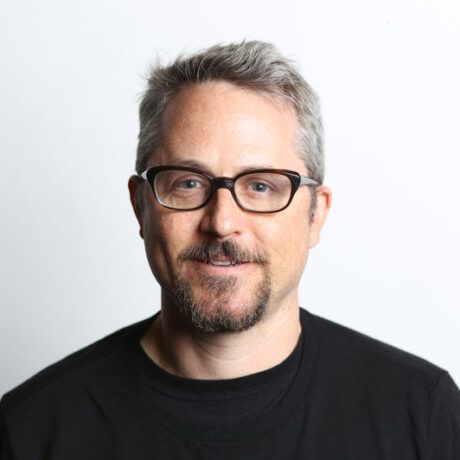
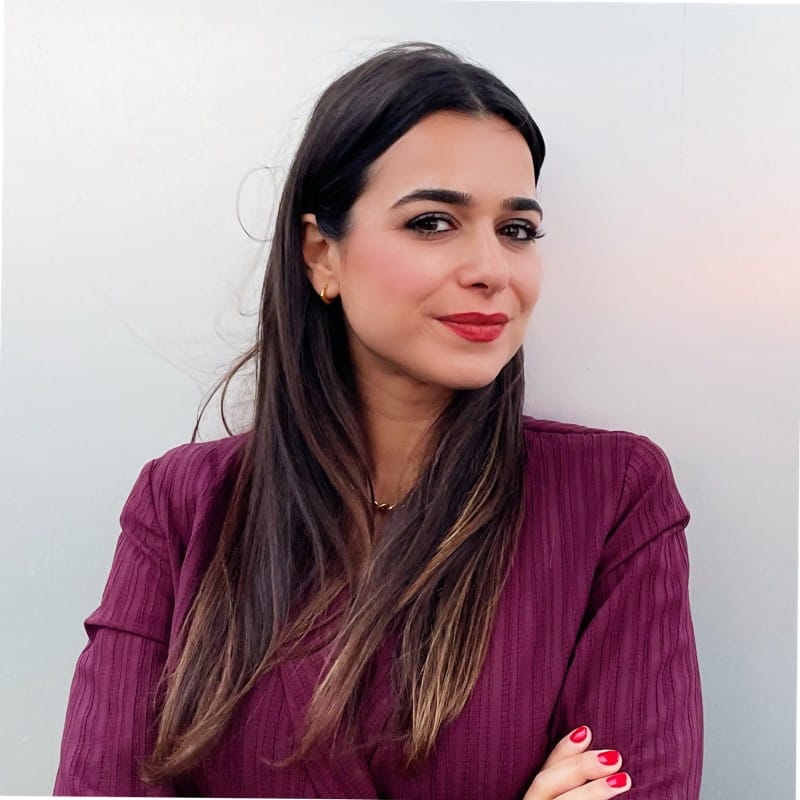