A Q&A with Stefaan Verhulst, Co-Founder of The GovLab and The Data Tank
Akash Kapur / Apr 17, 2025This piece is the second in a series in collaboration with New America.
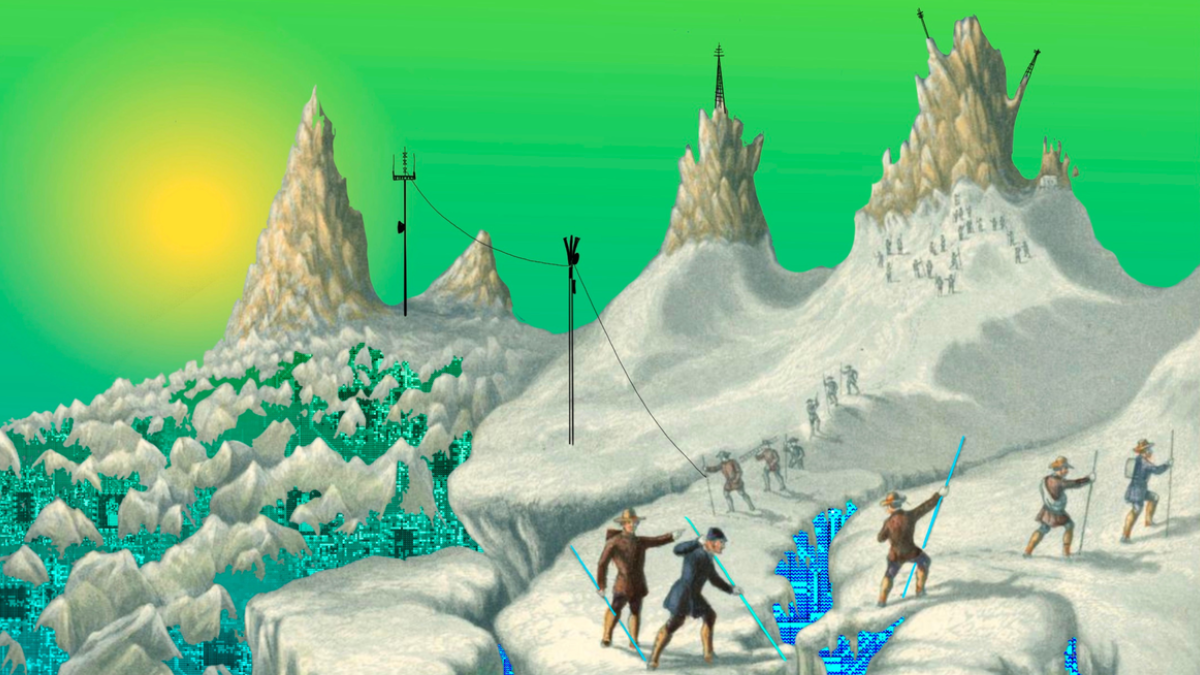
Data Mining 3 by Hanna Barakat & Archival Images of AI + AIxDESIGN / Better Images of AI / CC by 4.0
Although it is widely recognized that we live in a thoroughly datafied world, there is less understanding of just what this means and how we should respond. The public conversation is replete with somewhat facile metaphors about data being the new oil, or data as fuel for AI. Yet the policy and normative issues surrounding the “data value chain”–from collection to storage to use, and then reuse–are complex, involve difficult tradeoffs, and require nuanced, out-of-the-box responses.
To better understand this nuance, I spoke to Stefaan Verhulst, who has been thinking and writing about data since long before it became fashionable. Verhulst is the co-founder of The Governance Laboratory, or the GovLab (where I am also a Senior Fellow), based in New York City, and The Data Tank, a Brussels-based organization focused on reusing data for the common good. For over a decade, Verhulst has been writing about, among other topics, the importance of open data, the need to break down data silos, and the thorny yet vital questions surrounding how private data can be reused for the public good. This work has pushed the boundaries of conventional thinking, recognizing that data represents a unique kind of individual and social asset, very different from existing ones in the analog world, and needs to be governed accordingly.
Among various other roles, Verhulst is also the Editor-in-Chief of Data & Policy and Research Professor at the Tandon School of Engineering and the Center for Urban Science and Progress at New York University. In 2018, he was recognized as one of the 10 Most Influential Academics in Digital Government globally by the policy platform Apolitic.
You’ve been writing for over a decade now on the importance of data and how to govern it—long before AI brought it to the fore of the policy conversation. I’m wondering, as you survey the current landscape, what are the most pressing issues you see with regard to data and how it’s collected, handled, and governed?
As I see it, the biggest data challenges today fall into three key areas.
First, we are dealing with an explosion of data generation—a process called “datafication.” We’re collecting more data than ever before—everything from Internet of Things (IoT) sensors to satellite imagery and even biological data. But with this explosion come some big questions. How do we ensure equitable access to this data? How do we break down silos and ownership of data without sacrificing citizen and group privacy? What should the balance be between private control of individual data and public benefit? The governance issues are really challenging.
Second, we are seeing a shift in emphasis to unstructured data. Existing governance models were mostly built for structured data like spreadsheets and databases. But now AI is making sense of unstructured data—videos, audio, social media–and that raises tough questions about accuracy, bias, and responsible use. Among other things, we need better metadata standards and improved provenance-tracking to keep up.
Finally, we have a number of issues related to data control and asymmetries. Today, a handful of big companies control vast amounts of data while researchers, civil society, and even governments struggle to access that data. This risks reinforcing existing political and economic power imbalances. So we need initiatives like stronger data-sharing frameworks, public-private collaboratives, and new incentives for responsible sharing to promote wider data access.
It’s become fashionable to talk about data as “fuel” for AI. But beyond that broad statement, what are some of the key issues you see when it comes to surveying the intersection of AI and data?
The metaphor that data is fuel for AI, while true, also oversimplifies things. For AI to work, the data it learns from needs to be high-quality, well-labeled, and responsibly sourced. That’s where the idea of “AI-ready” data comes in. AI-ready data is a reminder that not all data can be or should be used as fuel for our AI systems. Rather, we need data that embodies a few key properties.
For one thing, AI-ready data requires clear sourcing and ways of establishing provenance; these could include data lineage tracking, high-quality metadata, and audit trails. Bias mitigation is also key, for example, by curating, labeling, and structuring data. All of this is really important because without high-quality data, there’s a risk that AI will simply entrench biases and exacerbate asymmetries. That’s why there is a movement toward “Data-Centric AI.”
The idea of data-centric AI begins with a recognition that the traditional approach to AI has been largely model-centric—improving algorithms while overlooking or minimizing the critical role of data. Data-centric AI places data at the heart of the AI equation. It recognizes that better data– not just better models–is key to AI performance. I strongly believe that the real challenge with AI is not just accumulating vast amounts of data but ensuring the quality of that data.
Earlier in your career, you wrote a lot about open data. That was during a period of great abundance. More recently, you’ve been writing about the risks of a “data winter.” Can you tell us what you mean by that?
I introduced the concept of a “data winter,” drawing an analogy to the well-known “AI winters”–long periods when AI research stagnated for various reasons. The idea behind a data winter is that, despite the growing volume and importance of data in the AI era, we are in fact seeing a decline in the accessibility of data. So from a period of abundance, we are–somewhat paradoxically–moving to one of scarcity.
There are many reasons for this data winter, which I believe we are already in. A key underlying driver is the fact that most data is today controlled by a few very powerful actors, especially from the private sector; this inherently creates access bottlenecks. To these asymmetries of access, I would add the fact that much of the data we have isn’t “AI ready,” meaning it is messy or exists in siloed, unstandardized forms; this makes it hard or impossible to access.
Also, companies and others who hold data often do so because they lack clear incentives to share data. Often, they consider data as a competitive asset; this is a longstanding problem that is probably getting worse in the AI era, given all the attention to the value of data. In addition, I think it’s also worth pointing out that companies may feel they are actually disincentivized from sharing data because of privacy, copyright, and other laws. Our legal system isn’t really set up to promote data sharing.
Finally, I’d point to an overall erosion of the open data ecosystem and sharing infrastructure. When I first started working in this field, open data was understood to be necessary and even virtuous, especially in governments. That’s a far cry from today, when many public sector data programs are underfunded and insufficiently backed. Overall, I think it’s clear there’s been a global decline in government transparency and accountability–this is part of a far bigger problem than just data.
What can we do to address this data winter?
Addressing the data winter is critical—both for the public good and also for fostering private sector innovation. It’s a tough problem, but I think a few things could help.
First, we need to strengthen and streamline demand. At the moment, even when data exists, it’s often not made accessible by companies because there’s no clear demand for it or even an understanding of how it could be beneficial. So, we need a much better understanding of how and what types of data can be useful in addressing core societal needs. We need, in effect, a stronger match between supply and demand. One way to do this, as I’ve argued elsewhere, is to work on developing and publishing high-impact case studies where the links between data and public impact are made clear. This would help civil society, governments, and other stakeholders decide which data to make available and to whom.
I’ve also been a strong advocate for developing and supporting the incipient profession of data stewards. These are professionals within organizations who are responsible for managing data assets responsibly, including developing partnerships for sharing with external organizations. Several organizations already have equivalent roles. I think that investing in data stewardship, for example, through training, can help organizations build internal expertise to navigate complex data governance challenges and develop when and how to make data accessible.
And finally, another idea I (and others) have pushed for is to support data collaboratives, especially those that work across sectors. For example, data collaboratives can encourage companies to share data with researchers, policymakers, and civil society so that private data is repurposed for the public good. In some cases, these collaboratives can be formal entities that even hold or manage data assets. In many others, they may simply be loose associations that work across sectors and stakeholders to promote responsible use and reuse of data—and, more generally, encourage openness and resist the data winter.
This discussion of data reuse brings me to my final question. Many experts (yourself included) argue that reuse allows private data to be repurposed for the public good. But when most users hear this, they may worry about their privacy. What would you say to them? Why is data reuse in their interests?
I completely understand why people are concerned about privacy when they hear discussions about data reuse, especially when the reuse concerns sensitive data. At the same time, for too long, we have been so focused on the risks of reuse (which are real; I do not deny that they are) that we have overlooked some of the potential societal and public benefits. As the saying goes, we’ve been too focused on the danger of misuse and not enough on the risk of missed use.
To get over these challenges, I think we need a new approach—one that is sometimes called a “social license” for data reuse. The idea of social license is that we move beyond the need to seek individual consent for each instance of data reuse and instead rely on broad societal agreement (expressed through norms, principles, and laws) that establish under what conditions, and for which purposes, data can be reused. For example, we now know that individual searches and social media posts regarding flu or other illness symptoms can help medical professionals respond early to disease outbreaks. Some of this data is accessible, but much of it is restricted because of privacy and other concerns. Would it be possible to have broad societal agreement that it is permissible to share individual data, only in anonymized and aggregated form, when it could contribute to public health? Or perhaps a society may determine that such sharing is only permissible in a pandemic state or when there is a medical emergency?
The issues involved are complicated and nuanced. It isn’t simple to arrive at a consensus for a social license for sharing. It requires actively involving people in decisions about how data is used rather than treating them as passive data subjects. Some tools and mechanisms that could be used include citizen assemblies and participatory audits (which are ways for the public to keep track of how its data is being used and ensure accountability). These and other deliberative processes can help define what types of data use are acceptable, under what conditions, and how the benefits should be distributed among the public.
Again, I’m not minimizing the complications or the potential risks. But we definitely need better ways to access the vast insights hidden away in the private, siloed databases that today define our digital ecology. I think it’s also important to point out that the goal of translating private data into public insights isn’t just for some abstract “public good.” By enhancing fields as disparate as health, law and order, agriculture, and education, data reuse can play a critical role in improving the quality of life for the very individuals whose data is being shared.
Authors
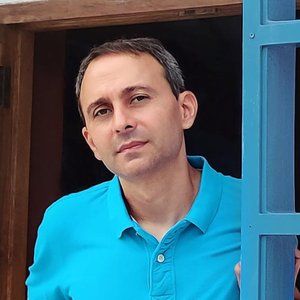