Most Researchers Do Not Believe AGI Is Imminent. Why Do Policymakers Act Otherwise?
Eryk Salvaggio / Mar 19, 2025Eryk Salvaggio is a Fellow at Tech Policy Press.
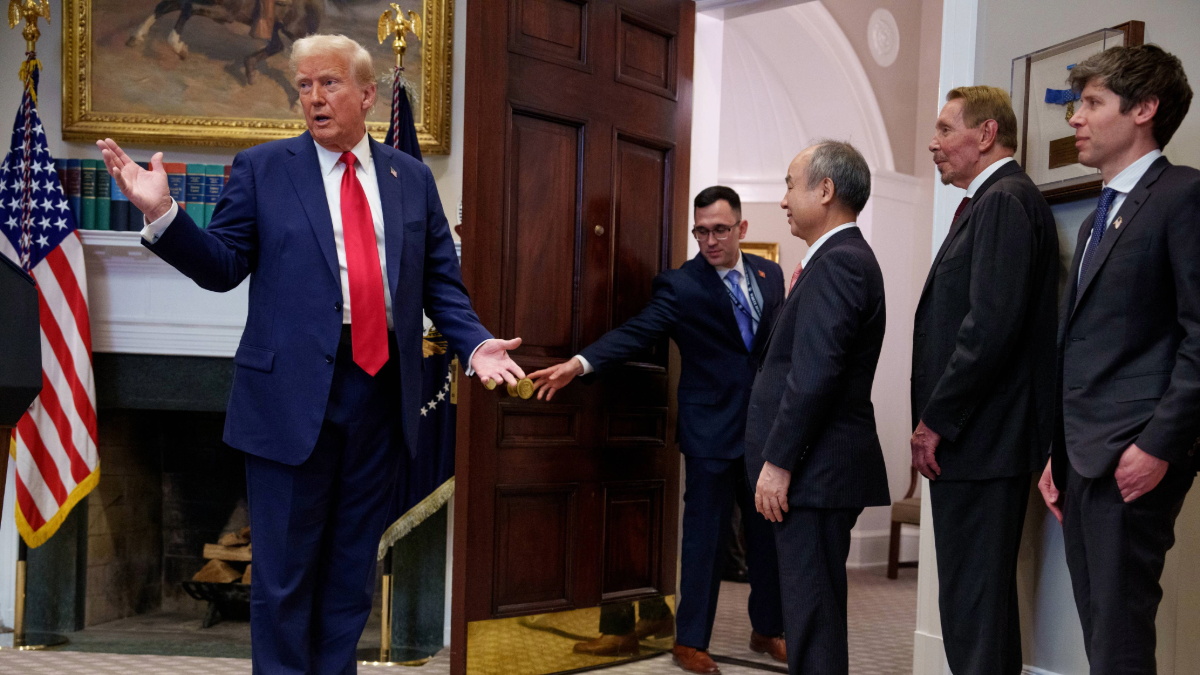
WASHINGTON, DC - JANUARY 21: US President Donald Trump, accompanied by (R-L) , OpenAI CEO Sam Altman, SoftBank CEO Masayoshi Son, and Oracle co-founder, CTO and Executive Chairman Larry Ellison in the Roosevelt Room of the White House for the announcement of the Stargate project. (Photo by Andrew Harnik/Getty Images)
AI research—these days primarily driven by corporate interests—often embraces strange priorities. Amidst multiple crises in public health, climate, and democracy, we could do better than synthetic image and text generation products and personalized chatbots as the defining technologies of our era.
But the companies hyping these products tell us every improvement demonstrates progress toward an even stranger goal: artificial general intelligence, or AGI. The splashy announcement of any new model is cast as evidence of the inevitable trajectory toward machines that learn and act as humans do. New capabilities are pitched as steps toward the goal of machines that may even outperform humans. Investment in the companies that build these systems is, of course, heavily dependent on this promise.
Around the world, policymakers appear increasingly eager to satisfy the interests of tech firms that claim they can deliver AGI. Perhaps it’s natural—if you were a politician or a head of state confronted with a complex, interconnected set of problems with no immediate solution, you might crave the answer these companies are selling. And you might be more than a little hungry for the type of transformation that such technology might create under your leadership.
However, there is danger in making AI policy goals just as invested in the promise of AGI as are the tech sector's leaders. When policymakers buy the hype, the public pays for it.
AGI is Unlikely in the Near Term
First, it’s important to establish that there is good reason for skepticism about claims that AGI is imminent, despite the speculative fever amongst industry figures and some in the press. A recent survey of 475 AI researchers by the Association for the Advancement of Artificial Intelligence (AAAI) conducted as part of its panel on the future of AI research found that “[t]he majority of respondents (76%) assert that ‘scaling up current AI approaches’ to yield AGI is ‘unlikely’ or ‘very unlikely’ to succeed, suggesting doubts about whether current machine learning paradigms are sufficient for achieving general intelligence.” The many limitations of transformer-based architectures suggest AGI is hardly right around the corner.
But such concerns do little to detract from the hype. One reason AGI is particularly susceptible to hype is that it is such an ill-defined concept. AGI serves as a thought exercise rather than a practical research agenda, and as a thought exercise, it distracts from better-defined goals that researchers and policymakers could work to achieve in the near term. The majority of the experts polled by the AAAI would appear to agree: 77% prefer prioritizing the design of AI systems with an acceptable risk-benefit profile “over the direct pursuit of AGI.”
In a recent paper, "Stop treating 'AGI' as the north-star goal of AI research," I worked alongside authors with various views from industry, policy, and academia. Together, we presented six traps — mechanisms through which an overvalued orientation to AGI interferes with achieving more scientifically sound, socially beneficial goals through AI research.
Here, I lay out the traps raised in that paper from my own perspective, which should not detract from the original paper's more carefully described consensus view. It does not necessarily reflect the views of that paper's other co-authors. My goal is to challenge policymakers to approach industry promises with skepticism and to be doubly suspicious of policy proposals championed by industry that center AGI as if it were necessarily in the public interest.
Trap #1: The Illusion of Consensus
Policymakers need to understand that AGI is a contested term. A recent conversation between New York Times columnist Ezra Klein and Ben Buchanan, the former special adviser for artificial intelligence in the Biden White House, offers a case study of the difficulty of landing on a single, consistent definition of AGI. During their discussion, AGI is heralded as "doing basically anything a human being could do behind a computer — but better," then reduced downward to "extraordinarily capable A.I. systems," and then escalated to "a system capable of doing almost any cognitive task a human can do," and then hedged once more as "not that," but "something like that," before becoming constrained to "its capacity to, in some cases, exceed human capabilities" (emphasis mine). Finally, Klein and Buchanan appeared to settle on "systems that can replace human beings in cognitively demanding jobs," which, to be clear, could apply to calculators and automated telephone switchboards.
Klein and Buchanan are not alone. Definitions vary widely. Anthropic CEO Dario Amodei defined AGI as "smarter than a Nobel Prize winner across most relevant fields — biology, programming, math, engineering, writing." In contrast, Microsoft AI CEO Mustafa Suleyman suggests AGI is any system that can turn $100,000 into $1,000,000. The bottom line for policymakers? Pretending there is a shared definition of AGI could distort the discussion before it even starts.
Trap #2: Supercharging Bad Science
Making AGI the goal can lead to bad science, especially when there is so much pressure to show progress. Claims that AI has been getting "10x better every six to nine months" provide a vague assessment of model performance with no statistically relevant meaning — part of a broader trend of meaningless benchmarks that confuse observers and overstate achievements.
Moreover, making AGI the goal heightens confusion between scientific research and engineering research. Engineering can validate through a practical lens ("does this system work?"), while scientific inquiry relies on validating or invalidating a hypothesis ("does this work for the reasons I believed it would work?"). In orienting AI research toward engineering, claims about intelligence are uncritically verified by actions such as observing LLMs as they write sentences. In that example, fine-tuning these systems to produce better illusions of intelligence can create an engineering marvel. But the successful replication of writing does not scientifically validate any hypothesis that a system is, therefore, necessarily on a trajectory toward so-called "general" intelligence.
This creates an environment where bad science abounds, incentivized by marketing hype that discredits meaningful AI research in other domains. It cultivates a tendency to assert unscientific interpretations of what the models produce after the fact, which inevitably aligns with whatever the research has set out to do. An engineering problem is solved when the structure works: a bridge bears the weight. However, a bridge bearing weight does not confirm one's hypothesis that bridges possess an understanding of gravity.
There have been endless debates about whether AI systems "understand language," but without AGI as a guiding factor, we might focus more on the value such understanding might bring to the task — i.e., why does understanding even matter? If we don't just assume that machines that understand is a goal unto itself, then we might ask, instead, what capacities (and benchmarks) do we need to move toward clearer, more precise goals?
With this frame, we could also evaluate an LLM on its merits rather than debate where it fits on some hypothetical path to a "general understanding" of the world. By underspecifying what AGI is and how it is measured, nearly anything can be framed as a "step closer to AGI." That alone does not explicitly justify widespread adoption, nor should it. And it certainly doesn’t merit putting the pursuit of AGI at the center of a scientific or industrial policy.
Trap #3: Presuming Value-Neutrality
A multitude of values compete in our world. Vague and context-free value assertions about the capacities of hypothetical AGI systems undermine the ability of AI research to build socially useful technology. One person's idea of "fairness" in one context does not always apply to another's definition of "fairness" in other contexts. Navigating such discrepancies is crucial to developing socially responsive technology. If AGI is the sole aim, a goal set for the sake of its own achievement, then we replace the set of values technology might serve with a technical achievement.
By framing goals as purely technical or scientific in nature, the AGI orientation resists political, social, or ethical considerations that matter most to society. But AGI is not a value-neutral concept. AGI remains a speculative technology, which is to say it is not a technical system at all. Instead, in the present, it is an imagined social arrangement. Any AGI system requires a specific articulation of politics to be feasible. Likewise, AGI imaginaries often center a highly rational "superhuman" decision-maker at the center of society, valuing a particular form of problem-solving over democratic decision-making (and its frequently illogical decisions).
At scale, AGI's false claim to value-neutrality reduces meaningful debates about which values to prioritize, restricting their premise to somebody's idea of so-called "alignment." Alignment discourse — in which machines are built in "alignment" with "human values" takes an overly broad view of the human. In practice, human values are (and must remain) constantly contested. Most humans are not involved in AGI discourse, which recasts political debates within a framework of technical problems with technical solutions.
These conversations sometimes ask how AI might help us understand our resources and collectively decide how to distribute them. More often, they ask how to build an AI intelligent enough to distribute resources on our behalf. It should be obvious why policymakers should be exceptionally skeptical of this frame.
Trap #4: Goal Lottery
Designed technology does not emerge in a vacuum: it requires investments and promises of return on those investments. It requires resources, and the availability of resources can shape the approach to solving a problem. While not exclusive to AGI, goal lottery reflects how incentives, circumstances, and/or luck drive the adoption of goals regardless of scientific, engineering, or societal merit. This relationship between available tools and what is socially desirable can create misfit technology.
Today, funded AI research seems stubbornly bonded to consumer data and social media metrics because both are prevalent and accessible. However, funding and availability do not necessarily provide the correct incentives or pathways toward solving the most meaningful problems.
Chasing state-of-the-art technologies excludes the development of technologies and tools designed for more specific problem areas. It can also shape technology based on mismatched priorities. In the end, we build a decision-making engine on data collected to meet marketing KPIs rather than the data a better world would need. Policymakers should avoid interventions (or failures to intervene) that lock in — or become dependent upon — these dynamics.
Trap #5: Generality Debt
AGI sets another trap for better decision-making by emphasizing future states of generality and flexibility while postponing crucial engineering, scientific, and societal decisions. For example, some experts are doubling down on energy usage while hoping that AGI will tell us how to solve the climate crisis. AGI must not create a rhetorical or technical excuse to "kick the can down the road" in mitigating immediate social and technical problems.
Emphasizing AGI as the goal — and extrapolating that, once achieved, AGI will solve the backlog of problems ignored over its pursuit — creates a dependency upon technical advances that may never materialize. This backlog includes crucial decisions about what the values and purposes of any given technology are meant to serve.
This compounds the problem of bad science: after all, if there is no clear definition of generality for these systems, how do we know a "general" technology will solve them? Rather than hoping the "right" AGI emerges, why not intentionally design technologies that help us tackle specific, narrower challenges? Policymakers can create conditions and incentives for this latter approach.
Trap #6: Normalized Exclusion
Excluding communities and fields of expertise from shaping the goals of AI research limits the development of innovative and impactful ideas. The orientation to AGI can exclude input from most social groups, regardless of their expertise. For example, there is sparse AGI input from labor unions despite AGI's (mostly agreed upon) goal of transforming labor.
Likewise, those who work to develop AGI pass through a filter that sorts believers from unbelievers: it would be rare for students to pursue skills for a career they did not believe possible or desirable.
It is appropriate to turn here toward the paper's solutions, which encourage three key ideas for AI research that seem more important than AGI: inclusion, pluralism, and specificity. Policymakers should use the tools available to them to steer conversations away from the development of catch-all systems designed with limited input while operating under an umbrella that is too wide. In its place, we should focus on developing more precise and clearly articulated scientific, engineering, and societal goals — and reward work that rises to meet them.
Three Points
- Specificity: Be very clear about what AI research is setting out to do, and likewise, bring greater care to how the results of these experiments are interpreted.
- Pluralism: Rather than relying on the purely technical goal of "AGI" to solve collective problems, aim to design tools based on collective input, from multiple fields and communities.
- Inclusion: Identify and seek out those impacted by AI systems to ensure the goals pursued address their needs and concerns.
These points are straightforward invitations to critique for policymakers and others monitoring the industry and the development of AI. Do not be swayed by promises of generality in systems: let's engage with a greater variety of disciplines (including the social sciences and humanities) and communities (beyond the tech world) to identify specific problems that AI could help humans solve, rather than assuming the tools will solve them on their own. Demand specificity whenever AI research claims systems can, or will do, anything without human input or oversight, and be critical of the assumption that this would always (or ever) be desirable.
Finally, it’s been argued that AGI inspires ambitious scientific undertakings and awakens the imagination of scientists. If we are in need of ambitious projects, we only have to look around. We walk on the surface of a deeply troubled, complex system; we engage with one another in ways that complicate and stall progress on that which jeopardizes all of it. There is no shortage of ambitious challenges demanding our attention and imagination that the field of computation could help us understand.
I deeply appreciate the original “AGI” paper’s co-authors for their thoughts and conversations.
Authors
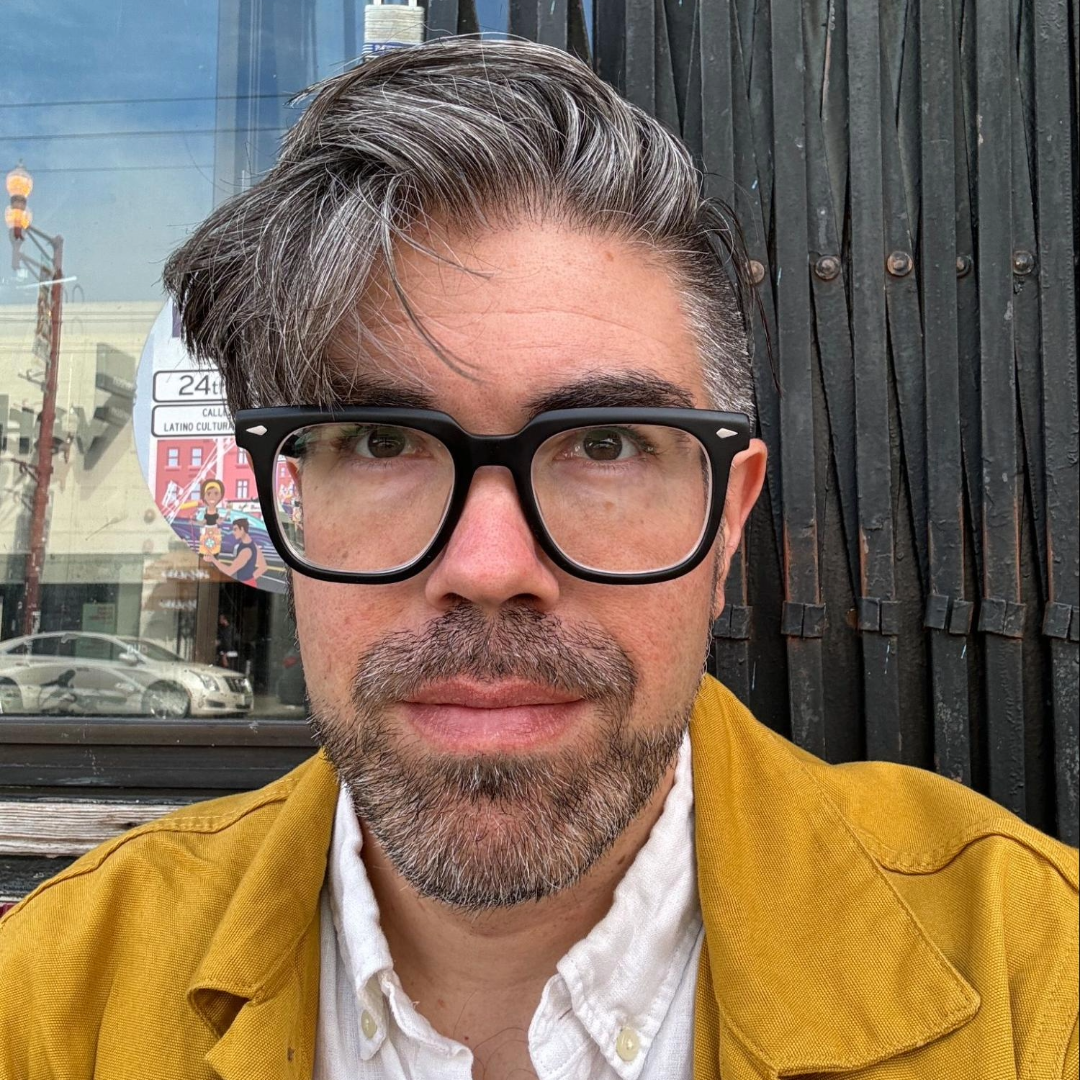