From Upload to Output Filters? How the AI Act's Code of Practice Could Threaten Freedom of Expression and Thought
Caroline De Cock / Jan 17, 2025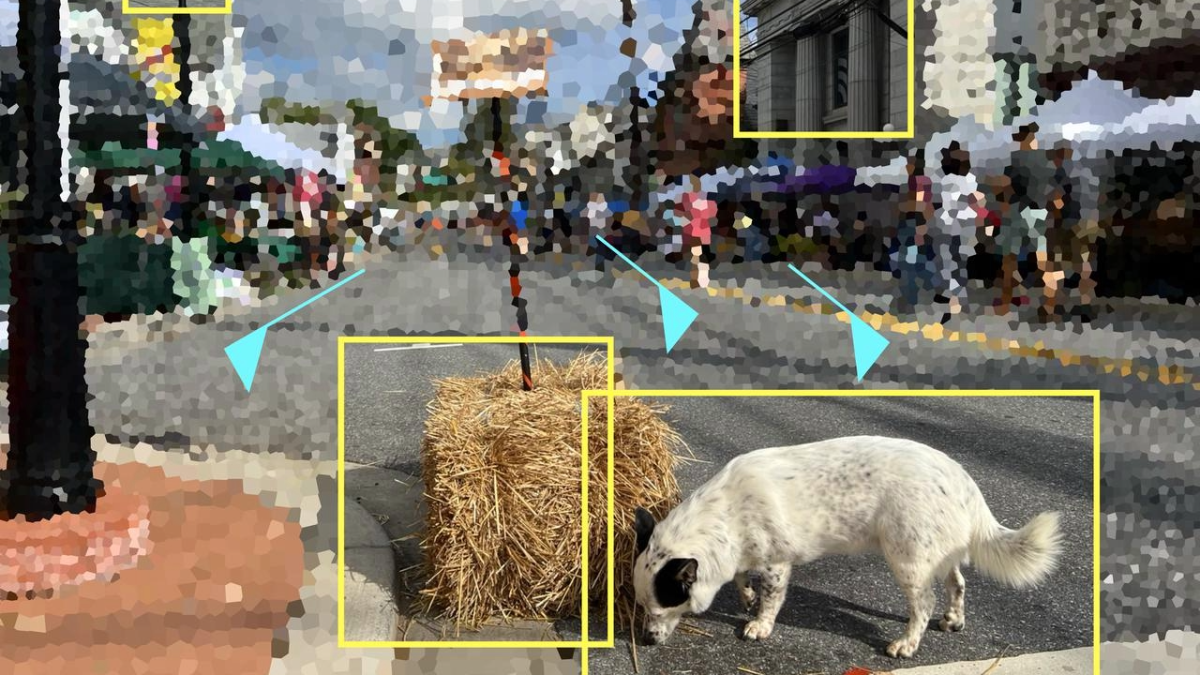
Elise Racine / Better Images of AI / Street Fair / CC-BY 4.0
The European Union’s AI Act is a landmark effort to regulate artificial intelligence, aiming to balance fundamental rights, creativity, and innovation. However, the second draft of the General-Purpose AI (GPAI) Code of Practice introduces measures that risk undermining this balance. Specifically, Measure 2.9 on copyright-related overfitting seeks to address copyright concerns by imposing strict compliance obligations on the outputs of generative AI systems. This approach risks replicating the original logic of the controversial "upload filters" proposed under Article 17 of the Copyright in the Digital Single Market Directive (CDSMD). Yet, it does so in a fundamentally different and arguably more problematic manner.
What is Measure 2.9?
Measure 2.9 of the second draft of the GPAI Code of Practice states that “Signatories that train a generative general-purpose AI model that will allow for the flexible generation of content, such as in the form of text, audio, images or video, commit to making best efforts to prevent an overfitting of their general-purpose AI model in order to mitigate the risk that a downstream AI system, into which the general-purpose AI model is integrated, generates copyright infringing output that is identical or recognizably similar to protected works used in the training stage.”
Aside from the fact that overfitting is not a crystal clear concept per se and can be justified in some cases, this measure could be understood as requiring some form of copyright “output filter” to be embedded in GPAI models.
From Upload Filters to Output Filters: The Risk of Preemptive Censorship
The upload filters mandated by Article 17 CDSMD seek to address copyright infringement in the context of digital platforms, where users publicly share content. The primary concern is to ensure that copyrighted material is not distributed without authorization while safeguarding legitimate uses like parody, critique, or education. Thanks to significant advocacy from civil society, Article 17(7) CDSMD introduced an additional obligation to ensure lawful content remains available. Importantly, upload filters operate in a public context, where the intended use of content can be partially assessed based on its visibility.
Generative AI, particularly in chatbot scenarios, operates in a fundamentally different way. Outputs are typically generated on a one-to-one basis, visible only to the individual who requested them. If the user later decides to share this content publicly, existing copyright rules—including the takedown mechanisms under Article 3 of the InfoSoc Directive and Article 17 of the CDSMD—already apply.
Compared to upload filters, output filters present new risks to fundamental rights because they cannot address the core issue: the potential disconnect between the legality of the original works used in training and the nature of the outputs generated. Moreover, copyright law allows for exceptions like parody, pastiche, or critique, which require an understanding of the intent and context of the use. Generative AI systems, however, lack the contextual awareness needed to make these determinations. As a result, output filters risk preemptively blocking lawful content, creating a chilling effect on creativity, innovation, and the exploration of ideas.
Moreover, the fundamental nature of AI output differs from the content uploaded to user-generated content (UGC) platforms. On UGC platforms, filtering mechanisms prevent potentially infringing content from being made publicly available. In contrast, generative AI outputs are generally private by default, and infringement only arises if a user decides to share the content in a public context. Unless the generative AI system itself publishes the content automatically—a scenario that is rarely the case—there is no public availability. At most, the issue is limited to a private infringing reproduction, making the justification for preemptive filtering even weaker.
The Risks to Freedom of Thought and Expression
The potential implementation of output filters poses significant risks to freedom of thought and expression, as well as freedom of the arts. Generative AI tools, particularly chatbots, have become vital for creativity, research, and education, enabling users to explore ideas and generate content privately. Imposing output filters would constrain these tools' ability to fulfill their potential, effectively policing private thought experiments and creative exploration.
As free expression advocates Jacob Mchangama and Jordi Calvet-Bademunt argue, generative AI systems are already prone to restrictive content moderation practices that limit their utility. Policies intended to avoid controversy often result in outright refusals to generate content on certain topics, thereby restricting users’ ability to engage with ideas freely. Expanding this model to enforce copyright compliance through output filters would exacerbate these issues, creating a regime of preemptive censorship.
The implications are profound. By recommending mechanisms that block outputs based on speculative copyright concerns, the Code of Practice risks turning generative AI into a gatekeeper of ideas. This approach directly undermines the EU’s commitment to fostering innovation and safeguarding fundamental rights, stifling creativity and the free exchange of knowledge in the process.
Beyond these risks, there is also the possibility that these filters expand the scope of protection given by copyright to new areas by, for example, prohibiting outputs “in the style of.”
Compliance with Vagues Overfitting Thresholds: The Risk of Overblocking
Output filters present significant challenges regarding technical feasibility and the risk of ‘overblocking’ legitimate uses. Generative AI models synthesize data in ways that cannot easily be reduced to binary determinations of copyright infringement. For example, a text generated by an AI model may bear superficial similarities to a copyrighted work without necessarily constituting infringement, depending on factors such as the level of transformation or the nature of the use. Expecting AI systems to navigate these nuances in real-time is unrealistic. The problem is further exacerbated by the potential applicability of copyright exceptions that may permit the use of otherwise infringing contexts depending on the use. Output filters are, by default, incapable of making this assessment since they are deployed before that use occurs.
The issue of "overfitting" deserves particular clarification. Overfitting is a technical concept describing a model that performs poorly by tailoring its outputs too closely to specific data, limiting generalizability. However, this is not inherently problematic in all contexts. For instance, a company might deliberately train an AI system to output content derived from proprietary technical documentation or customer records, which is lawful and legitimate. The concern is not overfitting itself but whether a model's outputs unlawfully reproduce protected works—a determination complicated by factors such as user prompts, lawful training data, and incidental similarities.
Furthermore, defining "identical or recognizably similar" outputs as inherently infringing introduces new legal thresholds that go beyond the scope of existing copyright frameworks under the EUCD and AI Act. These vague and subjective standards risk creating regulatory uncertainty, particularly when similarities may arise coincidentally or due to downstream user prompts. Without clear and enforceable guidelines, such measures could disproportionately burden developers and users while offering minimal additional protection for copyright holders.
Conclusion
The GPAI Code of Practice represents a critical opportunity for the EU to lead on responsible AI governance. However, the proposed output filters risk undermining this ambition by imposing disproportionate and unworkable measures that threaten freedom of thought and expression.
To avoid the pitfalls of output filters and ensure that the GPAI Code of Practice aligns with the EU’s broader goals of fostering innovation and protecting rights, measure 2.9 should be deleted from the Code. Looking beyond that, policymakers should explicitly reject pre-emptive copyright output filters of AI-generated outputs.
Authors
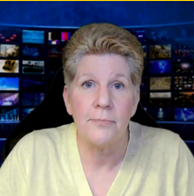