From Crisis to Control: Establishing a Resilient Incident Response Framework for Deployed AI Models
Kevin Neilon / Dec 18, 2024Kevin Neilon works at Rethink Priorities, a global think tank and implementation group.
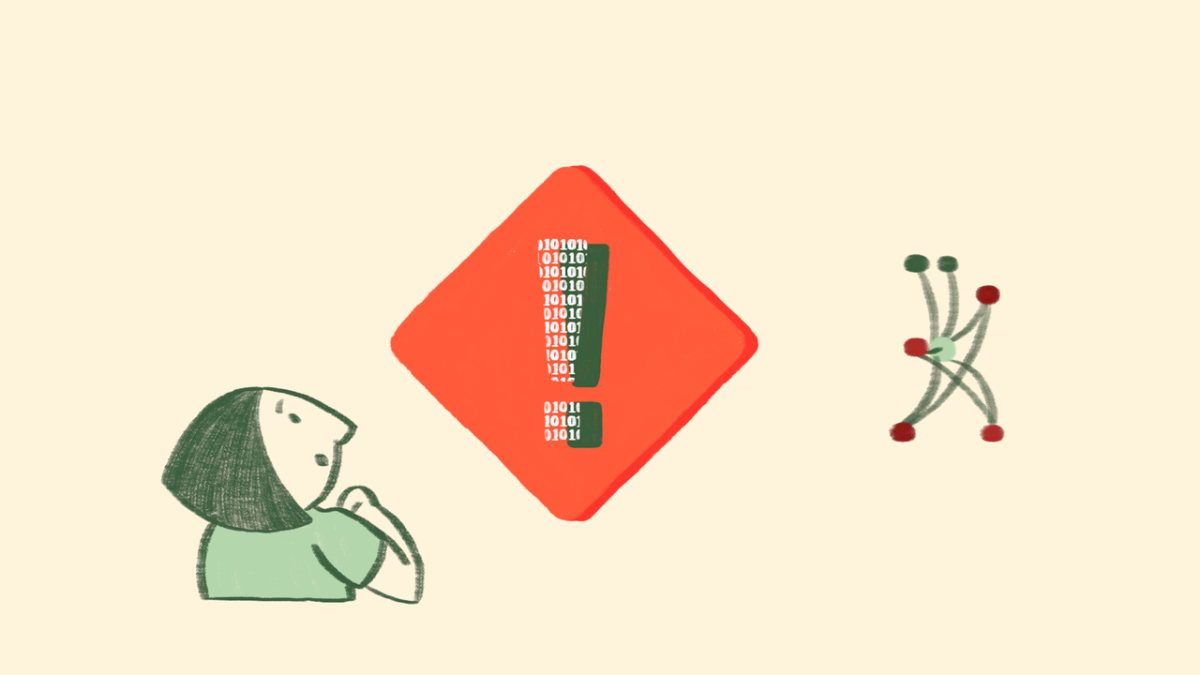
Yasmin Dwiputri & Data Hazards Project / Better Images of AI / Managing Data Hazards / CC-BY 4.
For all the excitement that the benefits of advanced AI models promise, the risks provide an equal measure of terror. Future potential for advancements in biological drugs and improved cybersecurity goes hand-in-hand with the future threat of proliferating bioweapons and creating more complex cyberattacks.
And make no mistake, there are actors determined to explore the most dangerous capabilities of frontier AI models. US adversaries are already using AI to further their agendas. There is evidence that AI Is accelerating Iranian cyber operations, and Chinese researchers have leveraged Meta’s open-source AI model for military use. Other adversaries have almost certainly followed the same thought. Even if frontier AI companies successfully secure their models and model weights, we can assume that bad actors will continue to test the limits of harm they can create with publicly accessible, or ‘deployed’ models.
To date, there are many examples of deployed, closed-source models being jailbroken, engaging in deceptive scheming, or otherwise producing unintended results. New capabilities have been elicited from deployed models via scaffolding, prompt engineering, unexpected new uses, and interactions with other models and systems. All this despite the careful pre-deployment training and model weight security at frontier AI companies. These incidents have been relatively harmless, as even today’s most capable models do not seem capable of significantly increasing the likelihood or severity of catastrophic risks.
However, these incidents provide a precedent that pre-deployment evaluations may significantly underestimate the upper bound of AI model capabilities. And unexpected outputs from much more capable future models could have dire consequences. As a result, preparing for severe post-deployment incidents is critical to a robust ‘defense in depth’ strategy for mitigating the risks of advanced AI models. Given the time required to implement robust incident response policies, it’s increasingly urgent that AI companies and policymakers prepare for such scenarios now.
A Framework for Post-Deployment Incident Response
An effective incident response framework for frontier AI companies should be comprehensive and adaptive, allowing quick and decisive responses to emerging threats. Researchers at the Institute for AI Policy and Strategy (IAPS) have proposed a post-deployment response framework, along with a toolkit of specific incident responses. The proposed framework consists of four stages: prepare, monitor and analyze, execute, and recovery and follow up.
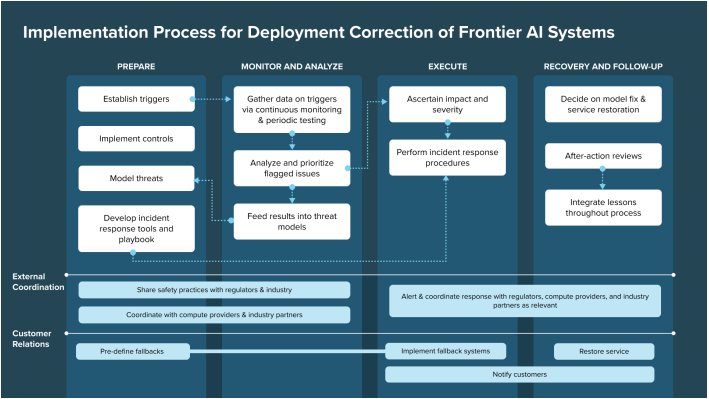
Source: The Institute for AI Policy and Strategy
In the preparation phase, the goal is proactive risk management. This phase involves conducting in-depth threat modeling analyses to identify potential risks associated with the AI model's deployment. Following the threat modeling, teams establish specific ‘triggers,’ which are specific conditions or events that signal a potential incident, such as unusual usage patterns or external reports of misuse. Developing a detailed incident response playbook provides team members with clear guidance on their roles, responsibilities, and communication channels in various scenarios where triggers are activated.
The next stage of the framework, monitoring and analysis, requires continuous monitoring of the deployed model to detect incidents early. This monitoring involves gathering data from the model and external sources, such as user feedback and performance analytics. Real-time monitoring dashboards can help identify unusual patterns quickly and enable immediate action. Since not all triggers warrant the same level of response, teams should categorize incidents by potential impact and urgency. This prioritization allows them to focus resources on the most critical threats first.
Once an incident is detected, the response execution phase is crucial to mitigating damage. In this stage, internal analysis is used to understand the severity of the incident and inform the best approach for mitigation and containment. Teams move to execute the incident response playbook and adjust as necessary based on real-time developments. Actions should consider addressing the primary issue's root cause and implementing any contingency plans for backup service. In severe incidents, this phase often requires coordination with regulatory agencies, such as the Cybersecurity and Infrastructure Security Agency (CISA), and industry partners to align on containment strategies.
Following an incident, the recovery phase focuses on restoring normal operations and capturing lessons from the event. This phase involves fixing the underlying issues and revalidating the model's functionality. The fix might include retraining the model or updating safety protocols as needed. Detailed documentation of each incident is crucial for compliance purposes and supporting a continuous improvement culture.
A Toolkit for Responding to Post-Deployment Incidents
Developers have a variety of actions available to them to contain and mitigate the harms of incidents caused by advanced AI models. These tools offer a variety of response mechanisms that can be executed individually or in combination with one another, allowing developers to tailor specific responses based on the incident's scope and severity.
- User-Based Restrictions: One of the fastest ways to manage active incidents is by blocking specific users or groups suspected of abuse. This can involve identifying and preventing malicious actors from accessing the model or strictly limiting access to only “allow-listed” users, such as pre-vetted employees, regulators, and evaluators.
- Access Frequency Limits: By limiting the rate at which users can access or query the model, response teams can mitigate risks associated with high-volume misuse, such as distributed denial-of-service (DDoS) attacks or large-scale phishing attempts. This restriction can buy valuable time while further analysis and response actions are planned.
- Capability or Feature Restrictions: For some models, selectively disabling certain capabilities or features can prevent misuse. For example, a model’s ability to autonomously generate content on certain sensitive topics could be curtailed temporarily, reducing risk without a full system shutdown.
- Use Case Restrictions: In cases where an incident is high-stakes but non-immediate, the model’s allowed applications can be restricted. For example, models in critical infrastructure applications may be limited temporarily.
- Model Shutdown: As a last resort, full market removal or even deletion of the model’s components might be necessary to contain the incident or avoid future recurrence. This may apply to models involved in major breaches or other high-impact incidents where the risk cannot be managed through less drastic interventions.
While these tools have the potential to mitigate and contain severe incidents created or assisted by AI, they will be most effective only with a clear plan in place for when and how to implement them, along with clear owners and decision-makers within this process. It’s also important to note that the ability to use these tools is minimal or non-existent for open-source models with publicly available model weights. So, there are far fewer options to respond to incidents resulting from open-source models.
To illustrate using these tools in practice, consider a hypothetical scenario in which an adversarial cyber group uses a frontier AI model to assist in a cyberattack. These attacks might include DDoS tactics, phishing schemes, or manipulating industrial control systems, putting essential services at risk. In this scenario, the response would begin with immediate coordination between the AI developer, relevant government entities such as CISA, and industry partners.
If malicious actors could be identified, the developer might use user restrictions to prevent their access. In cases where specific users cannot be easily identified, the developer might shift to only allowing access for pre-approved, vetted users. Additional containment measures, such as implementing access frequency limits and restricting high-risk cyber actions, could also mitigate the attack’s scope. For example, restricting autonomous model capabilities may prevent the model from being leveraged in targeted phishing schemes or similar malicious activities.
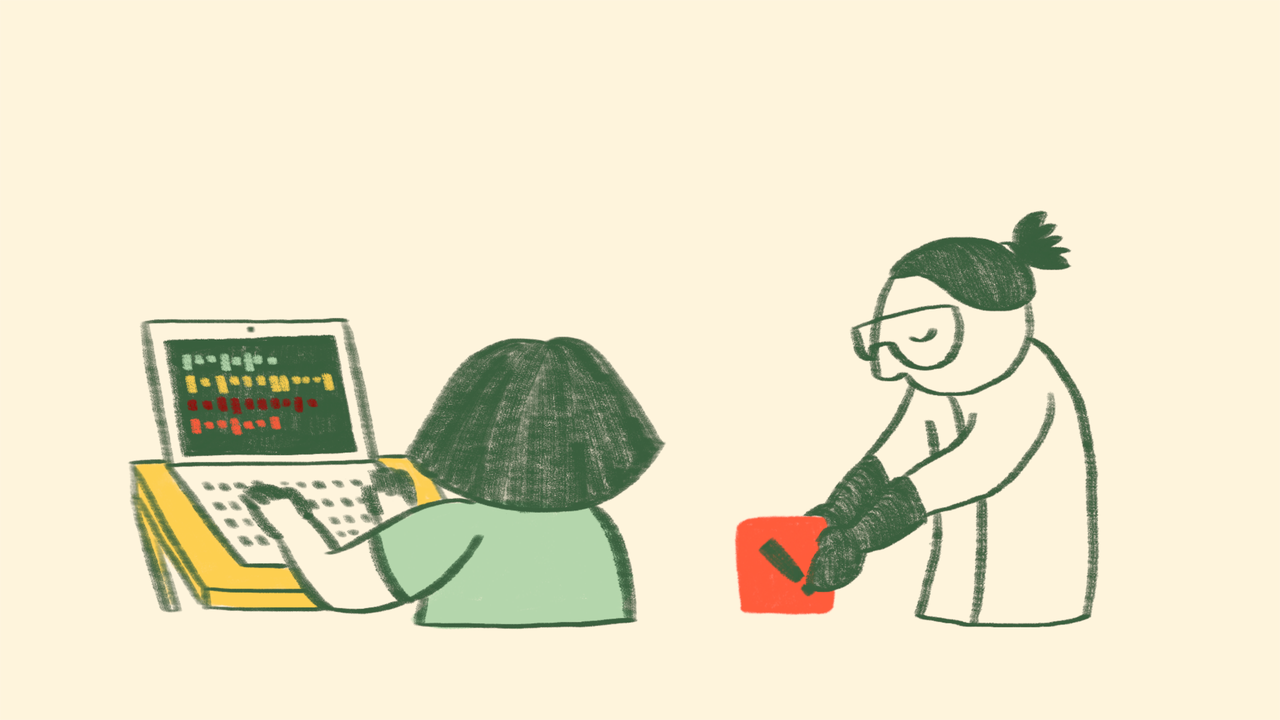
Yasmin Dwiputri & Data Hazards Project / Better Images of AI / Safety Precautions / CC-BY 4.0
Existing AI Company and Government Policies Do Not Sufficiently Address Post-Deployment Incident Response
Frontier AI companies have recently provided more transparency to their internal policies regarding safety, including Responsible Scaling Policies (RSPs) published by Anthropic, Google DeepMind, and OpenAI. When it comes to responding to post-deployment incidents, all three RSPs lack clear, detailed, and actionable plans for responding to post-deployment incidents. SaferAI, an organization that rates frontier AI companies’ risk management practices, rates all three leading AI companies as ‘weak’ on the risk mitigation metrics.
Anthropic’s RSP commits to “develop internal safety procedures for incident scenarios,” including “responding to severe jailbreaks or vulnerabilities in deployed models.” However, there are no further details or independent enforcement mechanisms. Both Anthropic and Google DeepMind emphasize securing model weights, which is critical to preserving the use of mitigation and containment tools, though the lack of specific commitments is disappointing.
Next, consider the US Cyber Incident Reporting for Critical Infrastructure Act (CIRCIA), which has some of the most direct and enforceable provisions governing how AI companies respond to AI incidents. CIRCIA requires critical infrastructure operators to report substantial cyber incidents and ransomware payments to CISA within specific timeframes – typically within 24-72 hours.
If an AI company were aware that its model was involved in a cyberattack impacting critical infrastructure, the company would be required to report the incident to CISA and collaborate to mitigate the broader risks its models pose. Importantly, this legislation focuses on reporting requirements rather than mandating a comprehensive incident response plan. AI companies need to instead refer to non-binding guidelines like NIST AI 800-1 ‘Managing Misuse Risk for Dual-Use Foundation Models’ for guidance.
Conclusion
As AI capabilities advance, a resilient post-deployment incident response framework becomes increasingly crucial for mitigating the risks of powerful AI. By proactively detailing incident response measures, teams can be better prepared to contain and manage AI incidents effectively. This approach contributes to a robust ‘defense in depth’ strategy to support the responsible growth of AI applications in diverse environments.
There are specific actions frontier AI companies and policymakers can take now to promote effective post-deployment incident responses. Frontier AI companies should implement strict controls over model access and weights to preserve the use of mitigation and containment tools. Additionally, AI companies should establish and adequately support appropriate roles, responsibilities, and communication channels related to post-deployment incident response, built on the principles of planning, monitoring, execution, and recovery. Government policymakers, standard-setting organizations, and AI companies should collaborate on implementing incident response plans, threat modeling, and information sharing to enhance the effectiveness and speed of responding to severe incidents.
Related Reading
Authors
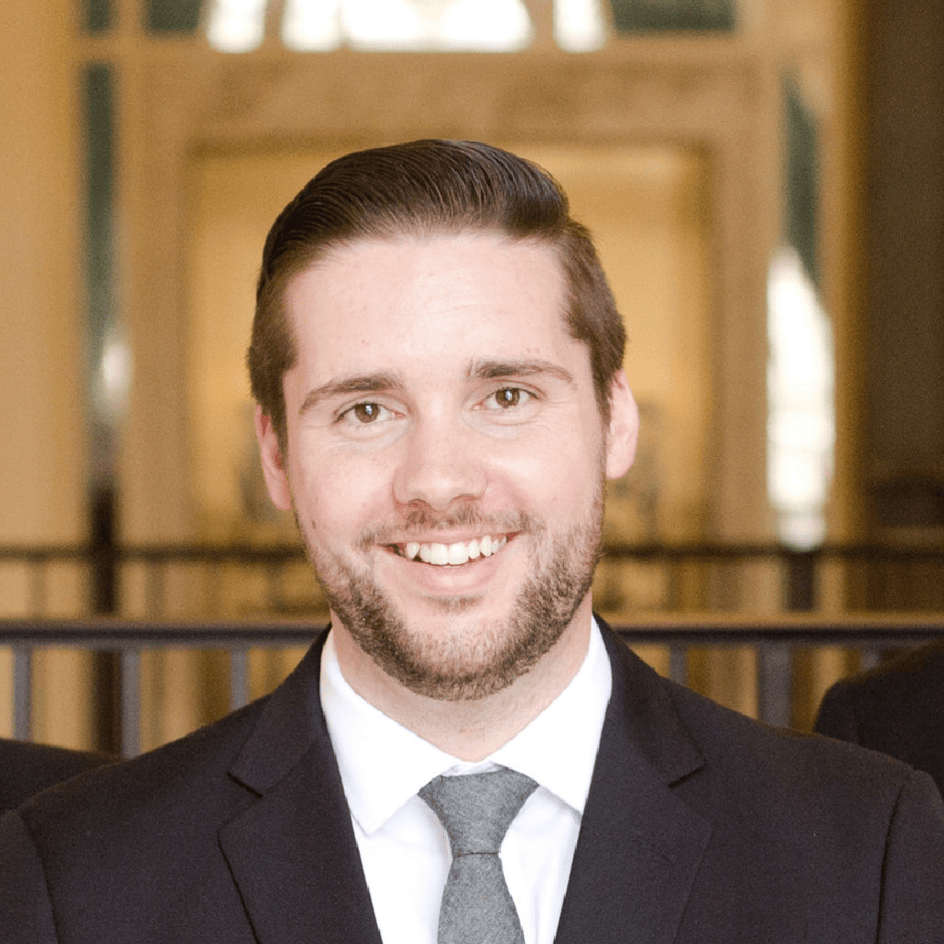