Can We Red Team Our Way to AI Accountability?
Ranjit Singh, Borhane Blili-Hamelin, Jacob Metcalf / Aug 18, 2023Ranjit Singh is a qualitative researcher with the Algorithmic Impact Methods Lab at Data & Society; Borhane Blili-Hamelin is the taxonomy lead at the AI Vulnerability Database, a community partner organization for the Generative Red Team (GRT) challenge at DEF CON; and Jacob Metcalf is program director, AI on the Ground, at Data & Society.
Last week’s much publicized Generative Red Team (GRT) Challenge at Caeasar’s Forum in Las Vegas, held during the annual DEF CON computer security convention, underscored the enthusiasm for red-teaming as a strategy for mitigating generative AI harms. Red-teaming LLMs often involves prompting AI models to produce the kind of content they’re not supposed to — that might, for example, be offensive, dangerous, deceptive, or just uncomfortably weird. The GRT Challenge offered a set of categories of harmful content that models such as OpenAI’s GPT4, Google’s Bard, or Anthropic’s Claude may output, from disclosing sensitive information such as credit card numbers to biased statements against particular communities. This included “jailbreaking” — intentional efforts to get the model to misbehave — as well as attempts, through benign prompts, to model the normal activities of everyday internet users who might stumble into harm. Volunteer players were evaluated on their prompts that made the models misbehave.
Co-organized by the DEF CON-affiliated AI Village and the AI accountability nonprofits Humane Intelligence and SeedAI, the challenge was “aligned with the goals of the Biden-Harris Blueprint for an AI Bill of Rights and the NIST AI Risk Management Framework,” and involved the participation of the National Science Foundation’s Computer and Information Science and Engineering (CISE) Directorate, the White House Office of Science and Technology Policy (OSTP), and the Congressional AI Caucus. Over the course of two and half days, an unlikely gathering of college students, community advocates, cybersecurity professionals, policymakers, and AI model vendors played a Jeopardy-style capture the flag competition as an educational on-ramp for the public to experience red-teaming and gain experience in the skills needed to prevent generative AI harms.
The event was a success in at least one sense: it moved red-teaming from the realm of focused attacks by professionals within a company to amateur and crowdsourced attempts at modeling the variable behavior of the public on the web. Yet the hype around red-teaming risks ignoring ongoing debates about it as a method, as well as discussion of other crucial protections that must be in place to ensure AI safety and accountability. And only time will tell whether the event produced data that is useful for substantive AI accountability efforts.
At the event, nearly every conversation among the experts on stage and in sidebars in the hallways concerned the ambiguous nature of red-teaming for AI: what does it include, and how should it be done to mitigate harms? One conference presentation from a tech company with relatively mature AI red-teaming practices emphasized that its methods drew imperfectly on many other tech governance models with divergent purposes. During the closing remarks for the competition, there was a broad discussion on how this exercise shouldn't even be called “red-teaming,” reflecting rapidly evolving terminology. This confusion isn’t merely semantic, it’s also about method — what is the goal everyone can agree on, and how will they all get there?
Perhaps the most significant success of the event is that it offered individuals an opportunity to participate in an activity that has become a central pillar of national strategy to make AI products safe and trustworthy. Tech companies are promoting red-teaming as core to AI safety, and it was featured in the White House’s recent agreement with seven leading AI companies, given the pride of place as the very first accountability process mentioned. Indeed, many more people should participate in the development of the governance of AI systems that affect our lives, and it might as well be enjoyable and rewarding to do so. (A fun example that anyone can try is Lakera’s “Gandalf” game, which challenges players to argue their way past a LLM acting as a wizard guarding a password.)
However, red-teaming is at best only part of the puzzle of the work of AI accountability laid out in the White House’s Blueprint for an AI Bill of Rights: the obligation to build safe and effective systems. The Blueprint has four more principles that red-teaming, as a technical exercise, cannot satisfy: algorithmic discrimination protections; data privacy; notice and explanation; and human alternatives, considerations, and fall back.
Red-teaming is not a plan; it finds flaws with the goal of improving existing plans, infrastructures, and practices. So the question is not whether red-teaming is effective or worthwhile as an AI accountability mechanism. The real question is about its place in the emerging ecosystem of practices for mapping, measuring, disclosing, and mitigating AI harms, ranging from impact assessments and audits to participatory governance measures and incident reporting. As tech companies seek to embed AI across society — capturing sensitive personal data, gatekeeping access to jobs, housing, and health care — red-teaming alone will not address the fundamental risks that AI presents to people and communities. It won’t protect people’s civil rights. It won’t protect workers’ jobs and their right to organize. It won’t address the problem of models built on data embedded in and with historical and social inequalities. AI policy should foreground democratic public participation, involving diverse publics; tech companies and policymakers should involve them throughout all stages of AI development, including asking whether a technology is needed or desirable in the first place. The GRT challenge was instrumental in building public awareness around these issues. Yet fundamental questions about operationalizing AI accountability remain.
Red-teaming can only support AI accountability if tech companies and the federal government have the other pieces in place: the laws, regulations, and enforcement to ensure protection from harm. It is a step in the right direction, but the White House and Congress have a long way to go in supporting these fundamental (if inherently unflashy) needs.
Authors
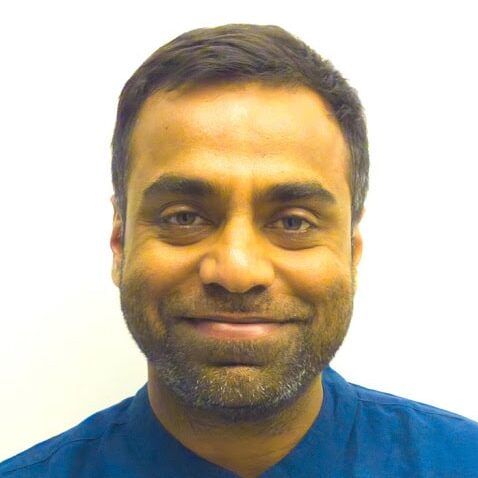
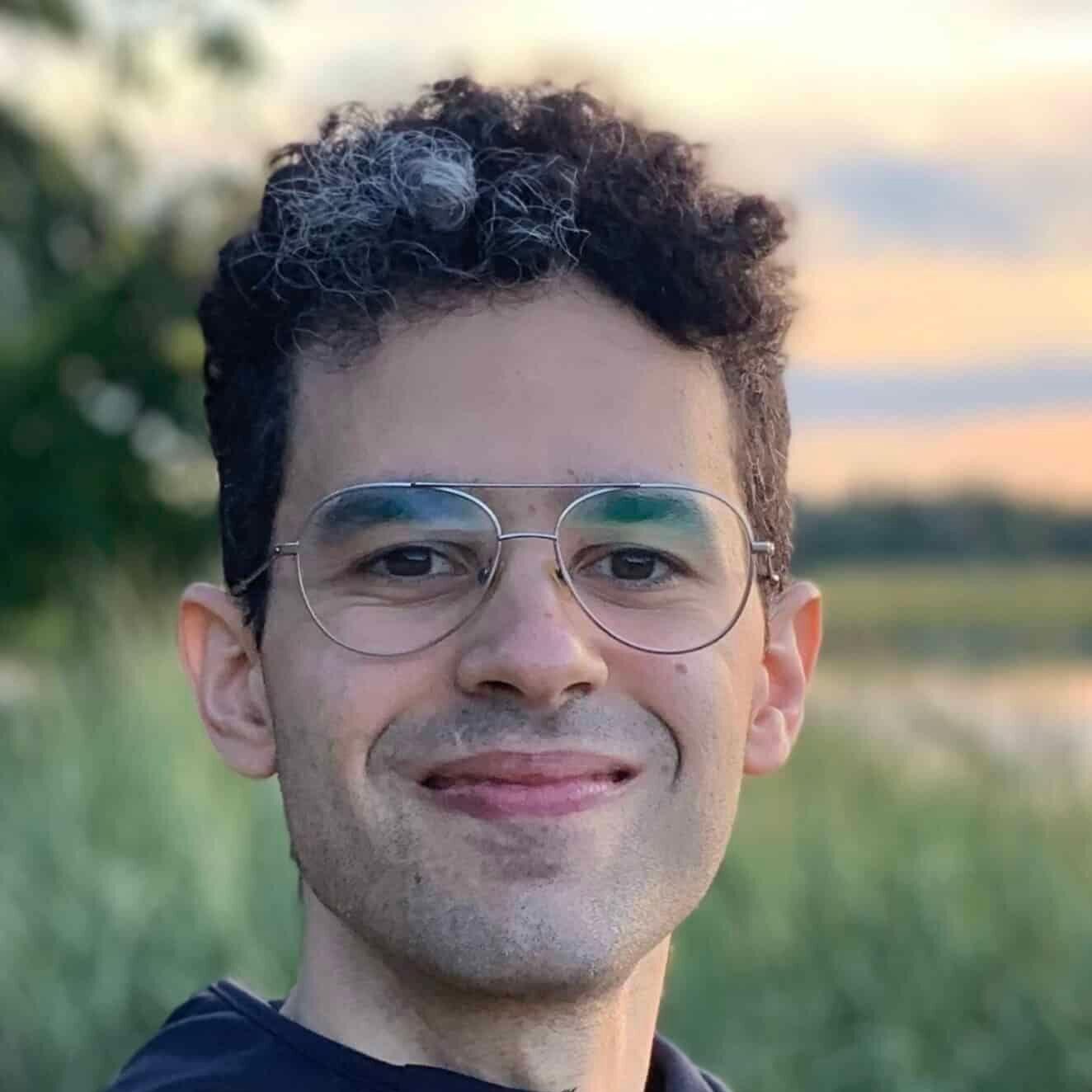
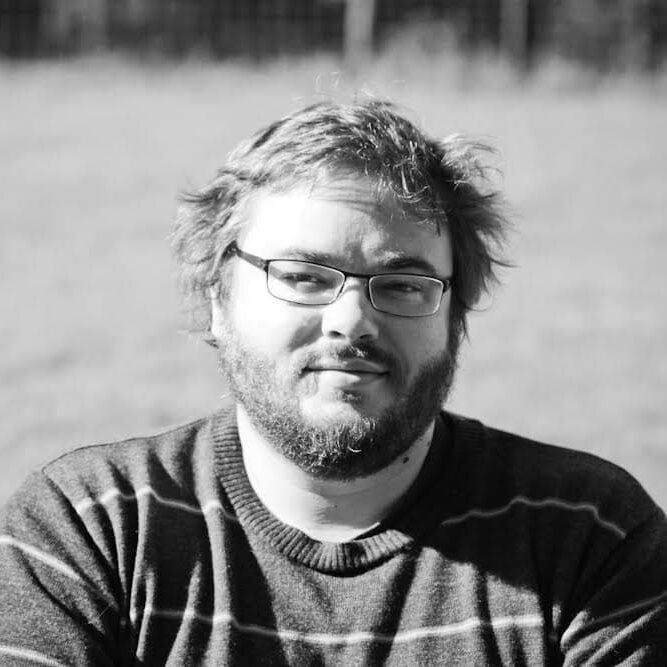