DeepSeek Prompts a Rethink
Justin Hendrix / Jan 28, 2025Audio of this conversation is available via your favorite podcast service.
If Chinese AI startup DeepSeek’s efficiency and performance achievements stand up to scrutiny, it could have big implications for the AI race. It could call into question the strategic approach that the biggest US firms appear to be taking and the wisdom of the current American policy approach to AI.
To discuss these issues, I spoke to Karen Hao, a reporter who covers AI. In recent years, she's reported on China and tech for the Wall Street Journal, written about AI for The Atlantic, and run a program for the Pulitzer Center to teach other journalists how to report on AI. Hao has a book about OpenAI, the AI industry, and its global impacts that will be released later this year.
What follows is a lightly edited transcript of the discussion.
Media montage:
Let's talk about DeepSeek because it is mind-blowing and it is shaking this entire industry to its core.
Global tech investors could face a one-trillion-dollar wipe out after the news of Chinese artificial intelligence startup DeepSeek releasing an open-source AI model at just a fraction of the cost of its competitors. The company's models appear to rival those from OpenAI, Google, and Meta despite the U.S. government's effort to limit China's access to cutting-edge AI technology.
And the entire AI industry in the United States and around the world has been thinking about high-level computation and big data centers and a lot of energy use and chips. And now they're thinking, eh maybe not.
Justin Hendrix:
It's rare that new research results spark a stock sell-off, but that's what happened on Monday after DeepSeek, a startup owned by a Chinese hedge fund called High Flyer, published claims that it trained its new model called DeepSeek-V3 using only a fraction of the financial resources that American firms like Meta, OpenAI, and Google use to train models of comparable performance. And without access to the latest high-end chips, which are restricted under U.S. export controls.
Not all experts agree that DeepSeek's claims hold up. For instance, Scale AI founder and CEO Alexandr Wang claimed that DeepSeek has access to many more high-end Nvidia chips than it can disclose. And others have suggested that it's using fuzzy math to compute the cost to train its model. But one computer scientist I follow, Columbia University's Vishal Misra, wrote that, "The DeepSeek-R1 paper represents an interesting technical breakthrough that aligns with where many of us believe AI development needs to go, away from brute force approaches toward more targeted efficient architectures."
If DeepSeek's achievement stands up to scrutiny, it could have big implications for the AI race. It calls into question some of the basic elements of the story that big AI companies have been telling over the last few years. To talk about the potential implications, I spoke to Karen Hao, a journalist who has joined the podcast on occasion in the past to discuss her reporting on artificial intelligence.
Karen Hao:
My name is Karen Hao. I am a reporter who covers artificial intelligence. I've been doing that for around seven years, and I have a forthcoming book about OpenAI, the AI industry, and its global impacts coming out later this year.
Justin Hendrix:
Karen, before we get started with the topic du jour, I must also emphasize you not only are a journalist covering AI, you teach other journalists to cover AI.
Karen Hao:
Yes. I run a program with the Pulitzer Center called the AI Spotlight Series. And we basically on free workshops and webinars, both virtual and at in-person conferences all around the world to teach other journalists on how to report on this crazy story. Because we certainly need far more capacity in the media industry to really tackle this topic.
Justin Hendrix:
I'm very grateful you've taken a moment away from your vacation to speak to me about this story that has been playing out across the day. Bloomberg says, "AI-fueled stock rally dealt trillion-dollar blow by Chinese upstart." The Wall Street Journal says, "Technology stocks tumbled Monday on news that China's DeepSeek could train to sophisticated artificial intelligence model at a fraction of the cost of its Silicon Valley rivals triggering a sudden reversal of the recent AI rally." And The New York Times says, "DeepSeek's release is a challenge to tech industry consensus that in order to build bigger and better AI systems companies would have to spend billions and billions of dollars on new data centers." What do you make of the DeepSeek story as someone who's covered AI and as someone who's covered China?
Karen Hao:
I think from a technical perspective of just what they did, it's not a surprise that they were able to pull off training a model with far fewer resources. I think if you have been following the technical aspects of AI development for a while, there is no scientific reason why they wouldn't have been able to do that. And it was sort of like a matter of time before someone somewhere came up with some kind of innovation to significantly reduce the amount of resources that were being used to train AI models. The fun surprise is that it's a Chinese company that is doing it right now, but we can get into that more in a bit.
But I have felt like covering this beat for a while, that there has been a really remarkable collapse in the kind of imagination of what AI development should look like within the AI field. In the past, before ChatGPT, before large language models, before transformers, there was a lot more investment into designing different types of neural networks, designing different types of algorithms to try and just improve the efficiency of AI models towards different types of tasks.
And basically what's happened in the last two, three, maybe four years is that there's just been a glomming on of everyone to just a singular approach. Which represents a teeny tiny slice of the pie of all approaches to AI development. Which is specifically, I'm going to take a transformer neural network. I'm going to pump extraordinary amounts of data into it, scraped from the internet and taken from textbooks and news articles and fiction books. And then I'm just going to keep scaling that, relentlessly exploit that approach and scale it. And get bigger and bigger and bigger data centers to do that.
I think the word choice that the New York Times has that it is challenging consensus is a really good one. There was basically just a dogma that developed within the AI field, within the AI industry that everyone should just do this approach. Not because of any kind of scientific truth, simply because it was in the short term, reaping what seemed like a lot of great commercial potential for companies.
Yeah, so it was only a matter of time before essentially someone reminded the entire field of AI that actually there used to be other ways of getting advancement out of these models that was not just throwing more chips at it. That forgotten path is actually one that really, really needs to be invested in right now. Because the sheer scale of the data centers that are being built and the environmental impacts they have, the impacts they have on communities is not actually something we should really be accepting.
Justin Hendrix:
One of the people that I follow in these matters is a fellow called Vishal Misra, who's now Vice Dean and Professor of Computer Science at Columbia University. He had a short post today talking about DeepSeek, about the paper, about the technical breakthrough that it represents. I think he mirrored some of the things that you're saying. Also talks a little bit about the contribution around model reasoning, the ability of this model to essentially discover step-by-step reasoning on its own, naturally without supervised training. He points to other things to admire. So, it seems like there's a real engineering contribution here.
Karen Hao:
I'm always a little bit cautious in just using the term reasoning. I know it's become sort of the term of art within the industry to talk about these. But there's still technically a lot of scientific debate around whether or not deep learning models can be capable of reasoning. Certainly, there is some kind of step-by-step process that they are sometimes able to go through to achieve the correct answer. But yeah, I wouldn't be surprised if there were additional innovations in addition to efficiency. Because DeepSeek, they fundamentally rethought a lot of aspects of AI model development and training something that ultimately cost only $6 million. When the state of the art in the U.S. by companies like OpenAI, Anthropic is $10 billion reaching higher than that.
I think just by virtue of not having that approach available to them as a Chinese company due to export controls, they did have to just tinker around with a lot of other different paths for getting this kind of result. And it doesn't surprise me that the kind of outcome of that process was potentially multiple different types of improvements and the model just working slightly differently.
Justin Hendrix:
So I don't want to get carried away with this, but you point out that a lot of folks are looking at this through the lens of U.S.-China tech competition. And that to some extent that may miss the bigger story about what this means generally about AI development going forward. But let's pause for a moment on U.S.-China competition.
It's almost like this thing's kind of inverted the way we think about these things, right? You've got what looked like the kind of character of this moment, these big bloated U.S. AI firms, all this capital, all this computing resource lumbering along with this expensive resource intensive approach. And now you've got this upstart, this resource constrained Chinese firm that comes up with something better, cheaper, faster. This is not the way most American tech folks like to think about Silicon Valley versus China. Do you think that is as much of a sea change as perhaps some of these headlines are suggesting?
Karen Hao:
That's a good question. I mean, it's probably not as dramatic of a sea change as maybe people might automatically think. But I do think that there is a reason why we've ended up at this point that I think will continue to bear out over time. Which is first of all, there has been a remarkable upskilling of tech workers in China too. There's been a lot more investments in AI education. There are a lot of AI PhD students that are graduating from top universities in China and working at Chinese companies.
I think in the past, there was more brain drain that happened where the best and the brightest were first trying to get their PhDs in the U.S. And if not, would get their PhD in China and then try to work at a U.S. company. And for many of these PhD students, it is actually still the dream to work at a U.S. company. But what has happened is because of the pandemic, because of just immigration policies being just more adverse to Chinese talent moving to the U.S., there's been a buildup of really talented Chinese AI researchers in the Chinese tech ecosystem. So that's one input, is the talent part of the equation.
And then the other aspect is having lots of capital is actually a double-edged sword when it comes to innovation. I went to MIT for undergrad, and we would always say in engineering context that necessity is the mother of all innovation. If you do not have to do something and there's an easy path or a hard path, of course you're going to take the easy path. And scaling has been that easy path for U.S. companies with a glut of capital. But because of export controls, which has severely limited the Chinese firms from being able to take that same path, there is no easy path open to them. So, the only path they can take is the hard path. And we were already seeing lots of signs of Chinese researchers testing out interesting novel ways of milking more compute out of handicapped chips and more mileage out of handicapped chips, right as export controls first landed.
I wrote a story at the Wall Street Journal in May of 2023 about some of the papers that I was seeing back then from Chinese researchers that was... they were pooling different types of chips, which you never see in a US. US. companies will wait for the next Nvidia chip to drop and then bulk buy them so that they can train a model on all H100s after A100s. Or all B100s, which the next generation after H100s. And Chinese firms don't have that luxury, so they were cobbling together A100s with A800s with H800s and just a mishmash of stuff and U.S. chips and Chinese chips. And they were already doing a lot of stuff on trying to also figure out how to train across different locations. Because a lot of these chips are very geographically distributed, dispersed. So they were trying to pull resources across different universities or different labs that had their own pockets of GPUs.
All of those things are now, companies are just beginning to think about this because they have just reached the ceiling of compute that they need to start getting more creative. So, China's had a two-year head start on this level of creativity. And so, I think the trend lines are that now there's more talent, there's also been more creativity, more innovation. And I think DeepSeek is going to inspire a lot more Chinese terms to also try to basically do more along the lines of what it did. Which is to just flip the conventional wisdom of the AI industry on its head.
There was also this really interesting article that I was just reading from a friend of mine, JS Tan, who he wrote about how DeepSeek is also organizationally a little bit different from the average Chinese tech company in that it is actually quite flat in structure as opposed to hierarchical. Which most Chinese tech companies kind of suffer a little bit from the hierarchical model. Which then makes it more difficult for people on the bottom run to innovate.
DeepSeek kind of took an approach where they hire a lot of new grads out of the best universities. They kept their team roughly around 150 people, so relatively smaller. And there is no hierarchy, in the official sense. I'm sure there's probably emergent hierarchy. But essentially the founder was really trying to empower anyone within the organization to come up with interesting ideas and run with them. So, there is also a little bit of this firm is actually adopting some more Silicon Valley style organization within the company itself to try and stimulate more innovation on the whole.
So yeah, so I think all of these things, we're going to start seeing more cases of this. But whether or not suddenly everything's going to flip to now all leading models are going to be from Chinese companies, I don't think that's going to happen. But yeah, we are certainly seeing an important moment here.
Justin Hendrix:
I'm sure that the battle is far from over as to who will create the best AI technologies. But I mean, it does sound like what you're describing, these Silicon Valley companies right now, are pushing all their chips to the center of the table at the beginning of the Trump Administration saying, "We've got to put hundreds of billions into data centers. We need all the capital in the world to make bigger and bigger models." And they're essentially getting beat out by a Chinese firm, which has taken the lean approach. It's almost like they've been out Silicon Valleyed.
Karen Hao:
Yeah, really. It really does feel that way. And it is highly ironic because I mean, Sam Altman was the one that famously always said, "Have a much easier time beating incumbents because incumbents get entrenched in their old ways. And startups can take risks than incumbents just cannot take." So literally, I mean, that is the playbook that DeepSeek has executed really well.
Justin Hendrix:
I wonder if this doesn't call into question so much of the political argument that's being made in Silicon Valley right now about the need to stave off regulation. This could be a strong sign that in fact preserving competition, keeping things a little leaner, trimmer might have perhaps led to a slightly different place than where we're at the moment. Where it seems like the only answer is, you know, you get SoftBank to come along and pummel several hundred billion dollars to go and build out your big data centers. And you pointed out on LinkedIn, almost the irony that this has happened that literal week after the big Stargate announcement at the White House.
Karen Hao:
It's just super funny that, one, the Stargate announcement was very emblematic of the US approach thus far, that OpenAI has introduced and championed and led of the just scale aggressively and continue funneling more chips into these models. And then DeepSeek is very emblematic of kind of why we should not be doing that. And one of the things about SoftBank that's super interesting as well, kind of going back to this idea of necessity is the mother of all innovation, I used to work at a startup in Silicon Valley as well. And we used to call a SoftBank investment the kiss of death because the amount of capital that would flood into a company, a startup with sort of half-baked technology and half-baked business strategy would suddenly suffer a killing of all innovation and a killing of all business acumen because they didn't need to anymore. You could just float on SoftBank money until essentially the whole thing tumbled down. But you could float for a pretty long time. And the existential drive to actually shore up weaknesses both technically and commercially just went away.
It's kind of funny that it is literally SoftBank now that is also pumping $500 billion, an insane amount of money, into this approach that is now being shown as fatally flawed. But also even before SoftBank kind of joined forces with OpenAI, one of the things that kind of made OpenAI what it is today was the fact that it is helmed by Sam Altman. And Sam Altman, one of his greatest, singular defining skills is his ability to raise enormous amounts of capital.
And so, I think we're kind of just seeing the facade crack a little bit with DeepSeek where it's like, "Oh, wait a minute. You didn't actually need to raise that much capital. And actually, raising that much capital and now having an even more giant infusion of SoftBank capital might actually be the thing that is hampering you, rather than helping you." In the short term, looks great, but in the long run is probably creating some really bad habits that are now part of the business's debt that it's going to need to shore up.
Justin Hendrix:
So, I don't want to force you too much into considering the policy implications of this moment. I mean, there's a possibility, of course, that we just double down and continue as is in this race against the Chinese. Take this perspective that this is the Sputnik moment, we've got to compete, etc, etc. But if you were a policymaker, were perhaps asking questions on Capitol Hill, what would be the type of questions you'd want to ask this industry, this American industry in the wake of DeepSeek?
Karen Hao:
Asking them, how much have you been investing in efficiency measures? What kinds of things are you doing right now that now that we've seen that we can actually significantly reduce the computational cost of this, do you have anything that you've been working on? I mean, they do. The answer is they do. But anything that rises to that level of efficiency cut optimizations? And if not, why? And what is your plan moving forward to actually invest more in efficiency gains?
And also, I guess not questions that I would ask, but things that I would really start focusing on is blanket statement. Obviously regulation is actually sometimes an accelerator, and I don't think that the U.S. has remembered that in recent years. Now, example number one of the fact that regulation can actually help accelerate businesses in terms of establishing their long-term sustainability. So, we should be thinking about regulation in all of the different kind of dimensions or layers of the AI development stack that people have already been advocating for.
We should be regulating data, we should have some kind of data privacy law. There should be more data transparency in what kind of data is actually being fed into the models. All of which are regulations that keep getting taken off the table because of this fear of over-regulating the companies.
And we should also be looking at regulating the size of these data centers, the energy source of these data centers. A lot of these companies, when they build their data centers, they also enter communities under shell companies because they don't want to deal with community pushback. Like, why do we even allow this practice? That should be an easily-regulatable thing where any company that's building data centers should disclose it to the public, disclose it to the community that they're going to break grounds in. And give an opportunity for the community to actually say that they don't want it. If they don't feel like they have the resources or they're going to reap the economic benefits, or they don't want to take that trade-off for whatever reason, give them a voice to actually express that.
We should be regulating the water intensity of these data centers. Some of OpenAI's largest, most intensive data centers right now are built in the Arizona desert, and are using Colorado River water to cool. And the Colorado River is literally diminishing because of overdrafting.
So, all of these dimensions of regulation that US policymakers were just too anxious to step into, they should be rushing into now. Because basically the argument toward that regulation that Silicon Valley has always used, which is, what about China, has clearly actually had the reverse effect. And yeah, I hope that this is a major lesson for U.S. policymakers that actually the, what about China, is actually just a rhetorical tool and not something grounded in reality.
Justin Hendrix:
Does all this make you a little more optimistic about the future?
Karen Hao:
I'm cautiously optimistic. I mean, I'm always optimistic that in the long-term future, we will get to a point where there will be enough discourse, enough resistance, enough mobilizing to redirect the course of AI development towards something that is more democratic. That involves the input and voice of more people, that is more privacy preserving, is more like dignity preserving. But in the short term, I'm still kind of cautious because I think we have sort of seen many cycles of, there is a lesson to be learned that seems really obvious. And then U.S. policymakers and Silicon Valley just go exactly the opposite direction. So yeah, I mean, I really hope that this is a wake-up call that makes people go in the right direction. But I still kind of have to wait and see a little bit before I fully commit to being optimistic.
Justin Hendrix:
Well, Karen, I appreciate you taking the time out of your trip to speak to me about this. And look forward to the book and also to the opportunity to talk to you about these matters again in the future.
Karen Hao:
Awesome. Thank you so much, Justin, for having me.
Authors
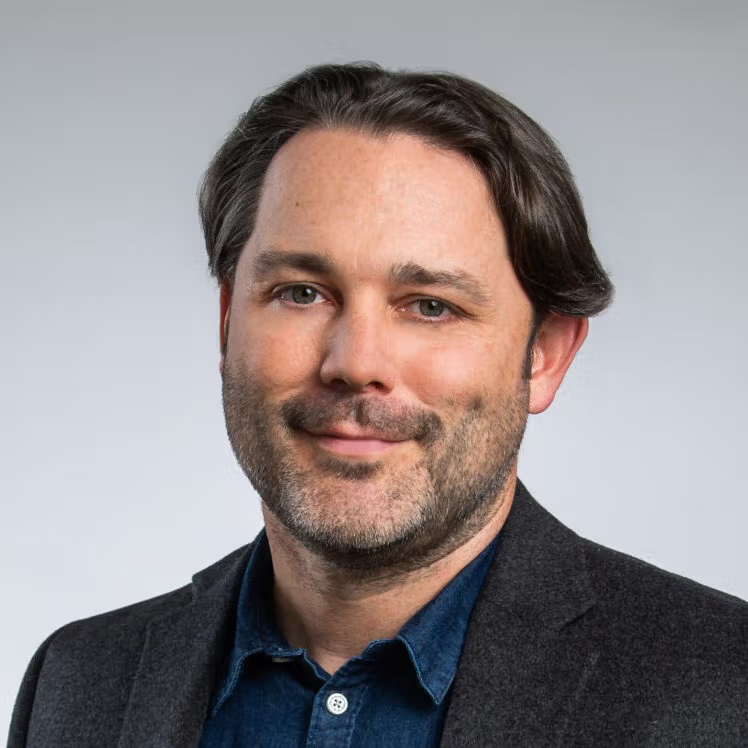