Can China Build Advanced AI Without Advanced Chips?
Matt Hampton / Jan 10, 2025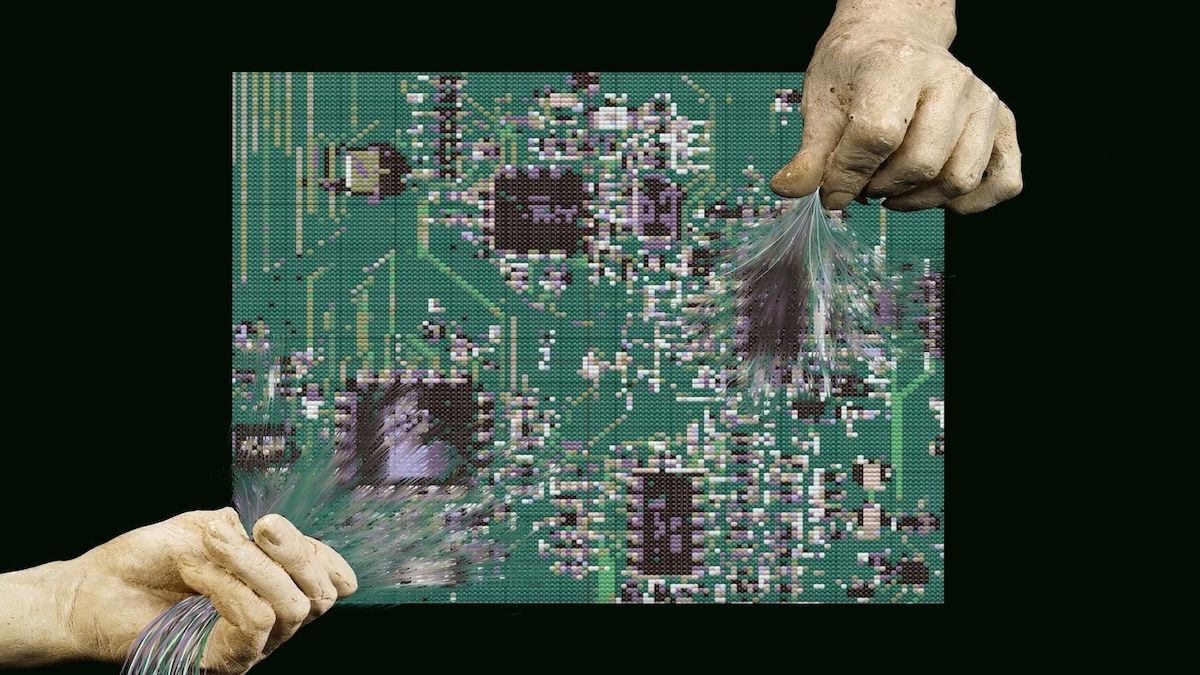
Hanna Barakat + AIxDESIGN & Archival Images of AI / Better Images of AI / Woven Circuit / CC-BY 4.0
In November, the Financial Times reported that a Chinese startup 01.AI trained an artificial intelligence model competitive with ChatGPT — at a small fraction of the cost of its Western counterparts. According to the company’s founder, the Yi-Lightning model cost $3 million to train, compared to an estimated $80 to $100 million for GPT-4. The cost efficiency was achieved by training the model on a small, high-quality dataset and employing clever engineering, thus requiring only a couple of thousand chips to power its model, compared to tens of thousands for OpenAI’s latest models.
Despite its model’s lower cost, 01.AI touts a high ranking from the University of California-Berkeley’s Sky Computing Lab and research group LMSYS based on user ratings of chatbot responses. As of December 9, the leaderboard shows Yi-Lightning tied for seventh place with the original GPT-4o, Grok, and Anthropic’s Claude but behind the most current version of GPT-4. Details about Yi-Lightning are scarce, and relying on a single ranking can be misleading. However, the development raises an important question: Can US controls on chip exports limit China’s AI development — or will Chinese tech companies find more efficient ways to develop AI technologies, undermining these efforts?
At the heart of the US-China AI race is access to “compute” or the massive processing power needed to train large models. The compute required for top generative AI models is estimated to double every six months. To constrain China’s AI ambitions and limit its computing power, the US has imposed strict controls, banning the export of advanced chips and the technologies used to create them to the People’s Republic of China (PRC).
Many experts believe regulations on computing power are key to governing AI. Yet Beijing has not sat idly by and instead has found ways around the restrictions, including accessing US chips via cloud computing, which the bans do not restrict. The country has also smuggled chips through shell companies and stockpiled chips before the export controls took effect. It has also sought to encourage Chinese production of advanced chips, subsidizing its domestic semiconductor industry to the tune of $48 billion (in 2024 alone). These evasions have been widely reported, but another way for China to stay in the AI race has received less attention.
Beyond Compute: The Algorithm Obstacle
Export controls can serve as a de facto limit on the amount of compute available to Chinese AI developers by making it more difficult for them to access advanced chips. But compute is not the only ingredient in AI development. Even if developers in China face restrictions on cutting-edge chips, they can make improvements by refining algorithms and training data to achieve better results with fewer resources. We can consider this the “work smart, not hard” approach.
Researchers from Massachusetts Institute of Technology and Epoch, an organization that researches trends in AI, estimated that, from 2012 to 2023, the compute required to train a large language model to a set performance level “halved every 8-9 months on average.” In a different AI tool, “computer vision,” used to train image classification models, Epoch found that the creation of better algorithms was equivalent to doubling compute every nine months since 2012. This made algorithms about equally as important as compute increases in fostering innovation in computer vision, whereas for LLMs, they were about half as important. Other studies also found contributions from algorithmic progress were as important as compute increases to AI development.
China has the innovative capabilities to take advantage of these realities. Experts estimate that China is as little as 18 months behind Western labs on frontier LLMs. The country produces the most machine learning papers in the world, and the most-cited paper in ML was written by Chinese authors. Chinese firms like Alibaba and DeepSeek produce models that are competitive with those produced by Meta. By mid-2024, top Chinese AI developers reached the level of GPT-4.
In addition, even a single innovation can change the course of AI. For example, the current compute scaling trend emerged from deep learning’s popularization. The Epoch paper on algorithmic progress in LLMs attributes 20% of algorithmic progress since 2017 to the invention of the transformer architecture (the “T” in GPT). Thus, rather than simply innovating along a pre-existing trend, Chinese researchers could make a breakthrough that puts them on a faster track than the US.
Yet, while it may be possiblefor China to algorithmically leap ahead, that does not make it likely. While Chinese universities publish more AI research, research from US organizations has more citations. Western labs have also led most of the recent breakthroughs.
To the extent that it remains strapped for computing power, it may get harder for China to innovate. This is because compute and algorithmic progress may be intertwined, as some experts argue that algorithmic improvements depend on growing amounts of compute to test and implement. This scarcity has already been a limiting factor. For example, around the time OpenAI released their video generation model Sora earlier this year, a Chinese lab was developing similar techniques but could only apply them to videos a few seconds long due to lack of access to sufficient computing power.
Another context in which compute can be a bottleneck is inference, i.e., the processes that run when a user submits a prompt to ChatGPT or asks Dall-E to generate an image. Many algorithmic improvements involve using less compute to train the model by allowing it to use more compute during inference. But this tradeoff can make it more difficult to process large inputs.
However, this obstacle only makes the proliferation of a model less practical and does not directly hinder its initial development. Furthermore, the amount of inference compute used for each copy of a model is much less than the amount of compute used for training. And even in reducing this cost, companies are finding ways to innovate, as is the case with 01.AI’s Yi-Lightning and with models that combine aspects of compute-intensive transformer models with other architectures that can process long prompts with less compute.
Conclusion
For US policymakers, the interplay of algorithmic and compute power for AI development poses a formidable challenge. Even if export controls successfully limit China’s access to advanced chips, breakthroughs in efficiency could still allow Chinese labs to achieve significant capabilities. Whether this outcome is good enough depends on whether relative or absolute capabilities are what matter. If the goal is not only to keep the PRC behind the US but also to prevent it from unlocking certain capabilities, algorithmic progress represents a potential hole in chip bans.
For example, if we consider the October 2023 AI executive order reporting threshold as a proxy for dangerous capability levels, China may be able to reach the equivalent of this level within a few years through algorithmic improvements without access to advanced chips. And smaller, specialized models may already pose significant risks, including the potential to create biological or chemical weapons.
Critics of export restrictions raise concerns that these measures do not effectively restrict China’s access to chips and counterproductively spur Beijing to develop supply chains that avoid Western inputs. In the process, they argue, lack of access to Chinese markets harms US semiconductor firms. Although these impacts remain debated, proposed alternatives often center around closing loopholes and deploying narrowly targeted restrictions to limit harm to US companies while more effectively denying China access to compute. However, to the extent that algorithmic progress proves to be a meaningful factor in the US-China AI race, this innovation could complicate the matter even further, as the situation becomes not only about controlling the flow of a physical good—advanced AI chips—but the more difficult challenge of restricting the code or algorithms that underpin AI models.
Authors
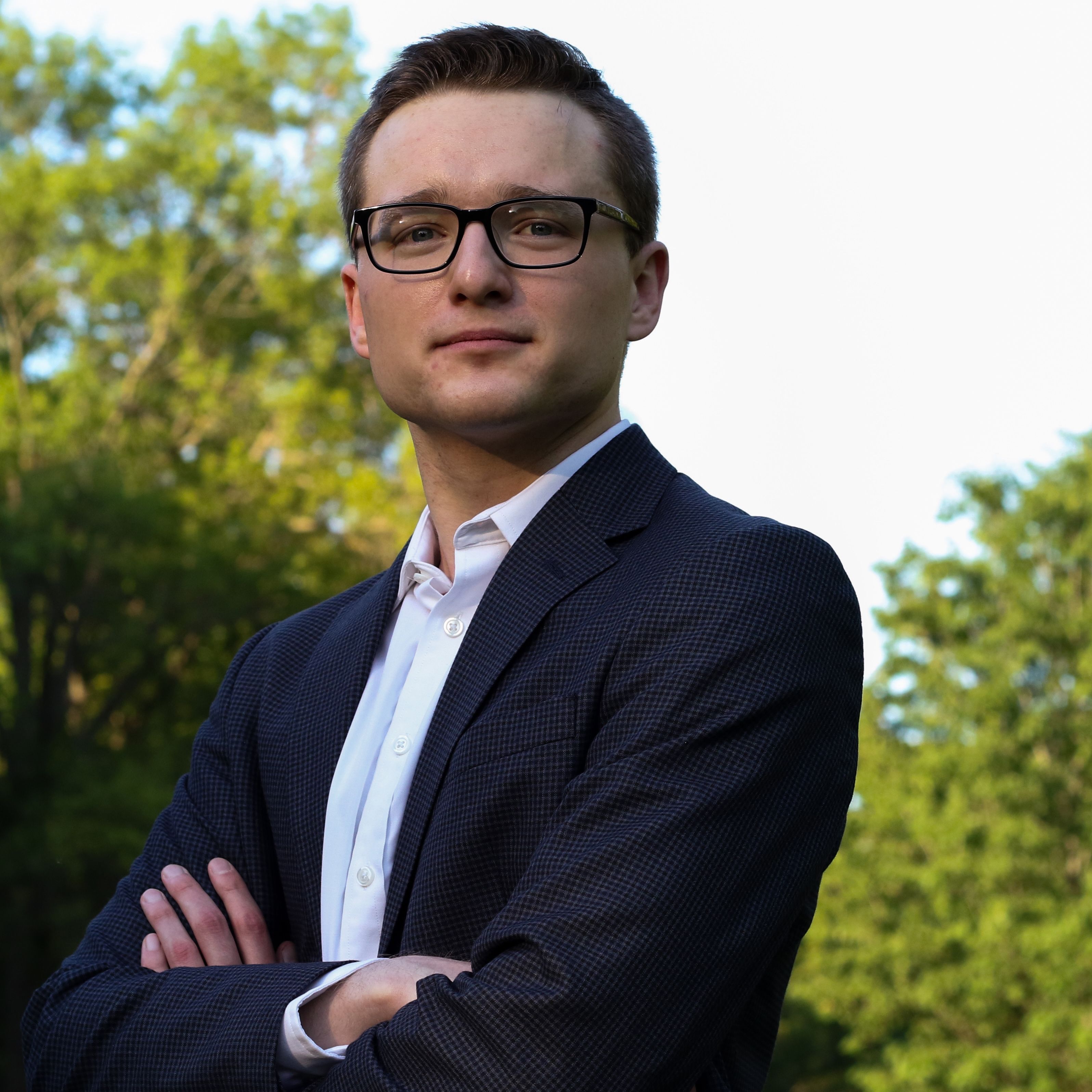