Beyond the Buzz: Clear Language is Necessary for Clear Policy on AI
Joseph B. Keller / Mar 28, 2024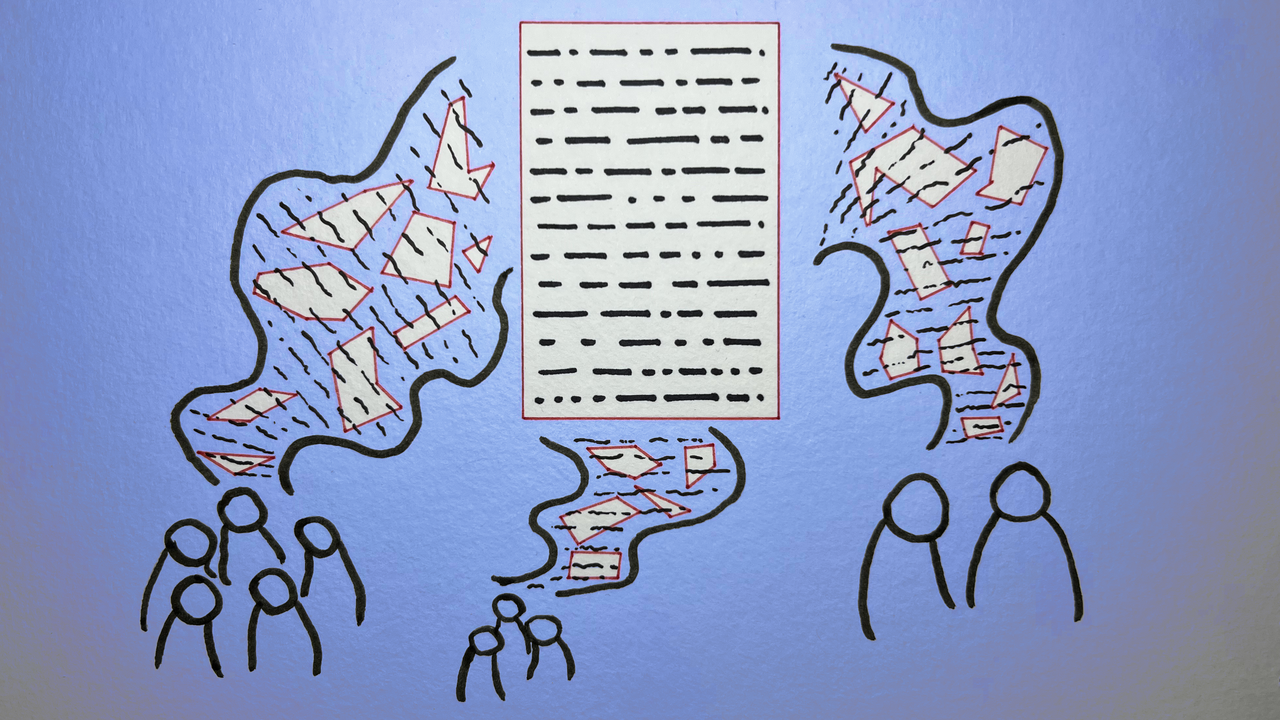
Yasmine Boudiaf & LOTI / Better Images of AI / Data Processing / CC-BY 4.0
Based on the number of new bills across the states and in Congress, the number of working groups and reports commissioned by city, state, and local governments, and the drumbeat of activity from the White House, it would appear that it is an agenda-setting moment for policy regarding artificial intelligence (AI) in the United States. But the language describing AI research and applications continues to generate confusion and seed the ground for potentially harmful missteps.
Stakeholders agree that AI warrants thoughtful legislation, but struggle for consensus around problems and corresponding solutions. An aspect of this confusion is embodied by words we use. It is imperative that we not only know what we are talking about regarding AI, but agree on how we talk about it.
Last fall, the US Senate convened a series of closed-door meetings to inform US AI strategy. It brought together academics and civil society leaders, but was disproportionately headlined by prominent industry voices who have an interest in defining the terms of the discussion. From the expanding functionality of ever-larger AI models to the seemingly far-off threat to human existence, lawmakers and the public are immersed in AI branding and storytelling. Loaded terminology can mislead policymakers and stakeholders, ultimately causing friction between competing aspects of an effective AI agenda. While speculative and imprecise language has always permeated AI, we must emphasize nomenclature leaning more towards objectivity than sensationalism. Otherwise, US AI strategy could be misplaced or unbalanced.
Intelligence represents the promise of AI, yet it’s a construct that’s difficult to measure. The very notion is multifaceted and characterized by a fraught history. The intelligence quotient (IQ), the supposed numerical representation of cognitive ability, remains misused and misinterpreted to this day. Corresponding research has led to contentious debates regarding purported fundamental differences between IQ scores of Black, White, and Hispanic people in the US. There's a long record of dubious attempts to quantify intelligence in ways that cause a lot of harm, and it poses a real danger that language about AI might do the same.
Modern discussions in the public sphere give full credence to AI imbued with human-like attributes. Yet, this idea serves as a shaky foundation for debate about the technology. Evaluating the power of current AI models relies on how they’re tested, but the alignment between test results and our understanding of what they can do is often not clear. AI taxonomy today is predominantly defined by commercial institutions. Artificial general intelligence (AGI), for example, is a phrase intended to illustrate the point at which AI matches – or surpasses – humans on a variety of tasks. It suggests a future where computers serve as equally competent partners. One by one, industry leaders have now made AGI a business milestone. But it’s uncertain how to know once we’ve crossed that threshold, and so the mystique seeps into the ethos.
Other examples illustrate this sentiment as well. The idea of a model’s ‘emergent capabilities’ nods to AI’s inherent capacity to develop and even seem to learn in unexpected ways. Similar developments have convinced some users of a large language model’s (LLM) sentience.
However, while these concepts are currently disputed, other scientists contend that even though bigger LLMs typically yield better performance, the presence of these phenomena ultimately relies on a practitioners’ test metrics.
The language and research of the private sector disproportionately influences society on AI. Perhaps it’s their prerogative; entrepreneurs and industry experts aren’t wrong to characterize their vision in their own way, and aspirational vocabulary helps aim higher and broader. But it may not always be in the public interest.
These terms aren’t technical jargon buried deep in a peer-review article. They are tossed around every day in print, on television, and in congressional hearings. There’s an ever-present tinge of not-quite-proven positive valence. On one hand, it’s propped up with bold attributes full of potential, but on the other, often dismissed and reduced to a mechanical implement when things go wrong.
The potential societal impact is inevitable when unproven themes are parroted by policymakers who may not always have time to do their homework.
Politicians are not immune to the hype. Examples abound in the speeches of world leaders like UK Prime Minister Rishi Sunak and in the statements of President Joe Biden. Congressional hearings and global meetings of the United Nations have adopted language from the loudest, most visible voices providing a wholesale dressing for the entire sector.
What’s missing here is the acknowledgement of how much language sets the conditions for our reality, and how these conversations play out in front of the media and public. We lack common, empirical, and objective terminology. Modern AI descriptors mean one thing to researchers, but may express something entirely different to the public.
We must call for intentional efforts to define and interrogate the words we use to describe AI products and their potential functionality. Exhaustive and appropriate test metrics must also justify claims. Ultimately, hypothetical metaphors can be distorting to the public and lawmakers, and this can influence the suitability of laws or inspire emerging AI institutions with ill-defined missions.
We can’t press reset, but we can provide more thoughtful framing.
The effects of AI language are incredibly broad and indirect but, in total, can be enormously impactful. Steady and small-scale steps may deliver us to a place where our understanding of AI has been shaped, gradually modifying behavior by reinforcing small and successive approximations — bringing us ever closer to a desired belief.
By the time we ask, “how did we get here,” the ground may have shifted underneath our feet.
Authors
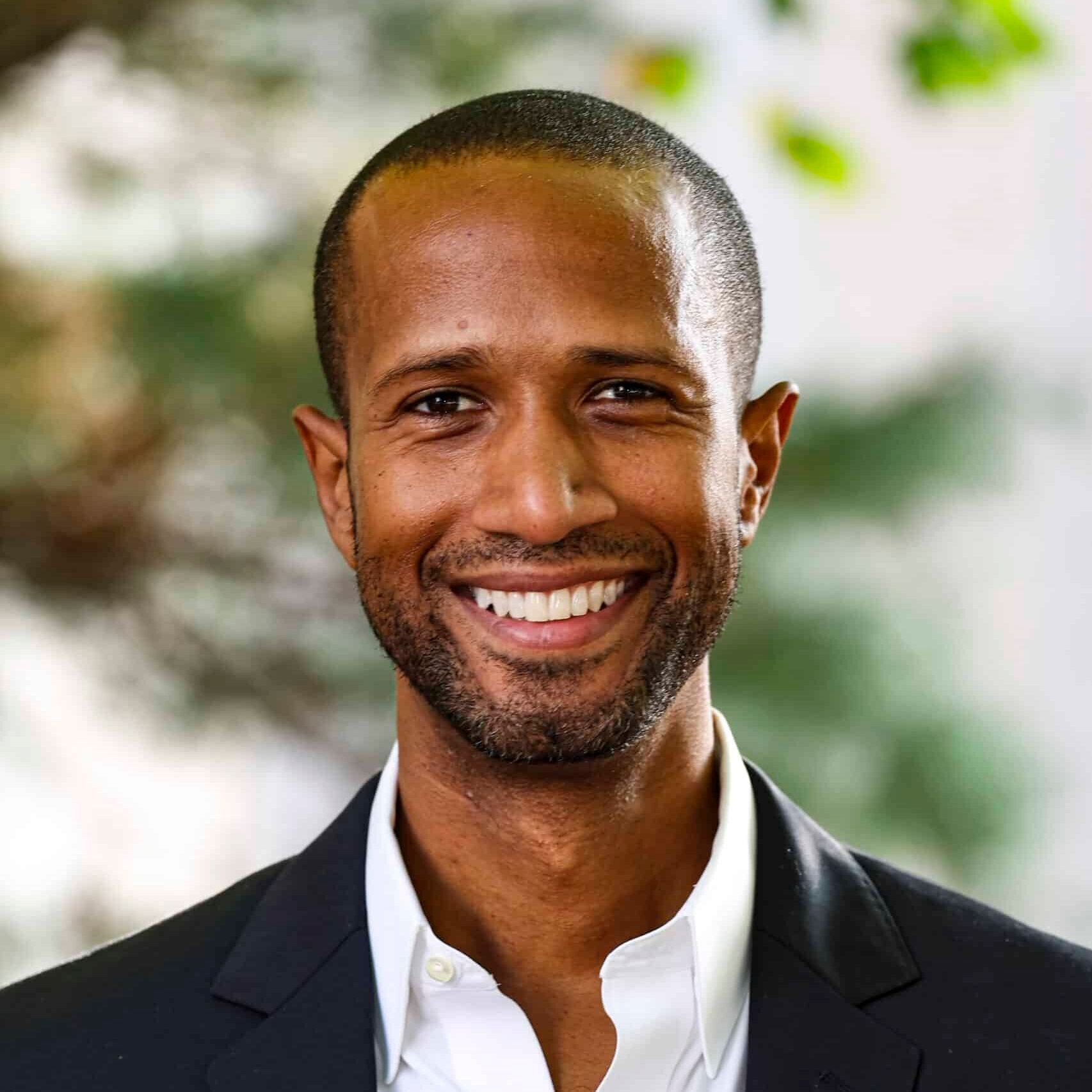