AI Bias is Not Ideological. It’s Science.
Genevieve Smith / Apr 1, 2025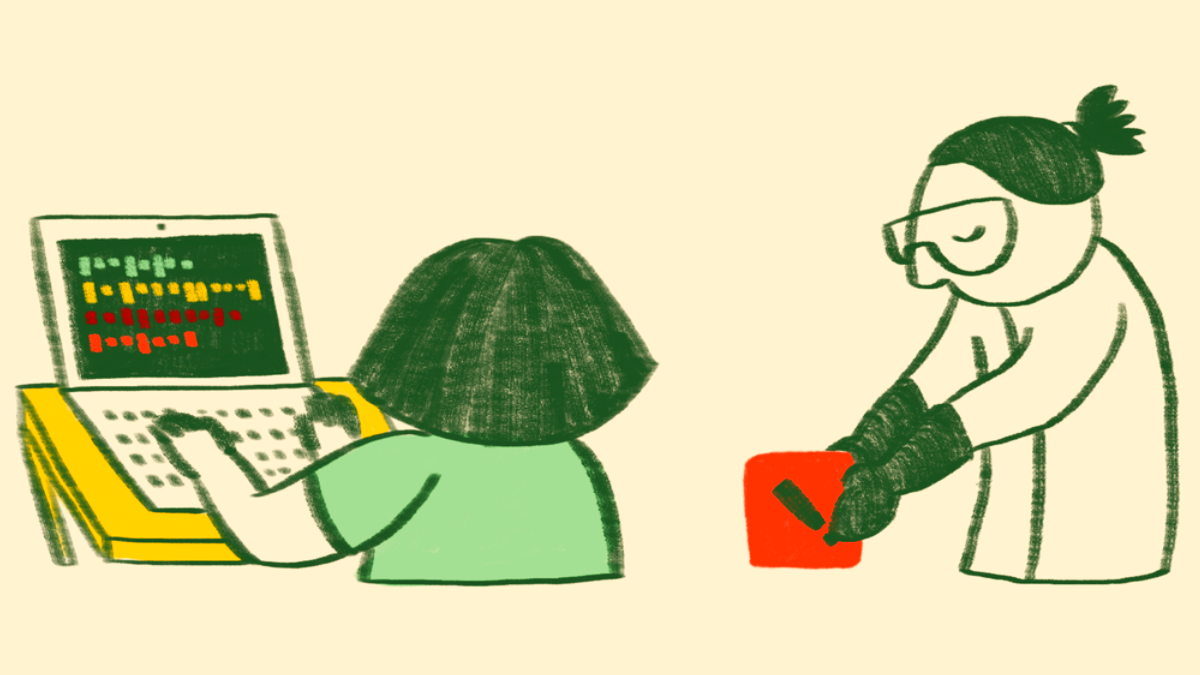
Yasmin Dwiputri & Data Hazards Project / Better Images of AI / Safety Precautions / CC-BY 4.0
The National Institute of Standards and Technology (NIST) recently issued new guidance to scientists who partner with the US Artificial Intelligence Safety Institute (AISI), directing them to prioritize “reducing ideological bias, to enable human flourishing and economic competitiveness,” as Wired recently reported. The language echoes a late January Executive Order from the Trump Administration for AI systems to be “free from ideological bias or engineered social agendas.”
At the same time, NIST removed “AI safety,” “responsible AI,” and “AI fairness” from the list of expected skills for AISI members. These changes reflect an abrupt change from the previous cooperative research and development agreement, which encouraged researchers to identify bias and discrimination in model outputs.
This is short-sighted. Removing terms like “AI fairness” or “responsible AI” and claiming that doing so is necessary to avoid "ideological bias" reflects a lack of understanding of both the issue and the science. AI systems today show significant performance discrepancies and amplify limiting stereotypes. These problems hold back the technology from serving– and being trusted by– all people. If the administration truly aims to advance AI to promote human flourishing, it must ensure the tech works for all Americans, not just some.
As generative AI tools become more common in decision-making and service provision in the public and private sectors, suppressing discourse or research on AI fairness will result in harm. Doing so will mean that those decisions and services will worsen outcomes for certain groups, including already marginalized ones, while further reinforcing existing discriminatory patterns.
On March 21, researchers signed and released the Scientific Consensus on AI Bias, affirming the “scientific consensus that AI can exacerbate bias and discrimination in society.” This consensus is grounded in a substantial body of peer-reviewed studies that have illustrated bias in AI systems that could lead to harmful and often unequal outcomes.
The persistent problem in machine learning today
The dominant forms of AI technologies today, including algorithmic decision-making systems and generative AI, use machine learning. These systems find patterns from massive datasets to make predictions that inform decisions or generate outputs like text. Generative AI technologies, powered by large language models and other systems, are learning from data (e.g., text, images, and videos) scraped from the internet–data that often reflects societal biases and fails to equally represent different populations.
As a result of these structural biases and representation gaps, researchers have identified two key issues related to fairness in AI outputs.
Issue 1 – Performance discrepancies
AI models work better for some groups and worse for others. Seminal research by Joy Buolamwini and Timnit Gebru found that facial recognition algorithms performed significantly worse for Black people and women, especially Black women.
The same holds for generative AI. My colleagues at UC Berkeley (Eve Fleisig, Madeline Bossi, Ishita Rustagi, Xavier Yin, and Dan Klein) and I conducted a large-scale study of linguistic bias in ChatGPT to examine its behavior in response to text in different varieties of English. We examined ten varieties of English, including, for example, Standard American English (SAE), African American English, Standard British English (SBE), and Indian English.
We found that model responses to varieties outside of SAE and SBE had lower comprehension (went down 9%), more demeaning content (25% worse), and greater condescension in responses (15% worse). They also default to “standard” varieties of English, reinforcing a certain way of communication as “correct.” These discrepancies matter: if a person has to go through a generative AI tool to access a service (e.g., a customer service chatbot for public benefits), it won’t work as well for them, potentially impacting the quality of information and access to services. This also means that as adoption increases, some people will be able to get more benefits from using the technology (particularly related to productivity or efficiency) than others, potentially amplifying inequality.
Issue 2 – Reinforcement of limiting stereotypes
Beyond performance discrepancies, generative AI tools are also prone to reinforcing harmful stereotypes. They are, after all, pattern recognition machines, and when trained on biased data, including stereotypes, they reflect and amplify those biases that exist in the world around us. In the aforementioned study on linguistic bias in ChatGPT, we also found that ChatGPT responses outside of SAE and SBE resulted in greater stereotyping (19% worse).
However, they don’t just replicate stereotypes – they amplify them. In a research study with colleagues (Leander Girrbach, Stephan Alaniz, and Zeynep Akata), we conducted a large-scale analysis of gender bias in text-to-image models by evaluating gender representation in outputs related to everyday activities and occupations. We observed that bias in male-majority occupations is almost always amplified. For example, when prompting the models to return images of financial analysts, only 16% of image outputs included women (despite women being 43.9% of financial analysts in the US). By reinforcing stereotypes, these tools can negatively shape public perceptions and impact one’s behavior.
It may come as no surprise, then, that there are trust gaps in generative AI. For example, fewer women than men report trusting generative AI, which leads to lower adoption. These persistent issues with AI technologies need to be addressed, not just out of social concern but as necessary to fix core product and service issues.
AI tools offer immense potential for influence and power in our society. Calling research on fairness in AI systems “ideological” is itself a political and ideological stance. It reflects a recognition that these tools are powerful mechanisms to shape social systems, and waves away valid concerns of performance discrepancies and biased outputs. This approach is not set up to support human flourishing, but rather to amplify inequality and divisiveness.
State leaders can take action
As the Scientific Consensus on AI Bias states, research on bias in AI has “been a basis for bipartisan and global policy makers for nearly a decade.” In short, do not abandon AI fairness. That will not serve your constituents or your goals. Abandoning goals for AI fairness means the tools you employ will work better for some and worse for others. This has real implications for those who access and benefit from government services and those who cannot. It also means discrimination will run through the use of these tools in ways that are difficult to spot and solve due to the black-box nature of powerful AI technology.
With that in mind, here are two key recommendations for state leaders:
- When using generative AI or algorithmic decision-making tools in government contexts, acknowledge that bias is reflected in these tools. Identify what types of biases the particular application or use case may be prone to, and consider whether that is acceptable for the particular use case (sometimes the answer is not to use the tool). Then implement strategies to mitigate that bias (e.g., through auditing and having a human-in-the-loop).
- Continue developing public policy informed by scientific consensus on bias in AI systems. As AI technologies are increasingly integrated into government services and in government workplaces, we must double down on efforts to prioritize AI fairness, not walk away from it.
If we want AI to support human flourishing, we must focus on ensuring the technology contributes to an inclusive and just society. That means addressing bias in AI and maintaining a commitment that these tools work for the whole of society.
As opposed to being complacent with flawed technology, we have the ability – and opportunity – to use AI in ways aligned with American values and continue imagining a just technological future. To leave with a quote from Prof. Alondra Nelson:
“we can be asserting today, every day, a different way, an alternative vision of thinking about how technology should be in society… [there] are the fundamental things that we say we stand for and that need to be enacted and modeled certainly, principally, by government.”
Authors
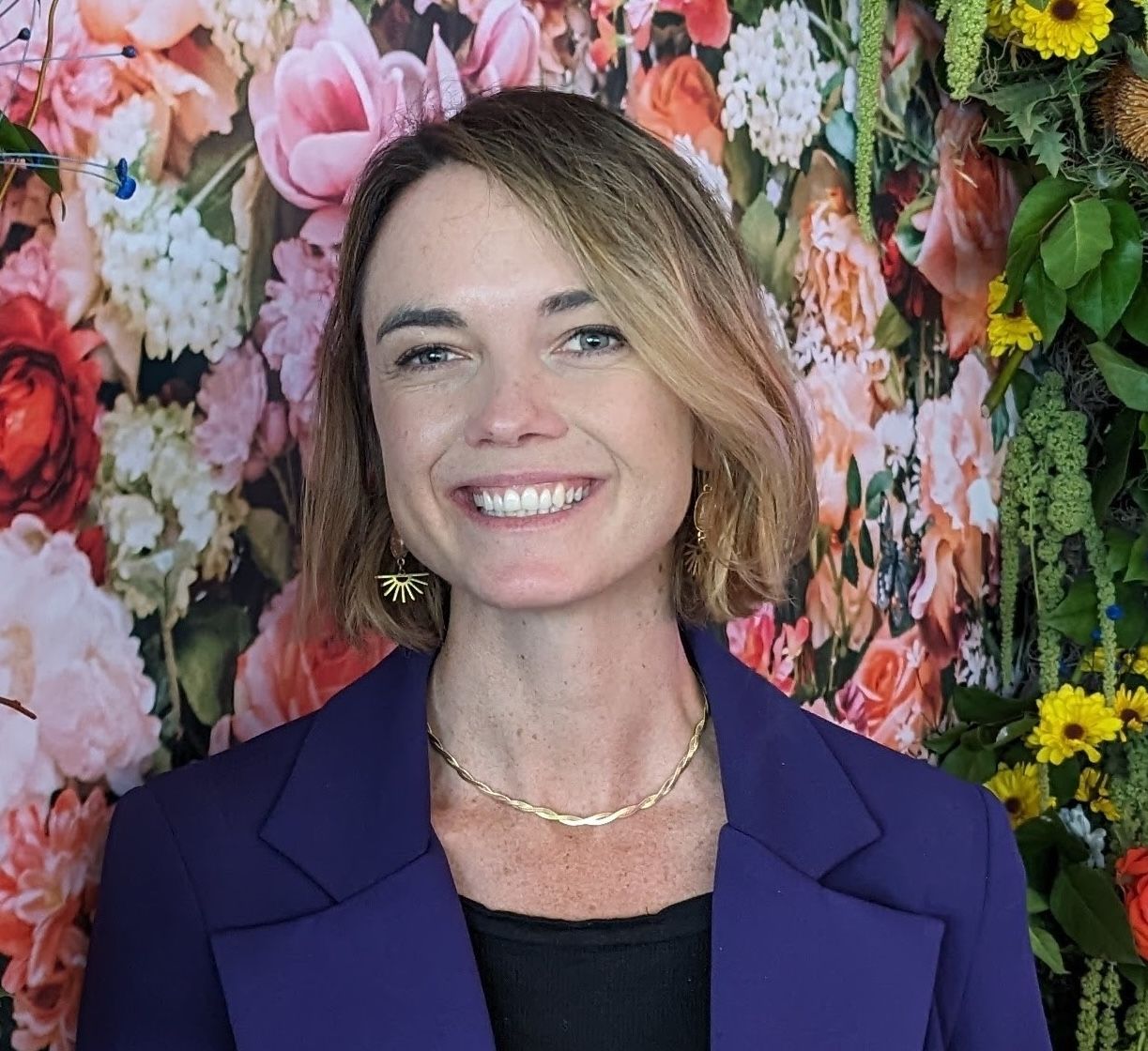