AI at Work: Maximizing Human Potential by Understanding Tech’s Limitations
Diana Enriquez / Feb 5, 2025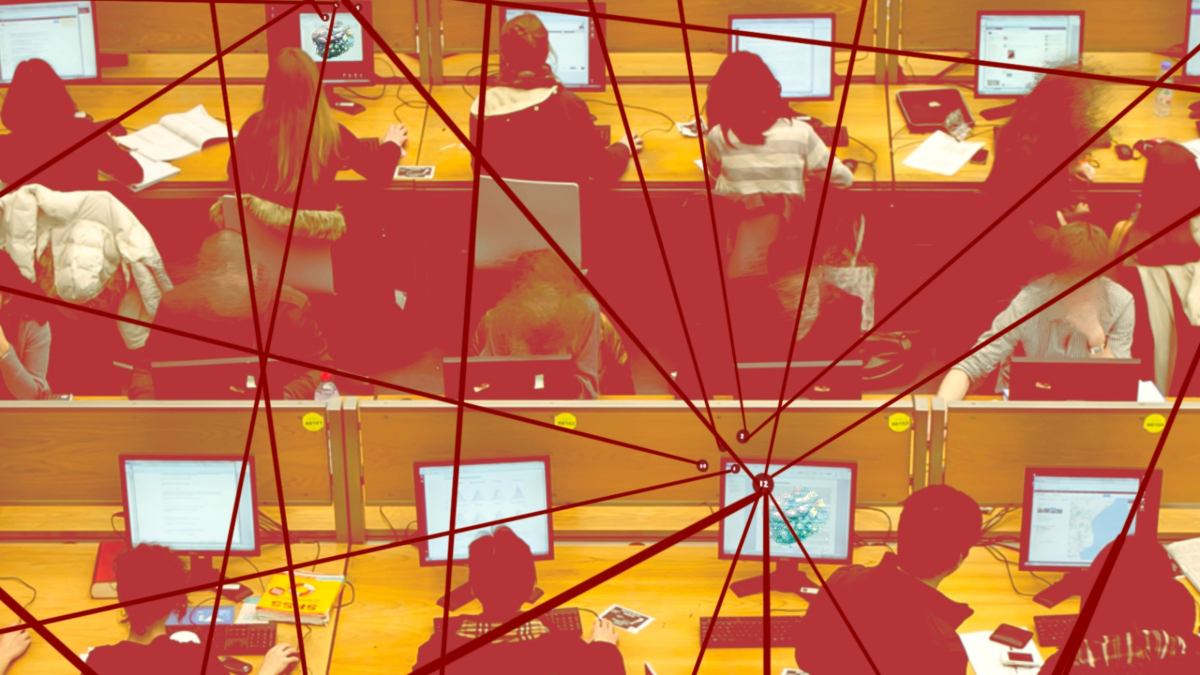
Hanna Barakat & Cambridge Diversity Fund / Better Images of AI / Data Lab Dialogue / CC-BY 4.0
AI hype is at an all-time high this month, with news from China suggesting the startup DeepSeek found a better, faster, cheaper way to develop AI models to compete with US firms. But even as breathless commentators blather on about what it all means for markets and the AI race, it’s already clear that the adoption of AI in the workplace is slower than the industry promised and that AI has its limits.
Looking beyond the hype, perhaps this moment allows us to more soberly assess 1) what automation does (and doesn’t) do well, 2) where we can see the last mile in most technology – even when we cover up the roles people play in closing the gap, and 3) how to acknowledge AI’s limitations so we make better design choices and treat technology as a helpful tool rather than a black box solution. Such an assessment starts with accepting that while today’s AI systems are far from perfect, these tools can already help people do some very useful things if they clearly see and understand the technology without exaggerating its power.
I am a sociologist and human-machine partnership designer who has spent the last 15+ years looking at the relationships between people and technology. I thought about how to make comments sections less toxic when I worked at TED (and found some solutions, including the experiments run at video game companies like Riot Games), tried to automate contact tracing during the Ebola pandemic (and saw the limits of automation first hand), then tried to make translation in sensitive environments work better at Twitter. I care a lot about bridging the last mile in technology and making the design choices more visible so that we don’t treat any object we’ve created as an all-knowing black box. I’ve found that whenever we treat technology as a black box with no knowledgeable editor or engineer able to fix its errors, we run into very serious problems of our own making.
What Technology Does (And Does Not) Well
Let’s start by addressing some assumptions in the current model. We hear every day how close we are to the synchronicity between human and machine intelligence. This type of convergence is hyped nearly every week by men like Sam Altman at OpenAI and other actors who benefit from building mystique around their products. For years, tech companies have relied on embedding their products into our lives and work. The goal is to ensure technologies such as AI are the indispensable sticky infrastructure of our lives.
It helps if most people don’t understand how that infrastructure works. AI is built using very complicated prediction models that are difficult for most people to review and comprehend. This is important because most folks don’t recognize that these prediction models draw heavily from patterns acquired by observing data from the past. This can be exciting, but while we can learn from the past, historians, sociologists, and many other experts can tell you the danger of using the past as a blueprint for the future. The power of human minds is that we can make new choices and designs, even when they feel risky or new.
Likewise, most people don’t understand that AI is often built with unsupervised models. Ask anyone who does a lot of math and tries to interpret the findings or logic of an unsupervised model, and they will tell you – it is often a pretty meaningless collection of correlations. In fact, in a giant experiment run by Princeton University with hundreds of teams composed of engineers, social scientists, and other experts, the most technical, advanced models on the planet were not good at predicting or explaining outcomes as people. Human beings are too creative and unpredictable to be constrained by their own past experiences or to merely explain phenomena by relying on a set of correlations, however advanced. We should see this as a gift rather than something to try harder to control or replace.
There Is And Will Always Be A Last Mile In Technology
Technology design often abstracts hundreds of use cases and tries to synthesize a theory or equation that can be replicated in other settings. Ask any engineer or designer how well this works to solve the entire problem– no one dislikes technology as a full solution more than an engineer or a designer because they know what the design is optimized for and where its deficiencies are. (I make this generalization as someone who is a designer, married to a very senior engineer, who spends most of her time around designers and engineers grumbling nervously about the ways people interact with the objects we produce.) Most people feel the holes in technology when they have a frustrating experience with an automated customer service agent, or the Spotify playlist is vaguely right but you still don't like many of the songs on your recommendations list. You can feel the quality diminish in objects designed by automation – think of how boring Netflix movies became when they were churning them out and reproducing the same data-driven formulas repeatedly. It’s even more frustrating in cases where the technology is supposed to fix a bigger and harder-to-define problem, and people see that the technology, designed as a one-size-fits-all, cannot serve a diverse community effectively without significant investment and commitment to making many tailored design improvements along the way.
We see the last mile between technology and people in so many places –- and we’ll continue to need to close it in new ways even as we find more and more granular ways to automate and reduce the gap. Even in cases where the technology may seem straightforward now, like the robots dispensing medication at your local pharmacy to “save costs” and “reduce error,” we see the human counterparts frequently fixing, correcting, and overall performing the task of these robots even when the upper management and customers believe the robots are doing the work. Fax machines and printers – technology we’ve had a lot of time to improve and address – still often require someone to fix them with a mix of knowledge and improvisation until the machine can be corrected. Experienced truck drivers, Uber drivers, and Amazon workers alike can tell you all the places where the new software in cars works really well – until they run into the unpredictable conditions they face driving through challenging weather conditions or other human-driven factors on roads. Even the hyper-advanced factories we’re led to believe exist in China are full of performance art by the workers on the shop floor working around the errors of the machines designed to automate and speed up their work. Middle managers often note that the improvisation skills of human workers surpass the deep limitations of the machines designed to replace them. In each of these cases, the manager sees the last mile between the technology and the worker in charge of finishing the job correctly. The human needs to know the task and its goals better than the machine so they can reliably edit the work and tailor the solution to the conditions at play.
Well, Nothing Is Perfect. Should I Still Work With AI?
Technology sometimes solves clearly defined and constrained problems well – but people add the creative design work that brings the solution into the present and makes it ready to address a problem. Technology is very useful for scaling a basic and constrained solution, making something routine faster, and giving you some raw materials to work with, but you need to be the designer and tailor to the last mile. It is a tool to be wielded, not an all-knowing force. But how can you assert these caveats when your boss wants you to integrate AI into your work?
- Pick cases where the problem is constrained enough that your request is clear and manageable for an automated process.
- Identify what areas of your request you’re hoping the AI fills in for you or teaches you – and the areas of “high risk” output where you may not wish to rely on AI at all.
- Make sure you have a clear enough picture of the desired outcome so that you can be a good editor or find a good editor who can guide you.
- AI is designed to give you what you want. You must also understand what piece of the output is a siren’s call designed to make you feel good, but might not be the right solution. Think critically about your expectations and what you might be projecting on the output that gets you into trouble when introducing this solution into another context. Producing something with AI doesn’t make it a better solution - you will always need to defend your design choices.
- Never accept the output as a perfect given asset– where can you see the “last mile” and improve on the model?
Faith in a “black box” technology like AI will not serve you or your teams if you cannot guide and push the design forward. Thankfully, companies are starting to build products designed for specific purposes, rather than pretending to solve everything at once. The strength we add to technology as the human in the human-machine partnership is that history and our past design choices are not inevitable. We have to make the push to change things. We must take AI seriously and acknowledge it for what it is – merely another tool with strengths and limitations that will work better when we can acknowledge its material reality. But that might not be pithy enough for Sam Altman’s pitch deck.
Authors
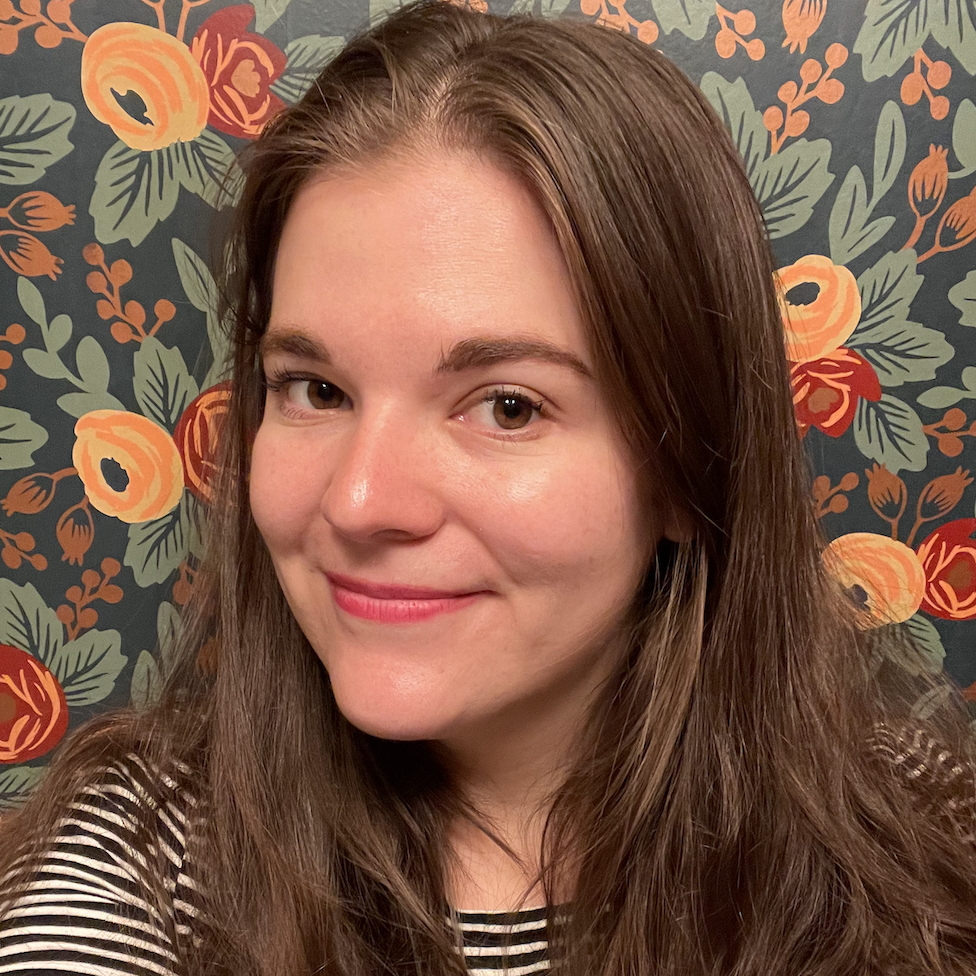