The Flexibility Trap: How Algorithms Drive Precarity in Platform Work
Atieh Razavi Yekta / Dec 10, 2024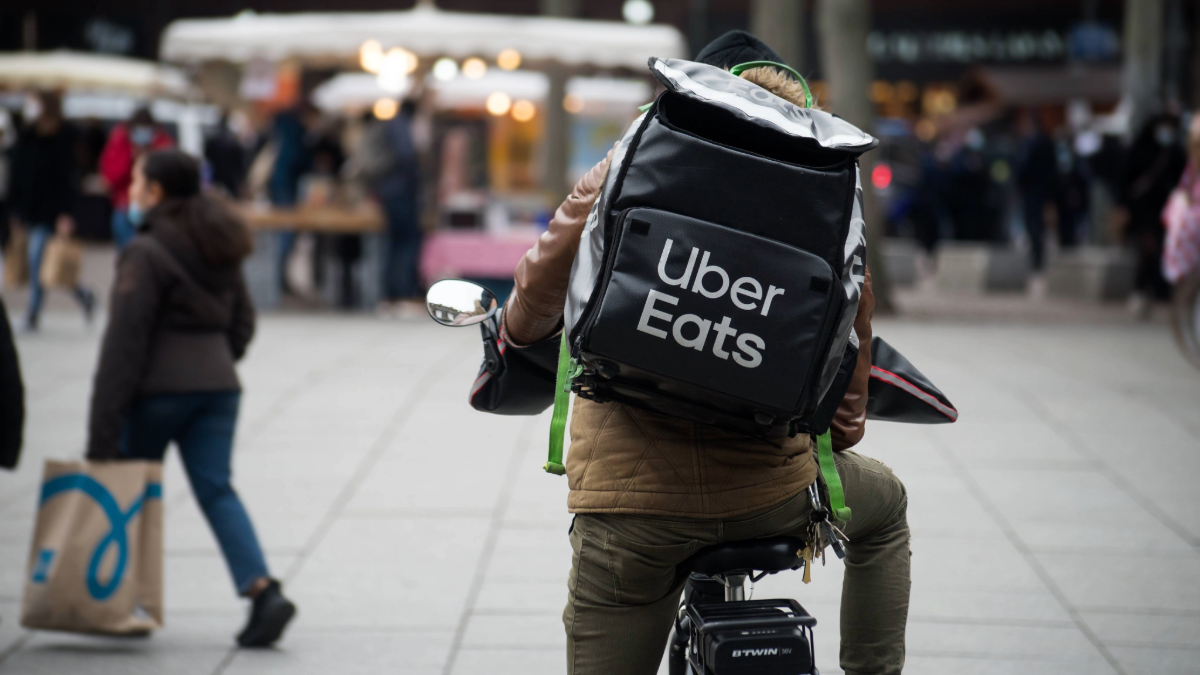
An Uber Eats delivery worker photographed in Strasbourg, France. Shutterstock
Technology, particularly the rise of algorithmic management systems used by digital platforms, is fundamentally reshaping the nature of work by altering how tasks are performed, the skills required, and the ways workers are compensated. Platform work exemplifies this transformation, with algorithms playing a central role in managing workers through task allocation, performance evaluation, and disciplinary actions. Algorithmic management specifies tasks through sequencing, timing, and accuracy requirements; evaluates workers via continuous performance tracking and productivity analytics; and enforces compliance through automated systems of rewards, penalties, and replacement. These systems not only consolidate digital platform control but also complicate the contested narratives of self-employment, flexibility, and autonomy, which are frequently promoted as defining features of platform work.
In the platform economy, algorithms often obscure how platforms extract value from workers, masking the ways workers' efforts translate into profit. Workers may be unaware of the extent of their exploitation, especially when algorithms are presented as neutral or fully automated. This lack of transparency prevents workers from understanding or challenging how their labor is being exploited, particularly in systems where performance ratings and earnings are algorithmically determined. For example, platforms may present algorithms as impartial, even though humans (e.g., machine learning engineers and data scientists) often intervene in their operation. This process of "agency laundering" obscures the source of unfairness or discrepancies, making it more challenging for workers to advocate for better working conditions.
Agency laundering involves distancing human decision-makers from morally questionable actions by attributing them to automated systems or algorithms. It is similar to "masking," where algorithmic systems conceal discriminatory practices, but agency laundering involves a more deliberate effort to shield human actors from accountability by attributing decisions to supposedly neutral technologies or organizational systems. The core of agency laundering lies in obscuring the source of responsibility, much like money laundering hides the illicit origins of money. By delegating responsibility to algorithms, digital platforms can avoid scrutiny, even when these systems lead to morally questionable or discriminatory outcomes. This blending of human actions with technological systems makes it difficult to pinpoint who is truly responsible.
Agency laundering also ascribes morally neutral qualities to technologies, allowing companies to justify questionable practices.
For example, Uber’s surge pricing might be framed as a simple algorithmic response to market demand, masking the moral implications of exploiting vulnerable workers or customers. This shifts the focus away from human accountability, making it harder to identify the true sources of these decisions. Ultimately, agency laundering undermines accountability. When decisions are framed as originating from neutral, automated systems, it becomes difficult for workers or consumers to hold anyone accountable for unfair outcomes. The lack of transparency prevents contestation or redress, as the system appears to operate independently of human influence. Through agency laundering, companies can deflect responsibility, obscuring the moral implications of their actions and making it more challenging to address exploitation in the platform economy.
Another frequently contested topic in platform work is the use of computational systems to track and quantify workers’ activities, often in real-time, creating what Vallas and Schor refer to as "digital cages." This includes monitoring not just the quantity of work done but also the quality of output and even the duration of breaks or idle time. This granular level of monitoring is pervasive in platform work, where algorithms can record every aspect of a worker’s engagement, from the speed at which tasks are completed to the frequency of interactions with clients or platforms. The information asymmetry between workers and platforms is a key element here. While workers are under constant surveillance, they typically have very limited access to data about their own performance. For example, freelancers on platforms like Upwork often do not know exactly how the algorithms weigh their ratings or which factors influence their visibility to potential clients. This creates a situation where workers are subject to the algorithms' judgment but cannot fully understand or contest the evaluation criteria. In contrast, platforms have a wealth of data at their disposal, allowing them to track worker performance and adjust pay or rankings, accordingly, creating significant power imbalances.
For instance, workers are either penalized (e.g., by deactivating or suspending accounts) or incentivized (e.g., with priority access to high-demand customers or gamified rewards like badges, points, or levels) by algorithms, which can replace them with others based on performance metrics, offering minimal human interaction in the process. This fosters a sense of disposability and creates a disposable labor force. For example, in ride-hailing or food delivery platforms, algorithms track when a worker is online, the distance traveled, and even the customer’s satisfaction score, often linking these data points to performance assessments and earnings.
Digital platforms use gamification functions as a form of algorithmic control, where platforms design reward systems that encourage workers to engage with tasks in a game-like environment. These systems often rely on elements such as digital points and badges to motivate workers and align their behavior with platform goals. For instance, platforms might track metrics like task completion speed, customer ratings, or the number of tasks completed and use these to award workers with gamified rewards, such as unlocking higher-paying tasks or achieving status symbols within the platform (e.g., badges or levels). This approach is designed to make the work experience feel more engaging and fun, enhancing worker motivation by presenting everyday tasks as part of a larger game. The competitive elements, such as earning points or competing for top spots, not only encourage productivity but also foster a sense of achievement and progress.
For example, some platforms might reward drivers or delivery workers with points for completing jobs quickly or receiving high ratings, and those who accumulate the most points may gain access to better shifts or higher-paying jobs. However, much like other forms of gamification in the workplace, these rewards are often designed to benefit the platform by maximizing productivity while obscuring the underlying power dynamics and reinforcing the platform’s control over workers. The result is a form of algorithmic management where the affective experience of work is shaped by game-like incentives, making workers more invested in the platform’s goals while masking the potential exploitative aspects of the platform economy.
In conclusion, while digital platforms offer valuable entrepreneurial opportunities and flexibility, the algorithmic management systems they employ to control, evaluate, and discipline workers often operate without sufficient transparency or accountability. This lack of oversight exacerbates precarious working conditions, reinforcing a labor force that is increasingly disposable and vulnerable to exploitation. As platform work grows, addressing these issues is critical to ensuring fair treatment and sustainable working conditions for all workers.
Related Reading
Authors
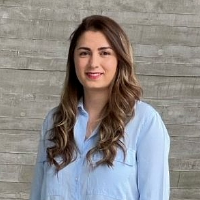