Why Generative AI Isn’t Transforming Government (Yet) — and What We Can Do About It
Tiago C. Peixoto / May 21, 2025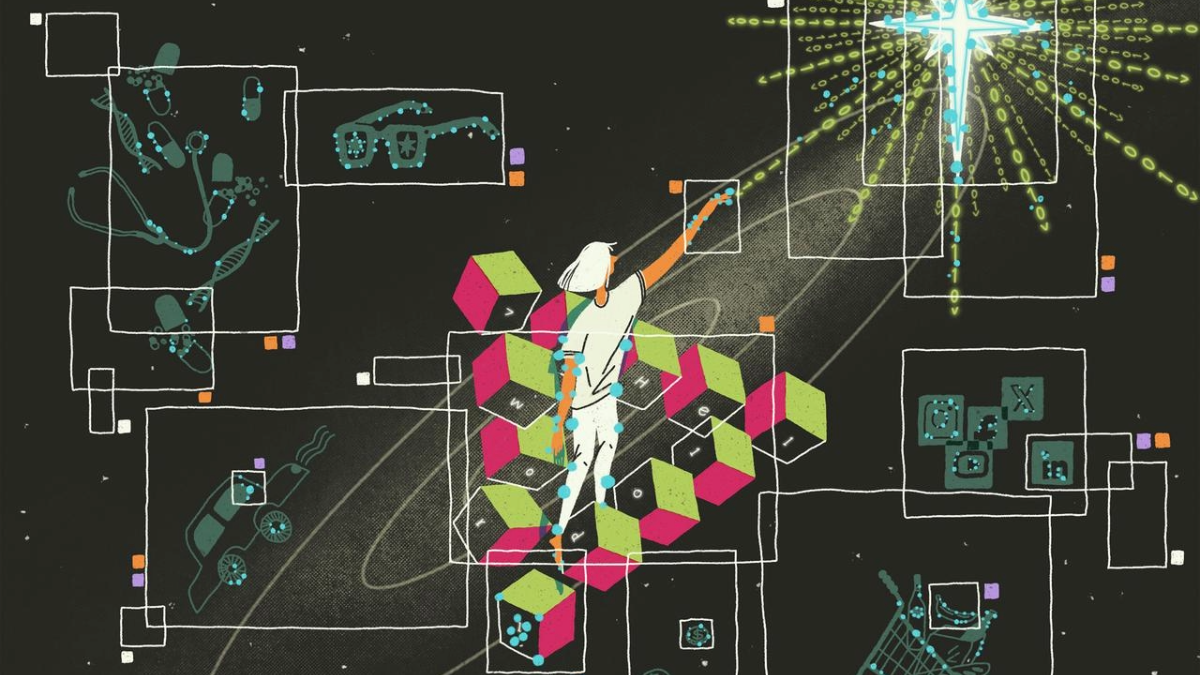
Yutong Liu & The Bigger Picture / Better Images of AI / AI is Everywhere / CC-BY 4.0
A few weeks ago, I reached out to a handful of seasoned digital services practitioners, NGOs, and philanthropies with a simple question: Where are the compelling generative AI (GenAI) use cases in public-sector workflows? I wasn't looking for better search or smarter chatbots. I wanted examples of automation of real public workflows – something genuinely interesting and working. The responses, though numerous, were underwhelming.
That question has gained importance amid a growing number of reports forecasting AI's transformative impact on government. The Alan Turing Institute, for instance, published a rigorous study estimating the potential of AI to help automate over 140 million government transactions in the UK. The Tony Blair Institute also weighed in, suggesting that a substantive portion of public-sector work could be automated. While the report helped bring welcome attention to the issue, its use of GPT-4 to assess task automatability has sparked a healthy discussion about how best to evaluate feasibility. Like other studies in this area, both reports highlight potential – but stop short of demonstrating real service automation.
Without testing technologies in real service environments – where workflows, incentives, and institutional constraints shape outcomes – and grounding each pilot in clear efficiency or well-being metrics, estimates risk becoming abstractions that underestimate feasibility.
This pattern aligns with what Arvind Narayanan and Sayash Kapoor argue in "AI as Normal Technology:" the impact of AI is realized only when methods translate into applications and diffuse through real-world systems. My own review, admittedly non-representative, confirms their call for more empirical work on the innovation-diffusion lag.
In the public sector, the gap between capability and impact is not only wide but also structural.
1. GenAI usage is not distinguished
Much of the current excitement (and funding) targets GenAI, yet when analyses, inventories, and datasets suggest that AI is automating public services, they're often referring to long-standing statistical techniques or rules-based decision trees.
To move this conversation forward, we need to shift from generic "use-case" discussions toward a more task-specific lens. Aligning GenAI use with actual work tasks, such as those codified in frameworks likeO*NET, can help decision-makers better assess feasibility, risk, and oversight needs. A generative model that drafts routine correspondence poses little institutional risk. But using GenAI for eligibility screening or adjudication may introduce accountability and technical demands that exceed the technology's current capacity.
Non-generative approaches may better serve some tasks. This is not because GenAI is incapable, but because other methods may offer better accuracy, cost-benefit, or be required to fulfill more complex requirements. In many cases, the optimal solution may involve combining systems: rules-based for formal decision-making, and GenAI for improved user interface and other time-consuming tasks such as summarization, drafting, or content triage.
2. Automation cases are rarely GenAI
Most automation efforts remain rooted in traditional machine learning or RPA, which often excel in structured, rule-based workflows, but face scalability limits when dealing with probabilistic, less predictable environments. By contrast, GenAI typically appears in lighter-touch roles: summarizing, drafting, translating, and almost always within human-in-the-loop arrangements.
Take France's Albert assistant, a GenAI-powered tool that helps civil servants summarize documents and draft replies. But the final communication is still reviewed and sent by a human. Or Brazil's MARIA, which the Supreme Court uses to support clerks by summarizing legal materials and surfacing relevant precedents.
These are not examples of end-to-end automation but of bounded, assistive use: useful within scope but unlikely to reconfigure underlying processes or institutional logic. However, we should not mistake task-level optimization for structural change in how services operate, particularly if those gains fail to materially improve the lives of typically underserved populations.
3. The RAG trap
Most GenAI deployments leverage some form of retrieval-augmented generation or "RAG”, including question-answering tools, document-search assistants, and internal knowledge bots. These systems pair a language model with institutional data sources to provide more accurate, context-specific responses. They improve information delivery but do not alter workflows or make operational decisions.
Take Utah's State Tax Commission experiment, which handles queries from over 200 call-center agents. The state tested GenAI chatbots grounded in tax law, call transcripts, and training materials. One model matched or outperformed human agents in 92% of test cases. Still, the system remains internal-facing. And intentionally so. As Utah's CIO put it, "the currency of government is trust."
RAG deployments simulate service proximity but don't perform services, as they still can't guarantee accuracy. They rarely trigger back-end processes, update records, or make determinations. After reviewing dozens of cases, I found many GenAI-powered chatbots, but virtually all function as informational tools. Even where information flows improve, the absence of infrastructure for secure execution and core systems integration (e.g., fallback protocols, escalation workflows, agentic infrastructure) means these tools remain isolated from the processes they might otherwise enhance.
Contrary to earlier estimates of AI's transformative potential, examples of end-to-end automation remain rare. India’s Jugalbandi, however, offers a glimpse into what a more integrated deployment might look like. Developed by OpenNyAI, it allows users to open tickets and trigger basic grievance transactions, mostly for social benefits, via voice interfaces.
Still, the gap between automation potential and deployment reality remains wide.
4. Benchmarks and estimates are not deployments
Progress in methods is not the same as progress in applications or diffusion. Even technically capable systems may encounter robustness failures or alignment drift when exposed to real-world complexity. And where technical capability exists, adoption is slow due to regulatory caution, integration complexity, organizational change costs, and public trust concerns.
GenAI is a general-purpose technology, but that doesn't mean it's plug-and-play. Like electricity, its impact requires re-configuring organizations, not just tools. Scoring well on legal exams tells us little about how a system performs inside actual legal workflows.
As noted elsewhere, evaluations must prioritize both construct validity — whether the system performs the intended function — and external validity — whether it generalizes to real-world settings with institutional constraints. But even if a system meets these criteria, a further question remains: Is it the best choice compared to viable alternatives at scale? GenAI may be technically capable, but that does not mean it is the most effective, scalable, or safest solution for the task at hand.
5. Structural and institutional barriers to diffusion
Narayanan and Kapoor point out that the external world imposes a “speed limit on AI innovation.” In the public sector, I should add, that limit isn’t just lower – it’s often enforced with bureaucratic speed bumps, detoured by regulation, and slowed further by institutional inertia. Most governments still don't know how to appropriately design basic informational websites. Despite extensive evidence that SMS, a 32-year-old technology, can improve service delivery, including public health programs, such efforts rarely move beyond pilot stages. This slow pace of diffusion delays the broader social impact that well-designed, low-cost interventions could deliver at scale.
This is what enthusiasts of GenAI struggle to articulate: why would this time be different? And, unless appropriate steps are taken, there are reasons to believe adoption and diffusion might be even slower.
A key issue is that public administration rests on Weberian foundations: rules-based procedures, hierarchies, and systems where officials can be held accountable for their actions. While deterministic for any given input, generative models produce variable outputs, creating a mismatch with bureaucratic design.
Inserting a system that improvises based on statistical likelihood into a rules-based bureaucracy is like replacing a notary with a jazz musician who riffs on each performance. Efficiency may improve, but interpretability suffers, and the locus of accountability becomes uncertain. Recent research on model interpretability offers promising avenues for understanding these systems' internal mechanisms, but challenges remain in making such insights compatible with bureaucratic requirements.
Public agencies are justified in hesitating before entrusting rights and benefits to systems with inherent output variability. Yet, instead of confronting this challenge directly, many deployments introduce GenAI through side channels: chatbots, drafting tools, and vague "assistants."
Another factor is how value is created. In the private sector, novel use cases are the driving force. Firms actively seek ways to leverage general-purpose technologies to create differentiated value. In government, the logic is often inverted. Rather than imagining new services enabled by emerging technologies, governments tend to focus on digitalizing existing processes. This administrative mindset limits innovation to slow, incremental improvements.
Public financial management also typically treats technology as capital expenditure: one-time investments with predictable, upfront costs. In contrast, GenAI usually involves operational expenditure, characterized by ongoing, usage-based costs that scale with implementation. Even when initial investments are necessary, these are best pursued iteratively and co-designed with users, a practice that traditional public procurement systems struggle to accommodate.
In short, the question isn't only whether the technology is ready, but whether the state is, and if not, what can be done about it.
Bridging the gap: from futurology to today's value
But dismissing GenAI altogether risks losing out on real benefits it can already offer.
Instead of asking "What might AI do in five years?", perhaps we should ask "What can AI do today compared to the best available human?" AI decisions should be benchmarked not against assumptions of limitless human expertise and virtue but rather against the "best available human" in that role. A well-tuned GenAI tool may produce more consistent triage results or eligibility checks for a government program than a top performer overwhelmed by caseload. That's the comparator we need, particularly in service contexts where improvements in quality, access, or inclusion translate into greater social impact.
To normalize probabilistic outputs in bureaucracies, consider the transplant analogy: We accept organs that our bodies might otherwise reject because, on balance, the anticipated benefit exceeds the risk associated with inaction: better a risky organ than no organ at all. In the same manner, we must learn to integrate systems that produce variable, yet statistically grounded, results. Rejecting them because they aren't deterministic would be like banning transplants to avoid any chance of rejection.
But if we only measure GenAI against current human performance, we risk missing another opportunity: identifying where GenAI could deliver transformational gains. Instead, we should systematically identify domains where GenAI can deliver the greatest marginal returns, particularly where qualified human support or system capacity is limited.
For instance, a recent GenAI tutoring experiment in Nigeria achieved learning improvements equivalent to nearly two years of typical instruction in just six weeks, surpassing 80% of comparable human-driven interventions. Results like these suggest that GenAI’s most transformative contributions may lie not in automating obvious, repetitive tasks but expanding access to domains where its capabilities, when well-targeted and aligned with public goals, yield the greatest social and developmental returns.
Yet realizing this potential responsibly requires rethinking not only how we deploy AI, but how we govern it.
Finding the right place for GenAI
We humans are less forgiving of machine mistakes than of human ones – a phenomenon known as algorithm aversion. This bias means that high-stakes, end-to-end automation risks provoking public backlash: one AI slip-up can undo months of trust-building, whereas a human error would be more likely to be contextualized and forgiven.
In government, strategic augmentation, where GenAI tools expand who is able to act, enabling, for instance, nurses or other medical professionals to perform tasks once limited to doctors, using systems that match physician-level performance, represents a promising first step. Realizing this potential involves reexamining how professional roles are structured and how tasks are delegated, including the scope of practice boundaries that established practitioners maintain through professional institutions. Updating these frameworks across sectors can support the redesign of public services around technological affordances that target clearly defined problems.
Beyond augmentation, operating within a zone of delegated autonomy, where models function under tightly scoped boundaries and humans remain the final decision-makers once thresholds are triggered, likely represents the most socially acceptable role for GenAI with the greatest returns while preserving appropriate oversight.
Yet, both approaches invite a harder truth: in some contexts, human oversight may not just be unavailable or overburdened, but occasionally counterproductive. In parts of sub-Saharan Africa, for example, research shows frontline providers correctly diagnose common conditions such as diarrhea in fewer than one-third of cases. In many frontline settings — across sectors like health, education, and social protection — the major problem is systemic underperformance or lack of access altogether. In these cases, more autonomous GenAI systems may plausibly improve safety, consistency, and accountability relative to existing options.
This contextual approach might appear to create a tension: cautioning against GenAI in typical government workflows while suggesting value in resource-constrained settings. However, it reflects a principled framework: one that evaluates not just technological or bureaucratic readiness, but also the quality of existing alternatives, oversight capacity, performance gaps, and the consequences of inaction. In traditional bureaucracies, existing systems, however imperfect, provide procedural safeguards that GenAI may disrupt. In severely under-resourced service environments, by contrast, the counterfactual isn’t “adequate but rule-bound bureaucracy,” it’s often “inconsistent delivery or no access at all.”
This isn’t about privileging one domain over another. It’s about recognizing that the risk calculus shifts when the status quo itself causes harm, and that governance must reflect the realities, not just the ideals, of public service delivery.
Where human capacity is limited, relying on idealized models of decision-making can obscure risks that alternative approaches might mitigate. Still, public-sector deployments must carefully assess both typical and worst-case behavior: even when GenAI systems outperform humans on average, rare failures, especially in high-stakes domains, can carry disproportionate consequences, particularly given the algorithm aversion mentioned earlier. This is where delegated autonomy, deploying GenAI under tightly scoped conditions with appropriate safeguards and context-specific thresholds, becomes a viable path.
These realities raise profound governance questions. Unlocking GenAI’s potential in such contexts requires accountability models that go beyond default human-in-the-loop assumptions – frameworks capable of evaluating probabilistic systems on their own terms. But these decisions cannot be left to experts or bureaucrats alone. Governance must draw on the dispersed knowledge of citizens, ensuring that those affected, whether by GenAI’s presence or absence, help shape whether and how it is used.
Moving forward
With a clearer understanding of where GenAI stands today – and why full automation encounters deep technological, institutional, and social headwinds – governments can move from hesitation toward institutional readiness. Real progress will depend not on grand predictions but on careful task selection, technical validation, and adaptive governance. Several recommendations emerge:
- Disaggregate AI types and align them with high-value tasks. Avoid conflating traditional machine learning, RPA, and generative systems when reporting progress or planning roadmaps – each entails distinct trade-offs. For GenAI, focus on tasks with the highest marginal returns in terms of social impact, where cognitive bottlenecks constrain access, quality, or scale.
- Benchmark against realistic human performance. Evaluate GenAI tools by comparing them to the best available human performance under current conditions, not to idealized expectations.
- Prioritize construct-valid experiments. Design pilots grounded in real institutional workflows, with evaluation frameworks that track both service uptake and development outcomes.
- Invest carefully in augmentation, while being realistic about automation. This includes building a second generation of digital public infrastructure that enables a progression from strategic augmentation to delegated autonomy and, eventually, automation, with clear handoff points to human decision-makers, auditability of model behavior, and integration with existing backend systems.
- Rethink governance and accountability. Develop frameworks capable of evaluating and legitimizing probabilistic systems, especially in settings where human oversight may prove to be unavailable, unreliable, or counterproductive. Effective governance must evolve alongside technical alignment efforts, ensuring that institutional safeguards and model behaviors cohere under real-world conditions.
- Ensure citizen-inclusive governance. Create decision-making mechanisms that incorporate dispersed citizen knowledge, ensuring that those affected by both the deployment and absence of AI help shape when and how it is used. Participatory red-teaming, such as structured bug-bounties that invite civic hackers to stress-test models, may also offer a practical way to embed citizen oversight early and complement traditional audits reviews.
- Adapt budgeting and procurement. Shift from one-off capital expenditures to more flexible financial models that match the operational, incremental, and iterative nature of GenAI deployments. Digital marketplaces are a good starting point.
- Enable greenfield innovation, cultivate talent development, and ensure model fitness. Support the creation of entirely new services enabled by GenAI, and build in-house teams capable of evaluating, adapting, and governing these technologies effectively. Foster a downstream ecosystem where smaller models complement larger ones, offering more adaptable and governable options for public-sector use.
- Create ethical, legal, and experimental environments – and share lessons openly. Test new applications in safe, controlled environments with clear fallback mechanisms, while documenting and disseminating both successes and failures.
- Foster collaborative development between governments and model providers. General-purpose models may need to be technically and ethically adapted for specific public-sector use cases. This requires deep cooperation, including adaptation to relevant domain-specific datasets, safety evaluations under real constraints, and shared accountability mechanisms.
Conclusion
This essay began with a simple question: Where are the compelling GenAI use cases in public-sector workflows? The answer is nuanced. While transformative end-to-end automation remains largely aspirational, strategic augmentation and delegated autonomy offer immediate benefits if properly implemented and governed.
Strategic augmentation – enhancing human capabilities rather than replacing them – addresses the bureaucratic mismatch between probabilistic systems and rule-based governance. Delegated autonomy acknowledges that in specific contexts, particularly where human expertise is scarce or inconsistent, GenAI systems with appropriate guardrails may outperform existing alternatives.
GenAI won't transform governments overnight. But with targeted use, adaptive governance, and practical realism, it can help deliver public services that are not only faster and more efficient but also fairer and more inclusive. The most effective governments will likely combine strategic augmentation with delegated autonomy, extending services to the underserved while maintaining clear guardrails and accountability mechanisms.
The real promise isn't automation for its own sake, but a more responsive state that uses technology to close gaps in access, quality, and trust.
The findings, interpretations, and conclusions expressed in this article are entirely those of the author and do not necessarily reflect the views or positions of any institution, its members, or associated counterparts with which the author is, or has been, affiliated.
Authors
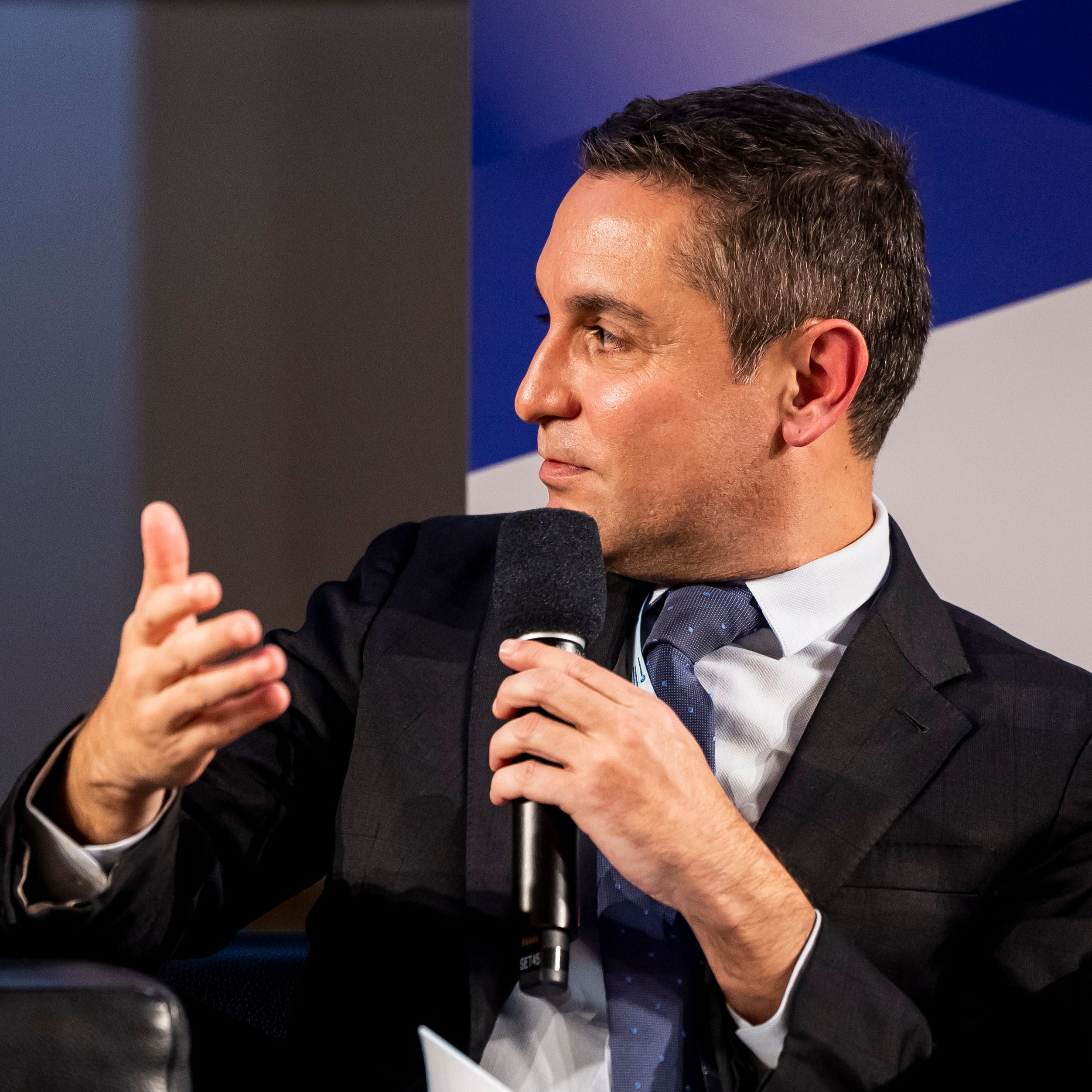