When Algorithms Decide Who is a Target: IDF's use of AI in Gaza
Atay Kozlovski / May 13, 2024In late November 2023, six weeks after the horrific Hamas attacks of the 7th of October, Yuval Abraham of +972 Magazine published a frightening expose article entitled “‘A mass assassination factory’: Inside Israel’s calculated bombing of Gaza”, in which he describes the mass bombing campaign undertaken by the IDF which was aided by the use of novel ‘artificial intelligence’ systems. In his article, Abraham discusses the AI system, ‘Habsora’ (‘The Gospel’), which reportedly enabled the IDF to produce 100 new bombing targets per day for their ongoing campaign in Gaza:
Habsora, explained one of the sources, processes enormous amounts of data that "tens of thousands of intelligence officers could not process," and recommends bombing sites in real time… the use of a system like Habsora makes it possible to locate and attack the homes of relatively junior operatives.
Following up on this first article, in April 2024, Abraham released a far more detailed report discussing two additional systems used by the IDF called 'Lavender' and ‘Where is Daddy?’. This second report, which is based on alleged interviews conducted with insiders who have used these systems in the ongoing war in Gaza, provides far more information on how the IDF designed and implemented these systems within the ‘kill-chain’ - a process which begins with identifying a target for a potential attack, and ends with the attack and elimination of that target.
Of the three AI systems discussed by Abraham, his articles provide the most detailed information on the Lavender system, which is said to be used by the IDF to generate a 'risk score' assessing the likelihood that an individual is a Hamas or Jihad Islamic operative. Abraham describes how Lavender is said to have "clocked as many as 37,000 Palestinians as suspected militants." With this description in mind, this article attempts to provide an ethical analysis of the use of AI recommendation systems in warfare, using the account of Lavender as a case study.
Before I begin, a preliminary comment is in order. The goal of this essay is neither to condemn the IDF – for that I point you to Abraham’s article, which offers a scathing rebuke – nor to corroborate Abraham’s account. If correct, Abraham’s account reveals the destructive manner in which AI has been put to use in the ongoing war in Gaza; if inaccurate, as the IDF has claimed in its response, Abraham’s account offers us a terrifying fictional illustration of how militaries may use such systems in the near future.
The Ethics of Lethal Autonomous Weapon Systems (LAWS)
The discussion over the morality of using AI systems in warfare does not begin with the current war in Gaza. A large-scale international effort to ‘stop killer robots’ has been ongoing since 2013. While the term killer robots may awaken in us the image of Arnold Schwarzenegger in The Terminator or floating 'Sentinels' from The Matrix – in reality, these Lethal Autonomous Weapon Systems (LAWS) have primarily been deployed in the form of drones or loitering munitions that have the technological capability to select and engage targets of opportunity without the interference or supervision of human operators.
The campaign to ban the development and deployment of LAWS has brought to light a variety of risks and ethical concerns. These can be roughly grouped into three categories: (1) The anti-codifiability of morality; (2) responsibility gaps; and (3) dehumanization.
Starting with (1), the anti-codifiability of morality thesis goes back at least to the philosopher John McDowell, who argues that we cannot codify the 'right' moral theory in terms of simple principles and rules such as if-then, and hence no artificial system will ever be able to meet the moral capacity for understanding what the right action is in a given situation. In applying this to the military context, the argument is that war is messy, difficult to understand, and requires judgment and improvisation skills. As a result, we cannot ensure that a LAWS actions will not violate International Humanitarian Laws (IHL).
For example, the two most basic tenets of Jus in Bello are the principles of discrimination and proportionality. Roughly, these mean that before any action is taken in combat, we must ‘discriminate’ between combatants and non-combatants, and ensure that our attack, and the damage caused by it, will be in proportion to the military importance and benefit gained. As one can see, these two principles involve a high degree of vagueness - should a boy holding a kirpan (a ceremonial knife) be considered an armed combatant? What about a young girl reporting soldiers’ positions on a cell phone? Does killing one insurgent justify the 'collateral damage' of five members of his family? These are just a few examples that try to stress the difficulty in ensuring that military action meets the standards of IHL.
Turning to (2), in addition to being unsure whether an AI system could make the necessary distinctions and moral reasoning to arrive at a legal and ethical decision during battle, a significant concern is the question of responsibility for any wrongful or mistaken outcome of an attack. When a soldier commits a war crime or when a commander gives an unlawful order, we know who should be held both morally and legally accountable for that action. However, when an artificial system’s actions have unintended results, we may not be in a position to hold anyone accountable.
This challenge is often referred to as the ‘problem of many hands,’ which claims that because so many actors are involved in designing, regulating, deploying, and maintaining a LAWS, no single actor will have contributed enough to the action to be blamed. If an autonomous suicide drone purposefully crashed into a school bus, killing 20 children, we may feel that someone should be blamed or held accountable for this tragedy, but who? If the system was in perfect maintenance; the designer could not anticipate that the self-learning autonomous system would generate an unforeseen rule that led the system to identify the school bus as a legitimate target. The regulators who deployed the system did so with the knowledge that at testing it performed to a very high degree of safety and accuracy. Legally speaking, perhaps we could arbitrarily determine that some actor is liable for such cases, but morally speaking, we seem to have a blameless tragedy for which no single individual can meet the requirements of control and knowledge necessary for moral blame.
Lastly (3), there have been a variety of claims that focus on dehumanization, which results from allowing an algorithm to mathematically determine who should live and who should die in a given scenario. Advocates against the use of LAWS have argued that there is something profoundly demeaning and disrespectful about allowing algorithms to make lethal decisions. For example, a report from Human Rights Watch states that "Fully autonomous weapons could undermine the principle of dignity… As inanimate machines, fully autonomous weapons could truly comprehend neither the value of individual life nor the significance of its loss." Robert Sparrow makes a similar claim, arguing that in deploying LAWS, "we treat our enemy like vermin, as though they may be exterminated without moral regard at all." These ideas seem to share the underlying intuition that even in war, there are rules that govern what is and what is not morally acceptable and that even our enemies have certain fundamental rights that governments must uphold.
Military Recommendation Systems and Responsibility
Based on the three groups of objections against the use of LAWS, which are laid out above, can a recommendation system like Lavender avoid any of these objections? One of the main objections to LAWS, which appears in each of the three groups discussed above, relates directly to the autonomous nature of the system and to the role, or lack of role, played by a human agent in the decision-making process, or in this case - the 'kill-chain.' As such, a recommendation system does not face this problem in that it does not remove humans from the decision-making loop, and so allows them to retain meaningful control.
Daniele Amoroso and Guglielmo Tamburrini discuss three criteria that must be met in order to achieve this form of control. According to their theory, a human agent must retain the roles of 'fail-safe actor, 'accountability attractor,' and 'moral agency enactor.' A recommendation system seems to allow us to meet each of these three requirements: Because the system merely provides recommendations, the analysts examining the recommendations function as 'fail safe actors' and can reject any output that fails to comport with the requirements of IHL. Similarly, by approving any recommendation, there is a clear line of responsibility that allows different actors to be held accountable for the system's outputs. Lastly, because these systems are semi-autonomous at best, each recommendation goes through the hands of many different humans, with each having the opportunity to utilize their moral agency to ensure that the system is used ethically and responsibly.
Unfortunately, rather than this being the end of a wonderful story about how technological innovation empowers humans to ever-greater achievements, the recent series of whistle-blower interviews on the IDF’s use of AI recommendation systems as part of the war in Gaza offers a stark contrast to this positive depiction. Of the three objections discussed in the previous section, the question of responsibility should be the easiest to resolve. After all, Lavender merely provides recommendations, and the analysts and intelligence officers ostensibly examine, approve, and pass on these recommendations. Thus, if a faulty recommendation is passed along, then those who approve the order should be held accountable. However, the way in which Lavender was used challenges even this seemingly straightforward issue:
During the early stages of the war, the army gave sweeping approval for officers to adopt Lavender's kill lists, with no requirement to thoroughly check why the machine made those choices or to examine the raw intelligence data on which they were based. One source stated that human personnel often served only as a "rubber stamp" for the machine’s decisions, adding that, normally, they would personally devote only about "20 seconds" to each target before authorizing a bombing — just to make sure the Lavender-marked target is male.
Naturally, one could argue that if we cannot hold analysts responsible because they merely 'rubber stamp' or just follow their officer’s instructions, then the responsibility for any bad outcome should fall on the officers who instituted this protocol in the first place. While this seems plausible, Elke Schwartz has raised concerns related to the phenomena of automation bias - the human tendency to unreflectively endorse the output of AI systems. She writes, "Automation bias cedes all authority, including moral authority, to the dispassionate interface of statistical processing… It also removes the human sense of responsibility for computer-produced outcomes." A similar, yet more fundamental concern, goes beyond the lost sense of responsibility and pertains to the complex nature of machine learning-based systems. Abraham quotes from a book written by the current head of the intelligence unit 8200 of the IDF:
"The more information, and the more variety, the better," the commander writes. "Visual information, cellular information, social media connections, battlefield information, phone contacts, photos." While humans select these features at first, the commander continues, over time the machine will come to identify features on its own. This, he says, can enable militaries to create "tens of thousands of targets,"
While no human could realistically sift through such large amounts of data, the more data we introduce and the more parameters used to calculate the relevance and impact of each data point creates what is known in AI research as a 'black box' problem. This kind of problem stems from a high level of complexity in the algorithmic structure, which prevents even the designers of the AI system from fully understanding how or why a specific input leads to a specific output. Without such an explanation, it would not only be difficult to dispute the validity of any recommendation provided by the system, but it may also preclude us from holding any involved actor morally responsible as they would not have access to the necessary information required for questioning the output.
As such, the rubber stamping protocol and the potential Black box nature of the Lavender system make human involvement in the loop virtually irrelevant. To add insult to injury, the reckless reliance on Lavender was done in full awareness that AI systems often contain algorithmic biases and produce false positive categorizations. In the case of Lavender, a source explained:
this lack of supervision was permitted despite internal checks showing that Lavender's calculations were considered accurate only 90 percent of the time; in other words, it was known in advance that 10 percent of the human targets slated for assassination were not members of the Hamas military wing at all.
A 90% success rate may sound good to some people until you put it into more concrete terms. If Lavender identified 37,000 targets for attack, then 10% of those, some 3,700 people, would have been erroneously placed on a kill list.
Are Lavender and Military Recommendation Systems Ethical?
The problem runs deeper than this. Let us assume that, in this case, Lavender was used irresponsibly but that future versions of these systems will be ever more accurate, ultimately leading to a 100% success rate. Would this then solve the problem? This question leads us to the anti-codifiability thesis discussed above. Per Abraham's article:
The Lavender software analyzes information collected on most of the 2.3 million residents of the Gaza Strip through a system of mass surveillance, then assesses and ranks the likelihood that each particular person is active in the military wing of Hamas or PIJ. According to sources, the machine gives almost every single person in Gaza a rating from 1 to 100, expressing how likely it is that they are a militant.
This type of risk assessment has proven to be problematic in other uses of AI systems. For example, researchers found that the American judicial aid system, Compass, was biased against minority groups, assigning them a higher recidivism risk score. In the case of Lavender, we do not know whether there are biases in the system or whether it systematically tends to ascribe a higher risk score to a certain combination of features. What we can say is that one of the sources in the article felt unease about this process:
"How close does a person have to be to Hamas to be [considered by an AI machine to be] affiliated with the organization?" said one source critical of Lavender's inaccuracy. "It’s a vague boundary. Is a person who doesn’t receive a salary from Hamas, but helps them with all sorts of things, a Hamas operative? Is someone who was in Hamas in the past, but is no longer there today, a Hamas operative? Each of these features — characteristics that a machine would flag as suspicious — is inaccurate."
The problem is not merely the in/accuracy of the system, but rather the underlying question regarding which proxies we use to translate an abstract assertion—‘X is a Hammas operative'—into quantifiable parameters. Like any attempt to represent reality in code, we must always be conscious of the adage that 'the map is not the territory'; that the representation of the world by an AI system is merely a representation and not the world itself.
Hanah Arendt famously warned, "The trouble with modern theories of behaviorism is not that they are wrong but that they could become true." Adapting this message to the world of AI, the researcher Brian Christian writes, "The danger… is not so much that our models are false but that they might become true." In determining the criteria for answering the question, 'What is a Hamas operative?', Lavender is not tracking some truth out there in the world but creating it through its algorithm. This should not be misunderstood as claiming that the system is merely spouting random recommendations, it is not. Rather, Lavender creates an illusion of scientific accuracy while, in reality, it is simply, through brute force calculation, regurgitating a simulacrum of the reality that is captured by the data at its disposal.
This brings us to our final point related to the dehumanizing effect of 'mere' recommendation systems like Lavender on both the decision-making process and the targeted victims. Abraham shows how the use of Lavender enabled the IDF to de-humanize the decision-making process by effectively removing humans from any meaningful control over the system. He writes, "...sources said that if Lavender decided an individual was a militant in Hamas, they were essentially asked to treat that as an order..."
But could they perhaps have treated the recommendation differently? As a starting point for an investigation? In theory, yes, but in practice doubtful. Beyond everything explained above, the automation bias, the lack of explanation for the outputs, the unknown internal algorithmic biases, etc, there is the claim with which we started this essay - that systems like Lavender and 'The Gospel' do the work of thousands of trained human specialists. This statement is both telling and dangerously misleading. It is telling in that it crystallizes why these systems were designed in the first place.
Abraham, quoting again from a book written by the current head of the intelligence unit 8200 of the IDF, writes that the commander "advocates for such a system without referencing Lavender by name...Describing human personnel as a "bottleneck" that limits the army’s capacity during a military operation." He adds:
the commander laments: "We [humans] cannot process so much information. It doesn’t matter how many people you have tasked to produce targets during the war — you still cannot produce enough targets per day."
Lavender was designed and implemented out of a belief that humans are the 'bottleneck' that needs to be resolved. However, Lavender is not an amalgamation of thousands of human beings; it does not replicate and multiply the ideal human analyst. It merely gives us this illusion while, in reality, it removes the last vestiges of humanity from war. Lavender is not a mere tool that militaries can use in different ways and for different purposes; it is value-laden and promotes a very specific worldview - one in which war is fought at scale and human judgment is relegated to the sidelines.
In addition, and of much greater concern on the victim’s side, the dehumanization process entails treating these real-life human beings as mere numbers or statistics. As Abraham writes:
"Mistakes were treated statistically," said a source who used Lavender. "Because of the scope and magnitude, the protocol was that even if you don’t know for sure that the machine is right, you know that statistically it’s fine. So you go for it."
Moreover, sources "described a similar system for calculating collateral damage...[where] the software calculated the number of civilians residing in each home before the war… and then reduced those numbers by the proportion of residents who supposedly evacuated the neighborhood…" "As such, the source said: 'the collateral damage calculation was completely automatic and statistical' — even producing figures that were not whole numbers."
The moral bankruptcy of relying on such systems cannot be overstated: there is no such thing as half a person - every human life is precious and worthy of dignity and respect.
People often speak of technology as a tool that can be used for good or bad. Lavender is not merely a neutral tool; its very existence shapes a reality in which life and death are decided by mathematical calculation. While we should be deeply concerned about the development and deployment of lethal autonomous weapon systems, it seems that the campaign to stop killer robots may have misjudged which type of AI system poses the more immediate threat. Lavender shows us that our concern should not be primarily about the system's level of autonomy, but how and whether we, as human agents, can maintain a meaningful form of control over our weapons of war.
Authors
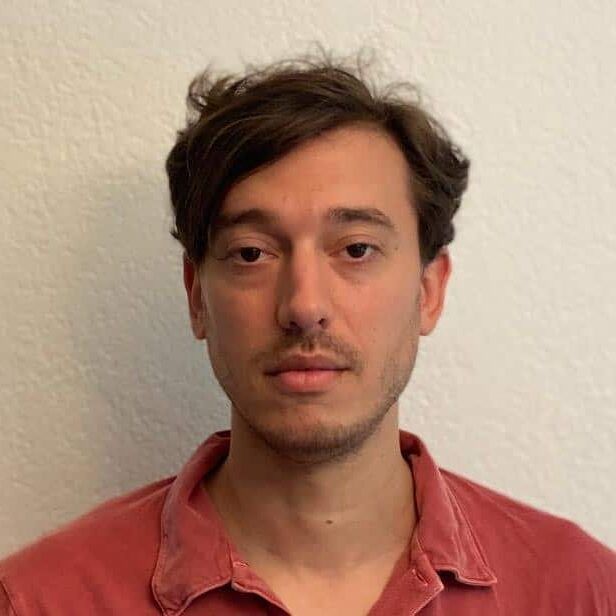