What Will “Amplification” Mean in Court?
Jonathan Stray, Luke Thorburn, Priyanjana Bengani / May 19, 2022Luke Thorburn is a doctoral researcher in safe and trusted AI at King's College London; Jonathan Stray is a Senior Scientist at The Center for Human-Compatible Artificial Intelligence (CHAI), Berkeley; and Priyanjana Bengani is a Senior Research Fellow at the Tow Center for Digital Journalism at Columbia University.
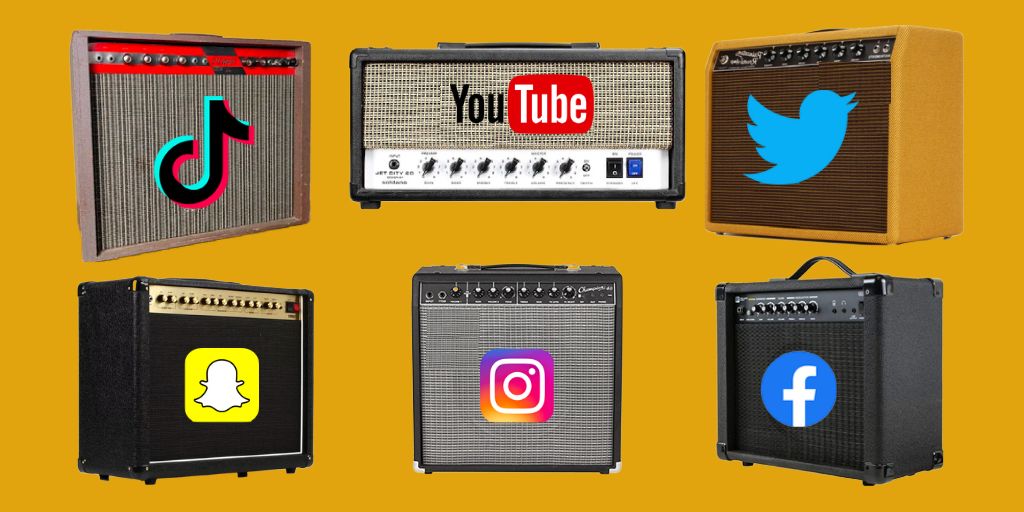
“Amplification” is a common term in discussions about how best to manage and regulate social media platforms, and is starting to appear in proposed laws. We’re not convinced this is a good idea, because the meaning of this word is not always clear. Social media surely does “amplify” user contentin the sense that anyone, anywhere can “go viral” and find themselves with a massive audience, but this intuitive notion hides a range of very complex questions.
The democratization of reach has profoundly benefited many people, but viral information cascades can also do harm in a variety of ways. On social media, human users amplify each other: information can spread rapidly through messaging apps such as WhatsApp and Telegram, and even journalists are understood to “amplify” certain issues or ideas just by reporting on them. So amplification doesn’t require any sort of algorithmic process, but many platforms incorporate recommender systems and other algorithms that filter and personalize information, including social media, news apps, streaming video, online shopping, and so on. In these systems, information spreads through complex interactions between people and software. While it can be hard to say if an algorithm “caused” the distribution of some item, it is certainly worth asking if algorithmic changes might lead to a healthier information ecosystem.
Lawmakers are increasingly focused on this question. There are several proposed laws that use the term “amplification” when considering harms from algorithmic information filtering, such as the Justice Against Malicious Algorithms and DISCOURSE Acts in the US, and the Digital Services Act in the EU. However, none of these drafts provide a precise definition for amplification. Further, most discussions of amplification concern social media platforms, and make much less sense for other types of recommender-driven platforms such as news aggregators, online shopping, or podcast discovery.
Definitions for key terms in laws are sometimes deliberately omitted, so that judges can exercise reasonable discretion in how they are applied, while respecting the intention behind the law. But in the case of “amplification,” the intent behind the term is often unclear, and attempts to define amplification have emphasized different effects. These include the absolute reach of an item of content, its reach relative to some counterfactual scenario, and a lack of transparency or control over whether or not an item is presented to a user.
We argue that the term “amplification” may be confusing rather than clarifying in discussions of recommender systems, and is certainly not precise or unambiguous enough to be used in law – especially law that may relate to fundamental speech rights. Reviewing the three most common interpretations, we speculate on how a judge might decide whether amplification has occurred, describing the definitional and methodological challenges they would face. These turn out to be significant, so we suggest several alternative ways of thinking about algorithmic information spread that might help people more easily say what they mean, or perhaps discover the implications of what they are already saying.
Three Interpretations
Put yourself in the shoes of a judge who is presented with a case that hinges on whether particular content has been “amplified” on a particular platform. For example, the content might be defamatory, or consist of illegal hate speech or incitement to violence. As the judge, you have access to internal platform data. How do you decide if amplification has occurred?
We reviewed a variety of proposed definitions of amplification, and asked a number of people to explain their use of the term. There seem to be more or less three different ideas that are commonly held.
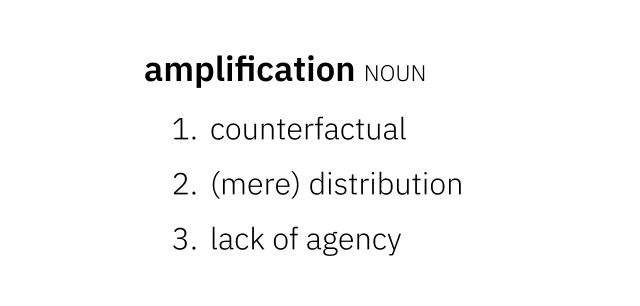
1. Amplification as counterfactual
The first interpretation is prompted by an obvious question: amplified relative to what? To say that something was “algorithmically amplified” means that if the platform had been designed differently, the content would not have been disseminated so widely. How widely would that have been? This notion of amplification requires us to articulate a counterfactual scenario, then compare the real and the imagined reach.
Sometimes this is plausible. When Twitter switched to an algorithmic “home” timeline in 2016, they kept 1% of their users on the chronological timeline. This provided a natural point of comparison for a recent study of the amplification of politics on Twitter. The researchers defined an “amplification ratio,” which quantifies the reach of content in one system relative to another:
We define the amplification ratio of set T of tweets in an audience U as the ratio of the reach of T in U intersected with the treatment [algorithmic] group and the reach of T in U intersected with the control [chronological] group.
This is a good example of the level of detail required to unambiguously define “amplification,” and is a reasonable definition in the context of Twitter. However, this definition doesn’t generalize to other cases and platforms.
In this study the “set T of tweets” came from accounts belonging to politicians in seven countries. The proposed Health Misinformation Act addresses a much more complicated class of items. Categories such as “health misinformation,” “violent extremism,” or “defamatory material” are hard to define precisely, but the measurement of amplification would first require classification of every item on the platform. There is an additional challenge of deciding how to aggregate outcomes for all such items. The authors of the Twitter study are upfront about the fact that methodological choices can influence findings, writing that
comparing political parties on the basis of aggregate amplification of the entire party … or on the basis of individual amplification of their members … leads to seemingly different conclusions: While individual amplification is not associated with party membership, the aggregate group amplification may be different for each party.
A deeper problem is that there isn’t always a clear baseline scenario or control group. While some have advocated for social media with “no algorithm,” what they usually mean is a simple reverse-chronological list of posts. This makes the most sense on platforms like Twitter, Instagram, or TikTok where there is a central, algorithmically-ranked feed, and a clear subset of content relevant to the user (the accounts they follow). This idea is less clear on platforms like Facebook where there are many different types of content and the user interface is less oriented around “following.” Designing a single “chronological” feed requires many detailed decisions such as whether it should include posts from groups the user has joined, or posts their friends have commented on. A chronological baseline is even less meaningful for platforms like Spotify, YouTube, or Google News, where a true chronological feed of every new item would be essentially useless.
Even where purely reverse-chronological rankings are possible, they are not neutral — they reward accounts that post more frequently, have no defense against spam, and can have higher proportions of “borderline” content compared to rankings generated by more sophisticated algorithms. In some contexts there may be other plausible baselines, such as a standard recommendation algorithm from a relevant textbook, or a previous version of the algorithm used by the platform in question. None of these options is an obviously natural or impartial point of comparison.
Then there is the problem of actually tallying the counterfactual. In the Twitter study, the authors had access to real data from the baseline scenario because Twitter kept 1% of users on a reverse-chronological timeline. But this is the exception. In general, the counterfactual scenario would need to be simulated to generate a quantitative estimate of the amount of amplification. The process would be further complicated by questions of who performs the simulation (the platform? a third party?), how to model user behavior in the counterfactual (people change their posting behavior when algorithms change), and how to assess the credibility and statistical significance of the calculated difference.
A judge would also need to decide how to measure distribution or exposure. Should they count people seeing the headline? Clicking a link to the article? Sharing the article? Should they consider whether or not it was seen over a wide geographic area? What if the same number of people saw an item as compared to baseline, but it was positioned more saliently? Or they saw it more frequently? Whatever the metric, there would also need to be a threshold for what counts as amplification. For example, you might decide “the defamatory article will be said to be amplified if it is seen by >10% more people relative to the baseline scenario.”
In short, there are a huge number of degrees of freedom in defining amplification relative to “what might have been,” and actually estimating an amplification figure can be a complicated exercise in counterfactual simulation. Perhaps with access to relevant expertise and resources, courts could rule on “amplification” decisions despite these subtleties. Unfortunately, researchers who conduct such studies often work for a social media platform or collaborate closely with people who do, which may limit their independence as expert witnesses. These ambiguities and difficulties could create confusion and undermine confidence in legal decisions involving speech and expression.
2. Amplification as (mere) distribution
Sometimes there doesn't seem to be any semantic difference between the claim that certain content was “amplified” and the claim that the content was “shown.” For example, this glossary compiled by misinformation researchers states that
Manufactured Amplification occurs when the reach or spread of information is boosted through artificial means.
If “manufactured amplification” is “reach or spread” by “artificial” means, then “amplification” seems to be just “reach or spread”. More complex definitions also frequently turn out to be equivalent to mere distribution. For example, this policy paper defines amplification as
the promotion of certain types of content … at the expense of more moderate viewpoints.
while the proposed JAMA Act considers
the material enhancement, using a personalized algorithm, of the prominence of such information with respect to other information.
The phrases “at the expense of more moderate viewpoints” and “with respect to other information” are redundant because there are a limited number of items on a page of recommendations, and ultimately because there are only so many minutes of human attention in a day. This zero-sum nature means that selection of any type of content will inevitably come at the expense of all other types of content. In this context, it is difficult to see how amplification can be distinguished from distribution, or for that matter from human editorial discretion.
Collapsing amplification to distribution or discretion would lead to very broad laws, because it would be equivalent to making certain types of content illegal. Would a platform be liable for “amplification” if they merely failed to suppress particular items? This could even prevent algorithmic distribution of accurate information on important topics such as public health misinformation or the incitement of political violence; the BBC already acknowledges that it unavoidably amplifies ideas by reporting on them. Legally and ethically, a law that treats amplification as distribution might be in violation of human rights principles on free expression, and in the US, could be unconstitutional due to the First Amendment. Both judges and legal scholars are skeptical that a law seeking to restrict amplification could avoid this.
Some versions of amplification law would be flatly unconstitutional in the U.S., and face serious hurdles based on human or fundamental rights law in other countries. Others might have a narrow path to constitutionality, but would require a lot more work than anyone has put into them so far. Perhaps after doing that work, we will arrive at wise and nuanced laws regulating amplification. For now, I am largely a skeptic.
— Daphne Keller, Amplification and Its Discontents (2021)
3. Amplification as lack of agency
In the third main interpretation we found, amplification occurs when a user is shown an item of content that they “didn’t ask for” or when the reasons for it being shown are opaque. Amplification is linked to a lack of user agency or control over the information to which they are exposed. The proposed Protecting Americans from Dangerous Algorithms Act takes this approach, exempting platforms from liability if their content is
(I) … ranked, ordered, promoted, recommended, amplified, or similarly altered in a way that is obvious, understandable, and transparent to a reasonable user based only on the delivery or display of the information (without the need to reference the terms of service or any other agreement), including sorting information—
(a) chronologically or reverse chronologically;
(b) by average user rating or number of user reviews;
(c) alphabetically;
(d) randomly; and
(e) by views, downloads, or a similar usage metric; or
(II) the algorithm, model, or other computational process is used for information a user specifically searches for.
This has intuitive appeal: many recommenders are black boxes, and ranking decisions can seem arbitrary. In contrast, reverse-chronological feeds or Reddit-style voting algorithms are “explainable”. But there are problems.
It’s not always clear what user intent was, or what it means to “specifically search for” something. If a user searches for “politics” and is subsequently offered some items that discuss popular conspiracy theories about politicians, did they intend to see those? Also, recommender systems are designed to allow users to delegate curatorial responsibility so as to avoid having to explicitly ask for each item. Some recommenders (such as those for news articles) are valuable precisely because they show you things you didn’t know to ask for. How would a judge draw the line between items that were “asked for” and those that were not?
The second problem relates to what counts as “obvious, understandable, and transparent.” While some algorithms are certainly easier to understand than others, explaining platform algorithms doesn’t necessarily explain platform outcomes. These are the result of complex interactions between automated systems, editorial or managerial decisions, and the online behavior of large numbers of people, and may not be explainable without detailed traces of user activity. Further, users are strategic: they will quickly learn, adapt, and attempt to exploit any distribution algorithm. User behavior thus has large effects on information distribution that are difficult to separate from algorithm design. For example, it is possible that the different social media strategies of major political parties might explain disparities in how left- and right-leaning content is amplified on Twitter.
There is also the possibility that agency might be undermined when items are recommended because of inferences made from the user’s personal data, which may have been collected or used without their fully informed consent. The JAMA Act would make platforms liable for harm from amplification that “relies on information specific to an individual”. As worded, this would appear to include almost all recommendation algorithms. Even a simple subscription-based, reverse chronological timeline relies on information about which accounts each user followed. If this bill was not intended to penalize explicit following, then judges will have to invent some criteria for determining when user data becomes “specific to an individual.”
Alternatives to “Amplification”
The term amplification is ambiguous, but information can spread through online networks in a way that appears different in degree, if not in kind, from what was possible prior to the Internet. Most distinctively, content from otherwise inconspicuous citizens can “go viral”, as a burst of engagement triggers large scale information cascades. There is a case for updating the way platforms, media, and online communications are regulated to keep pace with changes in information and societal dynamics.
Ultimately, we hope that those concerned with these matters will be clearer about what their goals are when they talk about regulating the amplification of content, crafting language and policy options that clearly reflect that intent. We are not lawyers, so we are not going to try to parse which goals and formulations might be permissible under any particular constitutional regime – others have attempted that. Instead we’ll propose plain-language interpretations of the above ideas that we hope are forthright and clarifying.
For instance, is the goal in diminishing amplification to suppress certain kinds of information or content? Stated this way, the potential threat to fundamental rights is obvious. Instead of X is amplified, perhaps you mean X is harmful when viewed, to a degree that outweighs certain speech rights, and additionally something like
- X has reach Y as measured by metric Z
- X had Y% greater reach in system U than it would have had under system V
- Y users liked X so it was algorithmically selected for Z additional users
Policy options that promote this goal include mandating “circuit breakers” that add friction to sharing functionality or applying additional moderation scrutiny to content that spreads beyond certain numerical limits. Circuit breakers could be content-neutral or narrowly tailored to target particular types of speech. A more unusual proposal focuses on people rather than algorithms, by making superspreaders of harmful content liable for the actions of their followers.
Is the goal to give users more control? Instead of X is amplified, you might say
- the user did not intend/want/ask to see X
- the user did not have control over whether X was shown to them
These require some sort of definition of “ask” and “control” before they can be clear. Regulatory models include mandating a reverse-chronological ranking option, platform interoperability, enabling third party content ranking middleware (a competition-based approach), or user control over how their personal data is used to target content (a privacy-based approach).
Is the goal to make the reasons content is shown more transparent? Instead of X is amplified, you could mean
- the user doesn’t understand why X was shown to them
- the reasons why X was shown are not disclosed
In this case, regulatory models include mandating truth and transparency in advertising, requiring users to use their true identities, requiring that recommendation algorithms provide explanations, or establishing a new regulatory body to set transparency standards for online platforms (all consumer protection-based approaches).
- - -
There is much at stake when regulating human expression, and the algorithmic systems that propagate it. To avoid unintended consequences, we need to think through the language we use when discussing and responding to the challenges of algorithmic media, especially when that language becomes law. Because it can mean so many different things, the word “amplification” may ultimately confuse more than it clarifies.
Authors
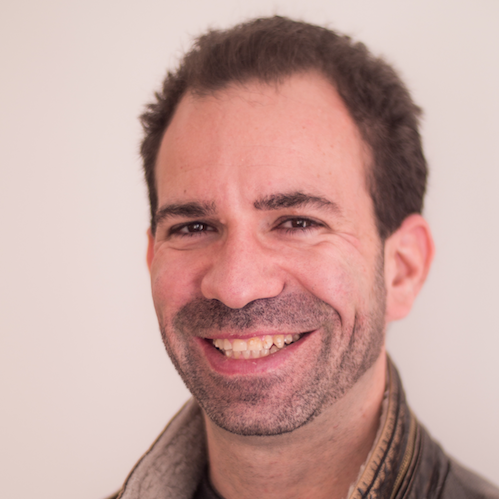
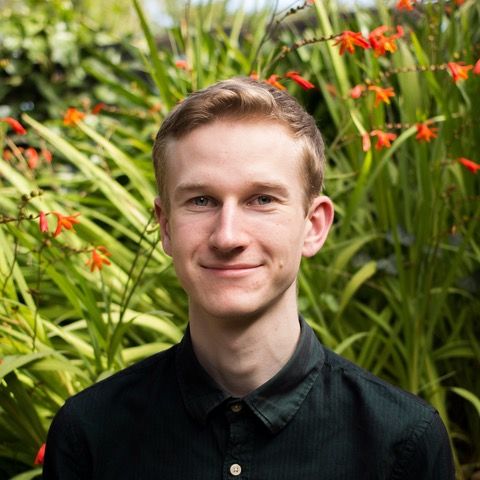
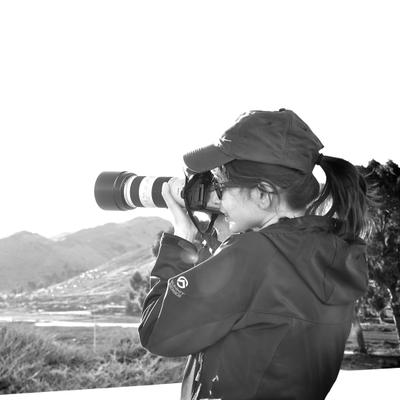