What Are We Building, and Why?
Justin Hendrix / Dec 17, 2023Audio of this conversation is available via your favorite podcast service.
At the end of this year in which the hype around artificial intelligence seemed to increase in volume with each passing week, it’s worth stepping back and asking whether we need to slow down and put just as much effort into questions about what it is we are building and why.
In today’s episode, we’re going to hear from two researchers at two different points in their careers who spend their days grappling with questions about how we can develop systems and modes of thinking about systems that lead to more just and equitable outcomes, and that preserve our humanity and the planet:
- Dr. Batya Friedman is a Professor in the Information School and holds adjunct appointments in the Paul G. Allen School of Computer Science & Engineering, the School of Law, and the Department of Human Centered Design and Engineering at the University of Washington, where she co-directs the Value Sensitive Design Lab and the UW Tech Policy Lab.
- Dr. Aylin Caliskan is an Assistant Professor in the Information School at the Paul G. Allen School of Computer Science & Engineering, is an affiliate of the UW Tech Policy Lab, part of the Responsible AI Systems and Experiences Center, the NLP Group, and the Value Sensitive Design Lab. She is also co-director elect for the Tech Policy Lab, a role she will assume when Dr. Friedman retires from the university.
What follows is a lightly edited transcript of the discussion.
Batya Friedman:
I'm Batya Friedman. I'm in the Information School at the University of Washington, a professor there and I co-direct both the value sensitive design lab and also the UW Tech Policy Lab.
Aylin Caliskan:
I am Aylin Caliskan and I'm an assistant professor at the Information School. I am an affiliate of the Tech Policy Lab right now. I am also part of the Responsible AI Systems and Experiences Center, the Natural Language Processing Group, as well as the Value Sensitive Design Lab.
Batya Friedman:
Aylin is also the co-director-elect for the Tech Policy Lab. As I am winding down on my career and stepping away from the university, Aylin is stepping in and will be taking up that pillar.
Justin Hendrix:
And we have a peculiar opportunity during this conversation to essentially talk about that transition, talk a little bit about what you have learned, and also to look at how the field has evolved as you make this transition and into retirement and turn over the reins as it were.
But Dr. Friedman, I want to start with you and just perhaps for my listeners, if they're not familiar with your career and research, just ask you for a few highlights from your career, how your work has influenced the field of AI bias and consideration around design and values and technological systems. What do you consider your most impactful contribution over these last decades?
Batya Friedman:
Well, I think one clear contribution was a piece of work that I did with Helen Nissenbaum back in the mid-nineties. Actually, we probably began in the very early nineties on bias and information computing systems published in 1996, and at that time I think we were probably a little bit all by ourselves working on that. I think that the journal didn't quite know what to do with it at the time, and that's a paper that if you look at the trajectory of its citations, it had a very slow uptake. And then I think as computing systems have spread in society over the last five to seven years, we've seen just an enormous reference back and from another sense of impact of the work I've done, which is not just around bias but around human values more generally and how to account for those in our technical work. Just as one example of evidence of impact and the Microsoft responsible AI work and impact assessments that they published within the last year, they acknowledge heavily drawing on value-sensitive design and its entire framework in the work that they've done.
Justin Hendrix:
I want to ask you just to maybe reflect on that work with Helen Nissenbaum for a moment, and some of the questions that you were asking, what is a biased computer system? Your examples started off with a look at perhaps the way that flight reservation systems work. Can you kind of cast us back to some of the problems that you wanted to explore and the way that you were able to define the problem in this kind of pre-web moment?
Batya Friedman:
Well, we were looking already at that time at ways in which information systems were beginning to diffuse across society and we were beginning to think about which of those were visible to people and which of those were in some sense invisible because they were hidden in the technical code. In the case of airline reservation systems, this has to do with what shows up on the screen. And you can imagine too that algorithms, that technical algorithms where someone is making a technical decision. I have a big database of people who need organs and everyone in the database is stored in alphabetical order. I need to display some of those. And so it's just an easy technical decision to start at the beginning of the list and put those names up on the screen. The challenge comes when you have human beings and the way human beings work is once we find a match, we're kind of done.
So you sure wish in that environment if you needed an organ, your last name started with an A and not a Z. So it's starting to look at that and trying to sort out where are the sources of bias coming from, which are the ones that already pre-exist in society like redlining, which we're simply embedding into the technology. We're almost bringing them over, which of them are coming from just making good technical choices without taking context into account. But then once you embed that in a social environment, bias may emerge. And then also starting to think about systems that given the environment they were developed for, may do a reasonable job managing bias that's never perfect. But then when you use them with a very different population, a very different context, different cultural assumptions, then you see what emerges or bias. And so at that time we identified these three broad sources for bias and systems. So pre-existing social bias, technical bias from just technical decisions, and then this category of emergent bias. And those categories have stood the test of time. So that was way back in the mid-nineties and I think they're still quite relevant and quite helpful to people working say in generative AI systems today.
Justin Hendrix:
That perhaps offers me the opportunity to ask Dr. Caliskan a question about your work, and maybe it's a compound question, which is to describe the work that you've been doing around AI bias and some of the work you've done looking specifically at translation engines. How do you see the frameworks, the ideas that come from Dr. Friedman's work sort of informing the research you're doing today and where do you see it going in future?
Aylin Caliskan:
This is a great question. In 2015, '16 was frequently using translation systems, statistical machine translation systems, and I kept noticing bias translation patterns. For example, one of my native languages is Turkish, and Turkish is a gender-neutral language. There is a pronoun all meaning he, she, it or they. And my other native language is Bulgarian and it's a grammatically gendered language, more gendered than English, and it has the Cyrillic alphabet. So I would frequently use translation to text my family, for example, in Bulgaria. And when I was translating sentences such as O bir doktor and O bir hemşire meaning he or she is a doctor, he or she is a nurse. The outcomes from translation systems were consistently, he's a doctor, she's a nurse. And then we wanted to understand what is happening with natural language processing systems that are trained on large-scale language corpora and why they are exhibiting bias in decision-making processes such as machine translation generating outputs.
And we couldn't find any related work or any empirical studies except Batya's work from 1996 bias in computer systems. And then we decided to look into this in greater detail as especially language technology started becoming very widely used since their performance was improving. Even all the developments we have in artificial intelligence computing and information systems and then studying the representations in the language domain, which you can think of as natural language processing models and the way they perceive the world, the way they perceive language. I've found out that perception is biased when it comes to certain concepts or certain social groups. For example, certain names that might be more representative of underrepresented groups or historically disadvantaged groups were closer in the representational space to more disadvantaging words versus historically dominant groups representation or words that are related to them were closer in the representational space mathematically to more positive words.
And then we developed a principled and generalizable method to empirically study bias in computer systems to find out that large-scale language corpora are a source of implicit biases that have been documented in social cognition in society for decades. And these systems that are trained on large-scale sociocultural data, embed the biases that are inhuman produced data reflecting systemic inequities, historically disadvantaging data and biases related to the categories Batya mentioned. And over years, we have shown that this generalizes to artificial intelligence systems that are trained on large-scale sociocultural data because large-scale sociocultural data is a reflection of society, which is not perfect. And AI systems learn these reflections in imperfect ways, adding their own, for example, emergent biases and associations as well. And since then I have been focusing on this topic, and it is a great coincidence that the person that contributed foundational work in this area is at the same school as I am.
Justin Hendrix:
Dr. Friedman, when you think about this trajectory of the initial foundational work that you were doing close to 30 years ago and the work that's just been described, do you think about this sort of trajectory of where we've got to in both our understanding of these issues and perhaps also our societal response or the industry response or even the policy response? Do you think we've really made progress? I mean, this question around bias and AI systems bias and technological systems generally it's better understood, but to some extent, I don't have exact numbers on this, it seems like a bigger problem today perhaps than it's ever been. Is that just a sort of function of the growth of the role of technology in so many aspects of society?
Batya Friedman:
Well, one, at a certain point just has to say yes to that, right? Because if we had, as was predicted many years ago, only five computers in the world and it wasn't used in very many sectors of society, then I don't think we would be as concerned. So certainly the pervasive, widespread, pervasive uptake is part of the motivation behind the concern. I think an interesting question here to ask is in what ways can we hold ourselves accountable, both as technologists and also as members of society and governments and private sector for being alert and checking these issues as they emerge? So for example, I talked about a situation where you have a database, everybody's in alphabetical order, and then you display that data in alphabetical order on a screen that can only list 20 names at a time. We know that's problematic. And initially, we didn't know that was problematic.
So if you did that say 30 years ago, there would be unfortunate biases that would result and it was problematic. But now that we know that's a problem, I would say any engineer who builds a system in that way should be held accountable, that would be actually negligent. And this is how we have worked in engineering for a long time, which is as we develop systems, as we gain experience with our methods and techniques, what we consider to be a best practice changes. And the same is true say for building reliable systems or correct systems. We can't build a reliable or correct system, a fully reliable or correct system yet we still hold that out as an ideal. And we have methods that we hold ourselves accountable to. And then if we have failures, we look to see if those methods were used and if they were, then we try to mitigate the harms but we don't cry negligence. And I think the same things can apply here.
So then to your question, I would say we have a lot less experience at this moment in time with understanding what are the methods that can really help us identify these biases early on and in what ways do we need to remain alert? How can we diagnose these things? How can we mitigate them as they unfold? We know that many of these things we will be able to identify or see in advance. So some we can, but other things are going to unfold as people take up these systems. And so we know that our processes also need to engage with systems as they're being deployed in society. And so that's a real... In some ways, a shift in terms of how we, at least with computational systems, how we think about our responsibilities towards them. If I were talking about building bridges, you would say, oh yes, of course you need a maintenance plan. You need people examining the bridge once a year to see if there are new cracks, mitigating those cracks when they happen. So we know how to do this as engineers with other kinds of materials. We're less experienced with digital materials.
Justin Hendrix:
We are though seeing a sort of industry pop up around some of these ideas, folks who are running consultancies, building technological tools, et cetera, to deliver on the ability to investigate various systems for bias. Some of that's being driven by laws. I'm sitting in New York City, there's a law around bias and automated employment decisions that's recently come into effect for instance, what do you make of that? What do you think of the kind of, I suppose, commercialization of some of your ideas?
Batya Friedman:
Let's go back to building bridges or I spent a lot of time actually in the Bay Area, so I'm familiar with earthquakes. And thinking by analogy, if we can build a building, if I use the very best techniques we know and it can withstand a 6.0 Earthquake, and we have a 3.0 Earthquake and the building collapses, then I'm going to be looking at, well, what were the processes that were used? And I'm going to make that cry of negligence and I have a sense of standards and the people who are doing the work are going to be held accountable. If on the other hand it was a 9.0 earthquake, and we actually don't know how to build for that, we're going to consider that a tragedy, but we aren't going to say that the engineers did a poor job. So I think one of the first things we need to be able to do is take an honest look at where we are with respect to our methods and techniques and best practices.
I think we're at the very beginning. Like anything, we will only get good at something if we work really hard at it. So I think that we need to be investing a lot of our resources in how to develop better methods for identifying or anticipating biases early on and techniques for having ways in which those can be reported and ways in which they can then be mitigated. And those techniques and processes need to take into account not just those people who are technically savvy and have access to technology, but to recognize that people who may never even put their hands on the technology might be significantly affected by biases in the system and that they need ways that are perhaps non-technical ways of communicating harms that they're experiencing and then having those be taken up seriously and addressed. So I would say that we're at the very beginning of learning how to do that.
I would also observe that many of the resources are coming from certain places and those places and people who are making those decisions have certain interests. And so can we impartially look at those things so that we take a more broader swath of the stakeholders who are impacted so that when we start to identify where and how the stakeholders need to be accounted for and where and how the resources are being allocated to ensure we develop methods that will account for these stakeholders that there's something even-handed happening there. So a lot of this is about process and a lot of the process that I've just sketched is fundamental to value-sensitive design, which is really how do we foreground these values and use them to drive the way in which we do our engineering work, or in this case we view policy as a form of technology. So one moves forward on the technical aspects and the policy regulatory aspects as a whole, and that broadens your design space. So to your question, I would say we're at the very beginning and a really critical question to ask is those forces that are moving forward, are they themselves representing too narrow a slice of society and how might we broaden there? How do we do that first assessment? And then how might we broaden that in an even-handed manner?
Justin Hendrix:
Dr. Caliskan, can I ask you, as you think about some of the things that are in the head lines today, some of the technologies that are super hot at the moment, large language models, generative AI more broadly, is there anything inherent perhaps in those technologies that makes looking for bias or even having some of these considerations any more difficult? There's lots of talk about the challenges of explainability, the black box nature of some of these models, the lack of transparency and training data, all the sorts of problems that would seem to make it more difficult to be certain that we're following the kinds of best practices that Dr. Friedman just discussed.
Aylin Caliskan:
This is a great question. Dr. Friedman just mentioned that we are trying to understand the landscape of risks and harms here. These are new technologies that became recently very popular. They've reached the public recently, although they have been developed for decades now. And in the past we have been looking at more traditional use cases based on, for example, decision-making systems. For example, in college admissions, resume screening, employment decisions or representational harms that manifest directly in the outputs of AI systems. But right now, the most widely used generative AI systems are typically offered by just a few companies. And they have the data about what these systems are being used. And since many of them are considered general-purpose AI systems, people might be using them for all kinds of purposes to automate mundane tasks to collaborate with AI. However, we do not have information about these use cases and such information might be proprietary and it might benefit with market decisions in certain cases, but without understanding how exactly these generative AI systems are being used by millions if not billions of people, we cannot trivially evaluate potential harms and risks.
We need to understand the landscape better so that we can develop evaluation methods to measure these systems that are not transparent, that are not easy to interpret. And once we understand how society is co-evolving with these systems, we can develop methods not just to measure things and evaluate potential harms, but also think about better ways to mitigate these problems that are socio-technical where technical solutions by themselves are not sufficient, and we need regulatory approaches in this space as well as raising public awareness as Dr. Friedman mentioned. Stakeholders, users, how can they understand how these systems might be impacting them when they are using them for trivial tests? What kinds of short-term and long-term harms might they experience? So we need a holistic approach to understand where these systems are deployed, how they are being used, how to measure them, and what can be done to understand and mitigate the harms.
Batya Friedman:
So I'd like to pick up on one of the things that you mentioned there, which is that there are very large systems, language systems that are being used for all kinds of mundane tasks. And I'd just like to think about that for a minute, have us think together about that. So I'm imagining a system, all kinds of things in my life, this system is now becoming the basis in which I am engaging in things. It begins to structure the language that I use. It not only structures language, but it structures in certain ways, thought. And I want to contrast that with a view of human flourishing where the depth and variety of human experience, the richness of human experience is the kind of world that we want to live in, where there are all kinds of different ways of thinking about things, cultural ways, language poetic ways, different kinds of expressions.
Even what Aylin was talking about in the beginning, she grows up speaking Turkish and Bulgarian and now English, right? Think of her ability for expression across those languages. That's something I'll never experience. So I think another question that we might ask separate from the bias question perhaps related, but separate has to do with a certain kind of homogenization as these technologies pervade so much of society and even cross national international boundaries embedded in them are ways of thinking and what happens when over time. And inter-generationally, you think of young people coming of age in these technologies and absorbing almost in the background, in their ocean behind them, a very similar way of thinking and being in the world. What are the other things that are beginning to disappear? And I wonder if there isn't a certain kind of impoverishment about our collective experience as human beings on the planet that can result from that.
And so I think that's a very serious concern that I have. And beyond that specific concern, what I want to point out is that arriving at that concern comes from a certain kind of, I would say principled systemic way of thinking about what does it mean if we take this technology seriously and think of it at scale in terms of uptake and over longer periods of time, what might those implications be? And then if we could agree on a certain notion of human flourishing that would allow for this kind of diversity of thought, then that might really change how we wanted to use and disseminate this kind of technology or integrate it into our everyday lives. And we might want to make a different set of decisions now then the set of decisions that seem to be unfolding.
Justin Hendrix:
I think that's a fairly bald critique of some of the language we're hearing from Silicon Valley entrepreneurs who are suggesting that AI is the path to abundance, that is the path to some form of flourishing that seems to be mostly about economic prosperity. Do you think of your ideas as sort of standing in opposition perhaps to some of the things that we're hearing from those Silicon Valley leaders?
Batya Friedman:
I guess, what I would step back and say is what are the things that are really important to us in our lives? If we think about that societally, we think about that from different cultural perspectives. What are the things that we might identify? And then to ask the question, how can we use this technology to help us realize those values that really matter to us? And I would also add to that thinking about the planet. Our planet is quite astonishing, right? It is both finite and regenerative to the extent that we don't destroy the aspects that allow for regeneration. And so I think another question we can also ask about this technology, it depends on data, right? And where does data come from? Well, data comes from measurement, right? Of something, somehow. Well, how do we get measurement? Well, somehow we have to have some kind of sensors or some kind of instrumentation or something such that we can measure things, such that we can collect those things all together and store them somewhere, such that we can operate on them with some kinds of algorithms that we've developed.
Okay, so you can see where this is going, which is if you take that at scale, there's actually a huge amount of physical infrastructure that supports the kind of computation we're talking about for the kind of artificial intelligence people are talking about now. So while on the one hand we may think about AI as something that exists in the cloud, and the cloud is this kind of ephemeral thing. In fact, what the cloud really is, is a huge number of servers that are sitting somewhere and generating a lot of heat, so need to be cooled, often cooled with water, often built with lots of cables, using lots of extractive minerals, etc, etc. And not only that, but the technology itself deteriorates and some needs to be replaced at a certain number of years, whether it's five years or 10 years or 25 years. When you think about doing this at scale, the magnitude of that is enormous.
So the environmental impact of this kind of technology is huge. And so we can ask ourselves, well, how sustainable, how wise is that of a choice to build our society based on these kinds of technologies that require that kind of relationship to materials? And by materials I mean the physical materials, the energy, the water, all of that. So when I step back and I think about the flourishing of our society and technologies, tools and technologies and infrastructure that can support that over time for myself, I'm looking for technologies that make sense on a finite and regenerative planet with the population scales that we have right now, right? We could shrink the population and that would change a lot of things as well. Those are the kinds of questions. So what I would say about many of the people making decisions around artificial intelligence right now is that I don't think they're asking those questions, at least seriously and in a way in which it would cause them to rethink how they are building and implementing those technologies.
So there are explorations. There are explorations about putting data centers at the bottom of the ocean because it's natural cooling down there. There are explorations around trying to improve, say, battery storage or energy storage. But the question is, do we invest and build a society that is dependent on these technologies before we've actually solved those issues, right? And just by analogy, think about nuclear power. When I was an undergraduate, there were discussions, nuclear power plants were being built, and the question of nuclear waste had not been solved. And the nuclear engineers I talked to at the time said, "Well, we've just landed on the moon. We're going to be able to solve that problem too in 10 years. Don't worry about it." Well, here it is, how many years later, decades later, and we still have nuclear waste sitting in the ground that will be around for enormous periods of time.
That's very dangerous, right? So how do we not make that same kind of mistake with computing technologies? We don't need to throw the baby out with the bathwater, but we can ask ourselves if this direction is a direction more like nuclear waste around nuclear power, or if there is an alternative way to go, and what would that be? And could we be having public conversation at this level? And could we hold our technical leaders, both the leaders of the companies, the CEOs and our technologists accountable to these kind of criteria as well? And that I think would be a really constructive way for us to be moving at this moment in time.
Justin Hendrix:
So just in the last few days, we've seen the EU agree apparently some final version of its AI Acts, which will eventually become law depending on makes it through last checks and process there. We've seen the executive order from the Biden administration around artificial intelligence. We're seeing a slew of policies emerge across states in the US which are more likely perhaps to become law than anything in the US Congress. What do you make right now of whether the policy response to artificial intelligence in particular is sufficient to the types of problems and challenges that you're describing? And I might ask you both that question, but Dr. Caliskan for you, how do you think about the role of the lab in engaging with these questions going forward in these next few years?
Aylin Caliskan:
We are at the initial stages of figuring out goals and standards moving forward with these powerful technologies. And we have many questions. In some cases, we do not exactly even know what the questions are, but the technology is already out there. It has been developed and deployed, and it is currently having impact on individuals and society at scale. So regulation is behind and accordingly nowadays, we see a lot of work interest and demand in this area to start understanding the questions and find some answers. But given that the technology is being deployed in all kinds of socio-technical contexts, understanding the impact and nuance in each domain sector will take time. Although the technology is still evolving very rapidly and proliferating in all kinds of application scenarios, it is great that there is some progress and there is more focus on this topic in society, in the regulatory space and in academia, in the sciences as well.
But it's moving very rapidly. So rapidly that we are not able to necessarily catch the problems on time to come up with solutions, and the problems are rapidly growing. So how can we ensure that when developing and deploying these systems, we have more robust standards and checkpoints before these systems are released and impact individuals, make decisions that change life's outcomes and opportunities? Is there a way to slow down so that we can have higher quality work in this space to identify and potentially come up with solutions to these problems? And I would also like to note that yes, the developments from the EU or the executive order are great, but even when we try to scratch the surface to find some solutions, they will not be permanent solutions. These are socio-technical systems that evolve with society, and we will have to keep dealing with any side effects dynamically on an ongoing basis. Similar to the analogy Dr. Friedman just made about bridges and their annual maintenance. You will need to keep looking into what kinds of problems and benefits might emerge from these systems. How can we amplify the benefits and figure out ways to mitigate the problems while understanding that these systems are impacting everyone and the earth with great scale?
Justin Hendrix:
That maybe gives me an opportunity to ask you, Dr. Friedman, a question about problem definition. So there's been a lot of discussion here about what are the right questions to ask? What are the ways that we can understand the problems and how best to address them? Close to 30 years on these questions, research career essentially about developing frameworks to put these questions into, what have you learned about problem definition and what do you hope to sort of pass along during this transition as you sort of pass the baton here?
Batya Friedman:
So I would just say that my work in value sensitive design is fundamentally about that. And we deploy human values as what is important to people in their lives, especially things with moral and ethical import. And that definition has worked well for us over time. And along with that, we've developed methods and processes. So I think of the work that we've done in a way, there's the adage about you can give a man a fish or you can teach him how to fish and he'll feed himself for the rest of his life, or I suppose you could give that to any person and they will be able to do that. I think that the work that we've been involved in is thinking about, well, what does that fishing rod look like? Are there flies and what are those flies about? What are the methods for casting that makes sense and how can you pass along those methods? And also there's knowledge of the river and knowledge of the fish, and knowledge of the insects and the environment and taking all of that into account and also knowing that if you over fish the river, then there won't be fish next year.
And there may be people upstream who are doing something and people downstream who are doing something, and if you care about them, want to ensure that your fishing is also not going to disrupt the things that are important to them in their lives. So you need to bring them into the conversation. And so I would say what my contribution has been, has been to frame things such that one understands the roles of the tools and technologies, those fishing rods, those flies, the methods, the also understanding of the environment and how to tap into the environment and the knowledge there and to broaden and have tools, other kinds of tools for understanding and engaging with other stakeholders who might be impacted in these systemic things. So my hope is that I can pass that set of knowledge, tools, practices to Aylin, who will in her lifetime encounter the new technologies, whatever those happen to be as they unfold, that she will not be starting from scratch and having to try and figure out for herself how to design a good fishing rod.
She can start with the ones that we've featured out and she's going to need refinements on that, and she's going to decide that there are places where the methods don't yet do a good enough job. And there's other things that have happened. Maybe there was a massive flash flood and that's changed the banks and the river, and there's something different about the environment to understand, but I hope she's not starting from scratch, but could take those things, extend and build them and has the broader ethos of the exploration as a way to approach these problems. So that's what I hope I'm passing and trust that she will take up and make good wise choices with it. I think that's all we can do, right? We're all in this together through the long term.
Aylin Caliskan:
I am very grateful that I have this opportunity to learn from colleagues that have been deeply thinking about these issues for decades when no one even had an idea about these topics that are so important for our society. And in this process, I am learning, and this is an evolving process, but I feel very grateful that I have the opportunity to work with the tech policy led community, including Batya, Ryan, Yoshi, who have been so caring, thoughtful, and humane in their research, always incorporating and prioritizing human values and providing a strong foundation, a good fishing rod to tackle these problems. And I am very excited that we will continue to collaborate on these topics. It is very exciting, but at the same time, it is challenging because these impacts come with great responsibility and I look forward to doing our best given our limited time and resources and figure out ways to also pass these methodologies, these understandings, these foundational values to our tech policy community and future generations as they will be the ones that will have these fishing rods to focus on these issues in the upcoming decades and centuries.
Batya Friedman:
I wanted to pick up on something also that Aylin had said, not in this comment, but the comment before about slowing things down. And I just wanted to make another observation for us. Historically, when people have developed new tools and technologies, they have been primarily of a physical sort, though I think something like writing in the printing press are a little bit different, but they take a certain amount of time to actually produce things, it takes a certain amount of time for things to be disseminated. And during that time, people have a chance to think and a chance to come to have a better understanding of what a wise set of decisions might be. We don't always make wise decisions, but at least we have some time and human thought seems to take time, right? Ultimately, we all have our brains and they operate at a certain kind of speed and a certain kind of way.
I think one of the things we can observe about our digital technologies and the way in which we have implemented them now is that if I have a really good idea this afternoon and I have the right set of technical skills, I can sit down and I can build something and by 7:00 in the morning, I can release that and I can release and broadcast that basically across the globe. And others who might encounter it, if the stars align, might pick that up. And by 5 o'clock in the evening, twenty-four hours from when I had the first idea, maybe this thing that I've built is being used in many places by hundreds, if not thousands or tens of thousands, hundreds of thousands of people, right? Where is there time for wisdom in that? Where is there time for making wise decisions? So I think in this moment we have a temporal mismatch, shall we say, between our capacity as human beings to make wise choices, to understand perhaps the moral and ethical implications of one set of choices versus another, and the speed at which new ideas can be implemented, disseminated, and taken up at scale.
And that is a very unique challenge I think, of this moment. And so thinking about that really carefully and strategically, I think would be hugely important. So without other very good ideas, one thing one might say is, well, what can we do to speed up our own abilities to think wisely? That might be one kind of strategy. Another strategy might be, well, can we slow this part down, the dissemination part down if we can't manage to make ourselves go more quickly here in terms of our own understandings of wisdom, but at least getting the clarity of that structural issue on the table and very visible, I think is also helpful. And from a regulatory point of view, I think understanding that is probably also pretty important. Usually when people say you're slowing down a technology, that's seen as quite a negative thing, I think it's squashing innovation. But I think when you understand that we are structurally in a different place and we don't have a lot of experience yet, maybe that's some additional argument for trying to use regulation to slow things in a substantial way. And what heuristics we might use, I don't know, but I think that is really worth attending to.
Justin Hendrix:
Well, I know that my listeners are also on the same journey that you've described of trying to think through these issues and trying to imagine solutions that perhaps, well maybe fit the bill of wisdom or a flourishing society, certainly a democratic and equitable and more just society.
I want to thank both of you for all of the work that you've done to advance these ideas, both the work that's come before and the work that's to come from both of you and from UW and the Tech Policy Lab more generally. I thank you both for talking to me today. Thank you so much.
Batya Friedman:
Thank you.
Aylin Caliskan:
Thank you.
Authors
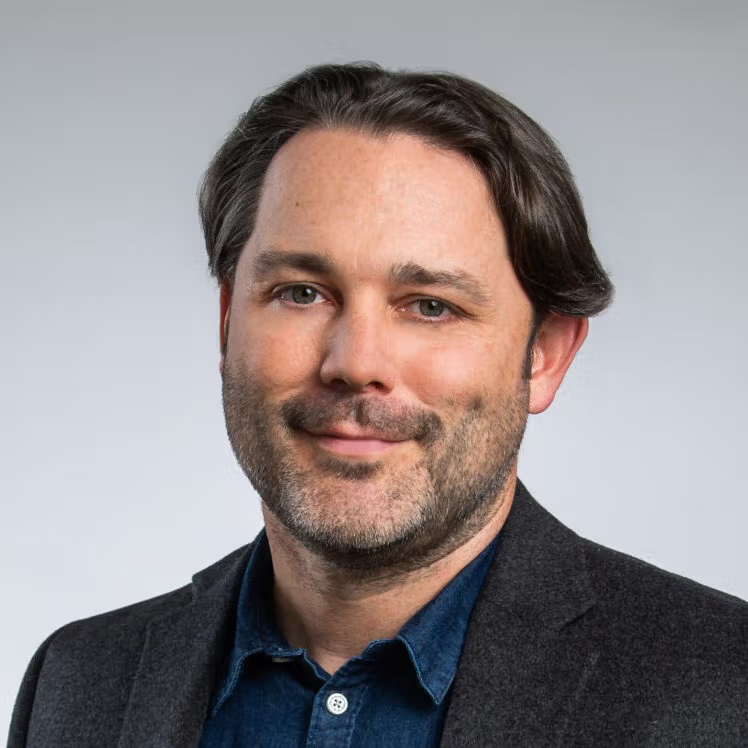