To Regulate General Purpose AI, Make the Model Move
Alex Engler / Nov 10, 2022Alex C. Engler is a Fellow at the Brookings Institution and an Associate Fellow at the Center for European Policy Studies, where he examines the societal and policy implications of artificial intelligence.
Thierry Breton, Commissioner for Internal Market of the European Union, April 21 2021. Source
- The EU has brought regulating general-purpose AI (GPAI) models, like GPT-3 and Stable Diffusion, to the forefront of the AI policy debate.
- While the attention to these models is warranted, the EU’s current approach has tunnel vision—it is too narrowly focused on this emerging type of model, and not on how high-risk AI models get deployed.
- A better solution would look to ensure that GPAI developers pass along needed information to third parties, including the AI models themselves.
It is becoming more common that multiple companies collaborate to develop artificial intelligence (AI) for commercial purposes. For instance, CoPilot, an AI tool for helping programmers code, is a descendant of GPT-3—first developed by OpenAI and then integrated into software by GitHub. The less well-known company Alpha Cephei takes open-source AI models for speech recognition and further develops them into enterprise software products. There are many such examples of multi-organizational AI development, which the EU has termed the “AI value chain.”
As a small number of companies expand their development of especially large AI models—think Google and its subsidiary DeepMind, Microsoft and its exclusive partner OpenAI, Facebook and the startup Anthropic—there is a general sense that these models will further enable new and more complex manifestations of AI value chains. A report from Stanford’s AI community has gone so far as to call them “foundation” models, and notes in the first sentence that their defining quality is that they “can be adapted to downstream tasks.” Beyond generating surreal images and writing believable text, some models are also manipulating robotic arms and mastering video games, although they still fall far short of human comprehension.
In recent drafts of the AI Act, the EU has proposed new regulatory requirements for these models, which they call “general-purpose AI” (GPAI). In reality, today’s GPAI is merely multi-purpose, but its potentially central role in the AI value chain makes it fair to ask how it could be regulated. The Council of the EU has proposed that GPAI models should be subject to specific regulatory requirements, including for risk management, data governance, technical documentation, as well as standards of accuracy and cybersecurity. This way, the GPAI models will be safe, tested, and well-documented for downstream use.
Except for one minor detail, which is that this won’t really work. While the GPAI developer can broadly improve the function of its model, there is no way to guarantee it will still be effective and unbiased when it is applied in a downstream application. The variety of downstream use cases is just too diverse—consider how a language model might be used for any of the following: searching through legal documents, generating advertising copy, grading essays, or detecting toxic speech. Good algorithmic design for a GPAI model doesn’t guarantee safety and fairness in its many potential uses, and it cannot address whether any particular downstream application should be developed in the first place.
There are other downsides to this approach. This will end up regulating GPAI models that are only used for trivial tasks, such as an adaptation of Stable Diffusion for recommending fashion choices. Some of the requirements may be truly difficult to meet—it’s not clear how companies might sell a GPAI model and still implement a risk management system.
I am not, as one of the U.S.’s unofficial policies advocates, encouraging no regulation of GPAI. Instead, I am suggesting the EU take an approach that is centered around the AI value chain, and not solely the idea of GPAI. In the long run, the size and complexity of a given model is much less important than whether it can be applied safely.
The EU should reformulate this part of the AI Act such that it encourages passing information from the GPAI developer to the downstream developers. Most critically, and most controversially, this includes sharing the AI model itself (the model object, in technical parlance) with those third-party developers. This has many advantages for building safe and effective AI systems.
If downstream developers have direct access to the GPAI model, they can use a wide range of methods to interrogate that model, including creating model-specific metrics, using other AI models to test the GPAI model (called red-teaming or adversarial training), cutting away parts of the model (called pruning), or altering the model in almost any way. These evaluations are more difficult, and at times impossible, when the GPAI model is enclosed in software or only available through an application programming interface (API). The case of access via API might be the worst (and is, notably, how models from OpenAI and major cloud providers are currently available). Access over API constrains how a GPAI model can be updated, at most enabling fine-tuning. Even if the possibilities to update or adapt the models are later expanded, since GPAI models accessed via APIs charge by the amount of data, this strongly discourages the data-intensive process of extensive and routine testing of GPAI models.
We don’t need technical jargon. AI models are helping to make a decision. Ultimately, the best situation is that the company that is making the decision has all the information it needs to scrutinize and make that choice, automated or not. If that’s the case, all the regulatory requirements can be applied to that company, leading to simpler and ultimately more effective government oversight. This is the best-case scenario for governing the AI value chain.
Yet, GPAI developers may not want to transfer their model objects to their clients, despite the obvious safety benefits. They may prefer keeping their models behind APIs, where they can continuously charge for access, while also maintaining tight control over their intellectual property.
For particularly impactful circumstances, this is not a good enough argument for lawmakers to defer to the GPAI developers. If GPAI developers want to make their models commercially available for downstream use in hiring, medical applications, educational software, or financial services—really any of the EU’s high-risk categories—they should have to transfer the AI model object, as well as documentation about the data and training process. These situations simply have too much at stake for downstream developers to use AI systems they do not fully understand. Model transfer would enable downstream developers to infinitely test and evaluate the GPAI model when it is fully integrated into their final product.
As a benefit to GPAI developers, under this governance framework, there is no reason to split regulatory responsibilities across the AI value chain. Once the model and its documentation are shared with the downstream developers, they can fully bear responsibility for the decisions and outcomes of the GPAI model and its use in any broader software system.
There is a compelling case that encouraging GPAI developers to sell or lease the GPAI model to downstream developers will lead to better uses of these models. Really, this should apply to all models used for high-risk purposes, not just GPAI. This alternative, requiring that any pre-trained AI model needs to be in-hand for high-risk use, would obviate the need to legally define GPAI. This matters since the EU has not yet finalized any definition of AI, much less the emerging and amorphous collection of models that might be GPAI. There are more suggestions in my recent report on the AI value chain for the Center for European Policy Studies.
Yet, this argument has focused on only one type of risk from these models, namely those that arise from commercialization. There is a second set of concerns around GPAI models that comes just from their existence and ease of accessibility. I call these proliferation harms.
These harms come from the misuse of GPAI models for everything aside from commercialization. GPAI models can generate non-consensual pornography and other deepfakes, hate speech or other harassment, and fake news and disinformation. These are already significant problems in many digital ecosystems, and the proliferation of GPAI models may well make them all worse. So, would the original EU proposal to regulate GPAI prevent the malicious uses caused by proliferation?
No. Even well-designed models can be used for malicious purposes. Any language model can be adapted to 4chan data and made monstrous, just like an image generator could be adapted to create non-consensual porn using a combination of an individual’s photos and other pornographic images. And attempting to prevent the spread of these models could have dire consequences for open-source development.
Unfortunately, my proposal also does nothing to prevent proliferation harms. Actually, by encouraging model movement, my proposal probably makes them mildly worse. There is no intervention at the model level that can prevent these harms. Most proliferation harms have to be tackled by other approaches, such as through platform content moderation and government interventions resembling the EU’s Digital Services Act.
However, encouraging the transfer of GPAI models to downstream developers does, perhaps dramatically, enable their safer commercial application, while simplifying government oversight. To make the AI value chain safer, make the models move.
Authors
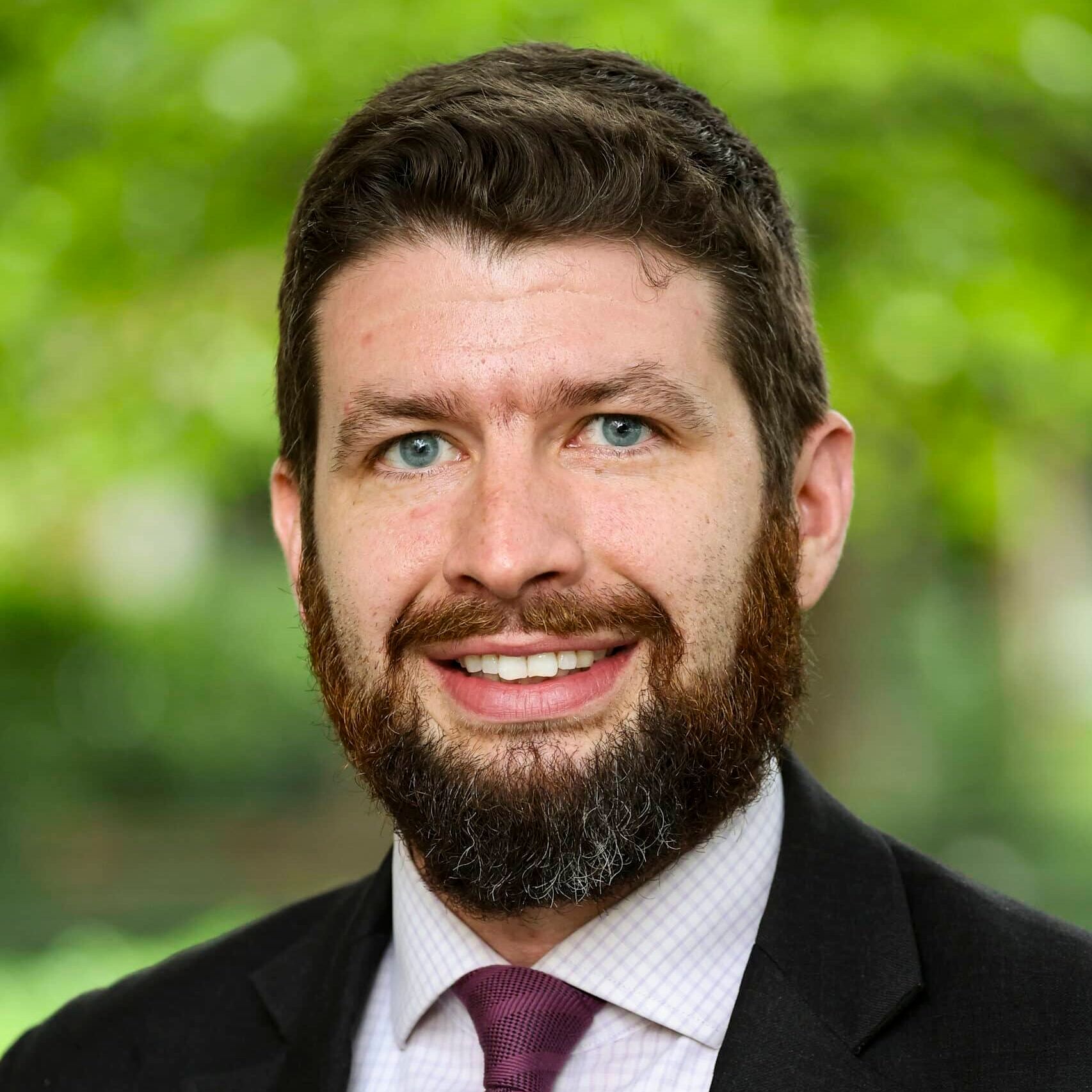