Three Strategies for Responsible AI Practitioners to Avoid Zombie Policies
Abhishek Gupta / Jun 14, 2024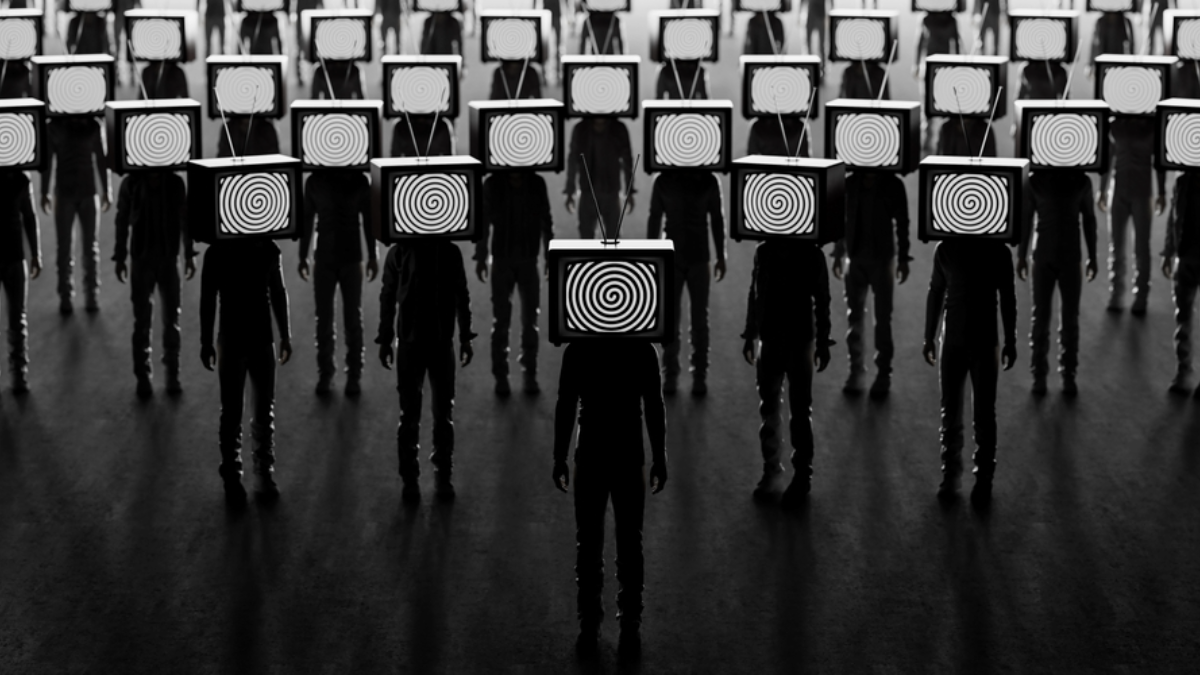
Zombies. Shutterstock
In an age in which technology, societal norms, and regulations are evolving rapidly, outdated or ineffective governance policies, often called "zombie policies," risk plaguing Responsible AI program implementations in organizations. By understanding the characteristics and persistence of these policies, we can uncover why addressing them is crucial for fostering innovation, trust, and efficacy in AI deployment and governance.
As AI technologies become increasingly integrated into critical aspects of organizations, the persistence of outdated policies can lead to significant resource drains, hinder progress, and damage institutional credibility. In particular, it can dampen enthusiasm and investments from senior leadership in nascent programs, a death knell for Responsible AI, which requires tremendous support to overcome organizational inertia.
Additionally, these zombie policies not only stifle innovation but also perpetuate inefficiencies that can have far-reaching consequences by perpetuating and encouraging behaviors and ‘gaming-the-system’ that happens when staff follow the policies in letter but not in spirit. By adopting strategies for continuous policy review, evidence-based decision-making, and cultivating an agile organizational culture, Responsible AI practitioners can dismantle obsolete structures, paving the way for more effective, ethical, and responsive governance and policies.
Where and how do zombie policies come about?
A "zombie policy" refers to a policy that continues to exist and exert influence despite being outdated, ineffective, or discredited. These policies persist due to bureaucratic inertia – when changing them requires significant efforts, resources, or political will. There might be other vested interests, or a fear of losing face for whoever instituted the policy in the first place. Sometimes, simply put, organizational cultures and structures are very slow to change, and that lets these zombies hang around. It is common to see this happen in economic policies, healthcare practices, and academic institutions that are slow to adapt to change.
Spotting the Walking Dead policies in your organization can be done with a simple three-part test:
- Outdated: Although the policy may have been relevant when first implemented, it is no longer suited to current conditions or knowledge.
- Ineffective: It fails to achieve its intended goals or results.
- Discredited: It may have been proven through research or practice to be flawed or harmful.
Understanding and addressing zombie policies is crucial for ensuring that governance and organizational practices remain relevant, effective, and aligned with current realities. Without that, they may actively harm the organization by draining resources, blocking the adoption of more effective and innovative approaches, and damaging the credibility of nascent programs and their backers. This makes it difficult to sustain resource commitment, which is critical for the success of the programs.
Harming Responsible AI program implementation
Some of the most common ways that we see zombie policies crop up in technology and AI governance is when we’re faced with a rapidly evolving landscape, both in terms of AI capabilities and the regulatory approaches to rein in the harms that arise from unchecked development and use of these technologies. This includes:
- Static Ethical Guidelines that do not evolve with advancements in AI technology or societal values can wreak havoc, for example, in terms of content moderation practices.
- Ineffective Oversight Mechanisms that fail to adequately monitor or enforce responsible AI practices can lead to product releases that land you on the front page of the New York Times (a veritable PR nightmare!)
- Outdated Training Programs for staff that do not reflect the latest in AI ethics, regulation, or technology can lead to them imbibing the wrong ideas regarding their roles and responsibilities for ethical use in their everyday work. This can manifest in the form of shadow AI and secret cyborgs.
Taking aim at the zombies
To prevent zombie policies within Responsible AI programs, we have three potent tools and approaches to help us make the proverbial headshot (arguably the most effective way to handle a zombie!)
1. Continuous Policy Review and Update
Avoiding policy rot requires active efforts from those responsible for program implementation and maintenance. If you don’t have resources allocated for that, start there first.
Regularly review and dynamically update AI policies to reflect technological advancements, new research findings, and evolving societal expectations. This means shortening policy review cycles and having that as a standing agenda item every quarter or semi-annually. This can become relevant when you have different paces of change in jurisdictions around the world. The European Union’s AI Act introduces stringent requirements for high-risk AI systems, whereas the United States focuses more on voluntary frameworks and guidelines. Companies operating across these regions must continuously update their AI policies to comply with both regulatory environments.
But how do we find out where the policies are breaking in the organization? Implement feedback loops that gather insights from AI practitioners, ethicists, and stakeholders to identify gaps and areas for improvement. The EU's General Data Protection Regulation (GDPR) mandates data protection and privacy practices that significantly impact AI operations. Regular feedback from data protection officers can highlight practical compliance challenges and areas needing policy adjustments. This can be done via surveys, tracking incident rates, adoption rates in terms of policy application to products and services, and questions raised at various town halls and forums within an organization, amongst other metrics.
Finally, compare your organizational practices with industry standards and best practices to ensure alignment and relevance. Larger organizations like Microsoft and Google frequently publish progress on their Responsible AI programs, which can serve as a starting point for this benchmarking activity.
2. Evidence-Based Decision Making
While already a best practice in medicine, current approaches in Responsible AI have been slow to adopt a more evidence-based approach to judging the effectiveness of these programs. For example, the rapid adoption of AI in healthcare during the COVID-19 pandemic demonstrated the need for data-driven policy adjustments. Evidence from AI applications in diagnostics and treatment highlighted both benefits and ethical concerns.
As mindful practitioners, we can begin to adopt this best practice from medicine by using empirical data and research to inform policy decisions and modifications. This includes monitoring the outcomes of AI implementations, their societal impacts, and whether the policies met their stated and intended goals. Another facet to track is whether that was the most effective way to achieve those goals, i.e., a good exploration of the solution space for policies is equally important.
But we don’t have a crystal ball in terms of which policies will work well a priori. Testing new policies and frameworks through pilot programs before full-scale implementation to assess effectiveness and making necessary adjustments is a great way to mitigate reluctance, hesitation, and risk in change adoption while giving you ample room to explore the potential upside of putting in place something that could ultimately be much more effective. For example, the AI Governance Framework in Singapore encourages organizations to test AI governance practices in sandbox environments before full-scale implementation. This approach allows for iterative improvements and learning.
Sometimes, the best ideas come from speaking to those who come from a different background, or at least from a field that lies in the adjacent possible. Engaging with experts from related fields such as ethics, law, social sciences, and technology can help ensure comprehensive and informed policy development. They’re often not resident within an organization. Hence, it behooves us to hunt outside, especially in the budding Responsible AI ecosystem where people come together in many different places to share their expertise. For example, the Partnership on AI, which includes stakeholders from academia, industry, and civil society, fosters interdisciplinary collaboration to address AI ethics and governance challenges.
3. Cultivating an Agile Organizational Culture
Show me the incentives, and I’ll show you the results, or at least something to that effect, is one way we can think about where and when we can expect zombie policies to rear their ugly head. We must begin by fostering a culture that values flexibility and responsiveness, encouraging teams to adapt quickly to new information and changes in the AI landscape. In particular, a culture that encourages staff to change their minds frequently, especially in light of new information, is a valuable trait that must be embedded deep into the organizational culture. In fact, the Canadian Directive on Automated Decision-Making emphasizes flexibility and iterative improvement in AI systems used by the government. This promotes responsiveness to new information and evolving standards.
Handling emergent issues sometimes warrants novel approaches that don’t fall into the traditional canon of policy approaches. For example, the UK AI Council’s Roadmap for AI proposes a regulatory sandbox for AI innovation, allowing companies to experiment with new technologies while ensuring ethical and legal compliance. Encouraging innovation in the form that governance approaches take, the policies that are implemented to support them, and the tooling that the organization adopts to enable staff to integrate those considerations into their everyday workflows can become the lifeblood of a cultural transformation that heads off zombie policies from popping up in the first place. And, of course, learning from failures when innovative approaches don’t work is equally important for the overall success of this mindset.
Building internal capacity, especially in roles that are not traditionally very tech-heavy or haven’t had much exposure to AI in the past, needs to have support and resources in the form of training and education so that they understand the relevance of the policies and find themselves empowered to raise their voices and point out when things are working well and when they aren’t. For example, the AI Ethics Guidelines issued by the Singaporean government emphasize ongoing education for AI practitioners to keep abreast of ethical considerations and regulatory changes. Hesitation often arises when employees feel discouraged because they feel that they don’t have the requisite background to raise issues because they’re not tech-savvy enough, and hence, they must be missing something rather than the policy having something wrong.
Conclusion
To ensure the ethical and effective deployment of AI, it is imperative that we confront and eliminate zombie policies that impede progress and innovation. We can replace outdated practices with dynamic, evidence-based approaches by committing to continuous policy review, leveraging data-driven insights, and fostering an agile organizational culture. I urge policymakers, AI practitioners, and organizational leaders to actively engage in this transformative process, ensuring that AI governance frameworks are current and capable of adapting to future advancements and societal needs. Together, we can create a responsible AI ecosystem that truly serves the public good.
Authors
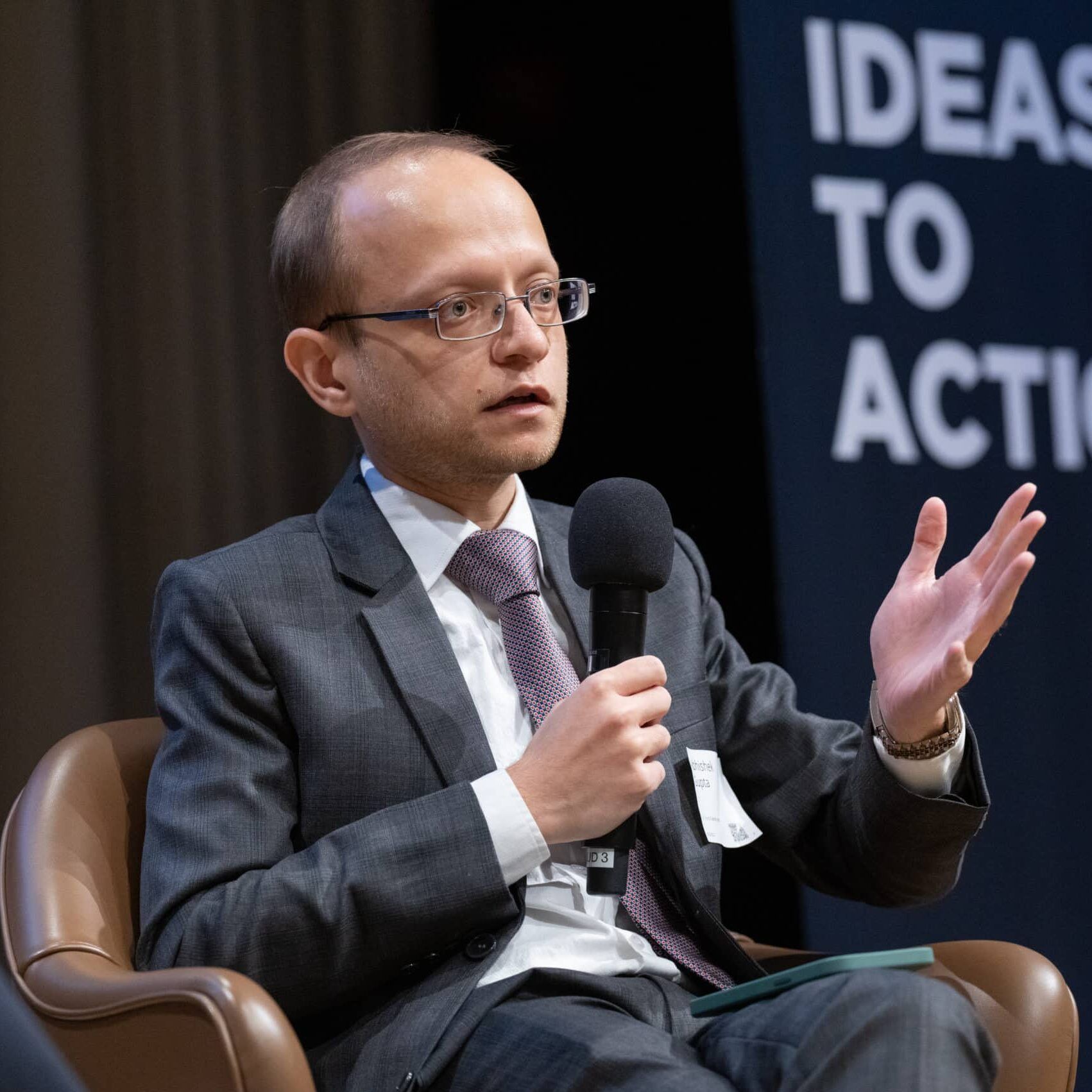