The White House AI R&D Strategy Offers a Good Start - Here's How to Make It Better
Sarah Myers West / May 30, 2023Sarah Myers West is Managing Director of the AI Now Institute.
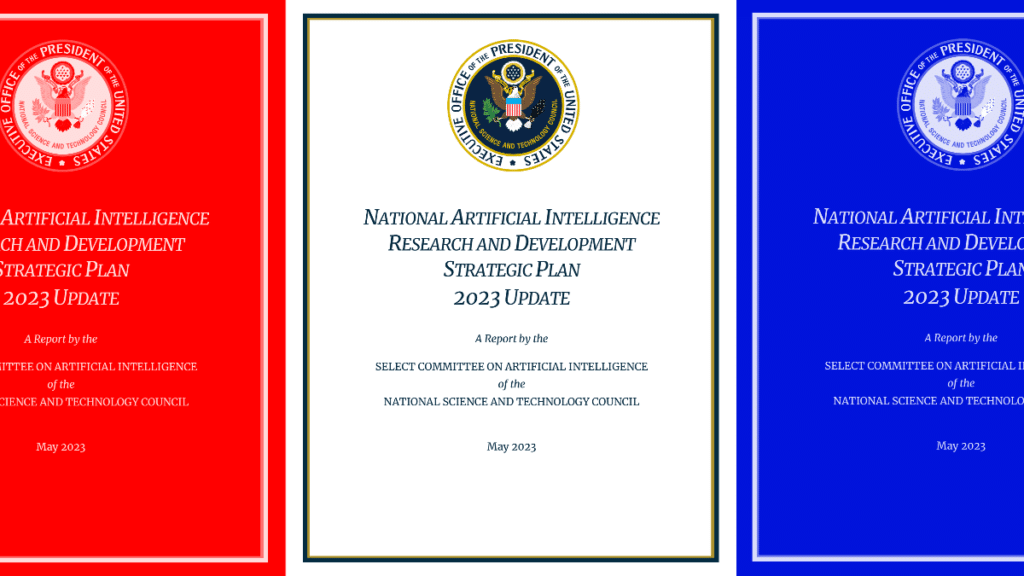
The White House released its national research and development strategy for AI last week, the latest in a flurry of activity in the executive branch of the US government to rapidly address the need for AI governance. The strategic plan focuses on where there are gaps in existing research that need to be filled, and it should be read in tandem with other measures, such as:
- The White House Blueprint for an AI Bill of Rights, released last year;
- The National Institutes of Science and Technology (NIST) Risk Management Framework;
- The National Telecommunications and Information Administration (NTIA) request for comment on algorithmic accountability;
- The Federal Trade Commission (FTC) cloud computing RFI, which could illuminate the role of computational infrastructure in shaping AI;
- The Equal Employment Opportunity Commission’s (EEOC) Artificial Intelligence and Algorithmic Fairness Initiative, part of its ongoing work on AI and hiring;
- The Consumer Finance Protection Board’s (CFPB) forthcoming work on AI chatbots used by financial institutions;
- The Department of Education’s new report on AI and the future of teaching and learning; and more.
The R&D strategy itself contains a number of measures that indicate that the government is listening to concerns raised by the research and civil society community around the broad impact of these technologies, foregrounding potential harms in the here and now over far-off concerns. This is welcome news.
But the Biden administration needs to go further: the document largely misses the role of industry concentration in shaping the future trajectory of artificial intelligence. Industry players control the resources needed for future research and development of AI. They also heavily influence the field of academic research, through dual affiliations and research funding. This is why strong accountability measures through enforcement of existing laws will be key.
It’s also critical that the White House attend to the detrimental effects of industry concentration where it chooses to make investments in research. Government can play an important role in incentivizing investments that serve the broader public interest, which industry players and the market will otherwise ignore.
Here are some of the bright spots in the document, as well as a handful of recommendations as to how it could go further.
1. The R&D strategy recognizes the need not just to address how AI exacerbates inequality. It also needs to advance equity.
This recognition means that we must consider who is benefitting from the use of AI, not just who is harmed or left out. AI is often used to make determinations about who gains access to resources - such as a mortgage at a favorable rate, or a high-paying salary. As the strategy document puts it, “If only wealthy hospitals can take advantage of AI...the benefits of these technologies will not be equally distributed." This broader focus attends to the analysis of leading experts such as Safiya Noble, Ruha Benjamin, Timnit Gebru and Joy Buolamwini.
It also points away from a flattened view of AI bias that positions it as solely existing within the system, and the need for a more expansive accounting of inequality.
2. The constant push to build AI at larger and larger scale carries significant environmental costs. The document emphasizes that investing in sustainability is the right path forward.
Building AI at large scale leads to many well-documented harms: the environmental cost is significant due to the resource intensiveness of training and maintaining AI systems, which have a large carbon footprint, draw down heavily from public water resources, and rely on minerals often procured under violent and exploitative conditions. Large-scale AI also contributes to other harms - increasing the discriminatory impact of these systems, exploiting the intellectual property of artists, relying on exploitative labor conditions and exacerbating harms to privacy.
Industry is currently all-in on building AI at scale. This is why seeing the White House encourage more sustainable approaches is particularly welcome: government funding that supports sustainability could be a powerful corrective to an overwhelming focus on building larger and larger - and more carbon and water intensive - AI models. This would also have other benefits to mitigate industry concentration, given that the biggest players are poised to benefit most from AI at scale by locking a greater number of customers into their cloud ecosystems.
3. The document identifies barriers to accessing advanced computing resources as a problem for the field. But increasing access alone won’t cure the fundamental challenge of industry capture in the field of AI.
The R&D strategy identifies barriers to accessing advanced computing resources as a problem for advancing research in the field: “...With resources concentrated in large technology companies and well-resourced universities, the divide between those with access and those without has the potential to adversely skew AI research. Researchers who lack access to rigorous data and computation will simply not be competitive”. This is a problem that the National AI Research Resource (NAIRR) task force is attempting to address, by providing wider access to public computational resources.
But by framing the issue as a problem of access, it only targets part of the problem and loses sight of the bigger picture. One recent survey by researchers at Georgetown identifies data and expertise as key bottlenecks in AI research, while another study published in Science documents increasing dominance in the research field by industry players, expressing concern that AI research will tilt as a result toward industry-driven interests. Without these concerns incorporated into the analysis, the expansion of access to AI resources could ultimately deepen, rather than ameliorate, industry capture.
4. The R&D strategy seeks to address the flaws with a largely self-regulatory approach to AI testing. These need to be coupled with strong disincentives against corporate experimentation in the wild.
The strategy glosses quickly over a crucial point: that companies are pushing out AI systems into wide commercial use without adequate prior testing. This raises underlying questions about why such testing is not in wide use, and why these companies aren’t facing enough liability when their systems fail to work as intended. Enforcement of existing laws and strengthening accountability frameworks will be critical to disincentivize companies from releasing AI systems before they are properly vetted and ready for widespread use.
The strategy does tackle a key part of this problem, that current approaches to AI testing leave “certain concerns unaddressed”, and we need more robust AI testing resources, mechanisms for public qualitative evaluation, and well-developed methods for testing large-scale AI models. This won’t happen if left to industry alone.
The strategy also mentions - briefly - a critical issue for the field, that novel AI research rarely receives testing because it’s often impossible to reproduce the results. Arvind Narayanan and Sayash Kapoor have been meticulously documenting what they describe as a ‘reproducibility crisis’ in machine learning research, and how the field fundamentally fails basic principles in sound scientific research.
This work is particularly important to keep in mind in light of Sam Altman’s testimony before Congress, in which he called for a licensing regime to be instituted for AI companies. It pokes holes in this self-regulatory approach, identifying where, left to their own devices, companies won’t make assessments that offer adequate scrutiny. We need a stronger regime in place, starting with strong measures to disincentivize corporate experimentation in the wild.
5. Lastly, the R&D strategy acknowledges the profound effects AI is having on workers. Step one to understand what the workforce needs should be to listen to worker organizers.
A substantial section of the strategy document focuses on the effects of artificial intelligence on the workforce. This is particularly welcome in light of worker-led organizing efforts highlighting the detrimental impact of AI on devaluing their work: these have been manifold, from the ongoing strike by the Writer’s Guild of America (WGA), a likely future strike by the Screen Actor’s Guild, unionization by the content moderators responsible for building ChatGPT, and ongoing organizing by platform and warehouse workers. These efforts make clear that workers have substantial ideas about whether and how artificial intelligence should be integrated into the workplace, and the research proposals from the White House would benefit from their clarity and guidance.
- - -
If complemented by strong enforcement measures, elements from the White House R&D Strategy could offer a powerful corrective to industry dominance in the field of artificial intelligence. But for this to be meaningful in practice, the White House must acknowledge that the national interest won’t be served by deepening industry capture in the field of artificial intelligence, but by applying sufficient friction to avert the harms caused by the use of these systems, and by making well-placed investments that serve the interest of the public, not of industry.
In doing so, it would build on its stated policy in the Executive Order on Competition: “the answer to the rising power of foreign monopolies and cartels is not the tolerance of domestic monopolization, but rather the promotion of competition and innovation by firms small and large, at home and worldwide.”
Authors
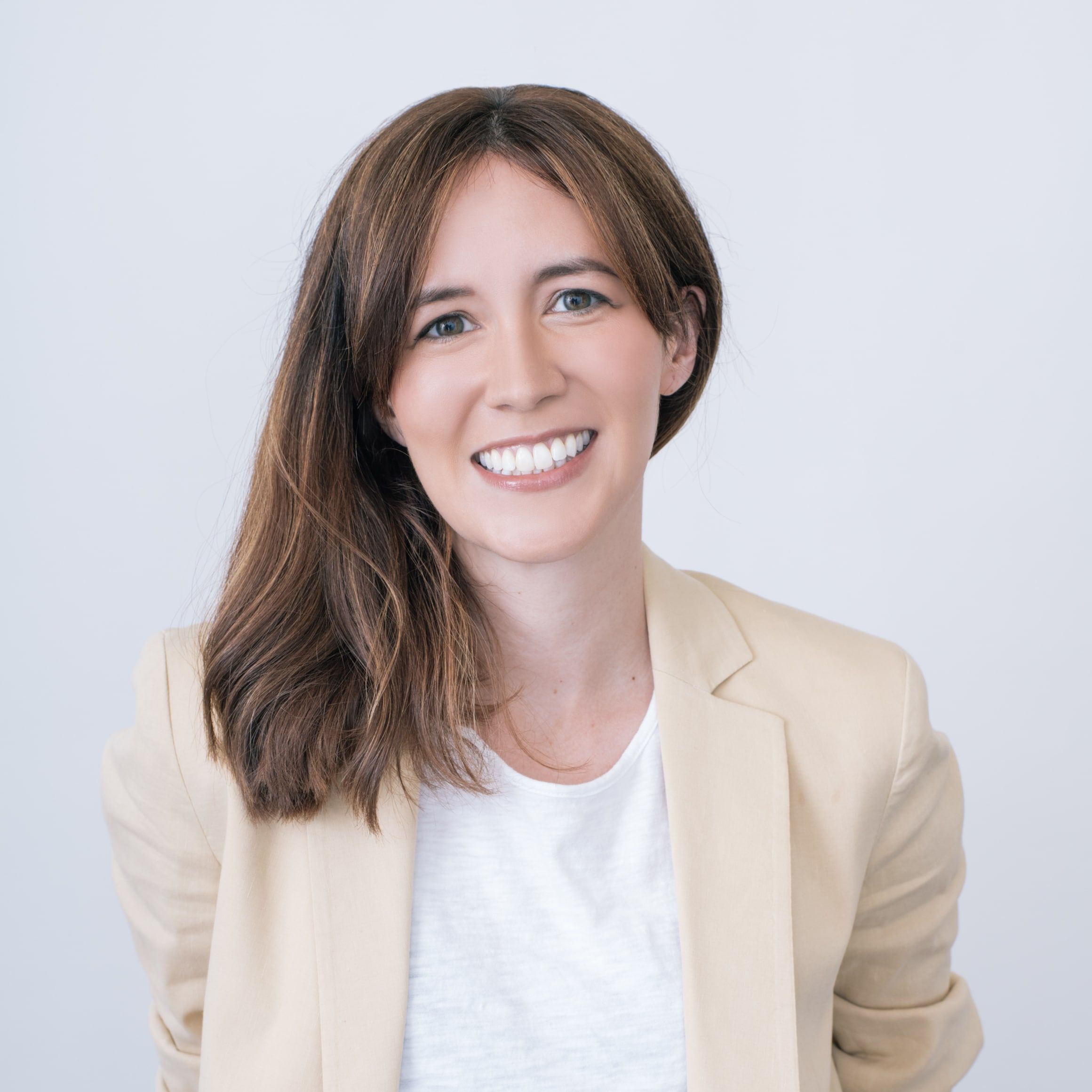