The Foundation Model Transparency Index: What Changed in 6 Months?
Prithvi Iyer / May 22, 2024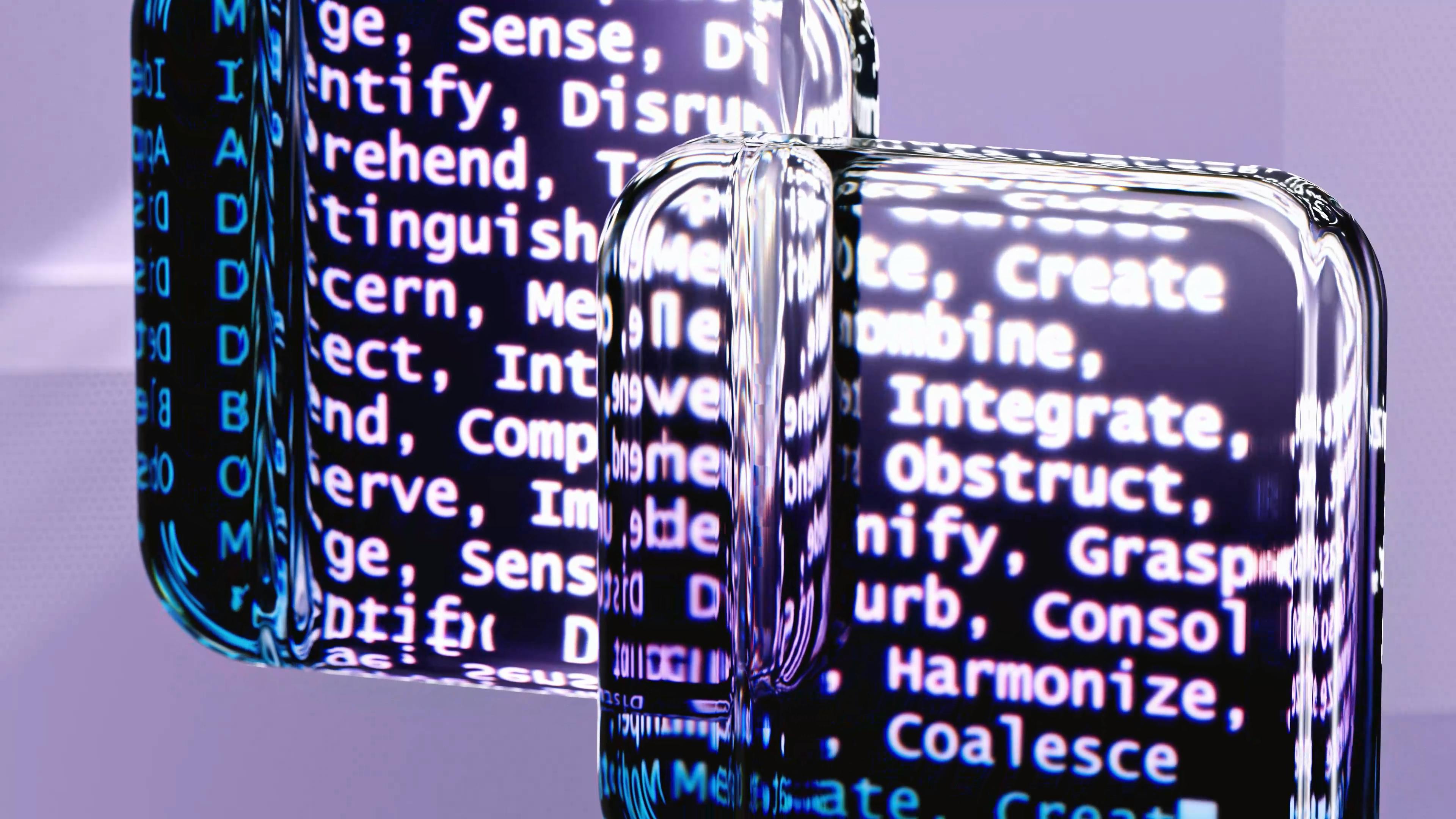
An artist’s illustration of artificial intelligence (AI). It depicts language models that generate text. CC Wes Cockx/Visualizing AI project.
The extent to which the developers of general purpose “foundation models” on which other artificial intelligence applications are transparent about their products is a pressing issue for regulators and those in the tech accountability community. Just as the technologies are new, standards and expectations around transparency are new as well. To advance the state of the art, researchers at Stanford, Princeton, and Harvard developed the Foundation Model Transparency Index (FMTI). The initial findings, released in October 2023, indicated that the “developers publicly disclosed very limited information with the average score being 37 out of 100.” Tech Policy Press covered the initial FMTI study, including providing a detailed summary of the index and its sub-domains.
To see how the AI transparency landscape may have evolved since then, the researchers conducted a follow-up study wherein they examined 16 model developers on the same 100 indicators studied previously. However, unlike the previous iteration that relied on publicly available information, the follow-up study went a step further by requesting model developers to submit transparency reports in response to the indicators, thereby including information that was not previously available.
The researchers contacted 19 AI companies, and 14 of them provided transparency reports on each of the 100 indicators. Based on each company’s report, the researchers assigned scores “based on whether each disclosure satisfied the associated indicator.” The overarching findings seem promising. For one, overall transparency improved, with developers showing a “21-point improvement in the mean overall score.” Each developer evaluated in the previous version also improved their scores by 19 points on average. However, opacity regarding upstream indicators like data access and labor persisted, indicating that companies are still hesitant to disclose information about how they build their foundation models.
The average score on transparency increased from 37 to 58 out of 100 from October 2023 to May 2024, with significant improvements “across every domain, with upstream, model, and downstream scores improving by 6–7 points.” While this is encouraging, there is still significant room for improvement, especially when it comes to the “upstream” domain, a set of 32 indicators that identify the ingredients and processes involved in building a foundation model. These include labor, data access, and computational resources used to train a model. For instance, of the 20 indicators where developers scored the highest, “just one indicator (model objectives) is in the upstream domain.” A closer look at the findings shows that, on the whole, “developers are less transparent about the data, labor, and compute used to build their models than how they evaluate or distribute their models.” In particular, the lowest scores were in relation to “data access (7%), impact (15%), trustworthiness (29%), and model mitigations (31%).”
The study also found that open-source developers score 5.5 points higher than the median closed-source developer. This difference is most visible in the upstream domain, where open-source developers provide more information about how their models were trained and developed. However, since open-source platforms have less control over how their models are deployed, they provide less information on downstream impacts compared to closed-source models.
The follow-up study found a few other notable differences compared to the previous iteration of the FMTI. For 26 out of the 100 indicators, four or more developers provided new information that was previously unavailable, especially in the data labor and compute subdomain. Comparing the rankings six months apart also indicates how some companies improved while others deteriorated. The below figure shows the change in overall transparency scores of the major AI companies.
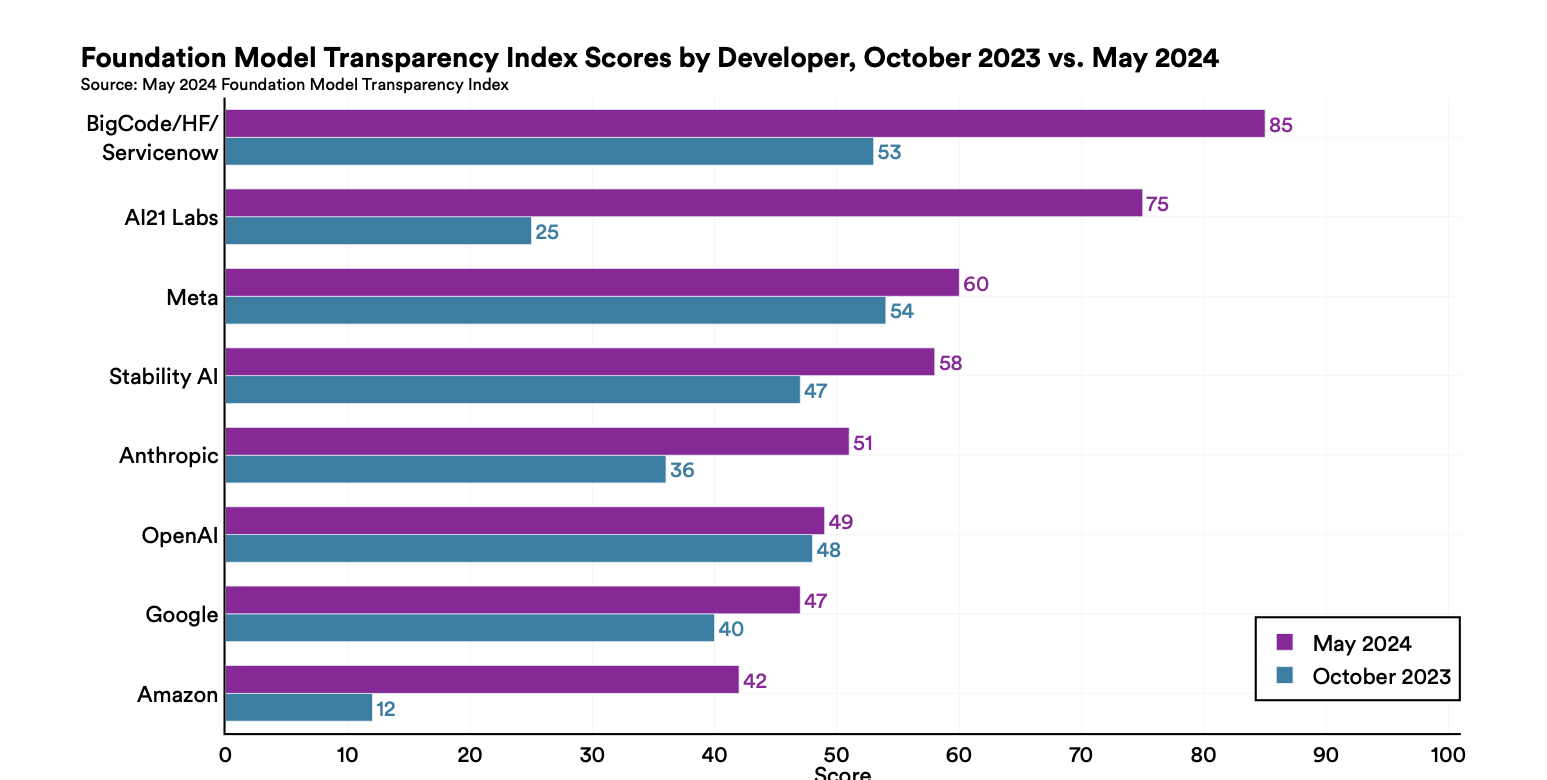
Change in overall transparency scores for the eight developers assessed in both versions of the Foundation Model Transparency Index.
Takeaways
The follow-up study provides valuable insights into how companies have been approaching AI transparency. It gives reasons for optimism while still indicating gaps that require major improvements. For instance, the follow-up study found some companies showed marked improvements in transparency by “releasing model cards or other documentation for their flagship foundation models for the first time.” In fact, the “compute” subdomain that measures transparency over training data and processing disclosures rose from 17% to 51%. This is encouraging since compute resources are often hidden from the public because they relate to “the environmental impact of building foundation models—and, therefore, could be associated with additional legal liability for developers and deployers.”
Interestingly, transparency around data access declined from 20% in October 2023 to merely 7% in May 2024. The researchers attribute this decline to the prospect of “significant legal risks that companies face associated with disclosure of the data they use to build foundation models.” Particularly, “these companies may face liability if the data contains copyrighted, private, or illegal content such as child sexual abuse material.” Companies were also secretive about who uses their models and their societal impact. Unfortunately, companies also scored low on “model mitigation,” meaning that they don't adequately disclose their strategies to mitigate inappropriate use cases.
The researchers urge policymakers to use the FMTI in order to be more specific in their understanding and redressal of transparency in AI. As the authors note, “Our iterative process with model developers provides an insight into how such specificity might arise in practice—government bodies might consider developing the institutional capacity to coordinate discussions with companies to ensure that they adhere to the standard required by a certain policy.”
As foundation models grow increasingly influential in shaping society, efforts like the Foundation Model Transparency Index provide a valuable mechanism for measuring and potentially incentivizing responsible AI development practices. While the May 2024 results show signs of improvement from before, it's clear that more work needs to be done to reduce the opacity of foundational model development and deployment and that smart regulation to require certain disclosures is necessary.
Authors
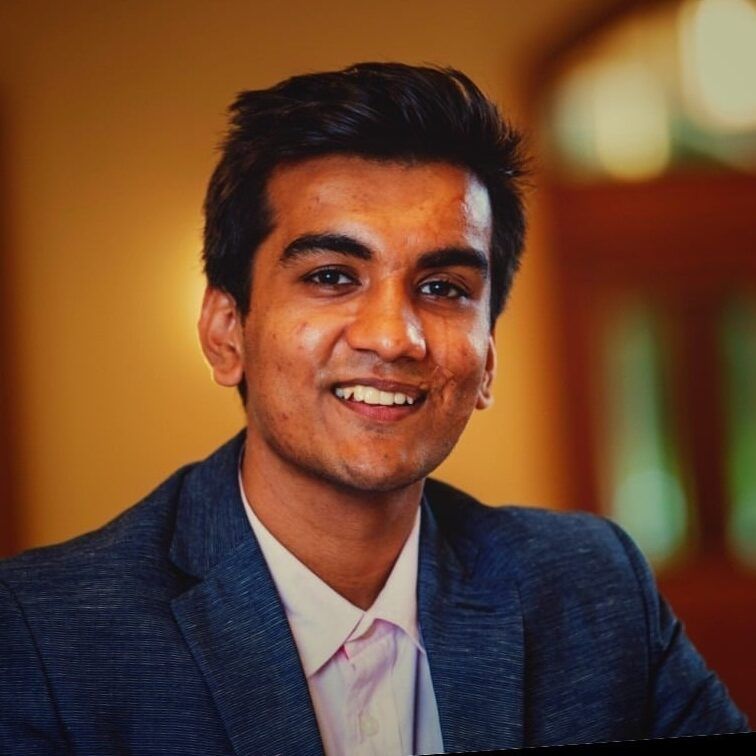