Responsible Release and Accountability for Generative AI Systems
Justin Hendrix / May 28, 2023Audio of this conversation is available via your favorite podcast service.
Today’s show has two segments both focused on generative AI. In the first segment, I speak with Irene Solaiman, a researcher who has put a lot of thought into evaluating the release strategies for generative AI systems. Organizations big and small have pursued different methods for release of these systems, some holding their models and details about them very close, and some pursuing a more open approach.
And in the second segment, I talk with Calli Schroeder and Ben Winters, both lawyers at the Electronic Privacy Information Center, about a new report they helped write about the harms of generative AI, and what to do about them.
A lightly edited transcript is below.
Justin Hendrix:
Just really quick for any listener that doesn't know what Hugging Face is, company with a funny name, can you give us the quick one two about hugging face and also about your role there?
Irene Solaiman:
Absolutely. Hugging Face is a IT community and a platform that is working to democratize good machine learning. So we primarily work towards the open end of my gradient, models, data sets and also spaces which makes the kinds of systems that are popular today more accessible, especially to people who may not have a computer science background. We also provide a limited amount of computing power for people to do the kind of safety research that needs to happen on these popular systems today. I've built our public policy, our policy work here at Hugging Face, but because I can't stay off my laptop, that's about a third of what I do. I spent two thirds of my time doing research. I find policy to be a mutually beneficial relationship with research, where I want to understand from policymakers what the public interest is and then work with technical systems, especially large language models on evaluating, on risk mitigation and publishing that research with the gradient framework that you see.
Justin Hendrix:
Irene, I quite liked this paper that you wrote earlier this year, The gradient of generative AI release methods and considerations. And I was pleased to see it in a slightly more accessible format, I suppose, in Wired in the last few days. Wanted to just could get your verbal summary of the idea here. This is looking at the way that companies and other organizations go about releasing generative AI systems and why it matters.
Irene Solaiman:
Yeah, absolutely. I'm so glad you found this helpful. I've just been working on system releases for a few moons now. In AI time that sounds like eons, but often I just hear this binary conversation of open versus closed. That is inaccurate for the landscape. For how influential generative AI is today, we really need to understand, not just systems impact, but how systems are being released to better understand their impact. So I created this framework. It's hard to share graphics on a podcast, but maybe we can walk through this gradient where all the way to the extreme leftmost end I have fully close and all the way to the extreme rightmost end I have fully open that considers a system, not just a model. I think often we fixate on a model when we need to be looking at many system components like training data.
Justin Hendrix:
Maybe let's walk through each of those categories, and perhaps give the listener just an example of the type of system that's been released in each category.
Irene Solaiman:
Absolutely. So I focus mostly on generative AI systems instead of foundation models or transformer based systems, mostly because I was one person trying to give insight into or release landscape that can be extrapolated to other types of systems, like foundation models that aren't explicitly generative. If we walk through fully close to fully open, what I would consider the most fully closed are systems that we don't even know about, that maybe even a tiny team within a highly resourced organization is aware and is doing that kind of development and testing. But the ones that we are aware, we've seen most often from companies like Google and DeepMind. I base my framework based off of how a company originally released. So something like Lambda from Google, today would be considered maybe slightly accessible due to Google's AI test kitchen, but for the purposes of understanding the landscape was first fully closed. It was publicly announced, but nobody outside of Google could access any part of that system or its components.
Part of what really stoked a lot of this release discussion conversation, at least in my spheres, was the release of GPT-2 in 2019, that I led with my colleagues and external partners at OpenAI. I also have a timeline in my gradient of release paper to be published at fact that shows GPT-2 as an inflection point for when systems really started to close, especially from larger organizations. And my experience at Open Source World and having led this release, people tend to feel quite strongly about options for release. A lot of proponents of open source believe that everything should be open all the time.
I don't consider myself an open source fundamentalist, but part of what helped me understand why people would find themselves at one part of the gradient is what I put as tensions throughout the gradient. But some of the reasons people feel strongly about openness is for the community research aspect, for getting broader perspectives into a system and its components. But then you have some more people who are concerned about really powerful systems having malicious use capability. People on the internet do really weird things with whatever they have access to, and if you make systems more accessible, you have less control. And that's where the fully closed argument comes in.
Justin Hendrix:
A lot of times folks do present this as a binary. Often in the media, I feel like these days, there's a binary between Open AI and the way that it's released more recently, ChatGPT, GPT-4 and then Stability AI and the way that eventually it made Stable Diffusion available. But on your gradient, they're actually closed together in on some level. Is that accurate to say that they're really perhaps not so far apart?
Irene Solaiman:
With the way that I lay out the systems in my paper, because it's based off of only their initial release and not what we see from, for example, stability so far, is I think a lot of folks are not aware that Stable Diffusion was originally a staged release that got leaked. I can't recall whether it was for 4chan or Discord, but it also speaks to how few mechanisms we have for securing more novel approaches to release. We see this with Llama where the Llama leakage really made folks question, can we trust more gated mechanisms or should we fully close? I think that was just a bummer that we can't start to prototype methods for release without trusting the researchers with whom we share. That was a tangential rant because I feel strongly about it.
Justin Hendrix:
Well, but let's talk about Llama for instance. There's been a lot of talk about that, about the fact that, I suppose, ended up on 4chan.
Irene Solaiman:
I believe so.
Justin Hendrix:
I guess this did play in somewhat in the hearing the other day, right? With Sam Altman and Gary Marcus and others. There was a conversation about open source versus other methods. This was a theme in the discussion, even though it wasn't exactly addressed. Did you get that sense as well?
Irene Solaiman:
Yeah, it's been an overarching theme around AI policy, and this is where I think it is absolutely critical to have nuance in the discussion, especially when we talk about more open source models. We don't always mean fully open. OPTs training data is not fully accessible, although I believe the paper shares that they train on publicly available data sets. But this is a question of accessibility. It's not really easy to find exact data sets that OPT trained on, which is why I classify it as downloadable. And if a model is gated, it would not be considered fully open.
Justin Hendrix:
I suppose in your gradient, there's no real, I guess, opinion about which approach is better or worse from a safety perspective.
Irene Solaiman:
From being, I guess, living in this field. That's the American cultural side of me, people feel very strongly, and I've found that approaches to safety generally tend to be ideological, living more in the open source world. People feel very strongly that the most open system, regardless of the kind of content it produces or how capable it is along some performance benchmarks, should be open at all times. That's not how I as Irene feel. We've seen with Hugging Face, we did take down a model for its content, not necessarily its capability, with the example of GPT, 4chan. This is an example that I generally give to policymakers as well, but I try not to publicize because I don't want many people playing with a really toxic model.
For those who aren't familiar, GPT 4chan was trained by, was fine-tuned on one of the open source models by a Yannic Kilcher. It was fine-tuned on 4chan's politically incorrect dataset, which is just some of the worst parts of the internet, but it wasn't a particularly powerful model. It just spewed really gross outputs. And that's a decision of safety, of content safety, where we don't necessarily have good thresholds for that, but it's not something that should be made fully accessible to everybody, at least that's the stance that we took when we took it down from our platform.
Justin Hendrix:
You've mentioned that it's kind of ideological, almost a matter of faith, I suppose, by the different companies that are taking the different approach. Do you think perhaps in five years and 10 years time we'll maybe have more data points, we'll have seen in the real world how these releases happen and be able to make a judgment?
Irene Solaiman:
We have a lot more data points today, for sure, than we did in the times of GPT-2, but I sadly, I feel like in this race dynamic, we don't have a ton of incentive to pioneer novel responsible research approaches unless we start to build the foundations for coordination and collaboration around labs. My optimism and especially seeing initiatives from, especially partnership on AI and Stanford, work towards more responsible deployment, I would like to believe that we will have more consensus on not just what is a responsible release, but what is the whole process leading up to release decisions, and also post-deployment, what does risk mitigation look like?
Justin Hendrix:
And I understand this is something that the folks at Stanford HAI, they have asked essentially for the industry to come together around this and to really hammer this out and create some shared understanding of how release should work.
Irene Solaiman:
Stanford's been phenomenal. They're also exceptional in the sense that they are placed physically and in the world of AI really well among different developers. So I think the world of the work that HELM is doing, the Holistic Evaluation of Language Models work from the Center for Research and Foundation models. I think this is a great example of how a trusted third party can evaluate models from different organizations even if those models aren't accessible to the public. But that requires a lot of the behind the scenes work that Stanford has been doing. And the same thing with partnership on AI on convening actors around the AI community.
Justin Hendrix:
I just want to get at some of those ideological ideas that you've mentioned. The way I understand it, and perhaps this is naive, so you'll correct me if I'm wrong, but the folks who are argue for a more closed approach, on some level, they're saying, we want to minimize the harms of our products in the near term, so we want to make sure they're as safe as possible. We want to red team the heck out of them. We want to introduce as many mechanisms to prevent harmful uses of our models. The folks who maybe are on the very other end, on the more fully open end, are saying, well, we would never really be able to do that terribly well, so we want others to do it along with us.
We'll have researchers look at the model, they'll be able to interrogate it. Government, perhaps regulators, can look exactly at how these systems are working and how they were trained, et cetera. And at the end of the day, that will produce a better environment where an ecosystem of actors can create a safer environment more overall, and perhaps we avoid some of the other harms of centralization of AI. Is that a fair way to characterize the, as you say, ideological extremes?
Irene Solaiman:
Well, something that I often say is that no one organization, regardless of how well-resourced it is or how large it is, has all the necessary perspectives, skills, expertise to fully assess and adapt a model. Just in the same way that there's no such thing as a base generative AI system that is unbiased or encompasses universal values. This is where some people really put themselves in a category of open API, access something along that gradient. I feel really strongly about broad perspectives that can be done to some degree in a really large organization, maybe like a Google, and this is some of the arguments for Google not sharing their models or even, for example, the example that I gave in the Wired piece that I wrote, was of more novel modalities like video where there's less literature, people want more risk control.
Meta has been really bullish about openness except for, for example, make a video, the video generative model. So I think to boil it down, something that's incredibly complex, you did capture, the key point that has no real solution. I try to avoid solution-oriented language. Has no consensus on the process to move forward, is how to seek appropriate feedback and perspectives, and how do we start to prioritize the type of safety work that needs to happen.
Justin Hendrix:
So I want to get a sense too of why this should matter to policymakers, and is there something that you believe they should do on this? Is this something that should be codified somehow in AI regulation? Is there an aspect of release strategy which should perhaps be touched by law?
Irene Solaiman:
Yeah, so especially for policymakers, I want to emphasize that no one spot along the gradient is the safest possible release, because if something's fully open, as a self-proclaimed internet gremlin, people on the internet might do really weird stuff with, it might hurt people for highly capable models that have fewer safeguards. That's why safety work on open source models is important with responsible AI licenses, better documentation, safety filters it's a lot of what you see OpenAI and Hugging Face and Stability working on. But the concern, I don't think that policymakers should automatically assume if it's closed, there's no risk, there's less malicious use. There's less insight into that model. There's less research happening. A lot of the safety research that we see today, for example, the watermarking work from University of Maryland researchers was done on an open model that was made accessible by Meta, that was tested on OPT. So in order to ensure that we have a thriving research ecosystem that we can make safety work happen, researchers need access to models.
Justin Hendrix:
I guess the thing is, it seems like it's too soon to say certainly that one way of going about this is better than the other. And so it feels like lawmakers should really stay away from any scheme that would diminish perhaps open release or open source approaches to generative AI. It seems like it's just really quite too soon to be able to make those types of judgements at all.
Irene Solaiman:
Cracking down on any sort of open source model feels really counterproductive to the research world, and where we are in AI is so much of what policymakers and stakeholders need to happen, is research. How do we make these models safer? How do we understand them? I have in my gradient to release paper to be published, in fact, the whole section on necessary investments and the actors who need to work on this. One of the examples is closing resource gaps and really bullish about the national AI research resource from the US government, just providing more research infrastructure like computing power. But I don't know if we'll ever get to the level of consensus needed to say "Don't release in this way." Having better fora for these discussions, and better understanding of, not just the technical but also policy measures for safeguards, is absolutely urgent.
Justin Hendrix:
One of those safeguards that some folks have proposed is having to seek licenses before you release a model. What do you make of that in this context?
Irene Solaiman:
Yeah, so I've been talking some to some of the folks who are proposing this licensing framework, and what I don't understand here is the capability threshold. This is the example that I give, with GPT 4chan, it's not a particularly capable model and however our society defines high performance. But there's a reason that we don't want to make it as accessible. So when we think about licensing, what I really fear is undue regulatory burden on smaller actors, the folks who don't have billions of dollars to do this kinds of testing, and especially folks who are closer to the lower resource end of this resource gap.
When we also think about capability, a lot of my conversations with policymakers is what the heck does that mean? What are our benchmarks group performance? We don't have standards bodies for, just like we don't have it for responsible releases, we don't have it for testing and evaluating models. The way that a lot of the AI research community has been dubbing a system as high performance has been based off of, honestly, arbitrary benchmarks that a lot of other folks have been using, and not necessarily including social impact evaluations, which I have a whole other rant on that we don't have to get into.
Justin Hendrix:
Well, perhaps we will bring you back on to get into that rant, and also maybe just to look at how this question's evolving sometime in the next few months.
Irene Solaiman:
I'd love that.
Justin Hendrix:
Thank you, Irene.
Irene Solaiman:
For sure. Thanks so much.
Justin Hendrix:
Next up a conversation with two of the authors of a new report from EPIC, the Electronic Privacy Information Center, on the harms of generative AI and what to do about them.
Calli Schroeder:
I'm Calli Schroeder, I am EPIC Senior Counsel and Global Privacy Counsel for the Electronic Privacy Information Center.
Ben Winters:
I'm Ben Winters, I'm senior counsel lead of the AI and Human Rights Project at the Electronic Privacy Information Center.
Justin Hendrix:
You are two of the authors, along with your colleagues, of generating harms, generative AI's impact and paths forward released this month. Great report and I'm looking forward to talking about it with you. I want to talk about a range of issues here, but first I just thought I'd ask you to speak a little bit about the frame you use in this. I found in the opening paragraphs of this, you refer to Danielle Citron and Daniel Solove's, Typology of privacy harms, Joy Buolamwini's Taxonomy of algorithmic harms. Talk about how you applied these frameworks, these rubrics to the question of generative AI.
Ben Winters:
The whole genesis for writing this report is the fact that with the release of generative AI and all the hype around it, a lot of people are focusing on harms that are very abstract and in the future, whereas just in non-generative AI we've been talking about and documenting and advocating around very current harms. And so we wanted to map those current harms and the way that those are being pseudo carried out and continued in generative AI contexts. And we want to ground those in as specific categories as possible so people can really feel this concretely and have language to use when discussing this, and when maybe addressing it, so that policy responses are not these vague things that are directed by corporations but are mappable to these things, whether they be about physical harms, economic harms, loss of opportunity, whatnot. So both of those taxonomies are a little bit overlapping at times, but I think quite complementary and gets a really wide range of the type of harms we have.
Calli Schroeder:
Part of the reason that we structured it, pulling from those set harm categories, was we wanted to make sure that when there's conversation about what can generative AI hurt or what's the problem with it, there was something concrete we could point to that was easily understandable, where we weren't talking across purposes but about the same thing. So when we went through the categories of like, well, what's the harm in the intellectual property space, and what's the harm in the privacy space, and what's the harm in misinformation and environment and all of these other areas? You could say, okay, the harm falls on this financial level or it falls at this communication or relational level. And it was something that was a more direct comparison that we thought would make it a lot easier to have these conversations in a productive way.
Justin Hendrix:
You do chronicle a range of harms, including some that we've discussed on this podcast quite a lot. The potential for these technologies to be used for information manipulation, disinformation, as well as harassment, impersonation, extortion, other forms of fraud. But I want to focus in particular on, I suppose, what is EPIC's bread and butter issue around privacy. I think one of the things that your report points out well is that not only do AI systems, these generative AI systems, that large language models rely on hoovering up a massive amount of data in order to train the models, but they also create incentives for companies to continue to hoover up as much information as possible in order to continue to develop more sophisticated AI. I want to ask you a little bit about that, how you see that dynamic playing out and what can be done about it.
Ben Winters:
So one thing that we've seen in this field, in a very short amount of time, is that there is a lot of money being poured into it. And so, as you said, the incentives are really there to be able to build, use and continually be able to refine and make slightly more impressive these generative AI systems. In the federal level, we said in the US we have real no controls on data collection, or the way that you collect data for one purpose and use it to another one. And so what organizations are incentivized to do here are to hoard and collect as much data as possible because they see that they can maybe use it in the future or sell it to somebody else. And so the uptake of these types of tools and the popularity of them and some deference by lawmakers, especially to them, as this inevitable revolution, makes it so that the average person's privacy is more endangered because AI systems forever have increased the incentive to get more and more data, but especially large language models are built on a large amount of data.
Calli Schroeder:
Yeah. I think at a very base level, there's two factors that really play into this constant absorption of more data. One is, in tech in general, there's this belief that more data equals better, data equals money. And so the more you can get, the better for you as a company. And the other flip side of that, when it comes to generative AI, is the belief that the more information that a model is trained on, the more that's in the dataset, the more specific and the more accurate and the more intelligent a system will appear in its output and what it's generating. And I think those two playing off of each other have really incentivized companies involved in generative AI to set the default as collect as much as possible, train on as much as possible. And so part of putting this report together was trying to provide a disincentive or a reason for companies to consider that not being the default, and why they need to look at, much more carefully, the amount of data and the type of data that they're taking in, and that they're generating and putting back into that cycle.
Justin Hendrix:
The risk you say is that the information that is perhaps not sensitive when it's spread across multiple databases or websites, could be extremely revealing when it's collected in a single place, which is effectively what these AI systems are doing. If they're hoovering up some large portion of the internet, of course they're essentially creating repositories that have never existed before. I mean we might argue perhaps that Google Search or other search engines in past have gotten close, but this seems to be putting a kind of interface layer on top of it, atop of all this information that kind of changes things a bit.
Calli Schroeder:
It's both the amount of data all collected in one place, and also that when you have that amount of data, there's the inferences that can be drawn from that. So sometimes even from different information points that are not sensitive in themselves, it's very easy to draw something that is extremely sensitive. So if I'm looking up, if people have information that I've been in a location where there's say an abortion clinic, and also saw that I was looking up work leave for medical procedures and things like that, you could make the inference that maybe I'm looking at having an abortion, which is very sensitive and personal from information that isn't necessarily by itself sensitive and personal. So it's also that factor of all of these information points coming together to create more risk for individuals.
Justin Hendrix:
You see six harms related to privacy and data collection. They range across physical, economic, psychological. And then three specific ways that generative AI systems may negatively impact individual autonomy. Can you take us through those?
Ben Winters:
I think at the very base level, the physical harms can be the fact that people's personal data is out there, as Calli just outlines that person considering or seeking an abortion may be able to be targeted. Same thing goes for the location or whether that's live or just more generally speaking, or the activities of domestic violence or stalking victims. And more generally, even if you have not yet been a victim of a crime, there is a really widespread targeting of people, particularly women. And so is there's a real physical safety harm to the fact that more and more data is out there and more at risk. That second one is economic loss. One interesting place where some halts about the adoption agenda of AI technology is actually from companies. I think Samsung is one of them. Because they're, as open AI for example, their chat GPT product learns from the inputs from their users.
And so people have input things that are trade secrets that they would never otherwise get out, and then that has been leaking elsewhere. So there's a real economic loss there for businesses. And that could also spread to individuals. Psychologically is related to the physical harm, to a certain extent. There is that fear, that knowledge that just like the fact that that type of precision of your location information or just information about where you live, things about you that you have either a right to remove in the past or just do not want to necessarily be connected to you et cetera, or just incorrect information. There is that increased and anxiety level at the very base sense, but also really warranted fear and high levels of anxiety if the data could impact them negatively if spread, especially given the wide risk of data abuse.
And in the three autonomy related harms for the privacy violations, the lack of control of your personal data, and the lack of ability to see what's in the training set, and take that out of the training set or in the system in general. That's a loss of autonomy. It's your data, it's information about you. If you cannot control where that's being used or how people can get access to that, whether directly or inadvertently through a output of a generative AI machine, that's really concerning. And there's been an example of a woman's medical records that was hoovered up into a photo generator. Then that person's literal face or record or something is out there onto something else's. They have zero control over that. Similarly, there there's a lack of autonomy in the fact that you are not aware at all that those, that data is being used.
There's not some tracking mechanisms where a certain piece of information about you is at these a hundred people. It's this diffuse wild, wild west where you could never really be confident about what's happening. And you certainly are not being asked by these companies, "Oh, is it okay if we use this data from you, this type of source." And the last one is related, especially given the likelihood and examples of chatGPT giving incorrect biographical information. There could be that loss of opportunity with, you saw the example of the law professor that was incorrectly listed as part of a search that was on chatGPT was like, "Can you give me a list of law professors that have been accused of sexual assault?" He was on it, but he had never been accused of that. So that could really, in addition to those psychological harms, really lead to loss of opportunity, loss of relationships, and really loss of control over how people are perceiving you.
Justin Hendrix:
Also get into various risks on data security, physical, economic, reputational, psychological, again, autonomy and discrimination. Discrimination's a topic that does come up in multiple ways throughout this report. You highlight risk to the environment, you highlight there, the excellent work on this by Sasha [inaudible 00:31:16] Talk a little bit about labor manipulation, theft, displacement. This seems like an area where there are still lots of question marks.
Calli Schroeder:
It definitely is, and it's interesting because I feel, at least from what I've been seeing in news reporting, labor seems to be one of the areas that is causing the most concern when it comes to questions, and about just being replaced by machines and by machine learning. We've seen already that there are newsrooms that have discussed or taken action on severely limiting or reducing their writers and reporters, and deciding to use generative text and generative articles. In Hollywood, there's a lot of real discussion about screenwriters being replaced, in some cases, by these systems. There's talk about editors being replaced or students using this kind of technology to generate more product. And so the problem is, not just that there's possibility of job loss, but there's also real harm possibilities in the monopolies in this market, because the early entrance degenerative AI frequently are these really large companies that are able to corner the market, not just on the development of these systems, but also on taking up all of the experts that are able to develop and audit and do ethics research in these systems.
So if everyone, that's an expert in this area, is working for one of say five companies, this space, it becomes much harder to have real discussions in this space where ideas are being challenged and people are allowed to speak freely without worrying that they're going to harm their own job prospects if they want to move to a different position or move to a different company. So I don't know, that's been a really interesting area to look into the lack of mobility for people that are specialized in this area and also looking at what jobs are going to be outsourced to machines in this sense and where you can trust machines to do that.
Because I think another aspect of the labor problem is that maybe there's too much trust that generative AI is able to take over these jobs and function at the same level that human laborers have been able to function. And we may not see what the downsides are of that or where these systems can't stand up to the same level that human workers have been until it's too late, and those jobs have already been eliminated or taken over and we see repercussions from it.
Ben Winters:
And the other main thing about the labor section that I think is a little bit undercovered, is the human labor, and often extremely exploited human labor, behind the creation and maintenance of these systems. And so there are data labelers and data annotators, which are basically paid to look through the raw data of these systems, whether those be text or photos. And one job is labeling what's in there so the system could train on that a little bit better because they don't know automatically.
And the second one, which there was a story but in Time about how open AI outsourced work to a company called SAMA, and they were paying workers in Kenya less than $2 an hour to label the most egregious text that was in the data set for openAI so that when it was to market, they would know some of the most egregious, most homophobic, most racist things, but first that is being forced of extremely low wage, exploited workers. They're subject to that, reading that, and given that responsibility. But then on the other side it's like, "Oh look at this shiny tool. It's a genius thing," but it's created by hundreds and hundreds of criminally underpaid workers.
Justin Hendrix:
One of the aspects of that story around SAMA that I feel like was undercovered was the apparent activity that SAMA was engaged in on behalf of chatGPT to collect what appeared to be images of child sexual abuse, sexual and violent images. I don't even really understand the legality of how it could be done, how a firm could potentially go on the open internet, collect these images and then essentially package them for a company to build classifiers. I feel like this is an under-reported question, and clearly these companies have to build these classifiers if they don't want these types of outputs to come out of these systems, but it seems like a real, real dark piece of this AI industrial labor landscape.
Ben Winters:
Absolutely. And it just exacerbates the extreme power disparity between the, as Calli said, the first movers that are these huge companies. The people that are coming out with these are Microsoft and Google, and those are the people that have the power to do it, and they're exploiting extremely underpaid workers at the bottom of the other side.
Justin Hendrix:
That is a part of this report that comes through, and I think other writing on this of course leads your mind in the direction of the devaluation of labor, heightened inequality. These are issues we've been contending with now for decades, but seems like these technologies are likely just going to push us further in that direction.
Calli Schroeder:
I think that's true, but I also think it's interesting looking at the spaces where generative AI seems to be most at risk of taking over jobs. So there has been uproar in the legal industry with the discussion that generative AI may take over contract writing and lawyers may all be out of a job, which I think that's overblown. But I also think it's really interesting that one of the industries that seems to be at risk of displacement is one that is so specialized and that does, previously at least, has taken a great deal of school and training and licensing. And also I imagine writers' rooms, journalists, things like that. It's this industry where you're supposed to have this background training and know the systems and know how to protect sources, and know all of these different requirements. And so it's interesting that what seems to be being replaced isn't what we have previously considered to be low level or entry level jobs. These are more specialized industries that are also at a high risk of replacement.
Justin Hendrix:
Well, I want to talk a little bit about forms of redress, and your recommendations. There are a ton of them in this. One in particular, you know talk about product liability law. You say that policymakers, plaintiff's attorneys, should explore ways common law and statutory product liability law regimes can apply to redress generative AI harms. There is a lot of hype and a lot of bullshit around what are effectively bullshit generators. And perhaps that's a good place to start. But let's talk a little bit about some of the other recommendations you have in this report. Maybe each of you give me your top three.
Calli Schroeder:
So first I think that businesses that are looking at using generative AI, not just businesses, businesses, government, anyone who's looking at using generative AI for a system, before bringing it at all needs to really seriously consider if generative AI is the best tool for whatever the goal is, in this case. I personally feel that generative AI is falling prey to a similar hype that blockchain did when it was first coming out, where it became such a buzzword that everyone just wanted to incorporate it right away, and was so thrilled about the promise of this new technology. But there are so many places where generative AI is being shoehorned into an area where it's not only not the best tool for the function, in many ways it's a much worse tool than what we've been using previously. So I think honest assessment there of where generative AI is appropriate and where it's not, is one of the key recommendations.
Another one is data mapping. If you're using a generative AI tool, you really need to map where is the information coming from that's going into that data set. You need to have a real clear awareness of what's in that data training set, how often more information is being added, whether that's automatic, whether that's reviewed. With the output, is that being fed right back into the system? Is that being checked at any level? Who is it going to? How is it being used? So the data mapping part of that, I think, is very important.
And then finally I would say doing impact assessments and audits to look at what's coming out of these systems, how are they functioning, and can you demonstrate, if you are claiming that this is an AI that's generating all this factual information, that's generating high level documentation, that doesn't have bias or discrimination in it, can you demonstrate, can you prove that your system doesn't have that stuff or is it all talk? I feel like all of these systems need to be able to actually back up their claims, forcing people to have audits or assessments, where there's a tangible document where you can show the work you've gone through to prove this is the first step there.
Justin Hendrix:
How about you Ben?
Ben Winters:
So I feel like the part of generative AI that I feel is most concerning to me right now is its use in misinformation and disinformation and scams, a lot of the, what we call, information manipulation in the paper. And so I think our number one recommendation right now is to pass a law at the federal level that makes the intimidation, deception or misleading about an election or a candidate, whether that be, "Hey, it's actually good to vote on Wednesday. The different parties vote on different days, or LeBron James endorses Donald Trump," and you can do this voice with generative AI. That should be made illegal and there should be a private right of action so that the person harmed can take it into their own hands and sue the parties that are responsible. And one bill that was introduced in past years is called the Deceptive Practices and Voter Intimidation Prevention Act.
So exactly that. That was not created with generative AI in mind. And we have seen this for years and years that people are doing these types of messages, and that they're targeted at certain communities, but generative AI is just going to make that easier and faster and more likely and less hard to discern. So that's probably number one, to pass that law, to make it very clear that that is illegal. It's pretty wild that is not a law right now, at least in my point of view. Second would be to, under the use of a combination of either rulemaking at the Federal Trade Commission or legislatively requiring some sort of data minimization standard. Data minimization, at the very basic sense, stands for the concept of that you only collect the data that you need for the product or service that the consumer requested.
You don't just get to hoover up more data, just like we were talking about earlier. And that takes a lot of different forms exactly how it's written, but it's a part of privacy laws like ones that have passed in Colorado and California and the states, and in the proposed American Data Privacy Protection Act, which passed out of committee last Congress, and is hopefully being able to get past this year. But that is a really essential way to try to stave off and make clear that it's illegal to do this, just extra hoovering up. And then lastly, I think there should be requirement, whether that be informally through conferences and papers or formally through a government administration. Publish information about the environmental footprints of generative AI models and the continued use.
So there is a substantial amount of water and energy required to do the training and cooling of computing systems, or the amount of time that you need to process all of this data. There's data centers that need to be done. And the more people use it, the more energy and rare minerals are used for certain computing systems. And while we're in the middle of a increasingly worsening climate crisis, it's really concerning that that is not a major concern with the increased use of generative AI. So really need to be, at the very least, clear and published information about it.
Justin Hendrix:
The American Data Privacy and Protection Act does appear multiple times throughout this report in its recommendations. I'm surprised that more people aren't making connection between the generative AI hype cycle and just the urgent need for some federal privacy legislation, particularly in this country. I'm talking to the two of you today. You both happened to be in Brussels. Of course the EU has, on some level, already addressed this fundamentally, but here we're essentially still flying blind.
Ben Winters:
Yeah, we are with you there. I think it maybe hasn't yet caught on just because it's still a fairly new, and the way it's being messaged about is extremely overwhelming, and there's been a successful effort to really make the focus on these harms that are really far away and talk about these time minded, different agency, different licensing schemes, the things that Sam Altman is trying to say in front of Congress. But yeah, you can use the solutions that are required for the problems we've had for the last 10, 15 years and start to address agenda of AI.
Justin Hendrix:
It just occurs to me that all sorts of things that have always been a problem and important in the conversation about tech, just are more important in the context of AI. Even things like encryption, the idea that perhaps we need to preserve encryption, not just to protect communications and the safety of the transfer of information from prying eyes, and perhaps authoritarian government interests, but also just from AIs that might want to hoover up information as it transits the internet. But I'll ask you maybe just the last question since you've, I suppose, referred in some way to the last 10 or 15 years in some of the fights we've been in on tech and on tech rights and digital rights, EPIC's been around since 1994. I think that's just right around the time of the advent of the worldwide web. We're clearly moving into a different phase at this point. Your goal has always been to preserve data privacy. You see that as core to democracy. How's that going? In the age of AI, how are you feeling about the remit of your work? Is it getting harder, essentially?
Calli Schroeder:
I think both yes and no. I think it's getting harder in that whenever there's new technology and new challenges to privacy, and new ways that data can be absorbed and generated, the speed and the volume is what is really challenging for us. The speed that these different technologies collect, reuse, take inferences from and bring up new challenges and new risks in privacy, that is always really challenging. But I think some of the problems that we see, it tends to be the same old privacy problems just repackaged in another form over and over. So in many ways, the fights for digital rights and the discussions about control over information and human autonomy and privacy and privilege and bias, democracy, all of that, those arguments tend to stay fairly consistent. It's just the way that we apply them that keeps evolving.
Ben Winters:
The one thing I'll add, and maybe try to end on a slightly hopeful note, is that I think that with the increase of, there's an increasing awareness about certain data privacy abuses. So there is Cambridge Analytica, there is all sorts of... There's Clear View. So people are getting more and more examples and seeing it in their everyday life, some of the concerning or risky things. So I think that more and more it's going to be more politically palatable to people. And so hopefully, I think the IAPP, a great resource, has this report that shows that from 2018 there was two state privacy bills and the whole country introduced, and last year there was like 60. So that's five years. There's been insane growth. And not every bill is particularly good or particularly strong, but that is reflective of the fact that even elected representatives are noticing that it's something they have to address. As Calli said, the same sort of things remain a problem and remain what we're talking about, but I think we're getting a little bit closer in connecting with more people.
Justin Hendrix:
An optimistic note to end on. I thank you both, and would recommend that everyone go and take a look at this report, which is on, of course, the EPIC website. Again, its title is Generating Harms, generative AI's, impact and Paths Forward. That's at epic.org. Thank you both so much for joining me.
Ben Winters:
Thank you, Justin.
Calli Schroeder:
Thank you for having us.
Authors
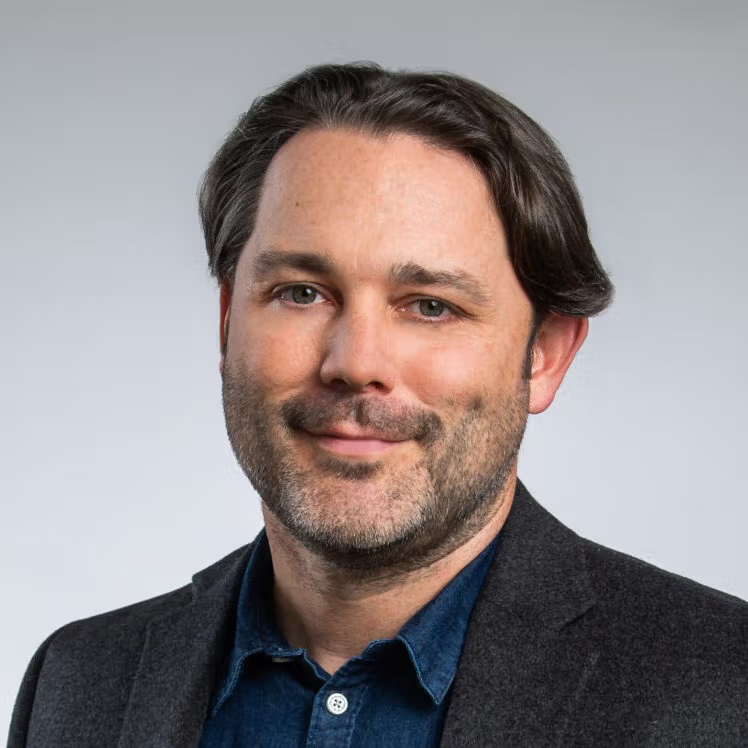