Responsible AI as a Business Necessity: Three Forces Driving Market Adoption
Marianna B. Ganapini, Renjie Butalid / May 13, 2025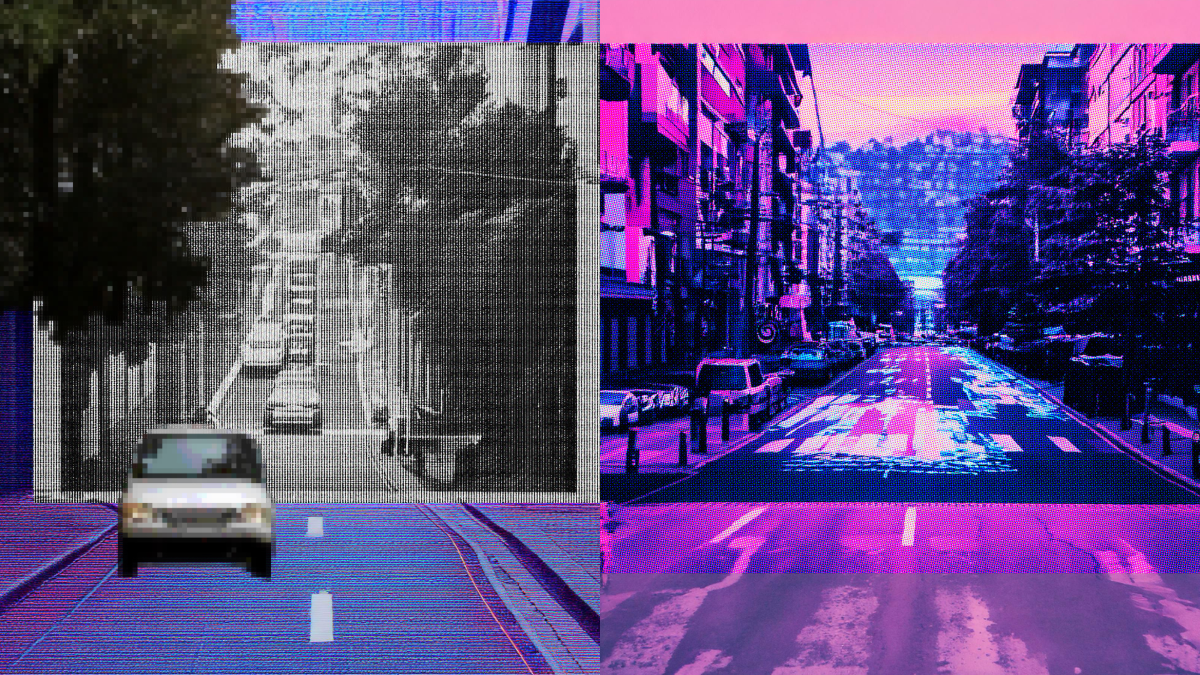
Is This Even Real II by Elise Racine / Better Images of AI / CC by 4.0
Philosophical principles, policy debates, and regulatory frameworks have dominated discussions on AI ethics. Yet, they often fail to resonate with the key decision-makers driving AI implementation: business and technology leaders. Instead of positioning AI ethics solely as a moral imperative, we must reframe it as a strategic business advantage that enhances continuity, reduces operational risk, and protects brand reputation.
In this article, we identify three primary drivers propelling the adoption of AI governance: top-down regulation, market pressure, and bottom-up public influence (Figure 1). When these forces converge, we contend that companies will increasingly treat responsible AI as a business necessity rather than an ethical ideal, creating an ecosystem where corporate incentives align with societal interests.
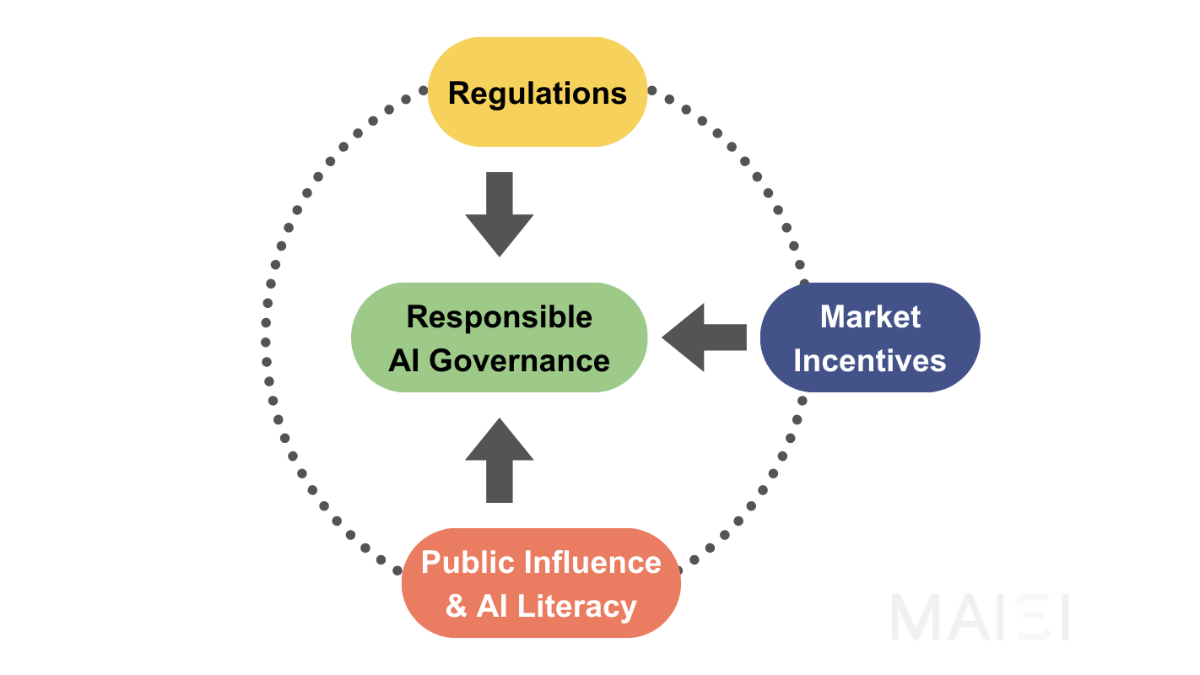
Figure 1: Converging forces driving responsible AI governance adoption.
The top-down regulatory landscape: setting the rules
Most AI regulations efforts globally have established risk-tiered approaches to regulation. The EU AI Act, for instance, categorizes AI applications by risk level, imposing stricter requirements on high-risk systems while banning certain harmful uses. Beyond legal obligations, standards such as ISO 42001 offer benchmarks for AI risk management. Meanwhile, voluntary frameworks such as the NIST AI Risk Management Framework provide guidelines for organizations seeking to implement responsible AI practices.
The complexity of EU regulatory compliance presents numerous challenges, especially for startups and small to medium-sized enterprises (SMEs). However, compliance is no longer optional for companies operating across borders. American AI companies serving European users must adhere to the EU AI Act, just as multinational financial institutions are required to navigate regulatory jurisdictional differences.
Case Study: Microsoft has proactively aligned its AI development principles with emerging regulations across different markets, enabling the company to rapidly adapt to new requirements, such as those outlined in the EU AI Act. Amidst rising geopolitical volatility and digital sovereignty concerns, this regulatory alignment enables Microsoft to mitigate cross-border compliance risks while maintaining trust across jurisdictions. This approach has positioned Microsoft as a trusted provider in highly regulated industries, such as healthcare and finance, where AI adoption heavily depends on assurances of compliance.
The middle layer: market forces driving responsible AI adoption
While regulations establish top-down pressure, market forces will drive an internal, self-propelled shift toward responsible AI. This is because companies that integrate risk mitigation strategies into their operations gain competitive advantages in three key ways:
1. Risk management as a business enabler
AI systems introduce operational, reputational, and regulatory risks that must be actively managed and mitigated. Organizations implementing automated risk management tools to monitor and mitigate these risks operate more efficiently and with greater resilience. The April 2024 RAND report, “The Root Causes of Failure for Artificial Intelligence Projects and How They Can Succeed,” highlights that underinvestment in infrastructure and immature risk management are key contributors to AI project failures. Mature AI risk management practices are critical not only for reducing failure rates but also for enabling the faster and more reliable deployment of AI systems.
Financial institutions illustrate this shift well. As they move from traditional settlement cycles (T+2 or T+1) to real-time (T+0) blockchain-based transactions, risk management teams are adopting automated, dynamic frameworks to ensure resilience at speed. The rise of tokenized assets and atomic settlements introduces continuous, real-time risk dynamics that require institutions to implement 24/7 monitoring across blockchain protocols, an approach now being evaluated for broader adoption by institutions such as Moody’s Ratings.
2. Turning compliance into a competitive advantage: the trust factor
Market adoption is the primary driver for AI companies, while organizations implementing AI solutions seek internal adoption to optimize operations. In both scenarios, trust is the critical factor. Companies that embed responsible AI principles into their business strategies differentiate themselves as trustworthy providers, gaining advantages in procurement processes where ethical considerations are increasingly influencing purchasing decisions.
According to PwC’s 2024 US Responsible AI Survey, 46% of executives identified responsible AI as a top objective for achieving competitive advantage, with risk management close behind at 44%.
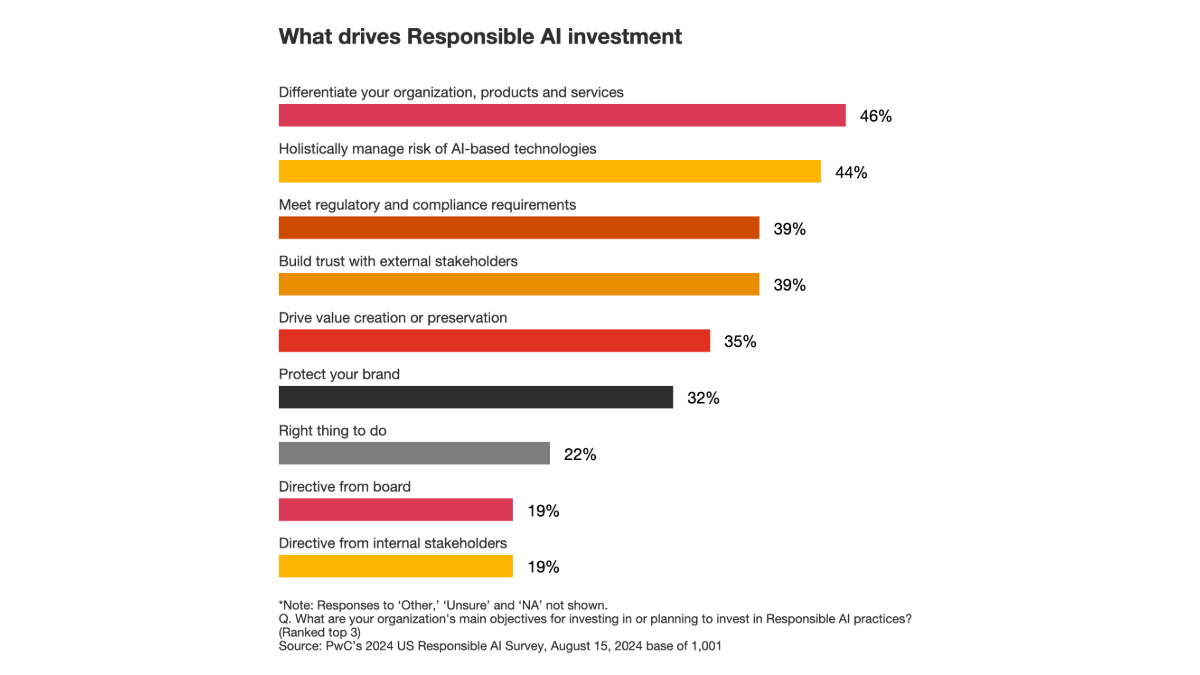
Figure 2: A graph depicting the drivers of responsible AI investment.
3. Public stakeholder engagement as a growth strategy
Stakeholders extend beyond regulatory bodies to include customers, employees, investors, and affected communities. Engaging these diverse perspectives throughout the AI lifecycle, from design and development to deployment and decommissioning, yields valuable insights that improve product-market fit while mitigating potential risks.
Organizations that implement structured stakeholder engagement processes gain two key advantages: they develop more robust AI solutions that are aligned with user needs, and they build trust through transparency. This trust translates directly into customer loyalty, employee buy-in, and investor confidence, all of which contribute to sustainable business growth.
Partnership on AI's 2025 Guidance for Inclusive AI - Practicing Participatory Engagement highlights trust as an often underestimated advantage of participatory engagement:
“When companies actively involve the public — whether users, advocacy groups, or impacted communities — they foster a sense of shared ownership over the technology that’s being built. This trust can translate into stronger consumer relationships, greater public confidence, and ultimately, broader adoption of AI products.”
The bottom-up push: public influence and AI literacy
Public awareness and AI literacy initiatives play a crucial role in shaping expectations for governance. Organizations like the Montreal AI Ethics Institute and All Tech is Human equip citizens, policymakers, and businesses with the knowledge to evaluate AI systems critically and hold developers accountable. As public understanding grows, consumer choices and advocacy efforts increasingly reward responsible AI practices while penalizing organizations that deploy AI systems without adequate safeguards.
This bottom-up movement creates a vital feedback loop between civil society and industry. Companies that proactively engage with public concerns and transparently communicate their responsible AI practices not only mitigate reputational risks but also position themselves as leaders in an increasingly trust-driven economy.
Case Study: As referenced above, the Partnership on AI's 2025 Guidance for Inclusive AI - Practicing Participatory Engagement also explicitly recommends consulting with workers and labour organizations before deploying AI-driven automation. Early engagement can surface hidden risks to job security, worker rights, and well-being, issues that might otherwise emerge only after harm has occurred.
Moving beyond voluntary codes: a pragmatic approach to AI risk management
For years, discussions on AI ethics have centred on voluntary principles, declarations, and non-binding guidelines. However, as AI systems become increasingly embedded in critical sectors, such as healthcare, finance, and national security, organizations can no longer rely solely on high-level ethical commitments. Three key developments will define the future of AI governance:
- Sector-specific risk frameworks that recognize the unique challenges of AI deployment in different contexts. Organizations that understand how governance applies to their specific use cases will gain a competitive advantage in procurement processes.
- Automated risk monitoring and evaluation systems capable of continuous assessment across different risk thresholds. These systems will enable organizations to scale AI governance across large enterprises without creating prohibitive compliance burdens.
- Market-driven certification programs that signal responsible AI practices to customers, partners, and regulators. As with cybersecurity certifications, these will become essential credentials for AI providers seeking to build credibility in the marketplace.
The evolution of cybersecurity from an IT concern to an enterprise-wide strategic priority provides a useful parallel. Twenty years ago, cybersecurity was often an afterthought; today, it's integral to business strategy. AI governance is following a similar trajectory, transforming from an ethical consideration to a core business function.
Conclusion: responsible AI as a market-driven imperative
The responsible AI agenda must address market realities: as global AI competition intensifies, organizations that proactively manage AI risks while fostering public trust will emerge as leaders. These companies will not only navigate complex regulatory environments more effectively but will also secure customer loyalty and investor confidence in an increasingly AI-driven economy.
Looking forward, we anticipate the emergence of standardized AI governance frameworks that balance innovation and accountability, creating an ecosystem where responsible AI becomes the default rather than the exception. Companies that recognize this shift early and adapt accordingly will be best positioned to thrive in this new landscape where ethical considerations and business success are inextricably linked.
Authors
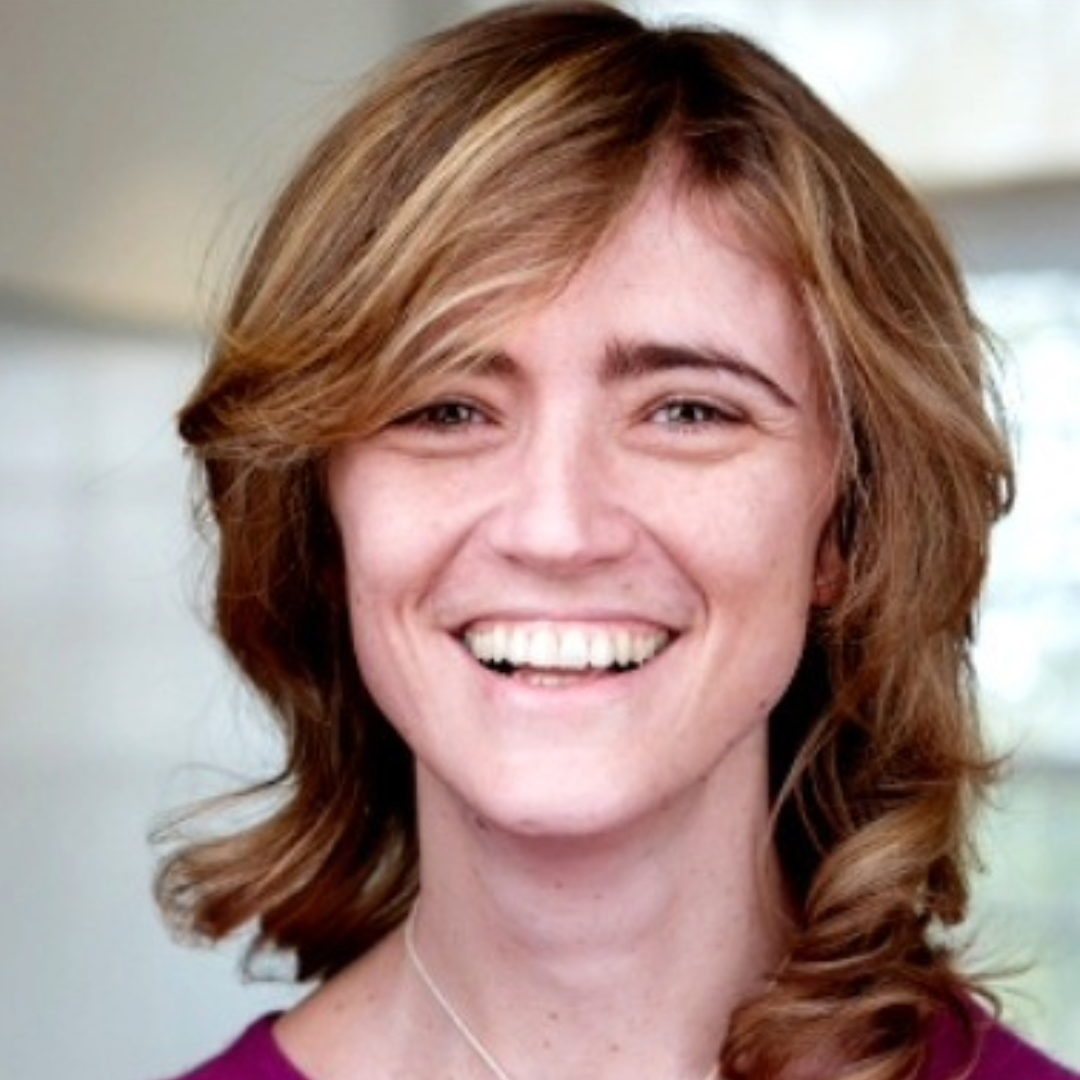
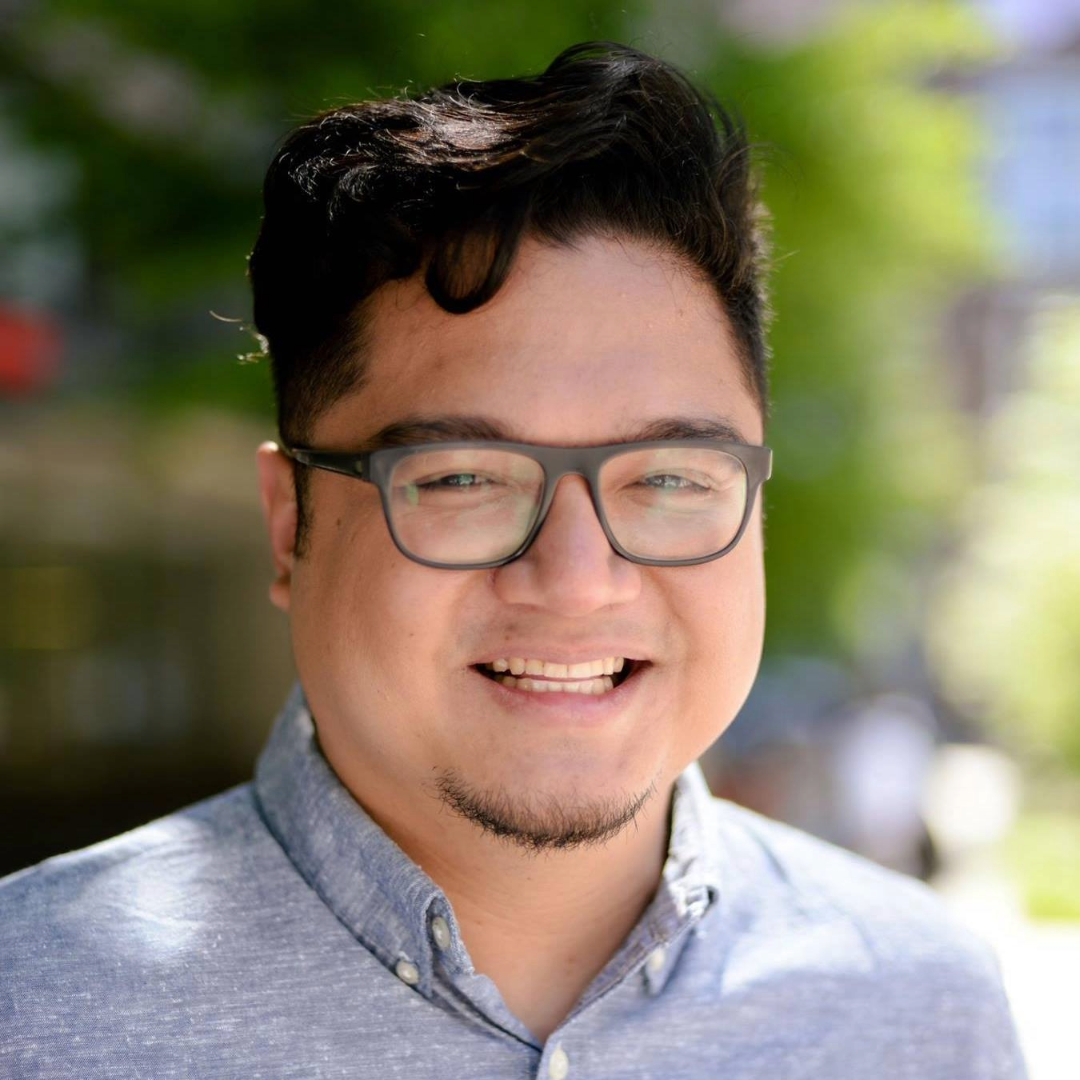