Participatory AI? Begin with the Most Affected People
Meg Young / Feb 19, 2025This essay is part of a collection of reflections from participants in the Participatory AI Research & Practice Symposium (PAIRS) that preceded the Paris AI Action Summit. Read more from the series here.
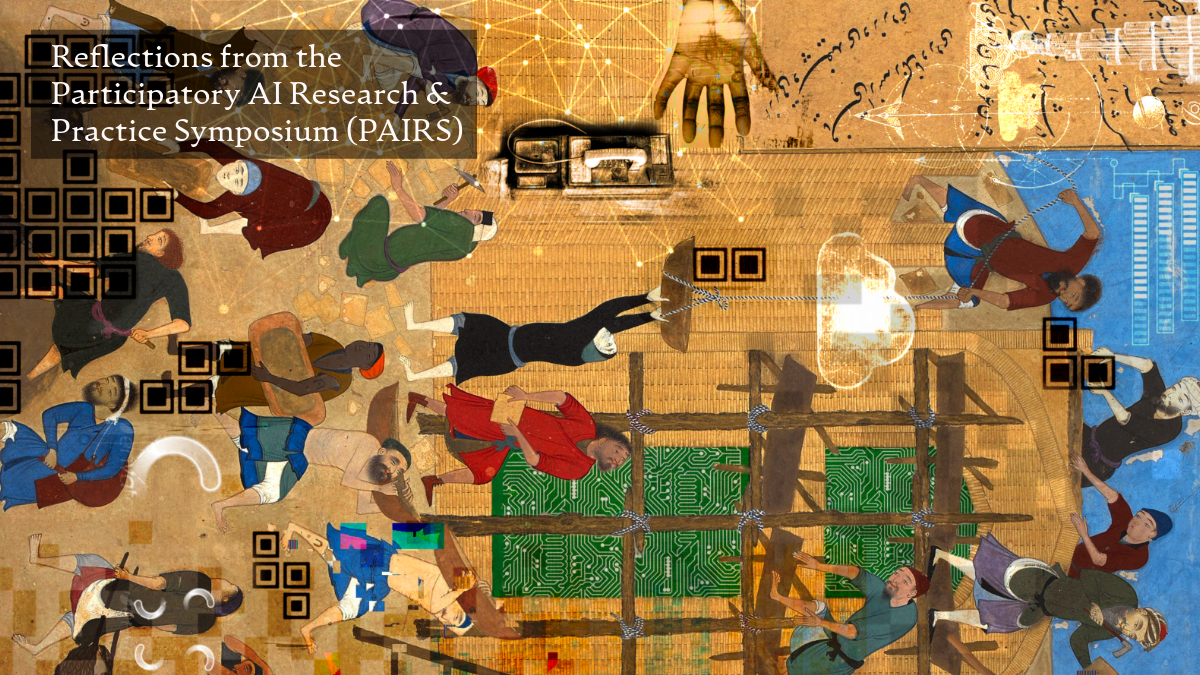
Shady Sharify / Better Images of AI / Who is AI Made Of / CC-BY 4.0
Every day, we are confronted with AI’s presence in a new part of our lives. Welcome or unwelcome, these changes are almost always imposed from the top down. What would it mean for AI to be created from the “bottom-up?” That goal—for the public to have more determinative input into AI design and governance—has become the focus of a stream of research and development known as “participatory AI.”
Before the Paris AI Action Summit on February 10-11, 2025, civil society organizations from the UK, US, and India met at Sciences Po to host the Participatory AI Research & Practice Symposium. They shared work from academic labs, civil society organizations, and companies. While the 250 people present attest to the growing interest in this area, a key question remains: what does “participatory AI” really mean? How might the vision of public power in AI be realized?
To advance this goal, it is essential for the field to prioritize input from people who will be impacted by a proposed system, especially those who (because of their identity, profession, or socio-economic status) are most likely to be harmed by its use. AI tools have a history of failures leading to discrimination, labor displacement, or administrative violence; seeking input from those closest to these risks will not (and does not claim to) represent “all” people but will yield specific, actionable changes to systems that will, in turn, protect the rights and interests of everyone else.
However, not all participatory AI work today adopts this approach. Some practitioners prioritize input from the general public; others focus on users—rather than the broader set of people impacted by a system. One source of this divergence is that participatory methods used today encompass a long history of wholly distinct traditions; as practitioners draw from different genealogies of work, this is reflected in our different ways of realizing the broader vision for AI.
First, some work follows fields like education, social work, and urban planning, which have used “participatory action research” and “community-based participatory research” since at least the 1970s. These methods have a strong commitment to shifting the locus of decision-making power to the people most immediately impacted by a policy or other project. These methods tend to produce smaller, bespoke systems to respond to community-identified needs. They also rely on durable partnerships and relationships, such as between academics and community-based organizations, to shape systems over time. However, it can be challenging to apply these methods at the scale of commercial AI, which operates across vast geographies and impacts innumerable communities.
A second tradition of work draws from fields like human-computer interaction to use “participatory design”—methods conceived in the Scandinavian labor movements of the 1970s to promote worker empowerment, but which have since trended toward a more instrumentalizing goal of improving products for a specific context of use. Participatory AI based on these methods can adapt existing products, such as large language models for different cultural contexts. In this way, it is more compatible with the needs of tech companies. However, it often emphasizes users without considering the broader array of impacted people. Its focus on improving existing products also means that people have fewer opportunities to re-direct or reshape the premise of the system in question or shape the goals of the deployer. This dynamic can also feel extractive.
A third tradition used in participatory AI comes from political philosophy—especially methods for deliberative democracy like citizens’ assemblies. It primarily focuses on the question of legitimacy: what are defensible processes for making decisions on behalf of the public? These methods rely on selecting a random, stratified sample of the general public for demographic representativeness. The goal of consulting this subset of people is primarily to represent a wide range of perspectives and to resolve diverging views through dialogue. For example, Meta and Stanford’s Deliberative Democracy Lab recently collaborated on community forums featuring thousands of participants from all over the world and produced polling results on participants’ opinions. For instance, they asked whether respondents thought that users should have romantic relationships with AI chatbots. Projects like these achieve admirable scale but often produce input and reason at a high level of abstraction. This approach may also risk diluting the concerns of people most impacted by a given system with the opinions of those without as much stake in the final decision.
To be sure, there are deep tensions between centering impacted communities’ input and the way most technology developers are organized today. Both firms and governments tend to centralize decision-making power and deploy a single system at scale. However, these tensions are not irresoluble; eliciting impacted communities’ input can support true innovation by directing technology development to the problems identified on the ground—rather than those imposed from the top down. The vibrant work at the Symposium last weekend demonstrated that AI is more valuable this way, directed by impacted communities’ insight and expertise. It’s time for technology companies, governments, and public interest technologists to follow their lead.
Authors
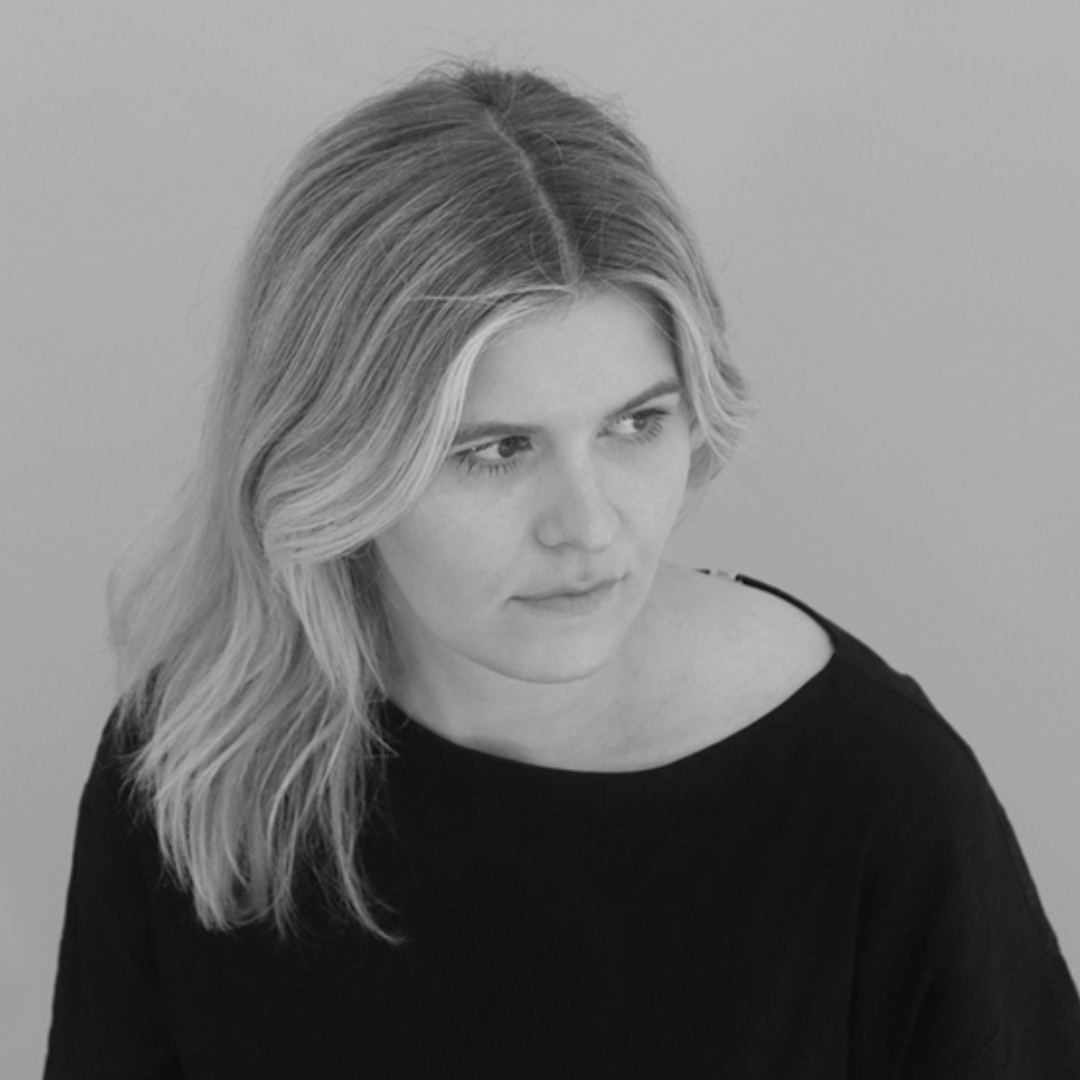