No Perfect Solution to Platform Profiling Under Digital Services Act
Claire Pershan, Jesse McCrosky / Aug 25, 2023Jesse McCrosky is Thoughtworks’ Head of Responsible Tech for Finland and a Principal Data Scientist. Claire Pershan is EU Advocacy Lead for the Mozilla Foundation.
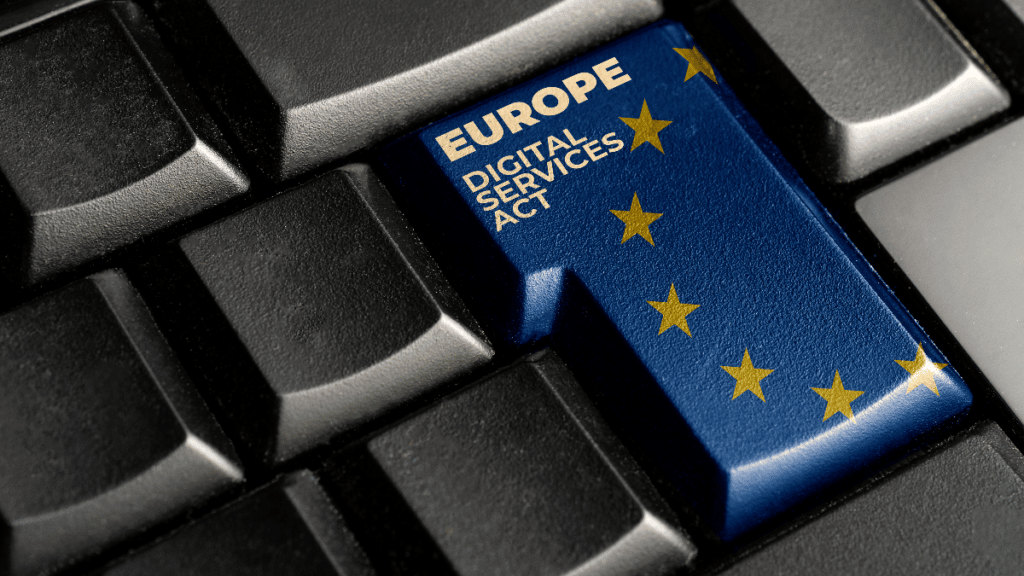
Regulators in Europe are requiring platforms to provide greater transparency and user control over profiling for recommender systems, including those targeting advertising. The EU’s Digital Services Act (DSA) sets out rules to increase advertising transparency and to give users more insight and control over their content recommendations. Many of the DSA’s requirements for the largest online platforms and search engines take effect on August 25, and compliance-related announcements from the designated services are already rolling in. For instance:
- Article 26 of the DSA demands that platforms show users meaningful information about the “main parameters” used in targeting and ways to modify these. It also calls for a ban on targeted advertising based on profiling using special categories of personal data identified in Article 9 of in the EU’s General Data Protection Regulation (GDPR), such as data that would reveal an individual’s race, religion, or health status.
- With respect to recommender systems, Article 27 requires platforms to clearly explain the main parameters that influence what information is suggested to users, and ways to modify these.
- Article 28 requires the largest online platforms and search engines to provide users with at least one recommender system option “not based on profiling” of individuals by automated systems as defined in the GDPR.
These requirements were hard fought by platform accountability and privacy experts during the negotiations on the DSA. Now the next challenge is meaningful implementation by platforms. This may not be straightforward, however, since behind targeted recommendations are machine learning models which rely on ‘profiling.’ These systems are designed to discriminate between users, and may in fact produce ‘unintended inferences’ that are difficult to mitigate, making compliance a challenge.
Targeting and Microtargeting
To understand these requirements, we need to better understand the nuances of how content is targeted; ad targeting is an illustrative example that will also help us understand organic content. We can generally think of advertisements being targeted in two layers (see The Inherent Discrimination of Microtargeting):
- In the first, the advertiser selects “an audience” using explicit targeting parameters: demographics, interests, location, etc. The platform uses user profile information, whether user-provided or inferred, to select users that are eligible to be served the advertisement. If the advertiser wants to target 18-25 year old women interested in gardening, the platform will do its best to select a matching audience.
- In the second layer, the platform will show the ad to individual users within the audience; however, it will use machine learning systems to select those individual users who it predicts are most likely to click on the ad.
This first layer of targeting may be limited to fairly coarse-grained targeting parameters. However, the second layer will generally use all the data the platform has available – a detailed profile of every user – based on likes, browsing history, and any other data that the platform has managed to capture.
This second layer is referred to as microtargeting. Non-advertising content (or “organic content”) is also typically microtargeted – for example, Facebook might surface posts from your friends that you are most likely to “like,” and YouTube might recommend the videos it predicts you are likely to spend the most time watching.
To provide transparency into advertisement targeting as required by the DSA, it is fairly simple to provide information about the first layer: this likely amounts to what characteristics the advertiser chose to target. However, as we see below, the second layer also influences what sorts of users will see an ad. Even without the advertiser or the platform’s direct knowledge, certain unintended inferences may occur, producing a sort ofautomated discrimination. For this reason, it is also very difficult to prevent targeting according to particular characteristics.
Unintentional Inferences
This has implications for personalization-related DSA obligations, especially Article 26 requiring user-facing explanations of their ad targeting and prohibiting targeting based on sensitive personal data. There are a range of interpretations, but it seems reasonable to expect that if an ad is shown primarily to users of a certain gender, or ethnicity, or political orientation, that these characteristics constitute “main parameters” and should therefore be disclosed, even if there is no intention from either the advertiser or the platform to make that targeting decision.
Indeed, it is possible that an ad is unintentionally shown targeted to users based on their personal characteristics. This is possible because of unintentional inferences.
An unintentional inference is a case in which a recommender system can recommend different content to different sociodemographic or otherwise defined groups. To simplify, we’ll call this discrimination and use gender as an example grouping. These unintentional inferences occur without the platform having any data on their users’ gender and, critically, without having any way of knowing that the discrimination is taking place. The system can discriminate on the basis of gender without knowing the gender of a single user.
How is this possible? Say for example that we have a set of users and the only information that we have about them is their hair length. We have an advertisement that we wish to show to those users that are most likely to click on it, and so we start by showing it to a few users at random and find that those with short hair tend to be much more likely to click on it than those with long hair. So we start showing it primarily to users with short hair.
Now we know that there are norms around gender expression and hair length, so we can see that the system has probably learned that men are more likely to click on the ad than women. It doesn’t have to be perfectly accurate – there can be some short-haired women and long-haired men in the population, but by learning to target short-haired users, the system will still tend to target more men than women.
This system has now learned to discriminate based on gender, without actually knowing the gender of any of its users. The relationship between hair length and gender expression is well-known, so it’s not hard to figure out what the system is doing. But this effect occurs in much more complex ways. The sophisticated data that platforms hold on content that users like, interaction patterns, location check ins, etc. is rich enough to produce unintentional inferences on pretty much every characteristic one can imagine, including in relation to sensitive categories of personal data under the GDPR like race and ethnic origin. The inferences are imperfect, but good enough to mean that content that is more likely to be engaged with by a group will mostly be shown to that group.
Indeed, as described in The Inherent Discrimination of Microtargeting:
Platform data, including Facebook likes and location check-ins, have been shown to be highly predictive of sensitive user characteristics. In one study, Facebook likes were found to be highly predictive of sexual orientation, political orientation, and membership in certain ethnic groups. Another showed that location check-in data is highly predictive of gender, age, education, and marital status. What this suggests is that when content is being targeted based on platform data, it is also, in many cases, simultaneously and implicitly targeted based on protected characteristics such as disability, gender reassignment, pregnancy, race, religion or belief, and sexual orientation.
Possible solutions
A platform with adequately rich data will inevitably tend to discriminate on many characteristics, including sensitive characteristics under the GDPR. What can be done to provide transparency into this process or prevent this discrimination? There are two general options: either “more data” or “(much) less data.”
More data
Paradoxically, to prevent discrimination on a given characteristic (gender, for example), the platform would need to collect data on its users’ gender.
If gender is known for every user, statistical methods can be used to ensure that, for those users that do not want their gender to influence their recommendations, that wish can be respected. And the degree to which gender influences recommendations can be analyzed allowing transparency into how gender influences recommendations. Without knowing the users’ gender, this is impossible.
Alternatively, if data on gender is collected for at least a representative sample of the users, it is then possible for the platform to statistically model the gender of their users and turn the unintentional inference into an intentional inference. This creates a similar situation to having data on the gender of all users, except that there will be some inaccuracies, which may result in some residual discrimination on gender, even when the platform is trying to prevent it.
This sets up a core tension between privacy and fairness. The more data that is collected, the more effectively discrimination can be prevented. In order to prevent discrimination on characteristics of interest, the platform must collect and hold data on those characteristics of their users. Even if this data is not used for any purpose other than preventing discrimination, there are still privacy risks: the data may be subject to government subpoena or acquired by hackers in a security breach.
This will also never be a complete solution. There are many personal characteristics that a system may discriminate on. It is impossible to consider them all, and certainly impossible to collect data on them all. Only a discrete list of characteristics can be handled this way, and not without privacy risks.
(Much) Less data
Alternatively, data collection can be strictly limited. This approach protects privacy, although perhaps at the expense of user experience. The unintentional inference of personal characteristics depends on the richness of the data used by the system. If the data is sufficiently lean, unintentional inferences will not occur, or if they do occur, they will be much weaker and thus less accurate. However, in order to evaluate whether an inference is occurring or not, data on the characteristic of interest is needed, as in the “more data” section above.
To extend our example above about hair length and gender, if the system were to collect data on some users’ gender, it would then be easy to see that males were being targeted at a greater rate than females. The platform might then decide they need to stop collecting data on hair length in order to prevent this discrimination. Thus, we see that the “less data” option is really a hybrid of “less and more data.”
If we want a purely “less data” option, we need much less data. Mozilla’s Lean Data Principles provide a valuable framework for minimizing data collection. Platforms in scope for the DSA’s most stringent requirements are beginning to announce compliance measures, including with the DSA requirement for a recommender system option not based on profiling (Article 38).
On August 4th, TikTok announced it will soon give EU users the ability to “turn off personalisation”:
This means their For You and LIVE feeds will instead show popular videos from both the places where they live and around the world, rather than recommending content to them based on their personal interests. Similarly, when using non-personalised search, they will see results made up of popular content from their region and in their preferred language. Their Following and Friends feeds will continue to show creators they follow, but in chronological order rather than based on the viewer's profile.
In this case, the only data collected and used about TikTok’s users seems to be their coarse-grained location and language settings. This data is inadequately rich for unintended inferences to be a major concern, and so appears to be a satisfying solution from a privacy and discrimination standpoint.
This is an early announcement and the actual roll-out must be monitored with respect to DSA compliance and user uptake. Some speculate this is unlikely to be a widely satisfying solution for many of TikTok’s users, since TikTok’s value proposition stems largely from its highly personalized feed.
Meta also announced DSA compliance measures for content ranking on Facebook and Instagram.
We’re now giving our European community the option to view and discover content on Reels, Stories, Search and other parts of Facebook and Instagram that is not ranked by Meta using these systems. For example, on Facebook and Instagram, users will have the option to view Stories and Reels only from people they follow, ranked in chronological order, newest to oldest. They will also be able to view Search results based only on the words they enter, rather than personalised specifically to them based on their previous activity and personal interests.
Similar to TikTok’s non-personalised option, this appears to use adequately lean data that unintentional inferences will not be an issue, except that the set of people that a user follows may in some cases create inferences – ie: do people tend to follow mostly others of their own ethnicity? To the extent that Facebook and Instagram have a more robust concept of following, though, a chronological feed here may provide a better user experience than TikTok’s option.
Overall, to what degree can platforms provide a personalized feed while still reducing privacy invasions and discrimination? We propose an approach that would need to go beyond the provisions of the DSA.
User Control Instead of User Monitoring
The data used by platforms like TikTok, Facebook, and Instagram can be divided into two broad categories:
- There are “implicit feedback” or “behavioral signals” in which the user’s behavior is monitored. This includes signals like how long the user watches a video before swiping to the next or how long the user continues using the app after watching a given video.
- There is also “explicit feedback” in which the user is intentionally providing information in an effort to curate their recommendations. The TikTok “filter video keywords” feature is an example of a pure explicit feedback user control. Likes and dislikes are also a form of user control.
If a platform were to recommend content purely based on explicit signals, problems of unintended inferences can still occur, but at least they would be based on signals that the user has willingly provided to the platform with an understanding that they will be used for personalization.
As our Mozilla study of YouTube has shown, existing user feedback tools do not provide meaningful control. Putting users truly in control may be a better or ultimately more viable solution than preventing profiling altogether. This will not prevent unintended inferences, but at least users will have meaningful control over the data they provide, making this a fairer trade.
Conclusion
The DSA demands transparency of targeting parameters, increased user control over targeting settings, and an end to targeting of ads based on the sensitive characteristics. In order to prevent (or make transparent) targeting on personal characteristics, the only sure solution is to dramatically reduce the data collected and processed. As a partial solution, collection of data on characteristics of concern can improve transparency and control over targeting, at the expense of increased privacy risks. As an alternative, platforms that target based exclusively using explicit user feedback would at least put users in control of the data they provide.
Authors
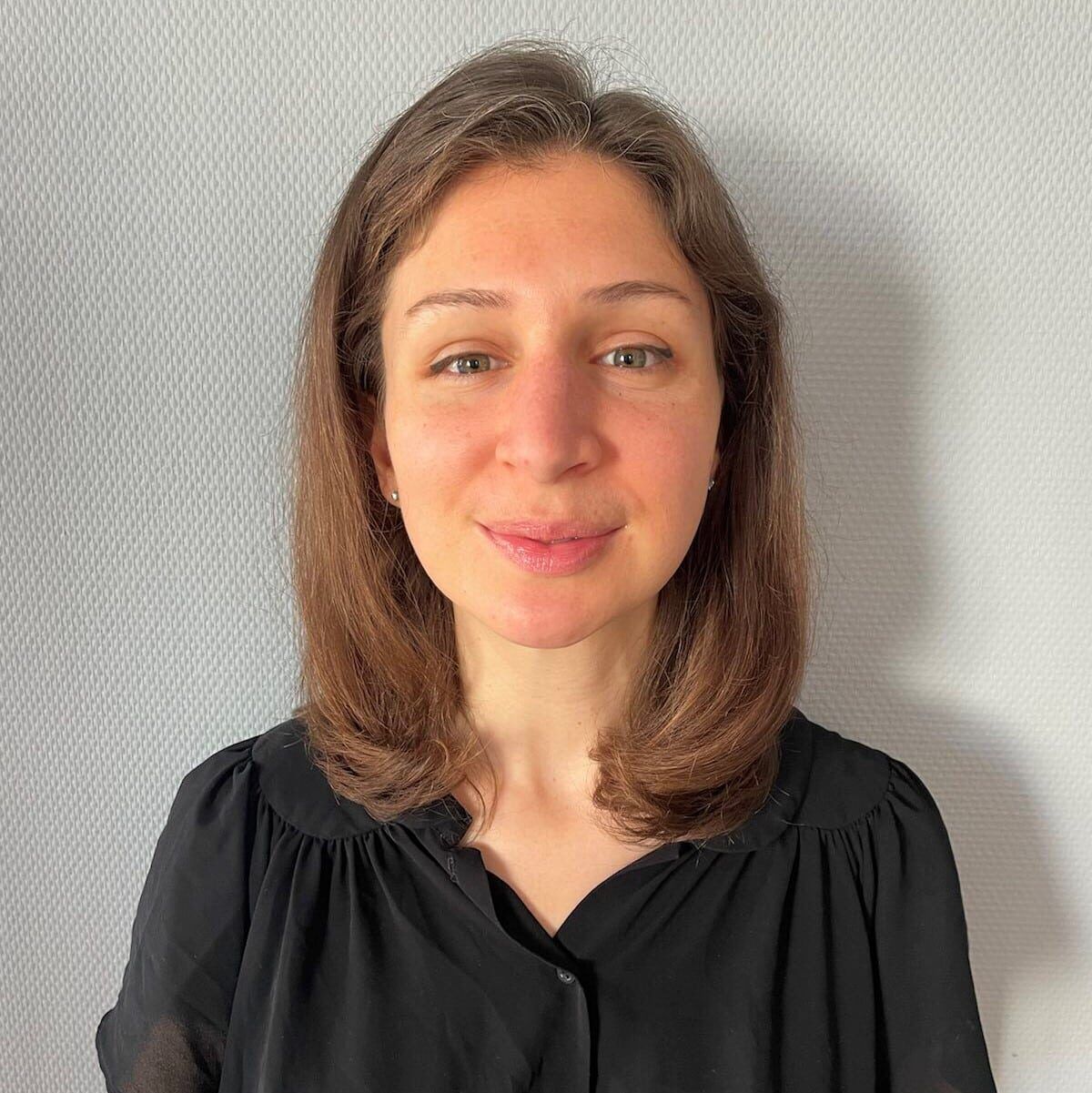
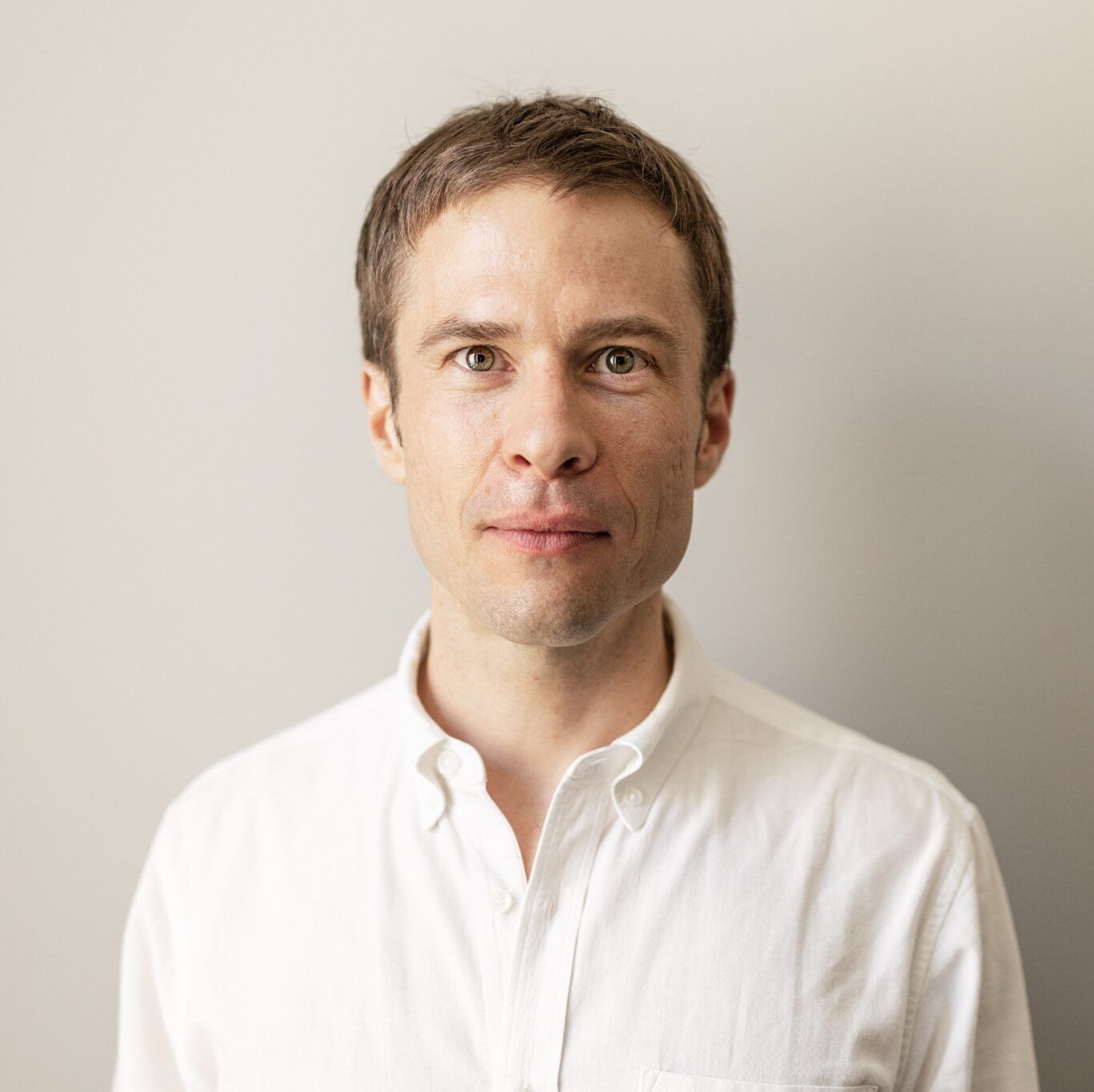