New Study Identifies Dynamics of “Stop the Steal” Images on Twitter
Justin Hendrix / Sep 29, 2022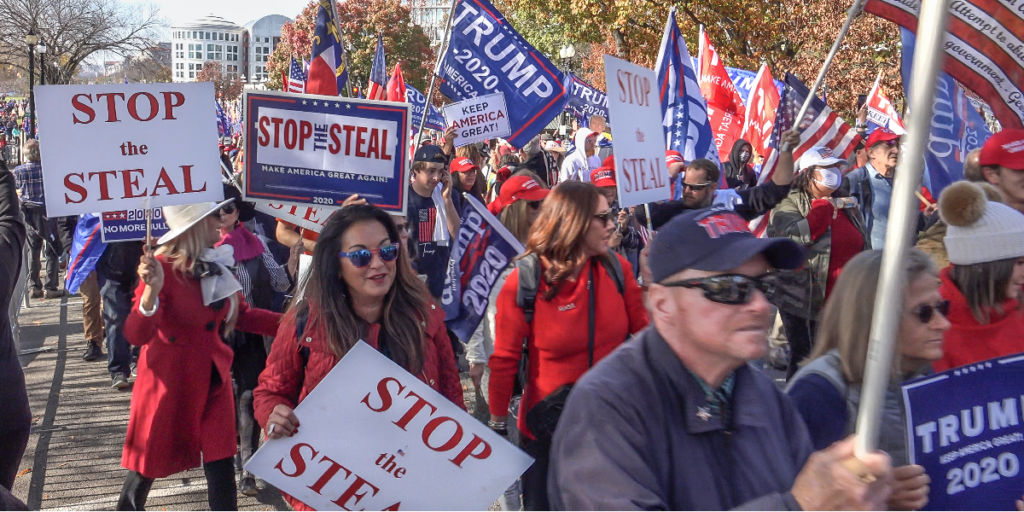
Bob Korn/Shutterstock
In a new study based on a dataset drawn from Twitter, researchers at Cornell Tech in New York and the Technion in Israel explored how images associated with the Stop the Steal campaign– which sought to sow doubt about the outcome of the 2020 election and helped motivate a violent insurrection at the U.S. Capitol on January 6, 2021– spread on the platform.
The persuasive power of images and memes is a relatively well studied phenomenon; but the ways in which such artifacts propagate on social media in the context of mis- and disinformation campaigns is less well understood. The researchers set out to address “a gap in understanding the type of images used, their characteristics, the role they played in the campaign, and the overall dynamics of their spread” on Twitter.
The VoterFraud2020 dataset that underlies the study gathered 167,696 media artifacts, including images and video. The researchers were able to extract the most popular images shared by accounts advancing mostly false voter fraud claims, focusing on those “that were shared at least 1,000 times each within the dataset, resulting in a set of 40 images.” (5 of the 40, for instance, were retweets shared by Ali Alexander, the far right activist credited with leading the Stop the Steal campaign.)
Some of these images were retweeted thousands of times, per the available data in the set, which was time bound and did not represent a full measure of activity across the entire platform. Half of the top images were photographic images; 40% were of text rendered as images, and the remainder included graphics and memes. Of the photographs, almost half depicted images of voting mechanics, such as ballots, ballot envelopes, or the transport of ballots. Per a qualitative review of their content, the researchers find that the top images served a variety of roles in advancing the Stop the Steal campaign:
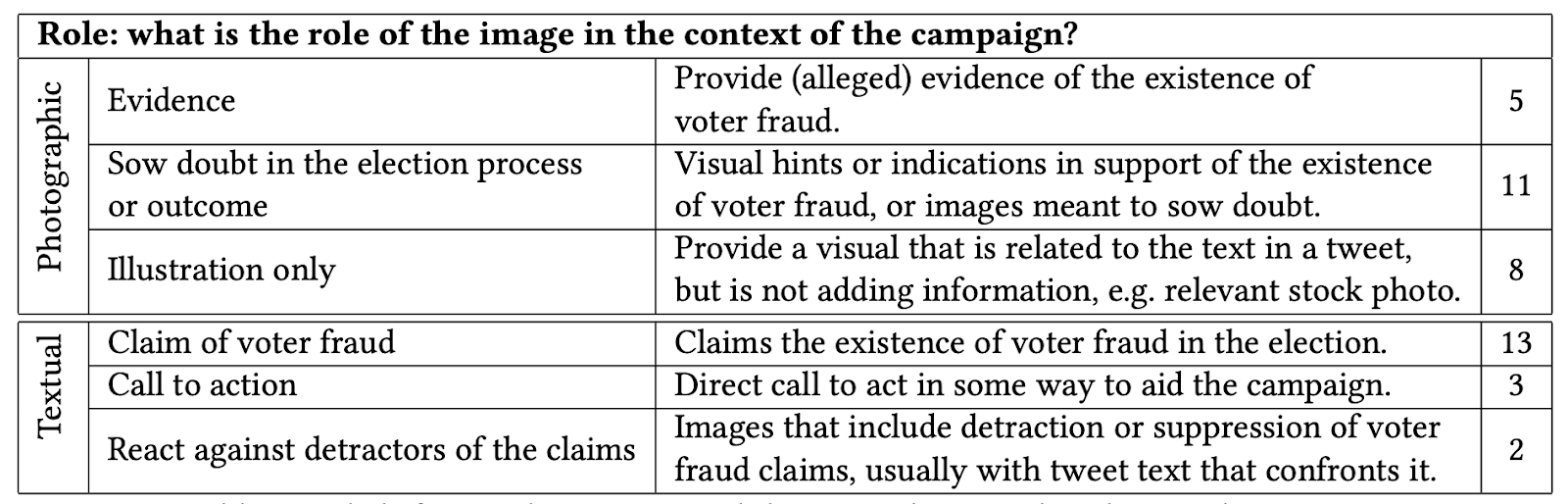
Perhaps the most compelling part of the analysis focuses on the temporal dimensions of the spread of Stop the Steal images. While the top three images enjoyed more than one period of spread, most were propagated in a single episode. The study considered the “half-life of the spread for each image, i.e., the time until each image reached half of its total shares in the dataset.” Almost half of the images reached their half-life within three hours; after seven hours, 75% had reached that threshold. This type of speed presents a challenge to content moderators and fact checkers; most response times are much longer.
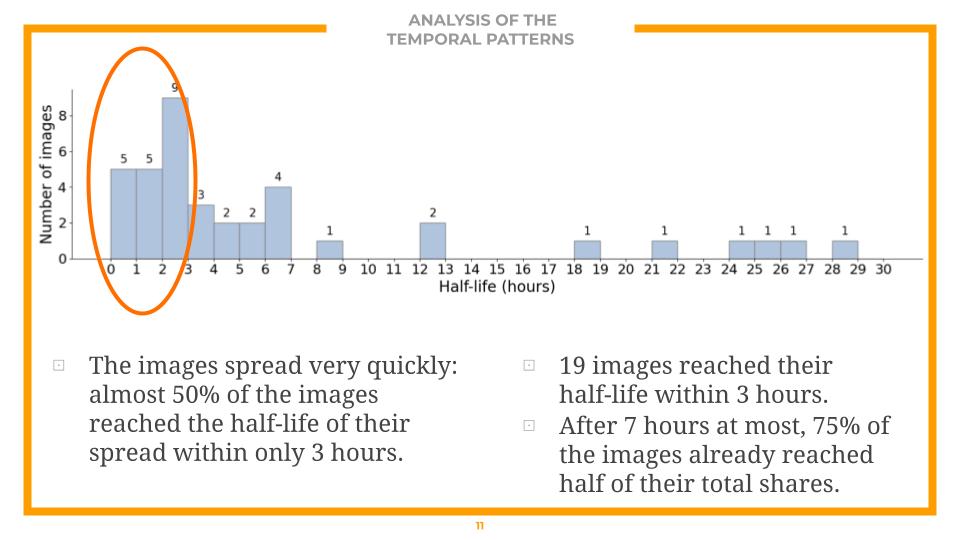
One analysis in the paper considered popular accounts with more than 100,000 followers, finding whom among them participated "early and often" in sharing the Stop the Steal’ images -- the chart below has the top ten, which includes the right wing media entity OANN.
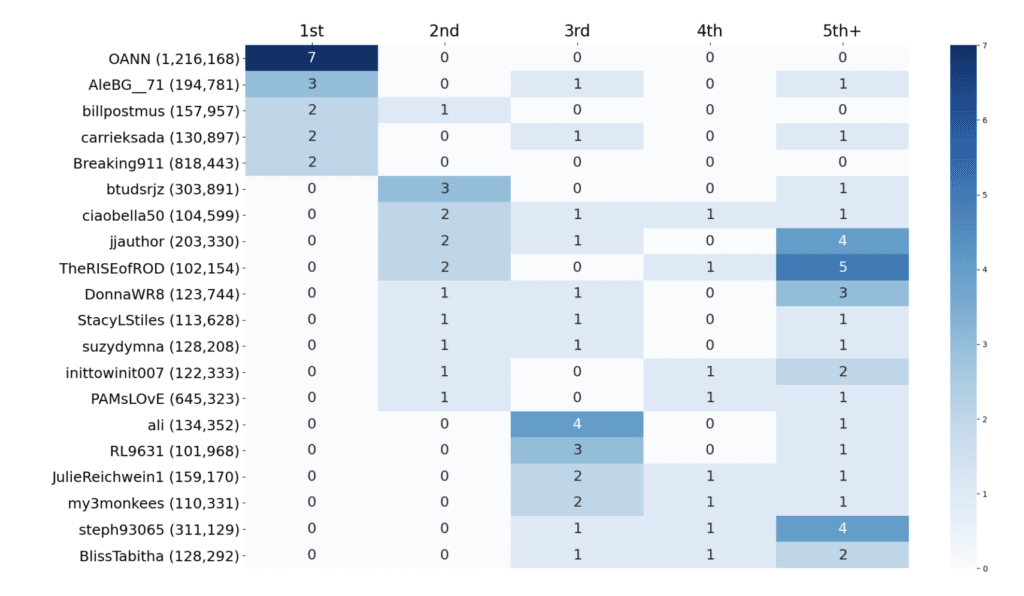
The researchers suggest their results may provide a useful “compass” to other researchers and technologists working on methods to mitigate misinformation. They write, for instance, that “given that many of the popular images were text or quotes rendered as an image, a reasonable direction for mitigating misinformation may be using text recognition and extraction techniques to make these textual images searchable and allow detection of misinformation in tweets even when there are no suspicious keywords in the post itself.”
But the results also show the limits of content moderation as it is presently executed, as “many of the popular images used in this campaign, and especially the photographic images, may not by themselves have violated content policies such as those that require identification of content manipulation.” And the speed of the spread of such images– while a natural phenomenon on a platform like Twitter- is a key challenge for any human review.
Unfortunately, the researchers point out the campaign is not over. Indeed, the Stop the Steal campaign was “still playing out at the time of publication, and will continue to impact the state of democracy in the U.S. and beyond for a long time to come.”
The authors of the study include the Technion's Hana Matatov and Ofra Amir and Cornell Tech's Mor Naaman, with whom I occasionally teach.
Authors
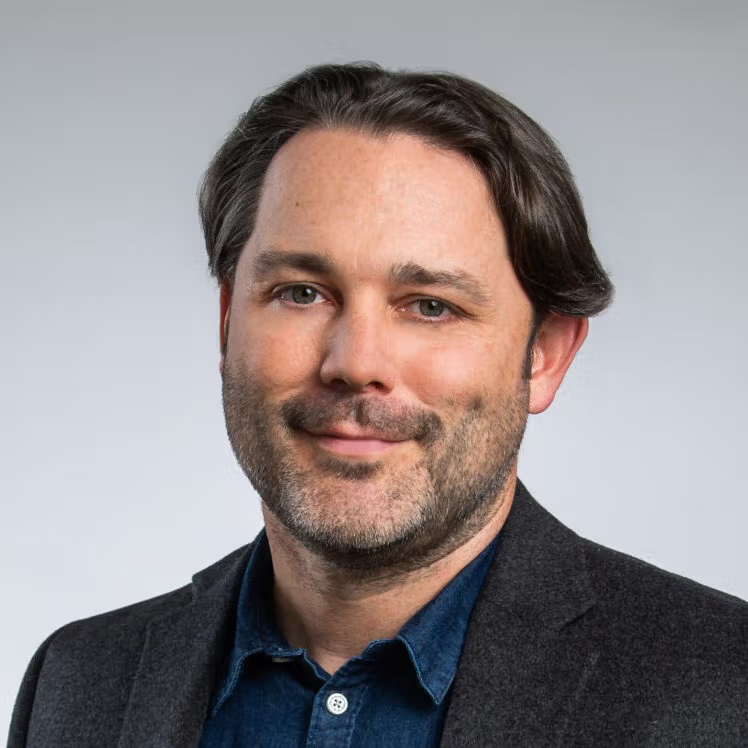