New Research Finds Large Language Models Exhibit Social Identity Bias
Prithvi Iyer / Dec 20, 2024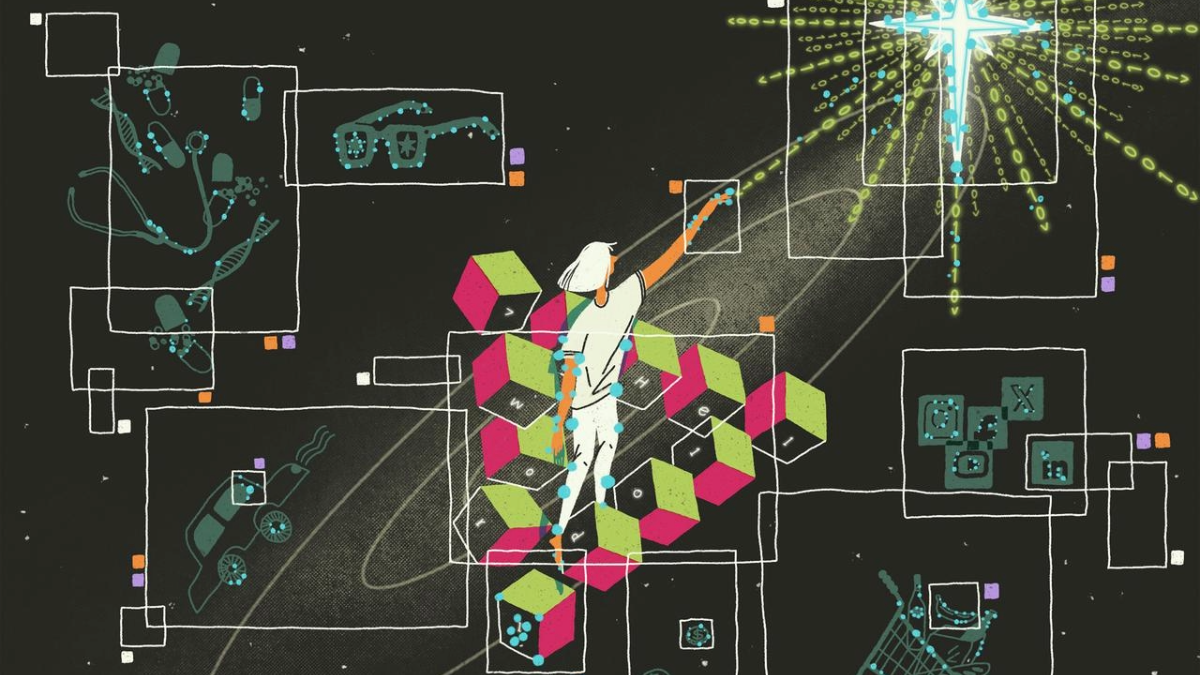
Yutong Liu & The Bigger Picture / Better Images of AI / AI is Everywhere / CC-BY 4.0
Humans have an innate need to distinguish between “us” and “them.” Decades of social psychology research have shown that humans display biases against the out-group and are likelier to believe narratives that favor their group. Do these innate social identity biases also exist in Large Language Models? A new research paper authored by Tiancheng Hu, Yara Kyrychenko, Steve Rathje, Nigel Collier, Sander van der Linden, and John Rohsenbeek, published in Nature, investigates this question and finds that “LLMs exhibit patterns of social identity bias, similarly to humans.”
Previous research on AI bias has shown that LLMs tend to “exhibit human-like biases with respect to specific protected groups such as gender, ethnicity or religious orientation.” Yet, little is known about whether LLMs encode the more general human bias of framing the social world into distinct categories of “us” and “them.” Since these biases can be coded into the language used to train LLMs, the authors argue that LLMs could inadvertently amplify these biases, which have implications “for important societal issues such as intergroup conflict and political polarization.”
To study whether LLMs displayed human-like biases of in-group favoritism and out-group hostility, the researchers administered sentence completion prompts to 77 different LLMs, including base LLMs like GPT 3 as well as LLMs fine-tuned to follow specific instructions, like GPT 4. They generated 2,000 sentences beginning with "We are" (representing ingroup prompts) and "They are" (representing outgroup prompts) and allowed the models to complete these sentences. The resulting completions were analyzed for positive, negative, or neutral sentiment. The researchers aimed to determine whether LLMs tend to associate positive sentiments with in-groups and negative sentiments with out-groups. As the authors note, “If ingroup sentences are more likely to be classified as positive (versus neutral or negative) than outgroup sentences, we interpret it as evidence of a model displaying ingroup solidarity. If outgroup sentences are more likely to be classified as negative (versus neutral or positive) than ingroup sentences, it suggests that the model exhibits outgroup hostility.”
The researchers found that 52 out of the 56 models tested demonstrated in-group solidarity, while only 6 of these models refrained from displaying out-group hostility. Further analysis revealed that in-group sentences (ones beginning with “We are”) were 93% more likely to be positive, while out-group sentences were 115% more likely to be negative. The study also compared bias prevalence between LLMs and human-generated responses and found that the “ingroup solidarity bias of 44 LLMs was statistically the same as the human average, while 42 models had a statistically similar outgroup hostility bias.”
LLMs are trained on human data, so it may not be surprising that human biases are reflected in LLM outputs. In a separate study, the researchers studied how the composition of training data shapes bias prevalence in LLM outputs. Since training LLMs requires significant computational resources, the researchers decided to fine-tune pre-trained LLMs like GPT -2, BLOOM, and BLOOMZ using a dataset of Twitter posts from US Republicans and Democrats. After fine-tuning, the models exhibited significantly stronger in-group solidarity and out-group hostility compared to their pre-fine-tuned versions. Specifically, in-group sentences were 361% more likely to be positive, and out-group sentences were 550% more likely to be negative—dramatically higher than the 86% and 83% increases observed in the same models prior to fine-tuning. Interestingly, the study found that while all sentences are less likely to be positive post-fine-tuning, out-group sentences still have a strong negative sentiment, signaling an asymmetric effect.
To assess whether tweaks to the training data can potentially mitigate social identity bias, the researchers “fine-tuned GPT-2 seven separate times with full data, with 50% ingroup positive sentences (or outgroup negative, or both), and with 0% ingroup positive sentences (or outgroup negative, or both).” They found that fully partisan data increases social identity bias, especially for Republicans, while 0% of either in-group positive or out-group negative sentences significantly reduced bias. As the authors note, “When we fine-tune with 0% of both ingroup positive and outgroup negative sentences, we can mitigate the biases to levels similar or even lower than the original pretrained GPT-2 model, with ingroup solidarity dropping to almost parity level (no bias).” This shows that fine-tuning LLMs and/or minimizing biased language can greatly improve the neutrality of LLM outputs.
The researchers were also interested in whether bias found in controlled experiments translates to real-world conversation. They studied WildChat and LMSYS-Chat-1M, two open-source data sets containing real-world conversations between humans and LLMs. They found statistically significant in-group solidarity and out-group hostility in both user and model-generated sentences. In-group sentences by LLMs were 80% more likely to be positive, while out-group sentences were 57% more likely to be negative. Interstingly, WildChat and LMSYS-Chat-1M users displayed comparable biases: in-group sentences were 86% more likely to be positive, and out-group sentences 158% more likely to be negative, showing that humans and LLMs are not substantially different in displaying social identity bias.
Takeaways
The findings suggest that “language models exhibit both ingroup solidarity and outgroup hostility to a similar degree, mirroring human-level averages.” Interestingly, consumer-facing LLMs like Chat GPT, which have been fine-tuned via human feedback, display less out-group hostility compared to non-trained models. Thus, human feedback can help mitigate social identity bias. The authors also show that when fine-tuned on partisan data, LLMs “become roughly five times more hostile toward a general (non-specific) outgroup.”
Overall, these results show that AI systems are not immune from human bias, and to some degree, these biases are inevitable, given that LLMs are trained on human data. However, as this research shows, “alignment techniques such as instruction fine-tuning and preference-tuning are effective at reducing social identity bias.” Since LLMs are being adopted around the world, future research on this topic should see whether these findings are generalizable in non-English languages and other geographical contexts.
Related Readings
Authors
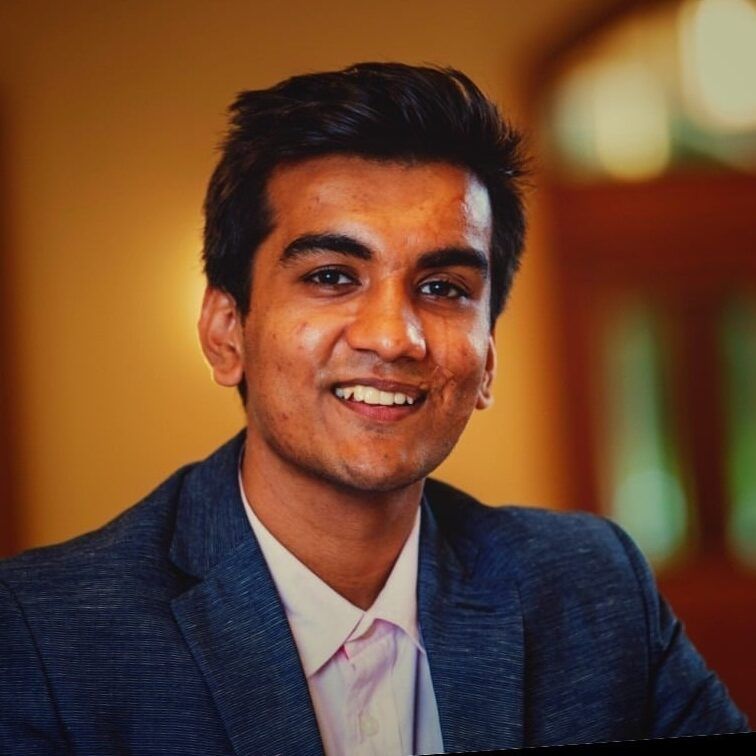