Local AI Research Groups are Preserving Non-English Languages in the Digital Age
Evani Radiya-Dixit / Oct 21, 2024Research groups such as Masakhane for African languages, AI4Bharat for Indian languages, and AmericasNLP for native American languages are developing AI that serves their own communities, countering the dominance of English-centric AI, writes Evani Radiya-Dixit.
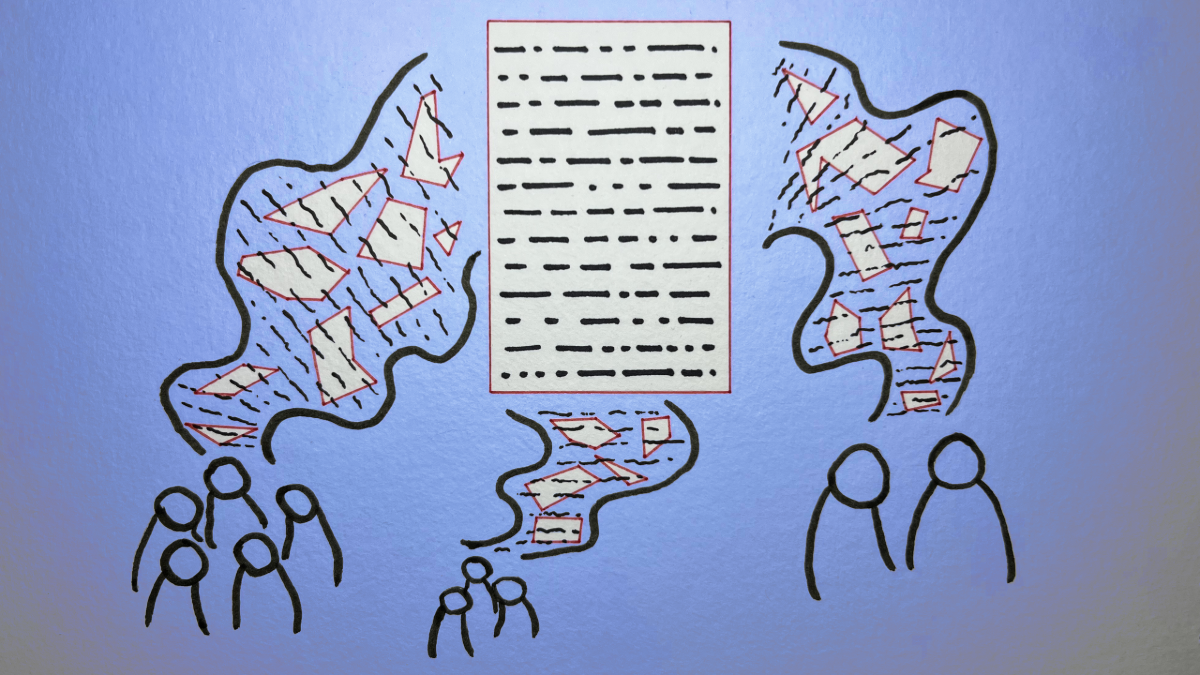
Yasmine Boudiaf & LOTI / Better Images of AI / Data Processing / CC-BY 4.0
The English language dominates the development of artificial intelligence (AI). Many leading language models are trained on nearly a thousand times more English text than other languages. These disparities in AI have real-world impacts, especially for racialized and marginalized communities. English-centric AI has resulted in inaccurate medical advice in Hindi, led to wrongful arrest because of mistranslations in Arabic, and has been accused of fueling violence in Ethiopia due to poor moderation of hate speech in Amharic and Tigrinya.
Language technology developed by prominent tech companies often falls short in addressing the needs of non-English speakers. Throughout history, non-English languages––especially regional and indigenous ones––have faced erasure by colonial powers, resulting in English becoming the default global language. Many multilingual AI efforts by Meta and Google reinforce this dominance of English, as they often rely heavily on machine-translated data, overlook cultural context, and have limited engagement with local language communities.
Yet, a growing number of language-specific AI research groups across the globe, such as Masakhane and AmericasNLP, have emerged to counter English-centric AI. Often housed in academic or grassroots settings, these groups are empowering their communities to not only build AI tools in their own languages but also benefit directly from them. Their contributions are crucial to preserving diverse languages and cultures in the digital age.
A key component of these groups is their focus on community participation, as I discuss in a new brief. Rather than imposing top-down solutions, these groups engage with native speakers, language experts, and local communities to create datasets, train AI models, and develop practical applications. This model of participation helps ensure that the resulting AI tools reflect the linguistic and cultural diversity of the communities they serve, pointing to best practices for multilingual AI development.
First, diverse communities should be involved to tailor datasets to local cultures.
Many AI research groups meaningfully engage with native speakers and language experts to create language datasets that are culturally relevant. For example, the AI4Bharat group created its IndicVoices speech dataset for 22 Indian languages by involving diverse community members—across ages, genders, and professions—to capture different language practices such as slang and local idioms. Some data was even collected on an 8 KHz telephone channel to ensure the representation of low-income users in India who may not have smartphones. AI4Bharat also tailored the data collection process by designing region-specific roleplay scenarios, such as conversations about Kashmiri handcrafted items or discussions about the types of rice native to Palakkad.
AI4Bharat’s efforts to create technology for Indian languages are particularly impactful, as its tools are used by the National Programme on Technology Enhanced Learning to subtitle higher education videos, and by the Supreme Courts of India and Bangladesh to translate judicial documents. AI4Bharat’s collaborative process not only strengthens the quality of AI models but also benefits communities through the development of these applications.
Communities should directly benefit from the AI tools built in their languages.
Some efforts to develop language technology can lead to tokenism or exploitation, where communities are consulted for data collection but do not experience the benefits of the tools built using their labor. Many AI research groups are countering this dynamic by ensuring that the communities involved in dataset creation also benefit from the tools built using those datasets. Several of these groups establish collaborative initiatives, where community members work together to build datasets and models that tackle specific problems facing the community.
For example, the AmericasNLP group leads an initiative to help revitalize indigenous languages, the idea for which emerged from researchers working with Mayan communities in Mexico. This initiative focuses on developing AI tools that automatically create educational materials for teaching native American languages, addressing the critical need to support the learning of endangered indigenous languages. The SIGARAB group, which focuses on Arabic and its dialects, organizes Arabic-specific initiatives oriented towards community needs like propaganda detection in Arabic to combat its spread on media and annotation of news articles about Gaza to uncover media bias.
Finally, data ownership and inclusive authorship should be prioritized to enable more ethical community participation.
Many language technology initiatives follow Western transactional approaches to data sharing and restrictive authorship models that only reward certain kinds of participation like data analysis and paper writing. A survey of researchers working on mid- and low-resource languages, for example, found that only 33% consistently received credit for their contributions to dataset or model creation.
In contrast, several AI research groups prioritize data ownership and use inclusive authorship models. These efforts help shift power to communities in exercising their agency and shaping AI development. This extends to data refusal, where communities say “no” to how their data is collected or used, such as the Māori community maintaining ownership over their language data to prevent Big Tech from using their datasets in ways that do not primarily benefit them.
The IndoNLP group considered data ownership in its language AI crowdsourcing effort, NusaCrowd, where they collected datasets for local Indonesian languages such as Javanese and Sundanese. Importantly, IndoNLP did not copy or store the crowdsourced datasets, but rather maintained the control and ownership with the original contributors.
Masakhane, a grassroots community focused on African languages, also exemplifies an inclusive approach to participation with its non-traditional authorship model that recognizes not only contributions to analysis and writing, but also those in the form of data and lived experiences. Masakhane’s community-driven approach is especially impactful through its partnership with Lelapa AI, a leading company in multilingual AI on the African continent, which provides transcription and content analysis products in Afrikaans, isiZulu and Sesotho for people and businesses.
So what is needed to continue preserving non-English languages in the digital age?
As the field of AI continues to evolve, the efforts of these research groups provide a blueprint for how to develop more inclusive AI systems. These groups are not only preserving non-English languages in the digital age, but also reimagining how AI can be developed with a more community-driven approach.
These groups are playing a crucial step towards addressing language disparities in AI development. However, more support and funding is needed from governments and international organizations to ensure that these efforts continue. Policymakers must prioritize the inclusion of non-English languages in technology, and companies should work with these groups to create AI systems that are better attuned to diverse cultures and languages.
By learning from these research groups, we can shift from creating English-centric AI to empowering non-English speaking communities to benefit from the technologies built in their own languages.
Authors
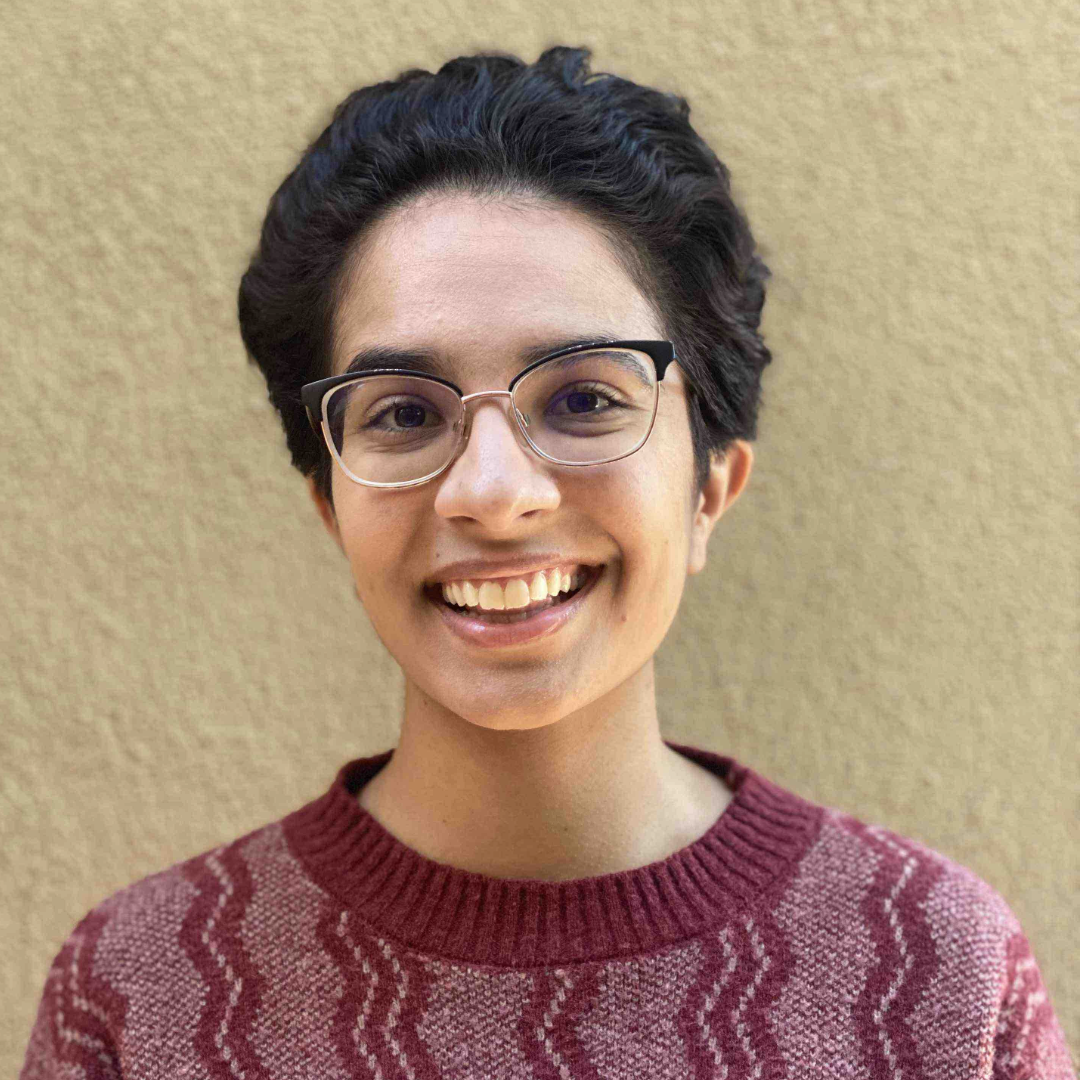