Leveling Up to Responsible AI Through Simulations
Steven A. Kelts, Chinmayi Sharma / Sep 4, 2024Simulations are a valuable tool to tease out the complexities of responsible AI development, write Steven A. Kelts and Chinmayi Sharma.
Most people want AI to be built and used responsibly. We even have a sense (however vague) of what it means to build AI responsibly. But, AI is built in complex corporate and regulatory landscapes. How does that affect the end product? How do we ensure that all stakeholder groups—both within a company and in the broader regulatory and societal context—develop a deep understanding of the complex environments in which responsible AI frameworks must be implemented? And how can we teach professionals, from engineers and board members to policymakers and civil society, to collaborate effectively within this intricate landscape to ensure that AI systems are developed and deployed responsibly? There is no panacea, but we think simulations are part of the answer.
Serious games
Simulations offer crucial insight for both engineers and non-engineers in a low-stakes (and fun) environment. They give non-engineers in the corporate sector, from chief financial officers and marketing executives to outside counsel, insight into the real workings of an AI production team and regulatory priorities and they help engineers practice the skills to navigate complex business processes. They give regulators and policymakers the technical literacy and awareness of corporate dynamics that can help ensure that as they develop frameworks for responsible AI (like the National Institute of Science and Technology Risk Management Framework) their eyes are wide open to the many ways that ill-conceived structures create unwanted outcomes. And they give engineers (and engineering students) insight into the team dynamics that lead corporate decision-making to go awry, and the habits they can cultivate to take individual responsibility even in complicated corporate structures.
Earlier this year, we put our theory into practice. One of us has been designing custom simulations to educate engineering students about the complexity of ethical and responsible AI in practice for years. The other has written about the integral role professionals in tech companies play in implementing responsible AI. So, in April, we held the first of its kind interactive simulation where we invited seasoned professionals from diverse fields—industry executives, engineers, policy advocates, academics, tech journalists, and government officials—to experience firsthand the challenges of embedding responsible AI in an Agile development process. Here we share our observations.
The Challenge
We know our ideal outcome—responsibly built AI—but we don’t know how to achieve it. The pillars of responsible AI frameworks are harder to implement than they were to articulate.
After the release of the White House Executive Order on the Safe, Secure, and Trustworthy Development and Use of Artificial Intelligence late last year, we wrote in these pages about the inherent incompatibilities between responsible AI frameworks and AI development. Today, most companies build AI within fast-paced, Agile development lifecycles, which are specifically designed to maximize productivity in breakneck “sprints.” We argued that the methods usually suggested for ensuring responsible AI don’t fit with the speed and low-information decision-making inherent to Agile:
- Impact assessments, for instance, are hard to carry out when a customer’s requested features are constantly evolving.
- Pre-training data checks require delays and caution releasing an MVP, both of which are anathema to Agile.
- Post-hoc audits require careful documentation, which Agile shuns.
- Red-teaming identifies “flaws” and encourages quick “patches,” not fundamental system re-designs.
In that piece, we also argued that decades of research on cognitive biases in corporate settings allow us to see how irresponsible decision-making unfolds, and should give us pause. Agile moves so fast that developers tend to compartmentalize information on a need-to-know basis rather than sharing it, leaving everyone unable to make global judgments about a system.
It’s an uphill battle, but companies will need to learn to implement responsible innovation frameworks in Agile, government officials will need to learn how to regulate Agile, and civil society will need to learn how to evaluate outcomes against this backdrop as well.
Our Simulation
So exactly how hard is it to operate responsibly in an Agile environment shipping an AI product? That’s what we wanted the participants in our simulation to experience for themselves. On April 26, 2024 at Fordham Law School, we convened a group of scholars, reporters, researchers and programmers to run them through a simulation adapted from the “Agile Ethics” program.
Responsible innovation frameworks (like this often-cited one by Stilgoe, Owen and Mcnaghten) often implores developers to “Anticipate” future wrongs, “Include” the voices of all team members and outside affected parties, and “Reflect” by taking a critical stance towards their own assumptions and values. Accordingly, Agile Ethics, a program developed by Steven Kelts at Princeton, runs students through a fast-paced simulation of the development process for a real product (gone wrong), asking them to make the same decisions that the company building the product needed to make with the same limited information.
Our simulation for professionals focused on a hypothetical, but realistic, AI product (quite similar in concept to one that a major tech company announced a mere few weeks later). The participants played roles as engineers, business analysts, project managers, UX designers and user researchers, in-house lawyers, CEOs and other corporate roles, working for a cell phone company attempting to implement a small-language AI assistant on their popular handheld devices. Over the course of successive “sprints,” the participants encountered things like privacy concerns as they chose whether to move model training data off-device, how to handle womens’ health data as users began employing their assistants for menstrual tracking, whether to allow the assistant to do web search for things like medical information, how to limit the AI’s agentic capacities when interacting with users’ apps, and how to re-train their model after a major country passed a “right to be forgotten” law. (A simplified description of each sprint can be found here. A replica of the entire game board can be found here.)
Crucial to the setup was that the participants had to play a role they were unfamiliar with, not rely on their real-life expertise. This made some uncomfortable, and drove many to break character at times. But it was intended to simulate the difficulty on development teams of surfacing the sort of information required to make decisions – to give just one example, often developers don’t know what legal needs to know from them, and legal doesn’t know how to ask the questions that will raise compliance-relevant information. We wanted our participants to have to question each other and dig deep to surface relevant knowledge (so a lawyer, for instance, had to ask leading questions and experience the game from an engineers’ perspective, rather than just blurt out what they took to be the “answer”).
Lessons Learned
Our participants soon discovered how very difficult it is to implement responsible AI frameworks in realistic corporate environments. They struggled to anticipate how new systems will affect users, include many voices in the deliberative process, or reflect on them when development sprints moved quickly, decisions were made without them, and daily reports on technical progress are required.
Our participants reported learning many lessons from the simulation that will impact the way they think, teach, regulate and develop in the age of AI. We enumerate the core takeaways below.
1. Expertise as a Barrier to Accomplishing the Principles for Responsible AI
Professional expertise, while invaluable, can also become a barrier to implementing the principles of responsible AI. Participants found that when they were asked to make decisions outside their usual areas of expertise, they often struggled to engage fully. This struggle highlighted a critical challenge in cross-disciplinary collaboration: professionals are often deeply rooted in their specific fields, making it difficult for them to step into another domain with confidence. However, this discomfort also simulated the real-world uncertainty that professionals face when making decisions with incomplete information. For instance, an engineer might need to consider legal implications, or a marketing professional might need to think about data privacy—areas where they lack deep expertise. This experience underscored the importance of fostering environments where cross-disciplinary dialogue is not just encouraged but essential, as it forces team members to view problems from multiple perspectives and understand how different aspects of a project are interconnected.
2. The Impact of Authority on Decision-Making Dynamics
Power dynamics can thwart the goals of responsible AI. When someone with perceived authority, such as a lawyer, stated a legal position (sometimes breaking the “role” they were assigned to play), the conversation often stalled. The assertion of expertise, particularly in legal or regulatory matters, frequently acted as a "trump card," effectively shutting down further discussion. This dynamic is problematic because it can lead to an over-reliance on specific individuals and their expertise, stifling broader group engagement and potentially overlooking important considerations. For example, if legal or technical experts dominate the conversation, important ethical or social perspectives might be ignored, leading to decisions that are legally sound but ethically questionable. This behavior risks creating blind spots, as the nuanced perspectives of other team members may not be fully explored or integrated into the final decision. The expert might in fact have reached a different judgment if they had heard more about what the others on the team (all experts in their own domains) know about the product and process.
3. Diffusion of Responsibility and Its Consequences
Deference to expertise can lead to more than just failures of information-sharing; it can lead team members to deflect responsibility onto others. Thus, paradoxically, expansive teams of cross-disciplinary experts are essential but can also be self-defeating—the catch-22 of responsible AI. When one person is seen as the go-to expert for a particular issue, other team members may feel less compelled to think critically or contribute their insights. Our participants reported that even in a simple “game,” they found themselves deferring to the authority figure and disengaging from the decision-making process, believing that the expert has already covered all the necessary bases. This can lead to a passive approach where the responsibility for complex decisions becomes overly concentrated, and others fail to even seek information about potential ethical problems. Participants sometimes regretted their failure to speak up once they discovered in later sprints that unforeseen problems had emerged. Encouraging all team members to actively engage, regardless of their expertise, is crucial in fostering a comprehensive approach to AI development.
4. Incentives and Their Influence on Team Dynamics
Role-specific performance metrics can undermine the broader goals of responsible AI. Participants noticed that their decisions were often influenced by the incentives tied to their assigned roles. For instance, a business analyst focused on profitability might push for decisions that maximize short-term gains, while an engineer might prioritize technical excellence over broader social implications if that is the basis of their performance reviews. This divergence in priorities can create friction within teams, making it difficult to align on a unified approach to responsible AI. Interestingly, our participants saw this change in their behavior even when they were simply assigned a title for the “game play” – role schemas (a person’s understanding of what someone with their role is “supposed” to do) and incentives seemed to go hand in hand. And this led to situations where the contributions of certain team members were underutilized or ignored, particularly when their contributions did not align with the dominant incentives driving the team. This dynamic underscores the need for organizations to carefully consider how roles and incentives are structured, ensuring they promote a balanced consideration of ethical, legal, technical, and business factors.
5. The Challenge of Disillusionment Among Team Members
A particularly poignant lesson from the simulation was the disillusionment experienced by participants when their thoughtful contributions were overlooked or dismissed. This sense of disillusionment is not uncommon in real-world corporate environments, where well-intentioned individuals may feel that their ethical concerns are secondary to business imperatives. In the simulation, this was particularly evident when participants made recommendations that were ultimately ignored in favor of more expedient or less controversial choices. Such experiences can lead to disengagement, where team members become less likely to voice concerns or push for responsible practices in the future. Addressing this issue requires creating a culture where all contributions are valued, and ethical considerations are given due weight in the decision-making process.
6. The Difficulty of Capturing Comprehensive Documentation
Finally, the simulation underscored the significant challenges associated with documenting the decision-making process in a way that captures the full scope of discussions and considerations. Participants found it difficult to keep track of the various threads of conversation, particularly when decisions were made rapidly and under pressure. This difficulty in documentation can be a major barrier to responsible AI development, as it hinders transparency and accountability. Without thorough records of how and why decisions were made, it becomes challenging to conduct meaningful post-hoc audits or to learn from past experiences. Effective documentation practices are essential for ensuring that AI systems are developed responsibly, as they provide a clear trail of the ethical, legal, and technical considerations that shaped the final product.
Room for Improvement: Enhancing the Realism and Impact of Simulations
Our simulation was not without its shortcomings. We identified opportunities to make improvements to better accomplish its goals.
1. Simulating Realistic Communication and Collaboration
One area where our simulation could be improved is in reflecting the reality that stakeholders are rarely in the same room having the same conversations. In real-world corporate environments, communication is often fragmented, with different departments working in silos. This segmentation can lead to misunderstandings and misaligned priorities, as each group may not have full visibility into the broader context. To better simulate this reality, future exercises could incorporate scenarios where participants must navigate the challenges of remote communication, asynchronous decision-making, and siloed information. This would provide a more accurate reflection of the complexities involved in real-world AI development, where seamless communication and collaboration are far from guaranteed. But given that decision-making was already so difficult in this “game” setting, this only highlights the problem for more complicated corporate settings.
2. Improving the Realism of Incident Reporting
Another area for improvement is the simulation of incident reporting. In our exercise, issues were identified and addressed almost instantaneously, providing a clear and direct line of cause and effect from decision to ethical/social impact. However, in actual corporate environments, incident reporting is often much slower and more fragmented, making it even harder to discern harms and their remedies. Information may take time to filter through the organization, and the response to incidents can be delayed by bureaucratic processes or competing priorities. Future simulations could better reflect this reality by introducing delays in incident reporting and requiring participants to manage the consequences of these delays. This would help participants understand the importance of timely communication and the potential risks associated with slow or incomplete incident reporting.
3. Enhancing the Complexity of Decision-Making Scenarios
While the simulation provided a valuable learning experience, there is room to increase the complexity of the decision-making scenarios. In particular, introducing more nuanced trade-offs and ethical dilemmas could help participants better appreciate the multifaceted nature of responsible AI development. For example, scenarios could include conflicting legal requirements across different jurisdictions, or ethical dilemmas where the "right" decision is not immediately clear. By adding these layers of complexity, simulations can more effectively prepare participants for the real-world challenges they will face in developing and deploying AI systems.
4. Encouraging Deeper Reflection and Discussion
One of the limitations of our simulation was that discussions sometimes ended prematurely when someone asserted their expertise. In future iterations, it would be beneficial to create structures that encourage deeper reflection and ongoing dialogue, even after an "expert" has spoken. This could involve setting up a process where different perspectives are explicitly sought out and where the group is encouraged to challenge assumptions and explore alternative viewpoints. One participant, for instance, suggested that participants be given one “silencer” card and one “keep debating” card to play during the game, to temporarily quiet the loudest voices or reconsider a decision taken. By fostering a culture of continuous reflection, simulations can help participants develop the critical thinking skills needed to navigate the ethical complexities of AI development.
Conclusion: Moving Forward with Responsible AI
This simulation highlighted the challenges and opportunities involved in embedding responsible AI practices within Agile development environments. The lessons learned from this exercise are clear: expertise, while essential, must be balanced with cross-disciplinary collaboration; incentives need to be aligned with ethical outcomes; and effective communication and documentation are crucial for ensuring accountability.
Moving forward, organizations must prioritize the development of frameworks and cultures that support responsible AI. This includes creating opportunities for ongoing education and reflection, fostering environments where diverse perspectives are valued, and ensuring that all stakeholders—from engineers to policymakers—are equipped and incentivized to navigate the complexities of responsible Agile AI development.
Simulations like the one we conducted are a valuable tool in this effort. By providing a realistic, immersive experience, they help professionals from diverse backgrounds understand the challenges of responsible AI development and prepare them to meet these challenges in their own work. As AI continues to evolve and become increasingly integrated into our lives, the need for responsible development practices will only grow. It is up to us, as a community, to ensure that these practices are embedded in the fabric of AI development, guiding the technology toward outcomes that benefit society as a whole.
Authors
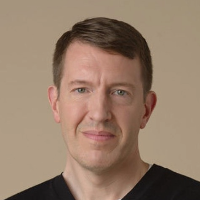
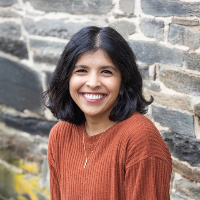