Is Generative AI the Answer for the Failures of Content Moderation?
Paul M. Barrett, Justin Hendrix / Apr 3, 2024Co-published with Just Security.
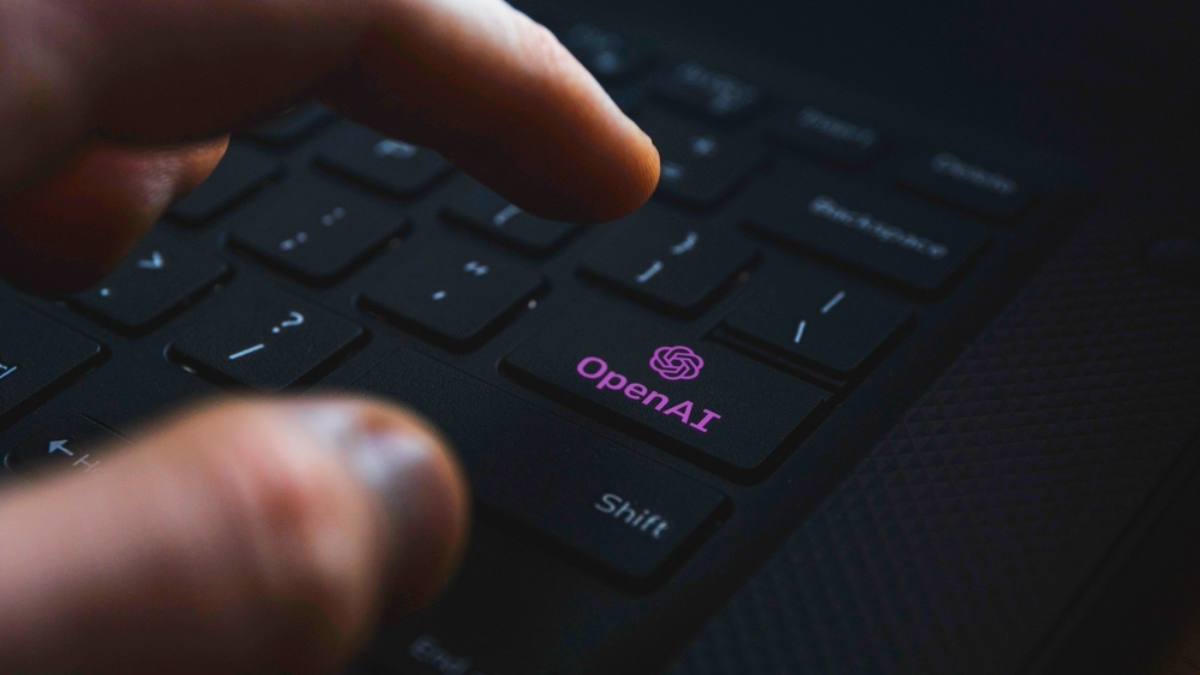
Illustration of a button on a keyboard that reads "OpenAI." Rokas Tenys/Shutterstock
[Editor’s note: This article is based partly on the event, “Moderating AI and Moderating with AI,” that Harvard University’s Berkman Klein Center for Internet & Society held on March 20, 2024 as part of its Rebooting Social Media Speaker Series].
As head of content policy at Facebook more than a decade ago, Dave Willner helped invent the moderation of content for social media platforms. He has served in senior trust and safety roles at AirBnB, Otter, and, most recently, OpenAI. On March 20, he visited Harvard University to talk about a bold new development in the field of content moderation.
From its inception, content moderation has been fraught with policy and operational challenges. In 2010, Willner and about a dozen Facebook colleagues were following a one-page checklist of forbidden material as they manually deleted posts celebrating Hitler and displaying naked people. But the platform had 100 million users at the time and was growing exponentially. Willner’s tiny team wasn’t keeping up with the rising tide of spam, pornography, and hate speech. So he began drafting “community standards” that eventually would comprise hundreds of pages of guidelines enforced by thousands of moderators, most of whom were outsourced employees of third-party vendors. Over time, Facebook also introduced machine learning classifiers to automatically filter out certain disfavored content. Other major platforms followed suit.
But nearly 15 years later, Willner told his audience at Harvard’s Berkman Klein Center for Internet & Society, it is clear that content moderation just “doesn’t work very well”—a conclusion confirmed by the persistent, bipartisan, society-wide complaints about the high volume of dreck that appears on platforms such as Facebook, Instagram, TikTok, YouTube, and X. Outsourced human moderators are ineffective, Willner said, because they typically are poorly paid, inadequately trained, and traumatized by exposure to the worst that the internet has to offer. The current generation of automated systems, he added, mimic human failure to appreciate nuance, irony, and context.
A New Hall Monitor for the Internet
In his remarks, Willner proposed a potential technological solution: using generative artificial intelligence to identify and remove unwanted material from social media platforms and other kinds of websites. In short, ChatGPT as a hall monitor for the internet.
Willner is not merely spitballing. For the past two-and-a-half years, he has worked full-time or as a contractor on trust and safety policy for ChatGPT’s creator, OpenAI. Fueled by billions of dollars in investment capital from Microsoft, OpenAI is at the center of the commercial and popular furor over technology that can generate uncannily human-like text, audio, and imagery based on simple natural-language prompts.
OpenAI itself has made no secret of its ambition to sell its wares for labor- and cost-saving content moderation. In a corporate blog post last August, the company boasted that GPT-4 can effectively handle “content policy development and content moderation decisions, enabling more consistent labeling, a faster feedback loop for policy refinement, and less involvement from human moderators.” OpenAI suggested that its technology represents a big leap beyond what existing machine learning classifiers can accomplish. The promise is that moderation systems powered by large language models (LLMs) can be more versatile and effective than ones utilizing prior generations of machine learning technology, potentially doing more of the work that has to date fallen to human moderators.
Just a few weeks after OpenAI’s blog post, in September 2023, Amazon Web Services posted its own notice on how to “build a generative AI-based content moderation solution” using its SageMaker JumpStart machine learning hub. Microsoft, meanwhile, says that it is investigating the use of LLMs “to build more robust hate speech detection tools.” A startup called SafetyKit promises potential customers that they will be able to “define what you do and don’t want on your platform and have AI models execute those policies with better-than-human precision and speed." And a relatively new social media platform called Spill says that it employs a content moderation tool powered by an LLM trained with Black, LGBTQ+, and other marginalized people in mind.
For his part, Willner is working with Samidh Chakrabarti, who formerly led Facebook/Meta’s Civic Integrity product team, on how to build smaller, less expensive AI models for content moderation. In a jointly authored article for Tech Policy Press published in January 2024, Willner and Chakrabarti, now nonresident fellows at the Stanford Cyber Policy Center, wrote that “large language models have the potential to revolutionize the economics of content moderation.”
The Promise and Peril of Using AI for Content Moderation
It is not difficult to understand why social media companies might welcome the “revolutionary” impact—read: cost savings—of using generative AI for content moderation. Once fine-tuned for the task, LLMs would be far less expensive to deploy and oversee than armies of human content reviewers. Users’ posts could be fed into an LLM-based system trained on the content policies of the platform in question. The AI-powered system almost instantaneously would determine whether a post passed muster or violated a policy and therefore needed to be blocked. In theory, this new automated approach would apply policies more accurately and consistently than human employees, making content moderation more effective.
In the long run, replacing some or most outsourced human moderators might also benefit the moderators themselves. As journalists and civil society researchers have observed, content moderation is an inherently stressful occupation, one that in many cases leaves workers psychologically damaged. Social media companies have exacerbated this problem by outsourcing moderation to third-party vendors that are incentivized to keep a tight lid on pay and benefits, leading to haphazard supervision, burnout, and high turnover rates.
Social media companies could have addressed this situation by directly employing human moderators, paying them more generously, providing high-quality counseling and healthcare, and offering them potential long-term career paths. But the companies have shirked this responsibility for 15 years and are unlikely to change course. From this perspective, simply eliminating the job category of outsourced content reviewer in favor of LLM-based moderation has a certain appeal.
Unfortunately, it’s not that simple. LLMs achieve their eerie conversational abilities after being trained on vast repositories of content scraped from the internet. But training data contain not only the supposed wisdom of the crowd, but also the falsehoods, biases, and hatred for which the internet is notorious. To cull the unsavory stuff, LLM developers have to build separate AI-powered toxicity detectors, which are integrated into generative AI systems and are supposed to eliminate the most malignant material. The toxicity detectors, of course, have to learn what to filter out, and that requires human beings to label countless examples of deleterious content as worthy of exclusion.
In January 2023, TIME published an expose of how OpenAI used an outsourced-labor firm in Kenya to label tens of thousands of pieces of content, some of which described graphic child abuse, bestiality, murder, suicide, and torture. So, LLMs will not necessarily sanitize content moderation to the degree that their most avid enthusiasts imply. OpenAI did not respond to requests for an interview.[WILL TRY THEM AGAIN]
Then there are questions about just how brilliant LLMs are at picking out noxious content. To its credit, OpenAI included a bar chart with its August 2023 blog post that compared GPT-4’s performance to humans on identifying content from categories such as “sexual/minors,” “hate/threatening,” “self-harm,” and “violence/graphic.” The upshot, according to OpenAI, was that GPT-4 performed similarly to humans with “light training,” but “are still overperformed by experienced, well-trained human moderators.” This concession implies that if social media companies are seeking the best possible content moderation, they ought to acknowledge the full cost of doing business responsibly and cultivate loyal, knowledgeable employees who stick around.
Explanations and Audit Trails
There are some tasks at which existing moderation systems usually fail but conversational LLMs likely would excel. Most social media platforms do a bad job of explaining why they have removed a user’s post—or don’t even bother to offer an explanation. More automated systems should have the capacity to show their homework, meaning they can be queried as to how and why they have reached a certain conclusion. This ability, if activated by platforms, would allow outsiders to audit content moderation activity far more readily.
(As an aside, such a facility for explanation might undercut a legal argument that social media companies are making in opposition to state legislative requirements that they provide individualized explanations of all moderation decisions. The companies’ claim that these requirements are “unduly burdensome,” and therefore unconstitutional, would seem far less credible if LLMs could readily supply such explanations.)
It’s possible that using LLMs would even allow platforms to select from a wider range of responses to problematic content, short of spiking a post altogether. One new option could be for an LLM-based moderation system to prompt a user to reformulate a post before it is made public, so that the item complies with the platform’s content policies.
Currently, when a platform updates its policies, the process can take months as human moderators are retrained to enforce amended or brand-new guidelines. Realigning existing machine learning classifiers is also an arduous process, Willner told his audience at Harvard. In contrast, LLMs that are properly instructed can learn and apply new rules rapidly—a valuable attribute, especially in an emergency situation such as a public health crisis or the aftermath of an election in which the loser refuses to concede defeat.
‘Hallucination’ and Suppression
There are other risks to be weighed, including that LLMs used for moderation may turn out to be factually unreliable or perhaps too reliable. As widely reported, LLMs sometimes make things up, or “hallucinate.” One of the technology’s unnerving features is that even its designers don’t understand exactly why this happens—a problem referred to as an “interpretability” deficit. Whether AI researchers can iron out the hallucination wrinkle will help determine whether LLMs can become a trusted tool for moderation.
On the other hand, LLMs could in a sense become too reliable, providing a mechanism for suppression masquerading as moderation. Imagine what governments prone to shutting down unwanted online voices, such as the ruling Hindu nationalist Bharatiya Janata Party in India, might do with a faster, more efficient method for muffling Muslim dissenters. Censors in China, Russia, and Iran would also presumably have a field day.
The implications for use of generative AI in content moderation cut in conflicting directions. Because of the possibility of cost savings, most social media companies will almost certainly explore the new approach, as will many other companies that host user-generated content and dialogue. But having employed highly flawed methods for content moderation to date, these companies ought to proceed cautiously and with transparency if they hope to avoid stirring even more resentment and suspicion about the troubled practice of filtering what social media users see in their feeds.
RELATED READING
Authors
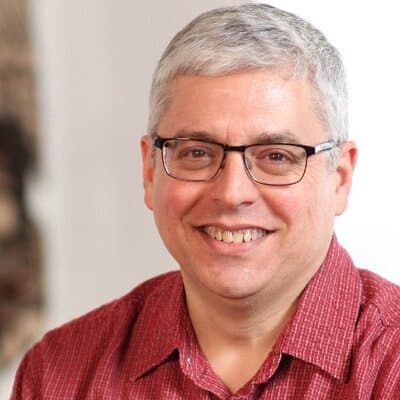
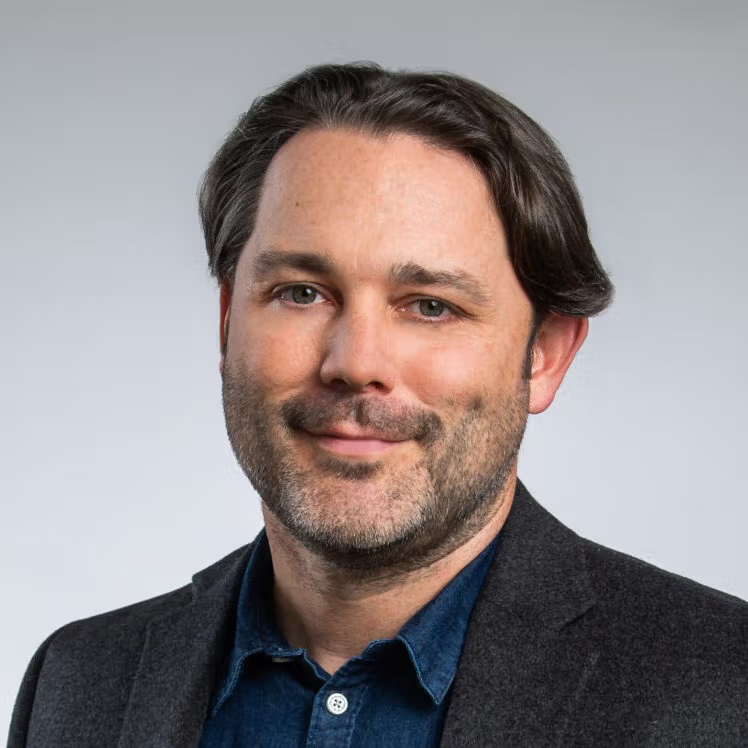