In the Name of Openness
Noah Giansiracusa / May 18, 2022Noah Giansiracusa is an assistant professor of mathematics and data science at Bentley University and author of How Algorithms Create and Prevent Fake News.
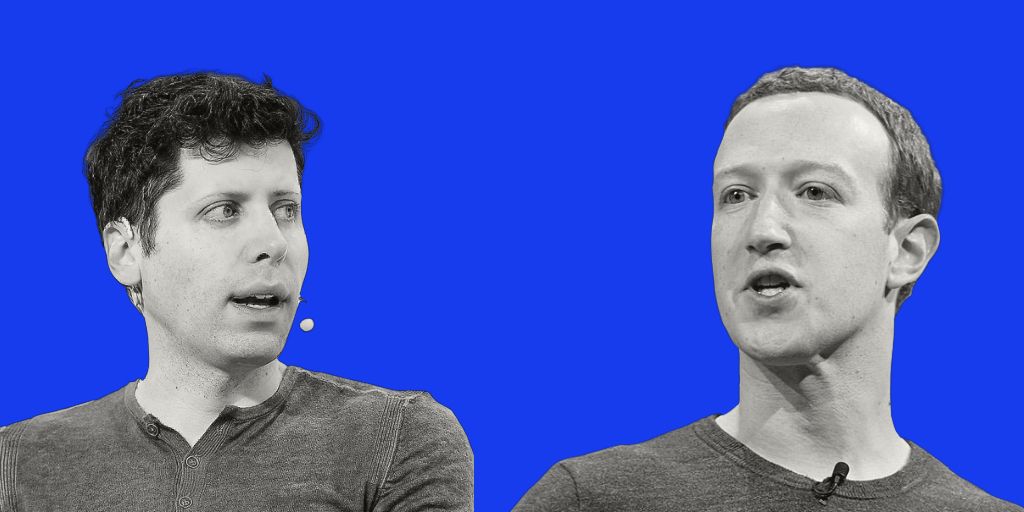
You might expect that an artificial intelligence research company with a name like OpenAI is a more transparent, ‘open’ organization than a more established company like Facebook. If so, you’d be surprised to hear that Facebook recently released to the public an open alternative to OpenAI’s popular and closely guarded closed-access large language model, GPT-3. Facebook calls its model Open Pretrained Transformer (OPT-175B). Did Facebook really just out-open OpenAI, and if so, is that a good thing for the world?
OpenAI was founded as a nonprofit in 2015 with a $1 billion investment from Elon Musk and others and a declaration that AI should be “as broadly and evenly distributed as possible,” and, more concretely, that its researchers “will be strongly encouraged to publish their work, whether as papers, blog posts, or code, and our patents (if any) will be shared with the world.” In 2019, OpenAI shifted to a “hybrid of a for-profit and a nonprofit,” with a corporate entity offering a return to shareholders even as the nonprofit board maintains its control. (Musk left the organization in 2018, though he remained a donor.)
OpenAI should be more open imo
— Elon Musk (@elonmusk) February 17, 2020
In 2020, OpenAI announced a large language model called GPT-3. If you’re not familiar with large language models, you can think of it as a really fancy autocomplete system: you feed it some text, and it generates additional text of any desired length, based on statistical patterns of words it picked up from a huge data set of webpages and digitized books. OpenAI initially limited GPT-3 access to a private group of vetted users—claiming that allowing public access would be too dangerous since the program is so powerful that the company needed to closely monitor its release and early usage to prevent abuses of the technology. Months later, OpenAI signed an exclusive $1 billion deal with Microsoft to grant GPT-3 access to anyone willing to pay.
OpenAI charges users between a tenth of a penny and 7 cents to generate around a thousand words, depending on the flavor of the model the user selects. Crucially here, users pay to use the model–on a website, after logging in to your account that has your billing info, you feed it a text prompt then click a button to have it generate the autocomplete text output—but users don’t have access to the inner-workings of the model itself, meaning the underlying neural network with the numerical value of all its 175 billion parameters. That neural network is what Microsoft was given exclusive access to. In other words, commercial use of GPT-3 is now open to anyone, but unless you happen to work for OpenAI or Microsoft, you are closed off from viewing or modifying the model itself—which means you can’t really study it carefully or fine-tune it or repurpose it the way developers often do with large neural networks that are expensive to train. In short, GPT-3 is application open but research closed.
Earlier this month, in a blog post titled “Democratizing access to large-scale language models with OPT-175B,” Facebook’s parent company Meta announced an open clone of GPT-3. (The PT part of the acronym has the same technical meaning, Pretrained Transformer, as GPT-3, while the O in Meta’s version, in a not-so-subtle jab at OpenAI, stands for Open.) This was billed as being “in line with Meta AI’s commitment to open science.” Meta is really opening the door on these large language models. Instead of merely providing a frontend user interface the way OpenAI has, Meta is allowing people to download the neural network itself; providing the computer code to interact with the trained model; providing the computer code that was used to train the model (and, in contrast with OpenAI, Meta’s model was trained entirely on publicly available data); and Meta even released “notes documenting the development process, including the full logbook detailing the day-to-day training process” with details on “how much compute was used to train OPT-175B and the human overhead required.” While this access is not universal, it is quite broad. Meta says it is granted to “academic researchers; those affiliated with organizations in government, civil society, and academia; along with industry research laboratories around the world.”
Meta’s blog post includes a paragraph that sounds like a vision statement from the early days of OpenAI, before the latter went increasingly down the path of commercialization:
We believe the entire AI community — academic researchers, civil society, policymakers, and industry — must work together to develop clear guidelines around responsible AI in general and responsible large language models in particular, given their centrality in many downstream language applications. A much broader segment of the AI community needs access to these models in order to conduct reproducible research and collectively drive the field forward. With the release of OPT-175B and smaller-scale baselines, we hope to increase the diversity of voices defining the ethical considerations of such technologies.
In contrast, OpenAI’s statement explaining the rationale to keep GPT-3 relatively closed is the following:
Why did OpenAI choose to release an API instead of open-sourcing the models? There are three main reasons we did this. First, commercializing the technology helps us pay for our ongoing AI research, safety, and policy efforts. Second, many of the models underlying the API are very large, taking a lot of expertise to develop and deploy and making them very expensive to run. This makes it hard for anyone except larger companies to benefit from the underlying technology. We’re hopeful that the API will make powerful AI systems more accessible to smaller businesses and organizations. Third, the API model allows us to more easily respond to misuse of the technology. Since it is hard to predict the downstream use cases of our models, it feels inherently safer to release them via an API and broaden access over time, rather than release an open source model where access cannot be adjusted if it turns out to have harmful applications.
It should be noted that OpenAI has recently allowed users to access one piece of the neural network—the model’s “word embeddings,” which are numerical encodings of words that are used in the text generation process but which have a variety of other applications as well (this is very similar to the output of Google’s publicly available large language model BERT).
Openness might sound like an unequivocally good thing when it comes to knowledge and technology, but—as with most things—in actuality it is a subtle and complex matter. For example, consider open access academic publishing. Making research articles free sounds great, but nothing is really free. This publishing model shifts the costs from the reader to the author, which democratizes peoples’ ability to access knowledge but narrows the scope of who can publish to a smaller group of well-funded elites. There are tradeoffs, though the social currents are certainly moving in the direction of open access publishing. Journalism is another fascinating example: free access to a wide range of news articles seemed wonderful in the earlier days of the internet, but now with our awareness of surveillance capitalism and the ills of funding news through targeted digital advertising, the old-fashioned (and less open) subscription model for newspapers is regaining some popularity.
When it comes to powerful AI models like GPT/OPT, it is open to debate which of the two approaches is more ethical—OpenAI’s limited access, which it says is to prevent this powerful technology from falling into the wrong hands, or Meta’s broad access, which it says will better help society explore, understand, and decide how to deal with this technology.
A recent post by researchers at Stanford thoughtfully describes the different dimensions of openness– there isn’t just a single spectrum, because these AI models can be “open” in several different directions– and presents a proposal for a governing board that would help companies coordinate the release strategy for such models. The authors make an important point that wide, open access is commonly confused with democratization, when actually “democracy is not just about transparency and openness. It is an institutional design for collective governance, marked by fair procedures and aspiring toward superior outcomes.” The post primarily seems to urge caution, but they explicitly state that they’re not really taking sides: “Our emphasis here is that the question is not what a good release policy is, but how the community should decide.”
My own inclination, surprising as it sounds, is to support the Meta approach. As a researcher, I'm inclined to favor more transparency rather than less. It’s impossible to keep technology– no matter how dangerous– under wraps forever, so rather than deluding ourselves into thinking we can, I believe it’s better to explore and understand the technology so we can properly regulate it and mitigate its harms. Besides, if the main fear is that releasing these models openly will make harmful applications such as misinformation cheap to produce, I ask: isn’t one penny for 10,000 words pretty darn cheap already? In theory OpenAI can monitor its usage and cut off bad actors, but then it becomes a content moderation issue—and readers of Tech Policy Press know how thorny and problematic content moderation is.
While society sorts out this complex issue, it is important for all of us to be aware that there are arguments for both approaches; openness is not unequivocally better. And, it’s important to recognize that just as you can’t judge a book by its cover, you can’t judge a company’s openness by its name.
Listen to Noah Giansiracusa discuss his book, How Algorithms Create and Prevent Fake News, on the Tech Policy Press podcast.
Image: adapted from Anthony Quintano and Steve Jennings (CC by 2.0)
Authors
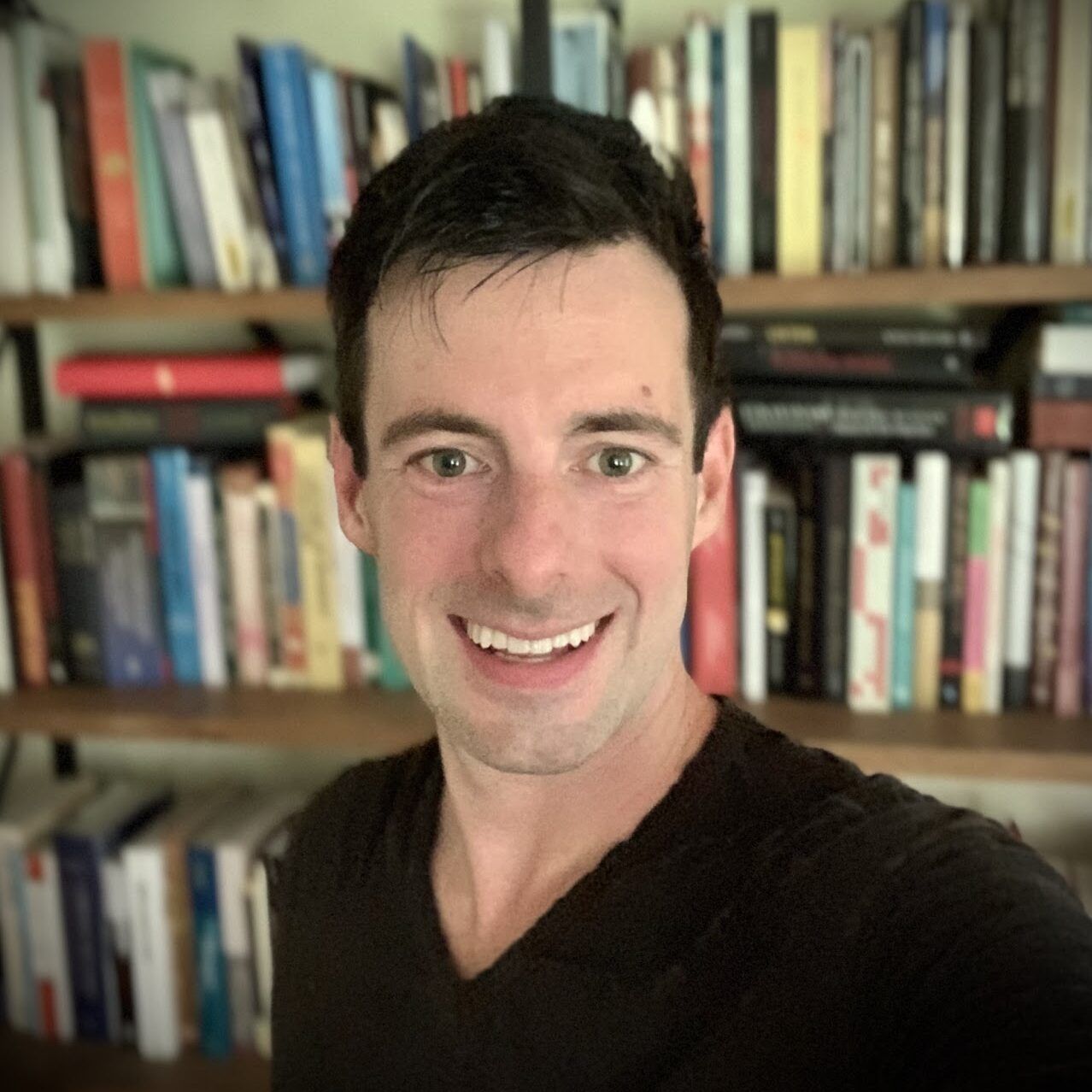