If AI is to Heal Our Healthcare Systems, We Need to Redesign How AI Itself is Developed
Michael Strange / Oct 25, 2024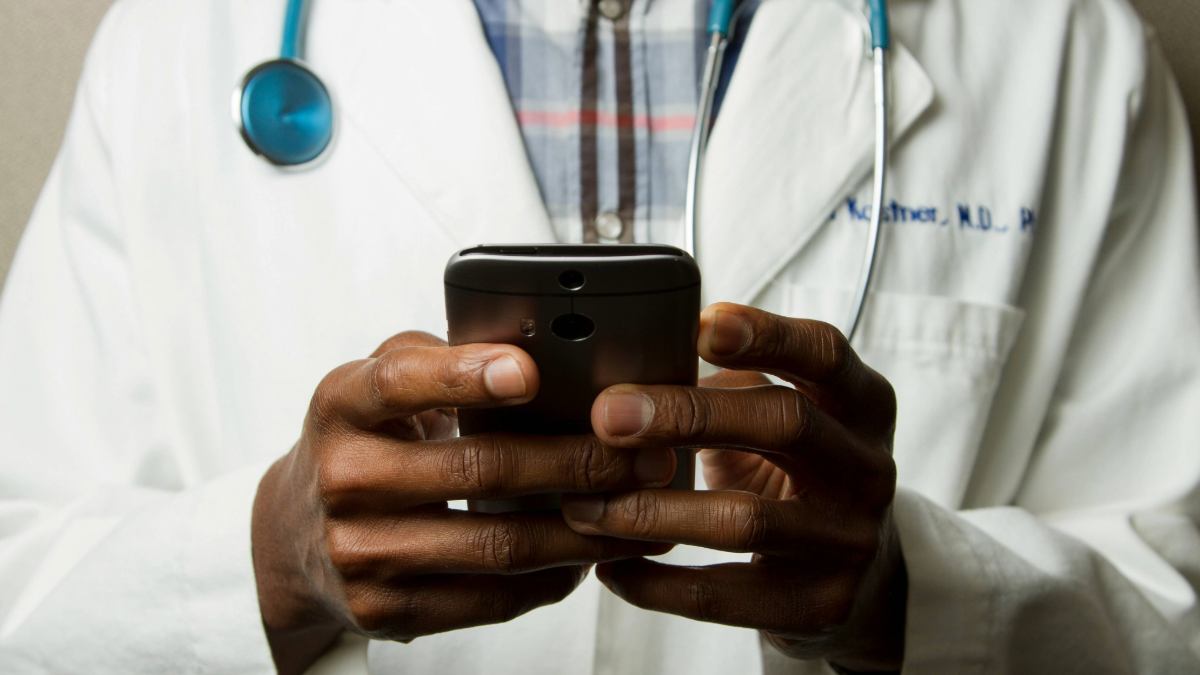
Photo by National Cancer Institute on Unsplash
Michael Strange researches on the politics and political-economy of AI in healthcare at Malmö University, Sweden.
Although healthcare is frequently cited as one of the fields where AI is set to benefit society the most, it also poses numerous challenges that risk harming human health. Ultimately, rather than the technology, it is the humans involved that will decide whether AI is good or bad for your health.
Much is promised, from solving rising costs with a mix of robots, better allocation of scarce resources, identification of illness at a point where it is easier to treat, and preventive and at-home treatments. Healthcare is, in many ways, a data business in which everything from medical research to supplying the right blood, treatment, and beds requires understanding complex patterns within big data sets. All of this suggests that AI, if applied correctly, can help improve the delivery of healthcare. Yet, to achieve that kind of innovation for good, we need to talk about the obstacles that need to be overcome.
Locking in biases
One of the biggest concerns about using AI in healthcare is that its training data is distorted by human bias. Unless somehow remedied, it not only repeats but also makes it harder to counter that bias. Medical research disproportionately focuses on the bodies of those already with the most resources in society. A woman receiving medical treatment is, for example, almost certainly going to be given surgery or drugs developed through research almost exclusively on the male human body.
Healthcare systems are known to be rife with bias; a person’s access to healthcare is dependent upon all the usual categories that shape their position in society. As odd as it might seem, we also know that statistically, many factors that determine how well a person responds to hospital treatment have nothing to do with what happens in the hospital but with societal determinants like education, quality of housing, and job security. If AI only learns from these existing and biased data sources, then as Obermeyer et al.’s study from 2019 revealed, if we ask it how best to allocate healthcare resources, it will recommend refusing expensive treatment for persons living in areas with high unemployment and poor housing. Obviously, that kind of output would greatly worsen existing social inequalities.
Intriguingly, some suggest that learning bias from humans makes AI a useful mirror by which we can better see and learn to correct our behavior. Yet, for that to work, it requires that we can be mindful humans critically monitoring what AI does with the data we feed it. Healthcare practitioners are often highly educated but is it realistic that they can always be an effective human ‘in the loop’ checking the AI?
Humans down the loophole
Healthcare is a high-risk field where mistakes can and do have fatal consequences. AI systems that, for example, detect early cancer or identify when patients require closer monitoring provide promising tools that might well save lives. Given that potential, it might even be said that it is unethical not to use AI in healthcare. Yet, as with any medical or healthcare technology, healthcare professionals need to know how to control the level of risk AI poses to their patients if they are to use it.
The so-called ’blackbox’ of AI poses a significant barrier for healthcare practitioners if there is no explainable linkage between data inputs and outputs. Another challenge is the effect of using automated tools – for example, in diagnosis or communicating with patients – on practitioners’ everyday skill sets as well as professional authority. Just as with autopilot, there is a worry that over-reliance on AI may lead to humans being unable to step in when things go wrong.
Despite these challenges, the mantra that humans are ‘in the loop’ is frequently repeated whenever concerns are raised over the safety of AI healthcare technology. For governments looking to promote the use of the technology in one of the most sensitive parts of society, the promise of human doctors and nurses monitoring the technology has become a loophole for escaping more critical questions.
Among the most important questions policymakers need to ask is who owns the algorithms trained on our healthcare data. For many countries, it might matter if its market actors own the AI infrastructure of their future healthcare systems. However, for all countries, it matters greatly whether those firms consider the needs of the local population. For example, how much space is there for an individual hospital to influence the design of an AI healthcare tool produced by a large foreign firm for which it is just one of many customers? How much space is there to meet the needs of local healthcare systems, serving diverse and often marginalized populations?
Innovating with diverse participation in the future of healthcare
Legislation such as the new EU AI Act is widely commended but also has its downsides, as it may require the acquisition of new technical expertise alien to much of society. Civil society, generally positive during much of the negotiation phase, has begun voicing concern that implementation of the Act will be led by technical committees in which technology firms will trump the voices of those more experienced in fighting for social rights like healthcare access. In most cases, it is unrealistic that charitable associations focused on patient rights, for example, will be able to acquire sufficient technical skills to have a meaningful voice in such committees unless their participation is somehow better supported.
For AI developers, the needs of patients and other relevant groups are understood in terms of ‘requirements engineering’ – identifying and meeting set conditions as to how the technology should operate. Where, for example, those requirements concern safety standards, if the developers can show that the AI meets those minimal standards, it is defined as ‘safe’ without the need for further discussion.
Engineers are also typically wary of the public. They provide useful data and function as users. Ask if the public was involved in the development stage, though, and you’ll usually be met with a roll of the eye and a sigh of frustration. The public is largely seen as disruptive and not in a good way. Customers sometimes require focus groups but aren’t seen offering much to the creation of AI systems. To be fair, such groups are often over-representative of those privileged groups lucky enough to have time and resources to join.
The COVID pandemic made clear that creating healthcare tools and policies without considering the needs of a diverse population creates counterproductive and damaging outcomes. What works for some won’t work for others due to the varied ways in which we are positioned in life, including levels of income but also culture and lifestyle.
We need courts and other legal mechanisms that allow individuals to seek remediation when AI in healthcare goes wrong. But, that is far from sufficient and, as previously said, may even make it harder for patient groups to seek redress if the language for protecting patient rights becomes that only of coders. To develop AI in ways that benefit healthcare for all humans requires that we change the basis of its development, seeing diverse voices not only as a challenge to be met but, rather, as a source of innovation.
It is far from proven that automating any aspect of healthcare will save labor costs, given that a) such tools will likely require additional human labor to check their output, and b) the history of information technology projects in healthcare is a wide cemetery of abandoned schemes and over-spends. Automation does have promise for improving healthcare, but only if it allows the reallocation of resources to where they can be better utilized.
For example, automating mundane bureaucratic processes might allow more time for humans to carefully consider the most complex and sensitive cases. In the process, bureaucratic decisions over how much care support an elderly person living at home might receive could be made more ‘human’ if the humans involved no longer need to spend time with the simpler cases. Yet, knowing which cases are ‘simpler’ and where patients are most at risk if things go wrong requires a better understanding of how they experience care assessment decisions.
How can the development process be redesigned to ask the right questions to the right groups to identify problems and innovate solutions that improve the wider system? We know that the current healthcare system is rife with exclusions, with patients feeling dehumanized and ignored. If AI can connect data in new ways, it has a role in challenging those exclusions and even rehumanizing healthcare. But, to answer how and in what shape AI should be built to achieve this potential, legislation should focus less on how to litigate harms and more on building ecosystems that support diversity within the development of AI tools in healthcare.
Authors
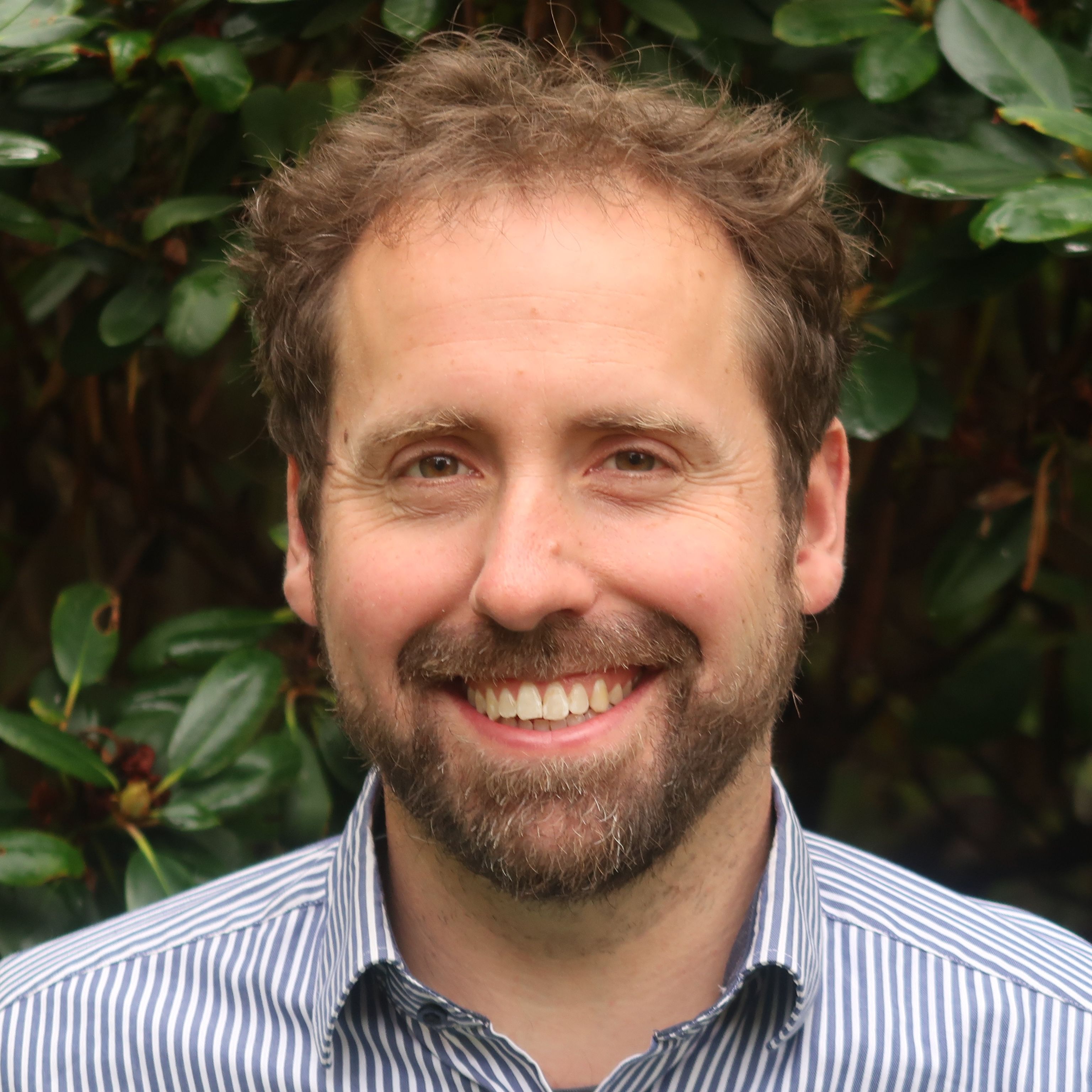