Humanity's Big Bet on Artificial Intelligence
Justin Hendrix / Apr 10, 2022Audio of this conversation is available via your favorite podcast service.
When many people– including experts– talk about Artificial Intelligence (AI), there are often some pretty big promises coded into the language they choose. Consider the language in an opening letter from former Google CEO Eric Schmidt and and former Deputy Secretary of Defense Bob Work, who chaired last year’s National Security Commission on Artificial Intelligence report:
AI is an inspiring technology. It will be the most powerful tool in generations for benefiting humanity. Scientists have already made astonishing progress in fields ranging from biology and medicine to astrophysics by leveraging AI. These advances are not science fair experiments; they are improving life and unlocking mysteries of the natural world. They are the kind of discoveries for which the label “game changing” is not a cliché.
It might appear that many political and government leaders have come to regard AI as a kind of panacea, right at the moment when the world needs one most. The third and final installment of the sixth UN Intergovernmental Panel on Climate Change (IPCC) report was published Monday: UN Secretary General António Guterres called the report "a litany of broken promises" and "a file of shame, cataloging the empty pledges that put us firmly on track towards an unlivable world."
Some leaders appear to be betting that somehow, AI will help us optimize our way out of this crisis. But what if that bet turns out to be wrong? And what if the bets we’re making within AI today, such as on technologies like deep learning, themselves turn out to be less fruitful than the hype might suggest?
To learn more about these issues, I spoke to Gary Marcus, a cognitive scientist, an entrepreneur and a writer. He’s written five books, including (with Ernest Davis) the 2019 book Rebooting AI: Building Artificial Intelligence We Can Trust, which Forbes said was one of the seven must-read books in AI. And, he founded the firm Geometric Intelligence, a machine learning company that sold to Uber.
Last month, Gary wrote a piece in the publication Nautilus titled Deep Learning Is Hitting a Wall: What would it take for artificial intelligence to make real progress? In it, he wrote that “because general artificial intelligence will have such vast responsibility resting on it, it must be like stainless steel, stronger and more reliable and, for that matter, easier to work with than any of its constituent parts. No single AI approach will ever be enough on its own; we must master the art of putting diverse approaches together, if we are to have any hope at all.”
I spoke to Gary about how his criticism of where AI researchers are placing their bets connects with the larger wager elites seem to be making on the promise of AI.
What follows is a lightly edited transcript of our discussion.
Justin Hendrix:
Gary, reading your recent writings on the state of artificial intelligence, you are concerned. What is driving your concern? What is perturbing you?
Gary Marcus:
Many things perturb me, to be honest. And I'm very worried about the state of the world in general, and I can only help in some pieces of it, maybe a little, if I'm lucky. But, I'm concerned about AI for a couple of different reasons. One is, I think that the public and policymakers have a very broadened cut on what AI is. They kind of treat it like magic, and it isn't magic, and it matters that it's not magic.
People will just talk about AI ethics or something like that in the abstraction of what a particular AI technology does, and one of the things I like for people to realize is that AI is really a set of tools. It's not one tool, but many, and each of those tools has its strengths and its weaknesses. If you talk to someone about the statistics and what he really knows, the first thing he'll say is, "Whether you can use a statistic in a particular place depends on whether your data meets certain assumptions. They should be normally distributed and so forth. And if they don't, then a particular statistic might be the wrong tool for the job."
Well, it turns out that we have some very powerful AI tools right now, and we have some limits on those tools. They're not universal solvents, but they often get treated as if they're universal solvents, and that's problematic when you think about policy. For one thing, today's tools are not tomorrow's tools, so we have to think about what we can do now, and what we can do in the future.
And then, different tools have different appropriate functions, so we don't need to worry too much about the AI that we use now in recommendation engines. We need to worry a little bit, but if Amazon tells you to buy one of my books because you bought another one of my books, and you don't like the second book, so what? You're out $20 bucks. Doesn't matter. But, if a radiology system is pretty good at reading images, but is not very good at compensating for dirt on a slide, and there's dirt on your slide, that's really bad. There could be serious consequence there. So, we have to think about things like, what is the consequence of the decision that is being made by the AI, and what contexts are it good in and not good in?
I can give you another example: driverless cars. People kind of treated them, in 2012, like they were imminent. Sergey Brin said we'd have them in five years, and there's been so much talk about driverless cars, and it turns out it's easy to build a prototype of a driverless car, but it is not very easy to build one that is reliable across all circumstances. So, if you think of a driverless car as a kind of general technology, you're like, "Great! That's cool! I won't have to drive! I'll be able to text message and talk to my kids in the back," it sounds really awesome, but the reality is, it's better on the highway than it is in the city, and it's better in sunlight than it is in rain and snow and fog. There's a broad range of contextual limits. It's better, it turns out ... and maybe this is the most important point ... with things that are familiar, and not so good with unfamiliar.
For example, a Tesla the other day ... There was a person attending to it, but an autonomous Tesla left to its own devices would've run into a human being holding a temporary stop sign in the middle of the road. Well, that's bad, right? And the reason that it would've happened is because it's been trained on pictures of humans and pictures of stop signs, but not pictures of humans carrying stop signs. That was out of its training set. And because the current AI that we use is naïve, it wasn't able to put together its knowledge about these things individually; how a person works, and how a stop sign works. It doesn't really have a conceptual understanding of this, and it makes it very unreliable in unusual circumstances.
And that's actually true of most of the AI that we know how to build today, and it's really important that policies understand that fundamental unreliability, and that they understand that it comes from a disconnect between what systems of the current regime are trained on, and how they extend into the world.
A good human driver can think about situations it hasn't seen before, based on that human's knowledge about how the world works, but the reality is the AI we have right now is very superficial. It mostly relies on memorization and some tricks a little bit beyond memorization, but it's really important to understand that, so that's one reason why I think policymakers really need to dig deeper into the substance of AI and where it is today rather than just treating it as like a magical wand.
Justin Hendrix:
Is there another example you might give?
Gary Marcus:
People are really enamored of these things called large language models. The most famous one is GPT-3, which has written op-eds in The Guardian and The New York Times, and parts of book reviews and whatever. It gets an enormous amount of attention, but it actually has a lot of drawbacks, and there are a lot of extensions to it, too. This is a class of models. We call them all large language models. They have other names, like Gopher is the largest one that DeepMind put together, and there's kind of an arms race to build bigger and bigger versions of these things.
And they turn out to have a bunch of problems, and it stems from the same source, which is the really superficial mimics of what they've seen before, and you move away from the things they've seen before and they do weird things. We know now that they produce toxic language, they perpetually pass historical bias, they pass along misinformation, and they create new misinformation. Doug Hofstadter, that probably a lot of people know from Gödel, Escher, Bach and some of his other great books, sent me an example the other day where one of the systems is asked, "When was Egypt carried across North America," or something; the system just makes up an answer to it. You don't know that it's made up, this example. So, they'll just blithely answer any question, often in fluent grammatical prose, but with nonsense.
So, you have these systems that are actually in some ways dangerous. If you put in a chat bot, it might tell you to commit suicide or genocide. Those are actual examples in the literature. Nobody acted on them, but the systems are fully capable of doing that. So, we have these systems that are getting enormous investing, billions and billions of dollars, and I don't think they're the right answer to AI. What's at stake is, we might be able to build an ethical AI that can reason about values and help us, and do the things that we want, or we might wind up with these things that I think are like broncos; are kind of wild, bucking creatures that are impressive, but have a lot of risks associated with them. That matters because we're putting more and more power in these things, so there are more and more chat bots that people interact with.
Even worse, and the thing that I think I'm most personally worried about right now ... only because it's one thing where I think I could help, I guess, but it's a serious problem ... is misinformation. So your listeners probably already know how huge a problem misinformation is, how central it's been in COVID, and how central it's been in the war in Ukraine and so forth. GPT-3 is terrible at detecting misinformation, but it's really great at making it, which is an awful thing. What it allows you to do is type in some sentences and then get 100 variations on them, and if you don't care whether they're true and you're running a troll farm, it's like a dream come true. If you post the same nonsense a thousand times, then a machine might pick it up, but if you post a thousand different versions of that nonsense, some current machines don't know how to pick that up, and so the problem of misinformation is going to explode in the next couple of years.
I'm thinking a little bit personally about whether I could help with that problem, so that's one that's on my mind, but it's yet another example of how we have technology right now that we don't have very good control over. So, I sometimes say this, which is, AI 100 years ago was no problem for humanity, and 100 years from now, it may be the best thing that ever happened to humanity. It might solve our problems for climate change. It might solve our problems for creating food more efficiently, although not the distribution networks, but it might make tremendous positive impact.
We could really get to a post-scarcity society, like Peter Diamandis has talked about, and that would be fantastic, but right now we're in the worse moment of AI, and it's the worst moment of AI because we have all these tools that we don't really have formal guarantees over, that do kind of erratic, strange things, and yet we're giving them power. We're giving them power to make decisions about people's jobs or loans, or giving them medical advice that they're not really qualified to give. So, we're going to look back at this not as the golden age of AI, though it feels that way maybe because there's so much more of it than before, but as kind of a lousy moment.
I mean, there are some things it does now, just in fairness, like tagging photos and stuff, sometimes ... not always ... can be a good thing. And speech recognition is great. So, there's some real positive AI, but there's a bunch of things to be worried about right now.
Justin Hendrix:
I want to kind of dig into one of the causes for your concern. This article you have in Nautilus, Deep Learning Is Hitting A Wall, and you ask, "What would it take for artificial intelligence to make real progress?" Can we, for my listeners' sake, spend a minute on why we've reached what you think of as a wall or technical boundary where it might be generating some of this concern?
Gary Marcus:
Yeah, and we can talk also about why my title pissed so many people off. There are two readings, I think, of the title, and maybe it's my fault that I chose a title with two readings. One is like, we can't go any further, and the other is, there is a serious impediment that, if we don't get around it, we have a problem. And I meant it more like the latter, which is maybe more moderate than people realize, and I think the substance of the article makes that clear, but the title might not have.
We're not hitting a wall in the sense of like, there's new discoveries every day with deep learning. We're always finding new things. We get better at making deep fakes, which is another ethical problem I didn't mention. Deep fakes are much better ... much better, in quotes ... much more convincing now than they were a month ago, or four months ago, and they're more convincing than a year ago, so there is steady progress in deep learning, but there is a set of problems that have been around literally for decades that I pointed to in my 2001 book The Algebraic Mind and in a New Yorker article we can put in the show notes, in 2012, that are just persisting and not getting solved, and really matter.
Primarily, those have to do with reasoning and language, and more generally with what you might call deep understanding. So, deep learning is the popular technique, and it has an enormous kind of propaganda value, because it sounds conceptually deep, but the reality is the word 'deep' in deep learning just means how many layers in a neural network. It's a very technical sense. It's not deep in the conceptual sense of understanding the things that it talks about or it interacts with.
The Tesla driving example I gave you is an example where the conceptual understanding of a person and a stop sign are not that deep. It really just knows, "I should go into this mode if I see this thing in this place." It doesn't really understand, "We're driving in a world with citizens." It doesn't have a deep conceptual understanding.
And in terms of reaching deep conceptual understanding, we're not actually making much progress. Here's an example that I published a little while ago. Ernie Davis I think actually made the example, or we did it together in joint work, which is, you tell GPT-3 you're thirsty, you have some cranberry juice, but it's not quite enough. You find some grape juice. You sniff it. You pour it into the cranberry juice. You 'blank'. And then what the GPT says is you drink it, which is statistically correlated with words like 'thirsty' and whatever, and then it says, "You die." So, it's not conceptually deep understanding of cranberry juice and grape juice to think that if you mix them together, you'll die. It is conceptually shallow, and has to do with statistics of words that are in some database.
And that's characteristic of these systems. What's been interesting is, for 30 years I've been critical of these things, and people always say, "Well, give me more data." Well, now these systems have more data than God. I mean, not quite literally, but they have terabytes of input data now, and they have billions of parameters. They're massive. They're a real test of John Locke's hypothesis that you could learn everything from data. And they're failing at that.
In 1990, you could've said, "Well, these systems don't really have what they need, and let's see. Let's give them a fair shake." But now they've had their fair shake, and they are still really stuck on these conceptual problems. They can create fluent language by kind of doing and kind of parroting back what they've seen, but they don't understand what they're talking about, and that is a wall, and it's a wall that I don't think current techniques can go past. And what I offered is that we need to hybridize them with another set of techniques from classical AI that are really about knowledge representation and language and symbols and so forth.
I'm not even saying deep learning needs to be tossed away or anything like that, but I think we're spending too much time looking at one possible answer when there are many other answers that might be more profitable. And it's a very unpopular thing to say in certain quarters right now. I got a lot of pushback. Yann LeCun posted a lot of mean things on Facebook making fun of the title, and on Twitter and stuff like that, so, some of the elites in that field are very upset that I should dare to question it, but I think we have to question it.
Justin Hendrix:
So, you are taking on folks, for instance, who are invested in the immediate promise of some of these technologies; the autonomous driving industry, the Elon Musks of this world, or even folks like Sam Altman, the CEO of OpenAI-- who, by the way, has recently said something that you reminded me of just a moment ago, he wrote earlier this year about how close we are to abundance, this idea of artificial general intelligence, and how that will help us to cure all human disease, build new realities... this sense of there being this immediate future that's available to us if only we can invest enough in these existing corporations.
Gary Marcus:
Well, I mean, of course he's going to tell you that, given the company that he's CEO-ing, but I don't think it's realistic, and I think actually most people in AI don't think that what Sam posted in his Moore's Law For Everything blog post is realistic. I mean, he's talking about in five to 10 years, we're going to solve all these problems. And the premise is that scaling, making models bigger, is going to solve the problems, and that's really what I was attacking in the Nautilus essay, and I was attacking it in a few different ways.
But, Sam's premise is basically, we found this law that says you put in more data and the systems get better in a very predictable way, and I pointed out a few problems with it in my Nautilus essay. One is that getting better on some measures doesn't mean getting better on all measures. I would argue that on deep comprehension, we haven't really gotten better. We don't have systems, for example, that could watch a movie and tell you who did what to whom. We don't have them now, we didn't have them 20 years ago, and we're not going to have them next year. We will eventually have them. None of these problems are impossible. But, I would not say that we've made the same speed of progress on these kind of more conceptually deep problems as we have on some more shallow problems, like can you recognize whether this digit is a three or a four, where we have made exponential progress. So, staying that we've made exponential progress in some domains doesn't mean that we are in all domains, and I think Sam was very fast and loose about that in his essay.
It's also a mistake to think that something that has happened will continue to happen. It's an inductive fallacy. And so Moore's Law, it turns out, held for a long time, but it was a generalization over data rather than a law. It's not a law like F=ma. It is just a regularity, and it turns out that Moore's Law actually slowed down in the early part of this century, and you could find that you keep adding more data, and you get better and better, and then you stop getting better. A place where people should really worry about that is the driverless car industry, because the main thing that people are doing there is trying to collect more data in all kinds of interesting ways. They have more cars on the road, they do more simulated data in Grand Theft Auto or something like that, but the premise is, if we just get enough data, this will work.
And it might be wrong. It might be that you never get these weird cases that I'm talking about, like the human carrying a stop sign, and if they're not in your training data and your whole shtick is to rely on the training data and making training data bigger, you might have a problem. And I think that's going to be true in the driverless car industry, but it's am empirical question. We don't have the answer. But, so far, we've gotten six orders of magnitude more data or something like that, and we still basically have the same problem of unreliability. You can't really trust these things.
There's a level two driverless car, which is cruise control that helps you out a bit. And there's level five, which is like you can just get in the car and say, "Go from point A to point B," and it'll do it and you don't have to be involved.
We don't seem to be getting close enough to reliability to actually do that. We might do things like, in Arizona, in good weather, on roads that have been well mapped, we might be able to make that work, much more narrow case. The general case, it doesn't seem like just adding more and more data is actually working, and so I pointed all of that out in the essay, and then I pointed out some cases where we actually might already see hints of a slowdown.
There's these theoretical problems, like you can't assume that it is going to be across the board. You have to actually show that, and it hasn't been shown. And then there are actually hints that maybe there are some slowdowns already. One example ... I don't remember if I put this in the Nautilus case, but ... is anthropic AI showed that adding more data made systems a little bit better at toxic AI up until a certain point, and it looked like already we were maybe reaching the point of diminishing returns, and on that particular measure, we were topping out at 80%. Well, you don't want your ethical AI to top out at 80%. That's not going to be good enough.
So, putting all this weight on scaling, I think is a mistake. And in some sense, that was the newest part of the essay, because there's a lot of the field right now obsessed with this idea that scaling is going to solve the AI problems. Sam Altman's essay is a really good, I think, example that he doesn't quite put it in those words, but that the title is basically saying that. And I don't think we can make those projections. I think, in fact, until we solve the problems of deep comprehension, then we're not that close. Maybe once we solve them, we will make rapid progress, but I think that there are many things involved, actually, so I think we need better algorithms, but we also need to have algorithms that can interact with large scale databases of human knowledge.
Here's an embarrassing fact about AI that has been mentioned before, but is not publicly known or contemplated enough. AI can't read. It's illiterate. It can do keyword matching, and Google is an amazing thing that does a lot of keyword matching, but we don't have systems where you can pour in a paragraph and have it come out a real representation of what that paragraph means. And there are tools like summarization, but they're always sloppy. They're never reliable.
Machine translation is a great victory, but the machine translation doesn't understand what it's translating. Still makes mistakes if you look at a fine grain level. And at a more general machine comprehension level, we just, we're not there yet, so I think there's a ... I don't want to call it a fantasy. Something like a fantasy. There's a vision, I think is the word I'm looking for. There's a vision of AI eventually being able to read the internet in order to rapidly make itself smarter.
And there are some questions about would that be dangerous, but let's hold those for a second. It would certainly seem to be a good way to make AI smarter. We send people to school, and AI's ought to be able to read in the same way that kids learn a lot from reading. And we just can't actually do that now, which is a reality check, and I think we will eventually be able to do that. I think we will see exponential advances at that point, but we should be asking, "How do we get our systems to read," and not just, "What happens if I pour more data in," because it's not getting us to systems that can read.
Justin Hendrix:
Part of what I sense in some of these what you call visions or perhaps perceptions of where things might be able to get to in the near term, is almost a kind of just stubborn insistence that we will solve these problems before we run out of time; that, even as we kind of see that some of the systems we're deploying–the technology systems, and certainly the transportation systems and energy systems, all the rest of the architecture of modern humanity– that we'll be able to kind of use artificial intelligence to help ourselves avoid all the downsides of all of that, and do that in a timeframe that allows us to kind of carry on consuming in perpetuity. That strikes me as a really big bet we're taking.
Gary Marcus:
I think that's the right way to think about it, as a big bet. You could think of my whole career as trying to up the odds on that bet, but it's a bet. And right now, I don't feel good about it. I feel like it could turn out positively. Right now, I think that the net impact of AI is arguably negative, so I think the single biggest thing that AI has done is to disrupt the texture and fabric of society through all of the News Feed type things on Facebook and so forth; that it really made people much less civil to each other than before. Not that we were ever great, as a human species, but we have problems like confirmation bias. We notice things that support our own ideas; we don't notice things that go against our ideas. So, we have some inbuilt cognitive limitations, and AI has actually made those worse.
News Feed, I think, has been the single worst thing from AI that I can think off the top of my head, because News Feed has really made people antagonistic to one another. Similar things on Twitter have not been great. And then the ecological footprint of these new large language models is pretty massive. Sometimes one training run can compare to what a small town will use for two weeks of energy or something like that. And there's constant efforts to make them more efficient, but they really do use a ton of energy, so that's the cost. And then there are some positive things right now, like Wikipedia's not really AI. It gives us more internet, but it's an example of a technology from the modern era that's fabulous. It really helps people around the world. And turn-by-turn navigations use classical AI ... not neural networks, but it's very useful to many people every day, so there have been some contributions.
The big ticket contributions that we might envision, we haven't made yet. There was a roundup of AI studies on COVID ... or AI's contribution on COVID, by Will Heaven in the Tech Review, and the headline was something like '400 Efforts To Have AI Contribute To COVID Have Led To Nothing'. And I'm not ever saying AI won't do X, but the reality is, right now the tools have often failed to live up to their billing, and just pouring more data is not necessarily solving those problems. In the worst case, if we continue the trend lines, if you want to talk about scaling, GPT-7 would use ... I'm making up the number. Maybe it's eight or nine, but it's a certain number of doublings. GPT-N would use more power than New York City or something like that. That would be not a good thing at a serious level for ecology.
What we hope is that AI will help with these deep problems. I think there's some promise of that. I think people are working on the kind of architectures that I'm lobbying for-- called neuro-symbolic-- and maybe there'll be some progress there. Those are not, by any means, done. I think they need more resource investment in them. A lot of this really is about where we should place our bets within the field so that that larger bet can work out in the right way. The larger bet is that we'll be able to find a form of AI that will be able to solve our problems. My view is we need to diversify our portfolio if we're going to win that bet. Right now, we're putting most of our investment in large neural networks with large training sets, and it's gotten us some fruit, but I think we need to look carefully at where it has failed, and to look at a broader set of architectures if we're going to make good on that promise of having AI help us with the big problems.
Justin Hendrix:
I reckon that policymakers are probably ages away from being able to think through some of the types of problems that you're describing here, and perhaps it's not their job to do that. Their job is more to look at creating a framework where we can reasonably be assured that society's interests are helped and not hindered by these technologies. If there were a congressional representative on this call right now, and you could spend a few minutes telling them what you think they ought to be looking at from a policy perspective, what would it be?
Gary Marcus:
I'll start with a couple of examples, and maybe I can come up with something more general. One is in the driverless car industry. We do not have enough regulation. Essentially, you can beta test anything without consequence, except such as might happen after the fact if you get sued. And I'll give you a very specific example that worries me, is Tesla has driverless cars out there ... I mean, they're not really driverless, but what do they call them? Full self-driving, which is not really accurate. Sorry. I'll actually interrupt myself.
Tesla should not be allowed to call what it has full self-driving. Congress should just shut that down. It is false advertising, and it is dangerous. It's not full self-driving in the sense that you see Elon Musk on 60 Minutes not holding onto the steering wheel, but you actually have to hold the steering wheel. If you don't, somebody can die, and people have died. I mean, some of the cases I guess are controversial. You could argue about that a little bit, but it is clearly dangerous to have consumers think that something is full self-driving when it is not certifiably level five self-driving, and so they just shouldn't be able to call it that. That's point one.
Point two is, we have people out there on public roads that are beta tests in studies of whether it works, and they are not consenting adults, and there aren't a lot of laws in place about what might be required. Here again, Teslas, I think, are particularly problematic. In California, they're not required to give data because they're not registered as being, despite their name, a full self-driving system. So, many other manufacturers are working on safe driving in California and turn over data regularly about how often humans have to intervene on their systems, but Tesla has found a loophole around that. That loophole needs to be tied up. Tesla needs to report the same data as everybody else.
So then ... continuing with Tesla, because I'm most concerned about them ... they have a known issue, and this is, I think, a good example to think things through, which is their cars sometimes running into stopped emergency vehicles, or stopped vehicles of any sort on the highway. I don't know the technical cause for that. They're not that forthcoming about the data, but it's happened at least a dozen times. They're under investigation from the NHTSA for it. I wrote about it in my 2019 book. I think there had already been five cases at that point, so this has been going on for a while. It's still going. There's no regulation that says that they have to take the car off the road, despite having no known issue, nothing that requires them to resolve that issue.
About two weeks ago, somebody in Taiwan died in what I would say is an indirect consequence of this particular problem. A Tesla ran into an emergency vehicle. Nobody died from the Tesla itself, but then an emergency worker who was putting up traffic cones to mark off the accident, the emergency worker was killed by another vehicle that stopped suddenly ... or didn't stop adequately, because they were taken off guard, because in the middle of flowing traffic was this problem. So, it wasn't directly caused by Tesla, but had Tesla solved this problem of repeatedly running into stopped vehicles, that emergency worker wouldn't have been out there and would not have been killed.
This is serious business, and I think we need to have more regulation about when can you beta test things, and what data do you have to provide, and what are the standards, if you have a known issue, for solving them before you can go back out on the road. This has been a problem that's been known for four years, and what I get on the internet when I post this is Teslas are safer than other cars, but we don't know that. They're probably, on average, safer because they're newer than the average car out there. You compare a 2021 Tesla with a 2005 Ford Escort, yeah, it's probably going to be safer, but we don't have the accurate data.
And I think that there’s another part of the regulation. They should basically– all driverless car manufacturers should have pretty full disclosure about what the data are that they're collecting, how they're evaluating, if they want to make safety measures relative to the ordinary drivers. We need to know what vehicles, under what circumstances. Were they put into self-driving mode as opposed to not? For example, if it's all highway miles and you're comparing with non-highway miles with the other vehicles or whatever, we need to understand that the academic community can look at these questions, if the data are out there, but Tesla has not been forthcoming. They shouldn't be able to make claims without full disclosure of the data, because the public are beta testers, whether they like it or not. So, we should have some say in how those cars are regulated. If anybody, any of your listeners wants a longer serious conversation, I'm happy to do it. You can look them up.
And then let me give you the second example, is misinformation. I think– and it might be self-serving because I'm interested in working on this problem, so, disclosure there– but I think that we need to have a regulation that has teeth, that requires social media providers to detect misinformation, as well as is possible with whatever available technology there might be at that point. In the financial industry, there are lots of things. You must use the best available technology. The legislation doesn't say what the best available technology is, but it says in order to comply, you have to use whatever's out there. I think we need to mandate that social media companies use best available practices, which could involve AI or humans and AI or whatever it is, to detect and label misinformation, or potential misinformation.
So, right now, there's a little bit of that once in a while, a particular claim, like around election time, there's a lot more monitoring of these things, but I think we need it across the board, which is a huge undertaking. But, ultimately, I think that the world is becoming contaminated deeply by misinformation, and it's not enough to say, "I'm an aggregator, I'm not editorial," which is kind of the stance that Facebook and Twitter and so forth have taken. I think we have to have legislation that says if you aggregate the news, and you do this in volume, you are responsible for some kind of best-in-class compliance check to make sure this stuff is true. And I think the legislation we have on that is minimal right now, and I expect that that will actually change, because the problems are so deep, and I think people care about it, but I would say get on it. It's important.
Justin Hendrix:
Looking at the situation that we were just going through, this idea that AI is the ultimate panacea that may allow us to kind of continue to consume, live our modern lifestyles, have the economy function roughly as it does today, while also avoiding the worst consequences of climate change, perhaps other issues around population or whatever, other resource issues.
To some extent, I always worry, lawmakers, especially in the United States, are just as invested in the idea of the panacea as perhaps these tech CEO's. I mean, they don't want to necessarily believe that type of cornucopia is so many decades away that we need to act more drastically. How do we reconcile all this? Is everybody invested in this religion of artificial intelligence?
Gary Marcus:
Everybody's always looking for a panacea, right? I mean, we had snake oil in the early 1900s. And I don't mean to say that AI is snake oil, but there is that panacea thinking that you're talking about, and it's not realistic, at least any time soon. And it is tempting. People can look for a higher power or something in a bottle, or they can look for a machine.
Even in the best case, if we had all-knowing really smart AI, it still wouldn't be a magical overnight cure. Take food security. Some of that's a question of, can you come up with a good plan to make food more efficiently? And some of it's distribution. What do you do about warlords who take the food and don't distribute it? So, there are always, I think, going to remain political problems that are pretty serious, even if you have systems that can reason better, that can keep track of more complex problems, can do technological innovation.
The biggest hope ... and I don't think it's totally unrealistic ... is that we will be able to make food much more efficiently. We will be able to figure out technological fixes to climate change. I don't think those things are ruled out. All of them, they're always going to require political will to implement, and many of the ... We shouldn't be resting a lot of hopes on them right now because we don't really have those technologies right now.
In each of them, there are ways that AI can already contribute, so there will be ways in which AI helps, for example, with drug discovery. There are lots of candidate molecules that seem interesting, that AI have identified some of them will turn out to be useful. There are some contributions to new materiel science that may turn out to be useful for climate change. So, there's already steps in those directions, but we don't have anything right now like the Star Trek computer where you could kind of pose a query. "I'm sitting on this planet, the temperature is rising a certain number of degrees every year. What do I do about it?" The Star Trek computer could be like, "Well, why don't you build this thingamajig and hook it up to a framulator," and you say, "Well, what's a framulator?" and it explains to you. We're nowhere near that.
We're more like, we have calculators that can help us calculate many different things. Many of them use AI, and in conjunction with humans, we can make some progress, but slower than we would like right now. And I do think ... I guess here's another thing to think about. I think it's good pouring money into AI, because AI can help these problems, but I think there has to be strong mechanisms to diversify our intellectual portfolio in AI. And right now there isn't. There's a billion-dollar grant or whatever, but there's no mechanism in place to make sure that it doesn't just go do the same stuff that industries are already doing. Then the money's not necessarily efficiently spent in terms of exploring a large space of potential solutions that haven't been explored, that might be helpful.
Justin Hendrix:
So, you started this conversation off with you're perturbed, you're anxious about some aspects of where things are headed. Do you remain an optimist in the long run?
Gary Marcus:
Short-term pessimist, long-term optimist. I'm slightly more optimistic about the short-term than I have been before, because I think even though I get a lot of flack for pointing out walls and whatever, there's actually a bunch of people thinking about these things now, and more so than before. It's great that Yoshua Bengio, Turing Award winner, is really taking this stuff seriously now, and it's great that Josh Tenenbaum, for example, is doing really interesting work at MIT. There's a bigger cluster of people thinking outside the box that the field has been stuck in for the last few years. I'm trying to make an even bigger set of people move outside the box, but at least there are some people doing that now, and that gives me some short-term optimism. Not at the scale that you're talking about, like that it's going to solve the world's problems, or driverless cars. I think all of these things are a number of years away, but if we get people on the right path, they can be smaller numbers of years away.
And with something like climate change, every minute counts. Chomsky gave this interview the other day where he said that basically the Ukraine situation, Russia's invasion, might mean the end of the world. He almost said this literally. And he didn't spell out the argument, but I think what the argument ... It was with respect to the climate report that just came out, saying we might already be too late, essentially. And I think the argument is, if we're distracted on other things ... I mean, we should, of course, be paying attention to what's going on there, but if we have to send a lot of resources over there, and it's in the top of the news every day, an important climate change thing doesn't make it to the top of the news, then we are distracted. Maybe distracted with good reason, but we're delaying.
And the temporal window is so narrow for us to fix these problems that it's worth massive investment. I mean, we don't know exactly, but there are conceivable worlds in which a year's delay in implementing the right technology could make the difference for billions of people's lives. We have to take that seriously, and think, "Are we solving these problems in the best way that we can?"
In the long-term, sure, if we don't have nuclear war or climate change or something like that, then the trajectory is positive even if it's not as fast as I would like, and eventually we'll be in a much better place, but we have to get there before we do something catastrophic.
Justin Hendrix:
I guess my great fear is that it's hard to get there in the long-term without going through this very short-term period of just authoritarianism and strife and climate disaster and refugee crises and the rest of these things. I worry that, while folks like Sam Altman want me to see the cornucopia across the ridge, that I'm not going to make it, or my children might not.
Gary Marcus:
I mean, far be it from me to tell you your head shouldn't be there. I think these are perilous times. Going back to what you said, there's a bet that the AI community is placing on behalf of the world or something like that. There are things that the AI community has very little influence over at all, like the Russia/Ukraine situation, and that could turn into a nuclear war and there's nothing the AI community can substantially do about that. So, there are that level of problems.
There are things like climate change, where I think it would be great if 90% of people in AI said, "I'm going to stop working on what I'm working on right now and see if I can help that." Or the disinformation problem, which maybe I might start doing.
Something like 75% of commercial efforts on AI, at least a couple years ago, were on advertising ranking and stuff like that, advertising placement. If everybody who worked on AI ads ... Maybe it's 60% now or whatever. If everybody who's working on AI ad placement, or things that are kind of peripherally related to that, woke up tomorrow morning and said, "You know, I care about my children, my grandchildren, and I'm just not doing this anymore. What can I do to help, either with the misinformation problem, which affects the climate change problem, or the climate change problem directly," that would be significant.
Justin Hendrix:
Well, something perhaps to talk about when your new venture comes along, I hope. We can have you back on and you can tell us what you're doing against that particular problem.
Gary Marcus:
It was a real pleasure being here. I really hope policymakers will dig deeper into what AI can and can't do, realize that it's not a panacea, and that trying to get it right really matters.
Justin Hendrix:
Thank you.
Authors
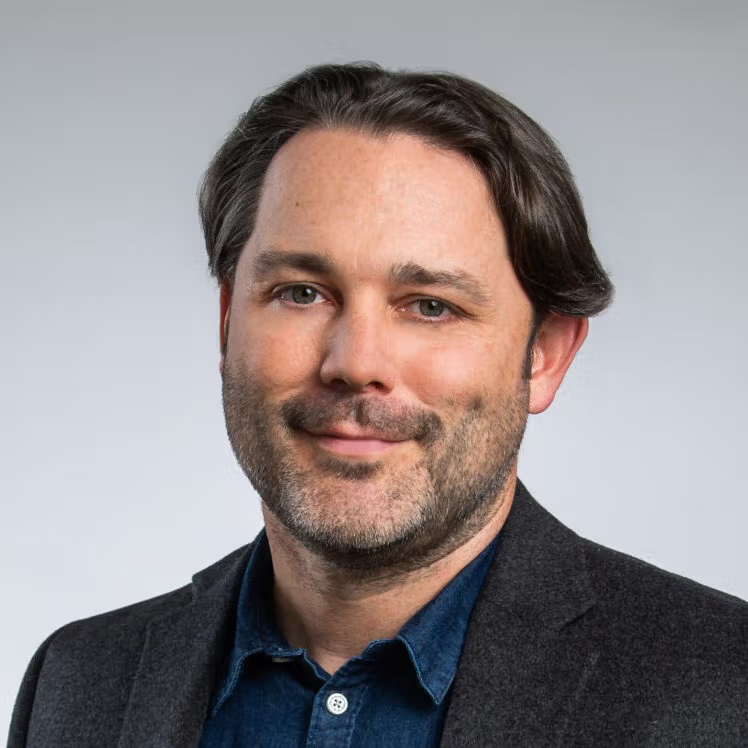