How the EU AI Act Can Increase Transparency Around AI Training Data
Zuzanna Warso, Maximilian Gahntz / Dec 9, 2024As AI companies' large language models get bigger and bigger, the reverse is true for what we actually know about how these models are developed. With increased competition in the space, leading companies have sworn off transparency in order to gain an edge over their competitors. While Open AI still published information about the exact composition of the training data used to train GPT-3, the model underpinning the breakout success of ChatGPT, no such information was shared following the release of its successor, GPT-4.
But gaining a better understanding of the data used to train generative AI is crucial to advancing our ability to scrutinize AI models and their impact more broadly. Transparency around training data can thus allow people to trace where this data comes from and scrutinize how it may affect the behavior of the model it is used to train.
The EU’s AI Act, which went into force earlier this year, provides the most significant opportunity to advance transparency around training data to date. Amongst many other things, it mandates that developers of so-called “general-purpose AI” (GPAI) models — EU lingo for “foundation models” — publish a “sufficiently detailed summary” of the data used to train their models. The aim of the summary is to better protect those with a “legitimate interest” in this data, such as copyright holders or privacy watchdogs who want to understand whether the training data comprises copyright-protected or personal data. What this summary should look like is subject to ongoing debate as the EU’s AI Office is developing a template for the summary and needs to answer a range of different questions: what data information should the summary comprise? What does “sufficiently detailed” mean? And what should qualify as a “legitimate interest”?
The case for comprehensive transparency
Earlier this year, Open Future and Mozilla Foundation published a policy brief addressing just these questions, accompanied by a blueprint for the “sufficiently detailed summary” of the training data. In the policy brief, we outlined the grounds – “legitimate interests” – that justify access to information about the content used to train GPAI. We explained that the range of legitimate interests of parties interested in increased transparency of data used in the development of GPAI models goes beyond copyright issues and encompasses privacy and data protection rights, the right to science and academic freedom, the prohibition of discrimination and respect for cultural, religious and linguistic diversity, fair competition, and consumer rights. The purpose of the sufficiently detailed summary should be to ensure that parties with any of these legitimate interests in knowing what content was used are able to exercise their rights.
The ability to exercise these rights depends on having access to different types of information about the content that was used in the development of GPAI models: the overall composition of the training data, data sets and their sources, data diversity, and (pre-)processing steps taken to, for example, filter, anonymize, or enrich the data. But AI developers are pushing back on this, often citing that this information constitutes trade secrets.
The following analysis explains why this information is necessary to fulfill the purpose of the sufficiently detailed summary under the AI Act — and why trade secrets cannot serve as a blanket excuse for a lack of transparency.
The AI Act’s preamble calls for listing main data collections or sets that went into training a GPAI model, such as large private or public databases, as a step to address the transparency requirements under Art 53 (1) d. The information about data collections and data sets is essential with regard to a range of legitimate interests. For instance, in terms of copyright, rightsholders need to be able to determine what works have been included in the GPAI training content; and to better protect people’s privacy and personal data, information about data sources and datasets can provide an indication as to whether GPAI training data may comprise personal data.
But the devil is in the details here. Companies have ample incentive to disclose as little information as possible — in fact, it might re-direct attention and scrutiny toward those opening up datasets. So without specific instructions on what makes for a sufficient summary, there is a risk that providers publish only high-level information about composite data sets. To alleviate this, the information required in our proposal for the template requires clearly identifying datasets, listing the data sources, and providing meaningful information about how this data was obtained. For datasets composed of multiple collections — including both public and non-public sources — providers should be obliged to clearly identify their origins.
It’s important to acknowledge that the various different data points won’t be equally relevant to each stakeholder. But all of the information included in our blueprint is relevant to protecting the different legitimate interests at play here. As mentioned above, disclosing whether AI developers train their models on data crawled from certain web domains will be highly relevant to publishers, as is information about how developers respect different opt-out signals for web data. Knowing whether a company uses data from a social media or email service they’re operating is important to users of those services to protect their privacy. Similarly, data protection advocates will have an interest in whether and how training datasets are anonymized and scrubbed of sensitive information.
At the same time, having more information about prospective sources of data can — without revealing the data itself — be valuable information for start-ups and challenger companies trying to identify and license high-quality training data. Fundamental rights and anti-discrimination watchdogs, on the other hand, may benefit from information about diversity and representativeness of training data, as well as data sources which may introduce harmful prejudice or potentially illegal content into the training data.
Finally, increased training data transparency will also greatly benefit public-interest research on AI. For example, researchers studying AI models’ propensity to “hallucinate” and spit out false information may benefit from information about the data range and subject areas covered by specific datasets. More transparency in this area can also shine a better light on how the characteristics and size of training datasets affect the behavior of the AI model itself, and based on what data this is assessed by companies. Other researchers, like those at the Data Provenance Initiative, could use information about companies’ web crawling practices to better study how current trends in AI training are eroding the web commons and undermining open sharing of content online.
Information about processing is crucial to understanding AI training data
To heed the spirit of the AI Act and provide those with legitimate interests with meaningful information, the summary of training data should not stop at simply listing data sources and datasets. Instead, it must also include a description of the (pre-)processing steps the data underwent. Processing decisions, such as data filtering or anonymization, can materially alter the composition of the training data and impact what models may return to any given prompt. For example, information on data filtering methods can provide insight into whether specific types or categories of data, such as personal data, have been removed from the training content. If data sets contain personal data, data subjects have several rights under the GDPR that they should be able to exercise, such as the rights to access and erasure.
Further, information on measures taken by developers to filter out (or not) toxic content, could at least partly help assess how they are seeking to prevent their models from generating harmful or discriminatory outputs. As such, transparency around the content used to train AI, coupled with information about how it was processed, can support the legitimate interests of preventing discrimination and respecting cultural diversity. Similarly, providing information about methods used for tokenization — that is, the process of breaking down raw data (such as words and sentences in natural language) into digestible units suitable for AI training — can help researchers assess potential linguistic biases.
Trade secrets can’t serve as a blanket excuse for intransparency
It is important to critically evaluate overbroad and misleading claims of trade secrecy. For example, a recent position paper by the Computer & Communications Industry Association (CCIA) argues in relation to the “sufficiently detailed summary” that “trade secrets and business confidential information of GPAI providers need to be safeguarded at all costs. [...] In such a highly dynamic market, publicly sharing the exact content used to train a GPAI model is very sensitive information that requires strict protection.” This argument misrepresents both EU trade secrets rules and the nature of the summary itself. First, as we argue below, in developing the template, the EU’s AI Office needs to weigh trade secrecy against potentially overriding public interests in line with the EU Trade Secrets Directive (TSD) rather than protecting trade secrecy “at all costs”. Second, the template was never meant to prescribe “sharing the exact content used to train a GPAI model” with the public. As is clear from the text of the AI Act, the template’s purpose is to help provide a sufficiently detailed summary of said data, not the data itself.
It thus becomes clear that a more nuanced discussion of trade secrets is necessary in the context of the AI Act’s training data transparency provisions. Vague references to trade secrets must not serve as a blanket excuse for intransparency. Instead, providers should substantiate their claims on a case-by-case basis for different categories of information (see Figure 1) to be made available through the template. Indeed, the presence of mere factual secrecy — i.e., the fact that information is not widely known — should not be conflated with sufficient reason to assume trade secrecy. It is a necessary, but not a sufficient condition. Further, as explained above, different legitimate interests should be taken into account. It is therefore worthwhile distinguishing between two steps in evaluating the legitimacy of trade secrecy claims: (1) assessing whether information addressed in the template would qualify as a trade secret at all and (2) whether there may be an overriding public interest warranting the disclosure of information protected by trade secret rules.
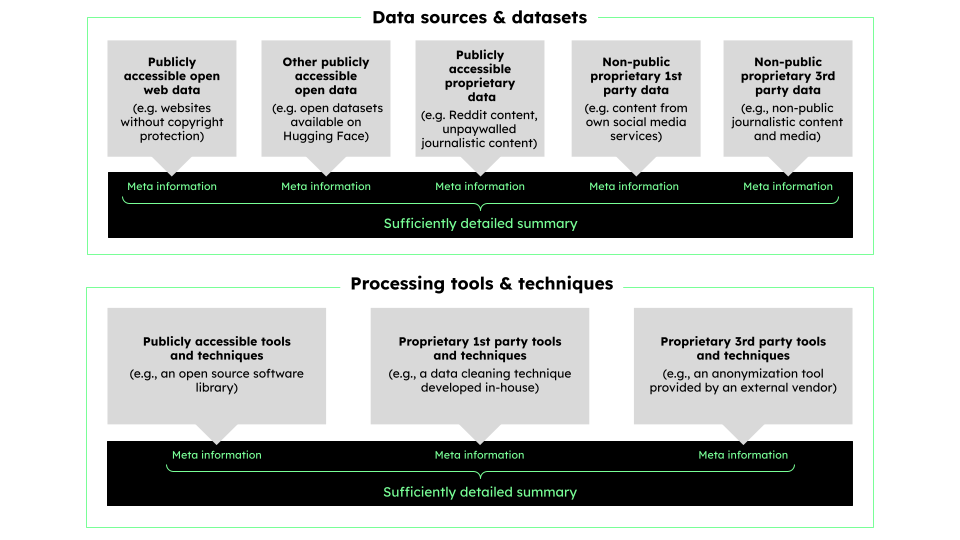
Figure 1.
First, it is necessary to assess whether information that may be disclosed through the sufficiently detailed summary meets the three cumulative criteria provided for in the TSD’s definition of trade secrets (Article 2(1)): i.e., that the information is secret; that it has commercial value because it is secret; and that reasonable steps are taken to keep it secret. While it is clear that providers go to great lengths to ensure the latter, whether the information in question is actually secret and whether commercial value is derived from this fact warrants a closer look.
In this context, it is important to distinguish between different categories of information that should be disclosed through a sufficiently detailed summary, as it is unlikely that all of these categories would be covered by trade secrets rules. For instance, training datasets composed of data downloaded from publicly accessible websites as well as datasets that can be downloaded from public repositories such as GitHub or Hugging Face arguably cannot be considered to be secret. The same is likely to apply to processing techniques and tools that are publicly available, such as open-source software libraries used to clean, filter, or anonymize data.
But even where data is indeed secret — for example, proprietary internal data — it does not necessarily follow that summary information about said data qualifies as a trade secret, or that commercial value is derived from such “meta” information. First, such summary information (as outlined in our blueprint for the template) does not allow others to reconstruct the data which is summarized. Rather, this summary provides value to legitimate interest holders while not itself adding to the value of the model which is trained on the data. It is important to keep in mind here that the sufficiently detailed summary does not mandate access to the training data itself. Second, while some of the information may hold value to a provider’s competitor, this does not apply to all of the information in the template. For example, it is unclear how much commercial value a competitor could derive from insights about anonymization techniques or from high-level information about the composition of the dataset without additional context. And even where, for example, detailed information about the data collection method may be protected, higher-level information about data collection or other “meta” information, for example about data sources, may not be.
Finally, even when information passes the test of the TSD and is deemed a trade secret, trade secrecy may still be outweighed by competing interests. In fact, Article 1(2)(b) of the TSD explicitly foresees the possibility of mandating disclosure of trade secrets by EU or member state law on public interest grounds. Recital 21 of the TSD also acknowledges that trade secrecy “should not jeopardize or undermine fundamental rights and freedoms or the public interest,” including concerns such as consumer and environmental protection. Disclosure of information pertaining to all legitimate interests, as mandated by the AI Act, is thus in line with EU trade secrets rules rather than in stark conflict, as claimed by some arguing the case against comprehensive transparency.
___
Time is tight for the EU’s AI Office, which aims to have a template for the “sufficiently detailed summary” ready before next summer. Yet getting this right is difficult and will require a true balancing act; it is indeed important to adequately consider the interests of all stakeholders. But this includes keeping in mind the breadth of interests that transparency around training data can further — and not giving credence to overbroad and misleading claims of trade secrecy that appear to be intended to obfuscate and undermine the purpose of the summary.
Related Reading
Authors
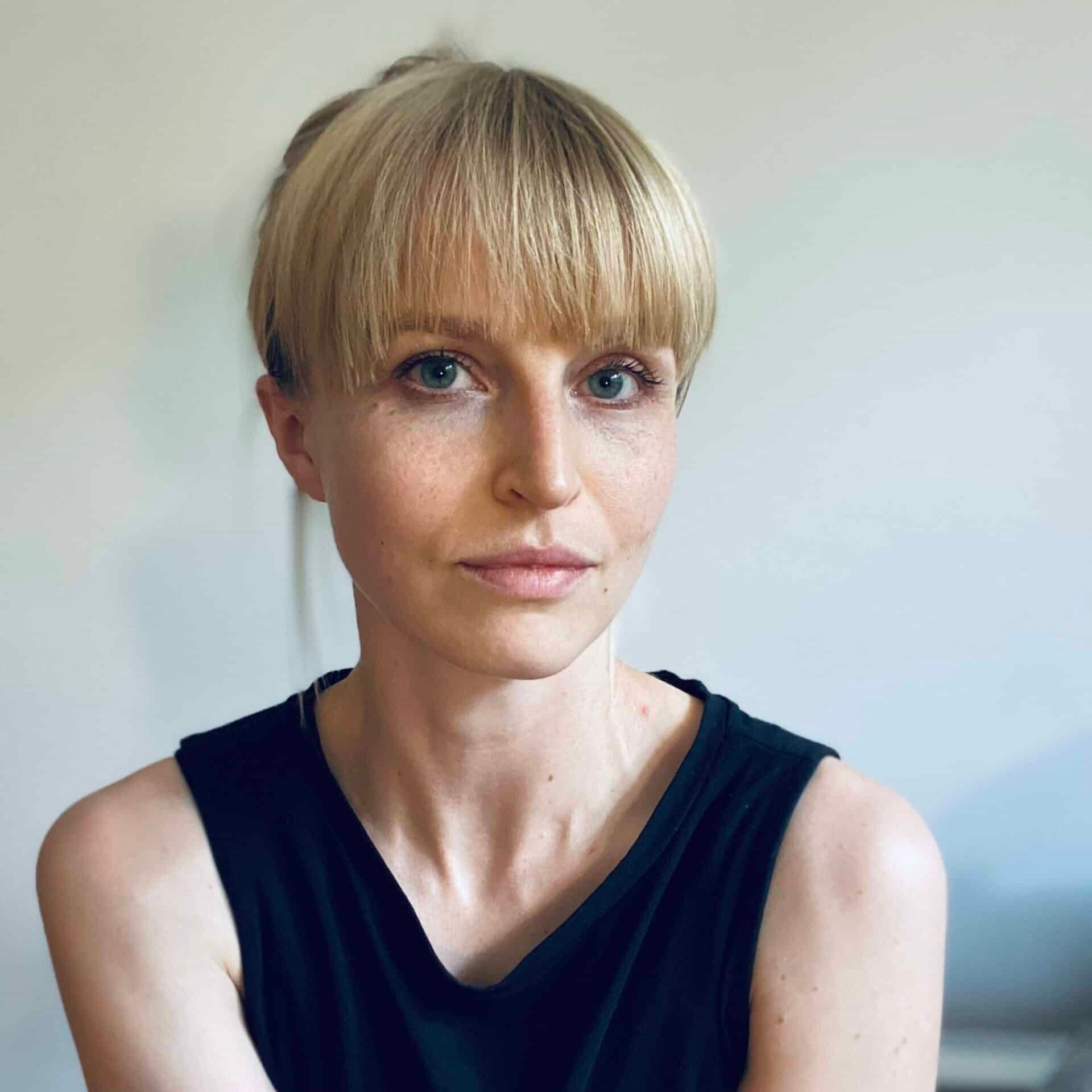
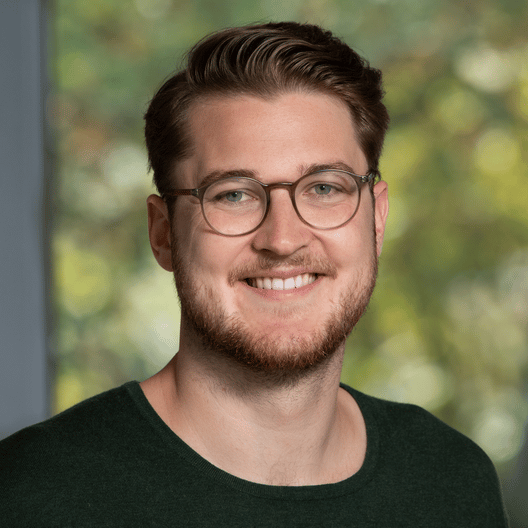